가능성과 불가피성: AI-관련 임상역량의 격차와 그것을 채울 필요성(Med Sci Educ. 2021)
The Potential and the Imperative: the Gap in AI‑Related Clinical Competencies and the Need to Close It
Kim V. Garvey1 · Kelly Jean Thomas Craig2 · Regina G. Russell3 · Laurie Novak4 · Don Moore5 · Anita M. Preininger6 · Gretchen P. Jackson2,6 · Bonnie M. Miller7
우리 삶에서 인공지능(AI)의 존재감이 커지면서 인간 상호 작용과 능력에 대한 이해가 활발히 변화하고 있습니다. AI가 강화된 디바이스의 도움으로 생활하고 일하는 방식은 우리가 세상을 경험하는 방식, 환경을 처리하고 상품을 소비하는 방식, 심지어 국가가 국가 안보 프로토콜과 지정학적 경계를 설정하는 방식까지 바꾸고 있습니다[1, 2].
The growing presence of artificial intelligence (AI) in our lives is actively transforming our understanding of human interaction and capability. Living and working with the aid of AI-enhanced devices are altering the way we experience our worlds, the way we process environments and consume goods, and even the way countries establish their national security protocols and geo-political boundaries [1, 2].
의료 분야에서는 전문가마다 AI를 구성하는 요소에 대해 의견이 다를 수 있습니다. 명확하게 하기 위해 우리는 AI를 의료진과 환자의 임상적 결정, 행동, 환경을 보강하기 위한 기술로 정의합니다. 이러한 기술은 예측 분석, 머신 러닝(ML), 자연어 처리(NLP) 등의 계산 수단을 통해 구현되며, 전자 의료 기록, 로봇과 같은 신기술 또는 개인 디바이스에서 구현될 수 있습니다. 예를 들면 위험 점수, 챗봇, 이미지 분석 등이 있습니다.
Within healthcare, experts may differ as to what constitutes AI. For purposes of clarity, we define AI as technologies that are intended to augment the clinical decisions, actions, and environments of healthcare workers and patients. These technologies are enabled by computational means such as predictive analytics, machine learning (ML), and natural language processing (NLP), and could be implemented in electronic health records, in new technologies such as robots, or in personal devices. Examples include risk scoring, chatbots, and image analysis.
특정 형태의 의료 AI는 수십 년 동안 사용되어 왔으며, 전문가 시스템 및 임상 의사 결정 지원 도구와 같은 애플리케이션은 1970년대 초에 개발되었습니다. [3, 4] 최근에는 정보 및 컴퓨팅 기술의 발전과 함께 대규모 데이터 및 컴퓨팅 리소스에 대한 액세스가 가능해지면서 혁신적인 잠재력을 지닌 강력한 세대의 AI를 개발할 수 있게 되었습니다. 토폴은 포괄적인 리뷰를 통해 이러한 차세대 AI 도구가 다양한 전문 분야에 걸쳐 환자, 의료 시스템, 임상의에게 어떤 영향을 미칠지 개괄적으로 설명했습니다[5]. 요컨대, 임상의는 자동화 및 로봇공학의 증가, ML 및 NLP에 대한 의존도 증가, 의료 IT 시스템, 개별 환자 및 인구집단에서 도출된 AI 인사이트의 통합을 포함하는 미래를 예상해야 합니다.
Certain forms of healthcare AI have been employed for decades; applications such as expert systems and clinical decision support tools were developed as early as the 1970s. [3, 4] More recently, advances in information and computing technologies, together with access to large-scale data and computational resources, have enabled the development of a powerful generation of AI forms with transformative potential. In his comprehensive review, Topol outlined the ways in which this new generation of AI tools will impact patients, health systems, and clinicians across a range of specialties [5]. In short, clinicians should anticipate a future that includes an increased presence of automation and robotics, more reliance on ML and NLP, and incorporation of AI-derived insights from health IT systems, individual patients, and populations.
잠재력, 의무, 그리고 격차
The Potential, the Imperative, and the Gap
의료 실무에서 AI 사용이 증가함에 따라 교육자, 의료 시스템 및 전문 조직은
- (1) 안전하고 환자 중심적인 사용에 필요한 역량 범위를 정의하고
- (2) 교육 연속체의 모든 단계에서 학습자를 위한 커리큘럼에 이러한 역량을 신중하게 통합해야 할 의무가 생겼습니다.
The increasing use of AI in healthcare practice creates an imperative for educators, health systems, and professional organizations to
- (1) define the range of competencies needed for safe and patient-centered use and
- (2) thoughtfully integrate these into curricula for learners across all phases of the educational continuum.
이미 많은 저자들이 의료 전문직 학습자에게 AI 기반 세계에서 실습에 대해 교육하기 위한 구조화된 접근 방식을 요구했습니다[6,7,8,9,10,11].
- 체계적 문헌고찰에서 Sapci와 Sapci는 의학교육자들이 AI 기술의 교육적 함의를 비판적으로 검토하기 시작했음을 보여주었습니다[9].
- Paranjape는 의료 전문직 학습자를 위해 시행된 다양한 이니셔티브를 설명하고 의학교육 연속체 전반에 걸쳐 프로그램에 포함되어야 하는 주제에 대한 권장 사항을 제시했습니다[12].
- James와 다른 사람들은 조정된 학습 접근법의 부재에 대한 우려를 공유하며 근거 기반 의학 커리큘럼을 모방할 모델로 사용할 것을 제안했습니다[13].
- 또한 윌저는 조직 리더가 이러한 신기술의 가능성과 위험성을 완전히 이해하고 새로운 평가 및 감독 역량을 개발하여 기술을 현명하게 도입하고 신중하게 모니터링할 수 있도록 해야 한다고 강조했습니다[14].
Numerous authors have already called for a structured approach to educating health professions learners about practicing in an AI-powered world [6,7,8,9,10,11]. In their systematic review, Sapci and Sapci showed that medical educators have begun to critically examine the educational implications of AI technologies [9]. Paranjape described a variety of initiatives that have been implemented for health professions learners and offered recommendations for topics that should be included in programs across the medical education continuum [12]. James and others shared concerns about the lack of coordinated learning approaches and suggested using evidence-based medicine curricula as a model to emulate [13]. In addition, Wiljer emphasized the need for organizational leaders to fully understand both the promise and the peril of these emerging technologies and to develop new evaluative and oversight capabilities so that technologies can be wisely introduced and carefully monitored [14].
6. Hodges BD. Ones and zeros: medical education and theory in the age of intelligent machines. Med Educ. 2020;54(8):691–3. https:// doi. org/ 10. 1111/ medu. 14149.
7. Masters K. Artificial intelligence in medical education. Med Teach. 2019;41(9):976–80. https:// doi. org/ 10. 1080/ 01421 59X. 2019. 15955 57.
8. Wartman S, Combs D. Medical Education must move from the information age to the age of artificial intelligence Acad Med 2018;93(8):1107-1109. https:// doi. org/ 10. 1097/ ACM. 00000 00000 002044
9. Sapci AH, Sapci HA. Artificial intelligence education and tools for medical and health informatics students: systematic review. JMIR Med Educ. 30 Jun 2020;6(1):e19285. https:// doi. org/ 10. 2196/ 19285
10. Alrassi J, Katsufrakis PJ, Chandran L. Technology can augment, but not replace, critical human skills needed for patient care. Acad Med. 2021;96(1):37–43. https:// doi. org/ 10. 1097/ ACM. 00000 00000 003733.
11. Harish V, Morgado F, Stern AD, Das S. Artificial intelligence and clinical decision making: the new nature of medical uncertainty. Acad Med. 2021;96(1):31–6. https:// doi. org/ 10. 1097/ ACM. 00000 00000 003707.
12. Paranjape K, Schinkel M, Nannan Panday R, Car J, Nanayakkara P. Introducing artificial intelligence training in medical education. JMIR Med Educ. 3 Dec 2019;5(2):e16048. https://d oi. org/10. 2196/ 16048
13. James CA, Wheelock KM, Woolliscroft JO. Machine learning: the next paradigm shift in medical education. Acad Med. 2021;96(7):954–7. https:// doi. org/ 10. 1097/ ACM. 00000 00000 003943.
사고 리더들에게는 분명 시급하지만, 실제 실무 환경에서는 체계적인 학습 접근법의 필요성이 쉽게 드러나지 않을 수 있는데, 이는 부분적으로는 우리 생활에 스마트 기기가 어디에나 존재하고 이러한 시스템이 어떻게 작동하는지에 대한 이해가 거의 없음에도 불구하고 이러한 시스템에 참여하는 위험한 경향이 있기 때문입니다. 예를 들어, 자동차는 본질적으로 바퀴 달린 복잡한 컴퓨터로, 대부분의 주말 수리공이 이해할 수 있는 수준을 훨씬 뛰어넘지만 우리는 운전을 합니다. 우리는 사회적 규범이나 규제 환경에 대한 암묵적 신뢰 때문에 난해한 기술을 무비판적으로 받아들이는 데 익숙해져 있습니다.
While undeniably urgent to thought leaders, the need for a systematic learning approach may not be as readily apparent in actual practice settings, in part because of the ubiquitous presence of smart devices in our lives and the perilous tendency to engage with these systems despite little understanding of how they work. For example, our cars are essentially complex computers on wheels, well beyond the comprehension of most weekend tinkerers, and yet we drive. We have become accustomed to uncritical acceptance of arcane technologies, perhaps because of social norms or tacit trust in a regulatory environment.
이러한 무비판적 수용 경향은 의료 환경으로 옮겨질 경우 위험할 수 있습니다.
- 우선, AI 도구와 같은 복잡한 시스템이 기존의 복잡한 의료 시스템에 도입되고 있는데, 각 시스템이 서로 다르기 때문에 의도하지 않은 결과가 발생할 가능성이 높아집니다.
- 또한 특정 AI 도구에 대한 암묵적인 신뢰는 시기상조일 수 있습니다. 미국 식품의약국(FDA)은 거의 모든 새로운 의료 기기에 대해 엄격한 승인 절차를 거치지만, 의료 기기로서의 AI/ML 기반 소프트웨어는 아직 공식화 중인 다른 위험 등급별 규제 프로세스를 필요로 합니다[15].
- 마지막으로, AI는 이전 세대의 의료 기술과는 다른 특징을 가지고 있습니다. AI는 인간과 유사한 지능을 제공하도록 설계될 수 있기 때문에 도구라기보다는 사고의 동반자로 여겨지며, 따라서 헌신적이고 지속적인 훈련의 필요성이 훨씬 덜 분명해집니다.
This tendency towards uncritical adoption could be dangerous if transferred into healthcare settings.
- For one, complex systems, such as AI tools, are being introduced into the enormous pre-existing complexity of healthcare systems, each one different from the others, increasing the potential for idiosyncratic unintended consequences.
- In addition, tacit trust in any specific AI tool may be premature. Although the US Food & Drug Administration puts nearly all new medical devices through a rigorous approval process, AI/ML-based Software as a Medical Device will require a different set of risk-stratified regulatory processes that are still being formulated [15].
- Finally, AI presents differently than prior generations of healthcare technologies. Because it can be designed to offer human-like intelligence, AI appears to be less of a tool and more of a thinking companion, making the need for dedicated and ongoing training even less apparent.
이러한 우려의 맥락에서, 저희는 임상 환경에서 AI를 사용하는 데 필요한 의료진의 역량에 관한 출판된 연구를 확인하기 위해 범위 검토를 실시했습니다. 2009년 1월 1일부터 2020년 5월 1일 사이에 MEDLINE, CINAHL, Cochrane Library 데이터베이스에서 발표된 문헌은 의료 전문가(예: 의료, 간호, 약학) 및 모든 교육 단계에서의 전문성 개발, 모든 임상 진료 환경에서의 AI 기반 도구, 전문 교육 역량 영역 또는 성과 측정(예: 의학전문대학원교육인증위원회[ACGME] 핵심 역량) 관련 용어 검색 쿼리를 사용하여 선별되었습니다. 영어, 사람을 대상으로 한 연구, 초록 유무, 미국(미국) 환경에 대한 제한이 있었습니다.
In the context of these concerns, we conducted a scoping review to identify published research on provider competencies needed for use of AI in clinical settings. Literature published between January 1, 2009, and May 1, 2020, from MEDLINE, CINAHL, and the Cochrane Library databases was curated using search queries for terms related to healthcare professionals (e.g., medical, nursing, and pharmacy) and their professional development in all phases of education; AI-based tools in all settings of clinical practice; and professional education competency domains or performance measures (e.g., Accreditation Council for Graduate Medical Education [ACGME] core competencies). Limits were provided for English language, studies in humans, presence of abstracts, and United States (US) settings.
문헌 검색 결과 3,476건의 고유 인용이 이루어졌으며, 이 중 109건의 논문이 전체 텍스트 심사 대상에 포함되었습니다. 전체 텍스트 심사 결과, 새로운 AI 시스템을 구현할 때 입증된 임상의 역량에 대해 보고한 연구는 4편에 불과했습니다.[16,17,18,19] 관찰된 역량은 ACGME 환자 치료 및 대인관계 및 의사소통 기술 영역에 속했습니다[20]. 이들 논문 중 임상의가 필요로 할 수 있는 새로운 AI 관련 역량을 설명한 논문은 없었으며, 구현 전 교육에 대해 언급한 논문은 단 한 편에 불과했습니다. 임상의가 개입의 성격, 개발 방법, 기존 워크플로우에 어떻게 통합해야 하는지에 대해 설명한 연구는 하나도 없었습니다. 검토를 위해 처음에 확인된 연구의 양은 의료 분야에서 AI의 성장에 대한 예측을 확인시켜 주며, 새롭거나 향상된 전문 역량을 설명하는 기사의 부족은 효과적인 사용에 필요한 역량에 대한 지식의 현재 격차에 대한 우려를 확인시켜 줍니다.
Literature searches yielded 3,476 unique citations, of which 109 articles were eligible for full-text screening. Upon full-text screening, only four studies reported on clinician competencies demonstrated in implementing the new AI systems.[16,17,18,19] The observed competencies fell within the ACGME Patient Care and Interpersonal and Communication Skills domains [20]. None of these articles described any new AI-specific competencies clinicians might need and only one mentioned any form of pre-implementation training. None of the studies indicated what clinicians were told about the nature of the interventions, how they were developed, or how they should be incorporated into pre-existing workflows. The volume of studies initially identified for review confirms predictions about the growth of AI in healthcare, and the paucity of articles describing new or enhanced professional competencies affirms concerns about the current gap in knowledge about the competencies required for their effective use.
주의 사례
Cautionary Tales
필요한 역량을 부여하기 위한 체계적인 접근 방식 없이 새로운 기술을 도입할 때 발생할 수 있는 피해를 보여주는 임상 의학의 몇 가지 역사적 사례를 소개합니다. 이러한 시나리오는 AI 도구의 사용과 직관적으로 유사하지는 않지만 일반화할 수 있는 시사점을 담고 있습니다.
We offer several historical examples from clinical medicine that illustrate the harm that can result when new technologies are introduced without structured approaches to imparting the required competencies. While not intuitively analogous to the use of AI tools, these scenarios have generalizable implications.
전기 수술
Electrosurgery
한 세기가 넘는 기간 동안 외과의들은 수술 중 출혈을 보다 신속하고 완벽하게 제어하기 위해 전기 수술 장치를 사용해 왔습니다. 수련 중인 외과의사들은 일상적인 상황에서 이러한 기기를 사용하는 방법을 보편적으로 배웠지만, 기기의 기본 물리학이나 비정상적이거나 예상치 못한 상황이 발생할 때 발생할 수 있는 위험을 설명하는 공식적인 커리큘럼은 없었습니다. 디바이스 제조업체는 적절한 사용법을 설명하는 정보를 제공했지만, 이러한 리소스에 대한 접근이 거의 이루어지지 않았고 다양한 환경과 디바이스가 사용되는 전체 시스템의 복잡성을 고려하지 않았습니다. 이로 인한 지식 부족은 20세기 후반까지 심각한 환자 화상과 심지어 수술실 화재의 원인이 되었습니다[21]. 2012년 말, 미국 위장관 내시경 외과의사 협회(SAGES)-수술 에너지의 기본적 사용(FUSE) 위원회는 "외과의사들이 수술 시 에너지의 안전한 사용에 대해 무엇을 모르는지 모른다"고 말했습니다[22].
For over a century, surgeons have used electrosurgical devices to more rapidly and completely control intra-operative bleeding. While surgeons-in-training universally learned how to use these devices in routine situations, there were no formal curricula that explained the basic physics of the devices, or the risks that can emerge when unusual or unexpected circumstances arise. Device manufacturers provided information describing appropriate use, but these resources were rarely accessed and did not account for the variety of environments and the complexity of the overall systems in which these devices were employed. The resulting knowledge deficit contributed to serious patient burns and even operating room fires well into the latter decades of the twentieth century [21]. As late as 2012, the Society of American Gastrointestinal Endoscopic Surgeons (SAGES)-Fundamental Use of Surgical Energy (FUSE) Committee stated that “surgeons don’t know what they don’t know about the safe use of energy in surgery” [22].
복강경 담낭 절제술
Laparoscopic Cholecystectomy
복강경 담낭절제술은 1980년대 후반에 일반 외과계에 도입되었으며, 환자 선호도가 높았기 때문에 대규모 연구를 통해 결과와 위험성이 결정되고 새로운 기술에 대한 최적의 교육 과정이 개발되기 전에 빠르게 자리를 잡았습니다. 경험이 축적되면서 일반적인 담관 손상이 크게 증가하는 것으로 나타났습니다. 이 심각한 합병증의 위험은 상대적으로 낮았지만 미국에서만 매년 약 75만 건의 담낭절제술이 시행되었지만 절대적인 영향은 놀라웠고, 결국 외과계는 포괄적인 교육과 지속적인 절차 개선의 필요성을 인식하게 되었습니다[23].
Laparoscopic cholecystectomy was introduced to the general surgery community in the late 1980s, and because of strong patient preference, the procedure rapidly took hold before large-scale studies determined outcomes and risks, and before optimal training processes for the new technical skills were developed. As experience accumulated, a significant increase in common bile duct injuries was noted. Even though the risk of this serious complication remained relatively low, with nearly 750,000 cholecystectomies performed annually in the US alone, the absolute impact was alarming, and the surgical community eventually recognized the need for comprehensive training and ongoing refinement of the procedure [23].
소화성 궤양 질환
Peptic Ulcer Disease
1984년 워렌과 마샬은 소화성 궤양이 위산 분비의 일차적 변화가 아니라 헬리코박터 파일로리(H. pylori) 박테리아에 의해 발생한다는 강력한 증거를 제시했습니다[24]. 그럼에도 불구하고 임상의들이 치료용 항생제 치료를 일관되게 권장하기까지 거의 20년이 지났지만, 이를 뒷받침하는 연구가 증가하고 수많은 가이드라인과 권고안이 발표되었습니다[25]. 임상의들은 질병 개념의 근본적인 변화를 정당화하기 위해 추가적인 증거가 필요하다고 주장하는 것은 당연한 일이었지만, 이러한 장기간의 지연은 정서적 역량에 대한 보다 일반적인 결핍을 반영하는 것이기도 했습니다. 새로운 질병 패러다임은 의사의 자율성, 신념, 진료 습관, 전문 분야 문화, 경우에 따라서는 금전적 이해관계 등 새로운 기술의 도입을 방해할 수 있는 모든 강력한 태도적 요인에 도전했습니다. 그 결과 많은 환자들이 예방 가능한 궤양 재발, 불필요한 수술 및 기타 합병증으로 인해 피해를 입었습니다.
In 1984, Warren and Marshall provided strong evidence that peptic ulcers are caused by the bacterium Helicobacter pylori (H. pylori) and not primary alterations in gastric acid secretion [24]. Nonetheless, nearly two decades passed before clinicians consistently recommended treatment with curative antibiotics, despite a growing body of supportive research and the publication of numerous guidelines and recommendations [25]. While clinicians were within reason to insist that additional evidence was needed to justify such a profound change in disease conceptualization, this prolonged delay also reflected a more general deficiency in affective competencies. The new disease paradigm challenged physician autonomy, beliefs, practice habits, specialty cultures, and, in some cases, financial interests, all strong attitudinal factors that can impede the adoption of new technologies. As a result, many patients suffered harm from preventable ulcer recurrences, unnecessary operations, and other complications.
AI 도구와 임상 기술은 서로 다른 것처럼 보일 수 있지만, 체계적인 분석과 훈련이 없는 상태에서 강력한 새 기능을 도입할 때의 위험은 비슷합니다. 또한 한 번에 한 명의 환자에게 부상이 발생하는 외과 수술과는 달리, AI 도구의 뛰어난 확장성은 위험의 복합화로 이어집니다[5, 26].
While AI tools and clinical technologies may seem dissimilar, the risks of introducing powerful new capabilities in the absence of systematic analysis and training are analogous. And as opposed to surgical operations where the injury occurs one patient at a time, the great scalability of AI tools also leads to the compounding of risk [5, 26].
전기 수술의 경우와 마찬가지로 임상의는 AI 도구를 사용하기 전에 해당 도구가 적절한 환자 집단에 의도한 목적에 맞게 구현되고 채택되었는지 확인하기 위해 AI 도구에 대해 "알아야 할 사항"을 파악해야 합니다[27]. 예를 들어, 만성 질환 결과를 개선하기 위해 개인 맞춤형 치료의 혜택을 받을 수 있는 환자를 예측하기 위해 미국에서 널리 사용되는 위험 점수 알고리즘은 체계적으로 흑인 환자에게 백인 환자보다 낮은 점수를 할당했습니다. 분석 결과, 이 도구는 질병의 중증도를 나타내는 지표로 의료비 지출을 사용했으며, 과거 비용을 통해 미래 비용을 예측할 수는 있었지만 구조적 불평등으로 인해 흑인 환자의 의료 서비스 이용률이 낮은 것은 고려하지 않았습니다. 그 결과, 흑인 환자들은 실제 질병 상태보다 낮은 비율로 만성 질환 관리 프로그램에 등록되었습니다[28].
Just as in the case of electrosurgery, clinicians need to “know what they need to know” about AI tools before using them to ensure that they are being implemented and adopted for the intended purpose on an appropriate patient population [27]. For example, a risk scoring algorithm widely used in the US to predict which patients would benefit from personalized care to improve chronic illness outcomes systematically assigned Black patients lower scores than White patients. Analysis showed that the tool used healthcare expenditures as a proxy for severity of illness, and while past costs did predict future costs, the tool did not account for lower utilization of healthcare services by Black patients as the result of structural inequalities. Subsequently, Black patients were enrolled in these chronic disease management programs at lower rates than indicated by their actual disease status [28].
복강경 담낭 절제술의 경험과 유사하게, AI 기반 의사 결정 지원 시스템의 초기 경험은 이를 사용하는 의료 시스템이 비용, 구현 과제 및 한계를 완전히 인식하지 못할 경우 발생할 수 있는 잠재적 피해를 보여주었습니다[5, 29, 30]. 예를 들어, 유방조영술 판독을 위한 컴퓨터 보조 진단(CAD) 도구는 2000년대 초반에 환자, 인구, 의료 시스템에 대한 이점과 위험이 분석에 의해 입증되기 전에 빠르게 채택되었습니다. 이러한 분석 결과, CAD는 위양성 판독을 증가시키는 반면 특이도와 전반적인 진단 정확도는 감소시키는 것으로 밝혀졌습니다. 저자들은 환자의 불안, 불편, 피해에 대한 영향 외에도 추가 영상 검사 및 생검의 증가로 인해 연간 55만 달러(미화)의 초과 비용이 발생할 수 있다고 추정했습니다[31]. 최신 세대의 CAD 도구는 입력과 결과가 발생함에 따라 학습하고 적응할 수 있지만, 이점이 위험을 능가하는지 확인하기 위해서는 면밀한 모니터링이 여전히 필수적입니다[15, 27].
Similar to the experience with laparoscopic cholecystectomy, early experience with AI-based decision support systems demonstrated the potential harms that can result if health systems using them are not fully aware of the costs, implementation challenges, and limitations [5, 29, 30]. For example, computer-assisted diagnosis (CAD) tools for interpretation of mammography were quickly adopted in the early 2000s before analysis demonstrated their benefits and risks for patients, populations, and health systems. When such analysis was performed, CAD was found to increase false positive readings while decreasing specificity and overall diagnostic accuracy. In addition to the impact on patient anxiety, inconvenience and harm, the authors estimated that the increase in additional imaging studies and biopsies could lead to excess costs of $550,000 (US dollars) per year [31]. Newer generations of CAD tools have the ability to learn and adapt as inputs and outcomes accrue, but close monitoring remains essential to ensure that benefits outweigh the risks [15, 27].
헬리코박터 파일로리의 사례와 유사하게, 전문가들은 검증된 AI 도구의 도입이 늦어지면 환자, 임상의, 의료 시스템이 잠재적 혜택을 누리지 못할 것이라고 우려하고 있습니다. 이러한 주저는 기반 과학에 대한 유사한 회의론과 전문가의 자율성, 진료 패턴 및 재정적 영향에 대한 유사한 우려에서 비롯될 수 있습니다[32].
Similar to the experience with H. pylori, experts are concerned that slow adoption of validated AI tools will prevent patients, clinicians, and health systems from reaping their potential benefits. Hesitation may stem from similar skepticism about the underlying science and similar concerns about professional autonomy, practice patterns and financial impacts [32].
반성
Reflections
이러한 역사적 사례는 발생할 수 있는 문제의 유형뿐만 아니라 이를 해결하고 예방하는 데 있어 구조화된 학습 접근법의 가치를 조명합니다.
These historical examples shed light not only on the types of problems that can arise but also on the value of structured learning approaches in addressing and preventing them.
복강경 담낭절제술의 경우 담관 손상 위험은 집도의의 경험과 관련이 있다는 연구 결과가 발표되었습니다. 이에 따라 SAGES의 지도자들은 전문가 위원회를 구성하여 복강경 수술 접근법의 안전한 사용에 필요한 역량을 포괄하는 포괄적인 교육 프로그램을 개발하도록 했습니다. 복강경 수술의 기초(FLS) 위원회는 처음에 내용을 정의하고, 더 광범위한 SAGES 커뮤니티 내에서 검토한 다음, 커리큘럼을 만들기 위해 내용을 다듬었습니다. 심리측정학자들은 지식과 기술을 모두 포괄하는 시험을 설계하고 평가하는 데 도움을 주었으며, FLS 커리큘럼과 시험은 이제 미국 외과학회의 인증에 필수입니다[33]. 시술에 대한 이해도가 높아지고 새로운 역량을 심어주는 공식적인 교육 접근 방식이 도입되면서 담관 손상 발생률이 감소했지만, 기존의 개복 시술에서 볼 수 있는 낮은 수준까지는 아니며, 이는 위험의 일부가 경험 이외의 요인에 기인한다는 것을 의미합니다[23]. 이제 환자들은 동의 절차의 일부로 이러한 위험에 대해 일상적으로 고지받지만, 빠른 회복, 통증 감소, 입원 기간 단축으로 인해 이점이 위험보다 훨씬 더 큽니다.
In the case of laparoscopic cholecystectomy, studies demonstrated that risk of bile duct injury was associated with a surgeon’s experience. In response, the leaders of SAGES assembled an expert committee and charged it with developing a comprehensive educational program covering the competencies needed for the safe use of laparoscopic surgical approaches. The Fundamentals of Laparoscopic Surgery (FLS) committee initially defined the content, vetted it within the broader SAGES community, and then refined it to create the curriculum. Psychometricians helped design and evaluate an examination covering both knowledge and technical skills, and the FLS curriculum and examination are now required for certification by the American Board of Surgery [33]. With better understanding of the procedure and formal training approaches that instilled the new competencies, the incidence of bile duct injuries has diminished, although not to the lower levels seen with traditional open procedures, meaning that some part of the risk is attributable to factors other than experience [23]. Patients are now routinely informed of this risk as part of the consent process, but because of the rapid recovery, diminished pain, and shorter hospitalization, the benefits far outweigh the risk.
마찬가지로 전기 수술 사용에 대한 부적절한 교육으로 인한 피해를 해결하기 위해 SAGES는 외과 의사, 마취과 의사, 간호사, 엔지니어로 구성된 다분야 전문가 그룹을 구성하여 전기 수술 기기의 안전한 사용을 위한 역량을 정의했습니다. 초기 역량 목록은 SAGES의 리더들이 검토했으며, 심리측정 전문가들이 타당하고 신뢰할 수 있는 시험 개발을 도왔습니다. 그 결과, 사용자가 다양한 실제 조건에서 전기수술 기기를 안전하게 작동하는 데 필요한 지식과 기술을 갖출 수 있도록 하는 수술 에너지의 기본적 사용(FUSE) 커리큘럼이 개발되었습니다[34].
Similarly, to address the harms resulting from inadequate training in the use of electrosurgery, SAGES assembled a multidisciplinary group of surgeons, anesthesiologists, nurses, and engineers, who defined the competencies for safe use of electrosurgical devices. The initial list of competencies was reviewed by the leaders of SAGES and psychometricians helped develop a valid and reliable examination. The resulting Fundamental Use of Surgical Energy (FUSE) curriculum ensures that users have the requisite knowledge and skills for safe operation of electrosurgical devices under a variety of real-world conditions [34].
인공지능 도구의 채택이 지연되는 기저에 있는 정서적(사회적, 문화적) 요인은 최적의 사용을 촉진하는 인지적, 기술적 요인보다 구조화된 커리큘럼으로 해결하기 어려울 수 있습니다. 헬리코박터 파일로리의 경우, 미국 질병통제예방센터와 국립보건원은 결국 환자뿐만 아니라 임상의를 대상으로 한 교육 및 마케팅 자료를 만들어 변화를 이끌어냈습니다[25]. 일반적으로 지식과 기술이 계속해서 빠른 속도로 성장하고 발전함에 따라 임상의는 진료 패턴을 위협하거나 방해할 수 있는 새로운 역량이 개발될 것이라는 사실을 자신의 직업적 정체성의 일부로 통합해야 하며, 심지어 정체성 자체의 측면까지도 통합해야 합니다. 과학적 회의론은 결코 사라져서는 안 되지만, 부정과 저항보다는 호기심과 학습으로 전환해야 합니다.
The affective (social and cultural) factors underlying delayed adoption of Ai tools may be more difficult to address with structured curricula than the cognitive and technical factors that will promote optimal use. In the case of H. pylori, the US Centers for Disease Control and Prevention and the National Institutes of Health eventually created educational and marketing materials aimed at patients as well as clinicians to help bring about a shift [25]. In general, as knowledge and technology continue to grow and evolve at accelerated rates, clinicians should incorporate as part of their professional identity the fact that new capabilities will be developed over the course of their careers that might threaten or disrupt practice patterns and even aspects of that identity itself. While scientific skepticism should never go away, it should be channeled into curiosity and learning rather than denial and resistance.
향후 방향
Future Directions
모든 유형의 의료 전문가는 진화하는 AI-증강 기술과 임상 치료의 인터페이스 내에서 성공하기 위한 역량을 명확히 하고, 측정하고, 반복해야 하는 교육적 의무가 있습니다. 새로운 기술이 도입될 때와 마찬가지로, 이러한 역량은 임상의사에게나 가장 주니어 학습자에게나 똑같이 관련성이 있을 것입니다. 필요한 AI 기술 중 상당수는 사용 중인 개입에 따라 달라질 것이며, 도구가 도입됨에 따라 적시 교육이 필요할 것입니다. 그러나 한 가지 AI 도구나 지능형 지원 유형만을 사용하는 데 필요한 기술보다 훨씬 더 광범위한 역량에 대한 질문이 있으며[35], 모든 의료 전문가는 새로운 테크놀로지가 구현될 때 알아야 할 사항을 학습하기 위한 일반적인 접근 방식을 개발해야 합니다.
Healthcare professionals of all types have the educational imperative to articulate, measure, and iterate competencies for thriving within this evolving interface of AI-augmented technology and clinical care. As is the case when any new class of technology is implemented, these competencies will be just as relevant to practicing clinicians as they will be to the most junior learners. Many of the required AI skills will be specific for the intervention being employed and will necessitate just-in-time training as tools are introduced. However, there are questions of competence much broader that the skills needed for use of any one AI tool or type of intelligent support alone [35], and all health professionals should develop a general approach for learning what they need to know about new technologies as they are being implemented.
임상 AI 도입에는 새로운 종류의 윤리적 문제가 수반되며, 임상의 역량 목록에는 이러한 문제를 예측하고 해결하기 위한 전략이 포함되어야 합니다. 예를 들어,
- 어떤 상황에서 환자가 의사 대신 AI 기반 로봇으로부터 진단을 받는 것이 적절할까요?
- 사전 동의는 언제 필요하며 어떤 정보를 포함해야 할까요?
- 기계 학습과 인간 학습 프로세스 간에 제한된 리소스를 어떻게 할당해야 하며, AI는 비용과 고급 인프라의 필요성 때문에 리소스가 풍부한 환경과 리소스가 부족한 환경 간의 격차를 확대할 것인가요?
- AI 도구가 편향된 데이터 세트로부터 학습하도록 허용되어야 할까요?
- 임상 데이터의 잠재적 편향성과 그에 따른 추천은 어떻게 평가하고 관리할 것인가?
- 개인정보 보호의 필요성과 투명성의 필요성은 어떻게 균형을 이룰 수 있을까요? [5, 36].
A new class of ethical challenges accompanies the introduction of clinical AI and any list of clinician competencies should include strategies for anticipating and addressing them. For example,
- in what circumstances will it be appropriate for a patient to receive a diagnosis from an AI-powered robot instead of a physician?
- When will informed consent be necessary and what information should be included?
- How should limited resources be allocated between machine learning and human learning processes, and will AI widen the gap between resource-rich and resource-poor settings because of its expense and the need for advanced infrastructure?
- Should AI tools be permitted to learn from biased datasets?
- How will potential biases in clinical data and resulting recommendations be evaluated and managed?
- How will the need for privacy be balanced with the need for transparency? [5, 36].
임상 환경에서 AI 사용을 연구하는 연구자가 [도구를 효과적으로 사용하는 데 필요한 교육], 특히 모든 연구 설계 및 보고서에서 [역량에 대해 설명하는 것]은 큰 이점을 창출할 수 있는 또 다른 간단한 단계입니다. 새로운 AI 도구의 구현 매트릭스에 전문 학습을 의도적으로 포함시키는 것은 도구 배포에서 최적의 결과를 달성하는 데 필수적입니다. 이는 보건 전문직 교육자와 이러한 도구를 개발, 테스트 및 구현하는 사람들 간의 새로운 파트너십을 의미합니다.
Another simple step that could generate great benefit would be for researchers who study the use of AI in clinical settings to describe the education necessary for effective use of the tool, specifically addressing competencies in all study designs and reports. Deliberately including professional learning in the implementation matrix for new AI tools will be essential for achieving optimal outcomes in their deployment. This will mean new partnerships between health professions educators and those who develop, test, and implement these tools.
AI 도구[30, 37] 사용을 위한 권장 전문 역량 개발의 필요성을 해결하기 위해, 우리는 보건 전문직 교육 및 AI 분야의 사고 리더들과 구조화된 인터뷰를 실시하여 시뮬레이션 및 실제 업무 환경 모두에서 테스트하고 개선할 수 있는 역량 프레임워크를 개발할 것입니다. 이러한 리더는 출판된 저술과 두 분야의 전문가 추천을 통해 선정됩니다. 이 프레임워크는 ACGME 핵심 역량 프레임워크에 기반할 수 있으며, 다음 등을 포함할 수 있습니다.
- AI 유형에 대한 기본 지식,
- 환자별 정보에 비추어 인구 기반 추정치를 변경하기 위해 베이지 정리를 사용하는 능력,
- 환자와 동료에게 확률을 명확하게 전달하는 능력,
- 특정 환경에 적합한 AI 도구를 결정하고 이에 액세스하는 방법,
- 환자의 목표와 가치가 AI 기반 추천을 어떻게 수정해야 하는지 공감적으로 식별하는 능력
이상적으로, 이러한 역량은 임상의와 임상 치료에 대한 연민, 인간성, 신뢰를 유지하거나 강화하는 [공유 의사결정 모델]에서 AI의 신중한 사용을 촉진해야 합니다[10, 38]. 그러나 이러한 이상을 달성하기 위해서는 의료 시스템과 개인 모두의 의도적인 노력이 필요합니다. 우연히 이루어지지 않을 것입니다.
To help address the need for the development of recommended professional competencies for the use of AI tools [30, 37], we will conduct structured interviews with thought leaders in health professions education and in AI to develop a competency framework that subsequently can be tested and refined within both simulated and authentic workplace experiences. These leaders will be identified through their published writings and by recommendation of experts in both fields. The framework could be based on the ACGME core competency framework and might include
- basic knowledge about the types of AI;
- the use of Bayes’ theorem to alter population-based estimates in light of patient-specific information;
- the ability to clearly communicate probabilities to patients and colleagues;
- the ability to determine which AI tools are relevant in specific settings and then how to access them; and
- the ability to empathically discern how patient goals and values should modify AI-driven recommendations.
Ideally, these competencies should promote the thoughtful use of AI in shared decision-making models that sustain or even enhance compassion, humanity, and trust in clinicians and clinical care [10, 38]. However, intentional effort on the part of both health systems and individuals will be required to achieve this ideal. It will not happen by chance.
궁극적으로 시스템적 사고, 비판적 사고, 실용적 지혜와 같은 [통합적 역량]이 AI 활용에 필요할 수 있습니다. 이러한 기술은 환자 중심의 인본주의적 치료의 기본이며 전문 임상의가 수년간의 교육과 감독을 통해 개발합니다. 한 사고 리더는 "AI는 인공적이지도, 지능적이지도 않으며"[39] 현명하거나 비판적인 사고 능력을 보여주지 않는다고 주장합니다. 판단력과 분별력은 여전히 인간에게서 나와야 합니다. 두려움과 대중의 통념과는 달리, AI는 인간의 사고를 대체할 가능성이 높지 않으며, 오히려 이를 보강하는 데 사용될 가능성이 높습니다. AI의 힘과 잠재력을 활용하려면 AI가 없을 때와 마찬가지로, 어쩌면 훨씬 더 잘 생각해야 할 것입니다. 그렇지 않으면 기계와 인간이 "함께 더 똑똑해지고 멍청해질 수 있다"는 노먼의 두려움이 현실화될 위험이 있습니다(그림 1 [40]).
Ultimately, there may be integrative competencies for the use of AI; for example, systems thinking, critical thinking, and practical wisdom. These skills are foundational to patient-centered, humanistic care and are developed by professional clinicians over years of training and supervised practice. One thought leader argues that “AI is neither artificial, nor intelligent”[39] and it certainly does not exhibit wise or critical thinking capacities. Judgement and discernment will still need to come from humans. Contrary to fears and popular mythology, AI is not likely to supplant human thinking, but more likely will be used to augment it. This will require that we think just as much—and perhaps much better—than we do without AI, if we are to reach its power and potential. If not, we run the risk of realizing Norman’s fear that machines and humans may become “smarter and dumber together” Fig. 1 [40].
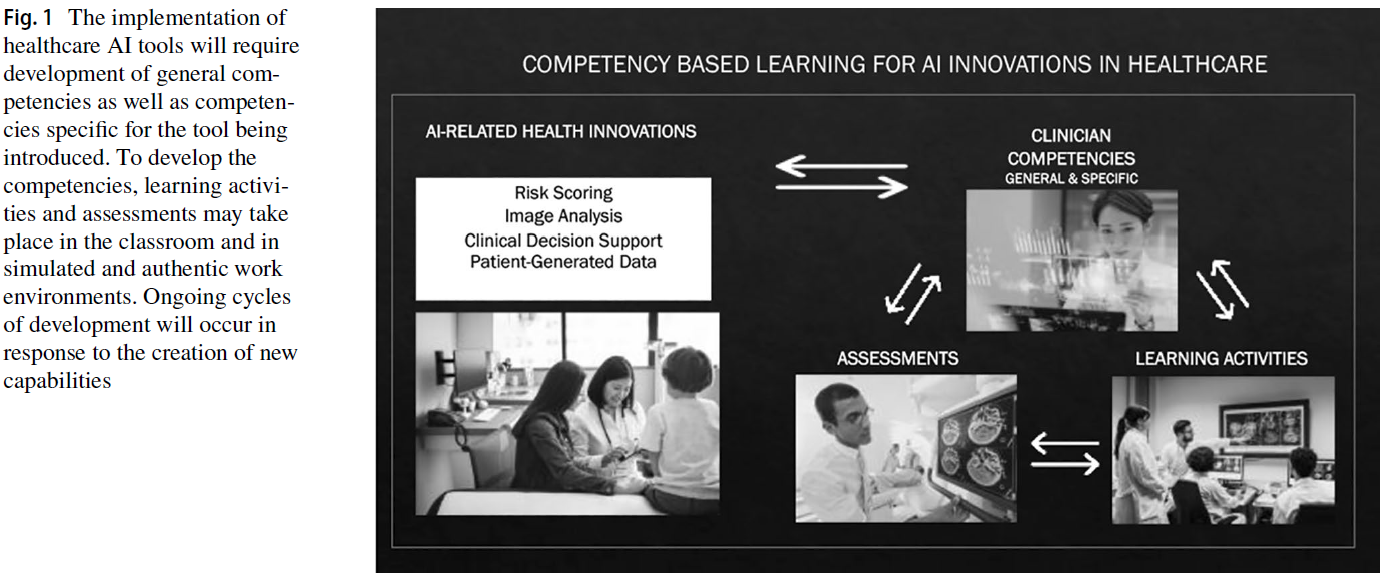
The Potential and the Imperative: the Gap in AI-Related Clinical Competencies and the Need to Close It
PMID: 34956712
PMCID: PMC8651813
DOI: 10.1007/s40670-021-01377-w
Free PMC article
'Articles (Medical Education) > 임상교육(Clerkship & Residency)' 카테고리의 다른 글
키워드 특이적 알고리듬으로 발전 문제가 있는 전공의 찾아내기 (J Grad Med Educ. 2019) (0) | 2023.07.16 |
---|---|
임상진료상황에서 능숙한 의사소통가의 특징 식별하기(Med Educ, 2022) (0) | 2023.06.30 |
구름낀 푸른하늘: 의대생-전공의 이행에서의 지속적 긴장을 식별하고 미래의 이상적 상태 그리기(Acad Med, 2023) (0) | 2023.05.14 |
임상추론 교육을 위한 세 가지 지식-지향 교수전략: 자기설명, 개념매핑연습, 의도적 성찰: AMEE Guide No. 150 (Med Teach, 2022) (0) | 2023.05.11 |
임상적 의사소통의 생각, 우려, 기대에 대한 비판적 고찰(Med Educ, 2022) (0) | 2023.04.23 |