의과대학생이 인공지능에 대해서 실제로 알아야 하는 것은? (NPJ Digit Med. 2020)
What do medical students actually need to know about artificial intelligence?
Liam G. McCoy 1,2 , Sujay Nagaraj 1,3, Felipe Morgado 1,4, Vinyas Harish 1,2, Sunit Das1,5 and Leo Anthony Celi 6,7,8
소개
Introduction
인공지능(AI)의 새로운 혁신이 의료 현장에 큰 영향을 미칠 것으로 예상됨에 따라 현재 및 미래의 의사를 대상으로 AI에 대한 교육에 대한 관심이 높아지고 있습니다1. 이러한 관심과 함께 의대생이 정확히 무엇을 배워야 하는지에 대한 질문도 제기되고 있습니다2. AI의 임상적 사용을 위한 역량은 의학 분야의 다른 신기술과 대체로 유사하지만, 설명 가능성, 의료 형평성, 데이터 보안과 관련하여 매우 중요한 질적 차이가 있습니다3,4,5. 우리는 이 분야에서 리더십을 배양하기 위해 기본 커리큘럼과 과외 프로그램에 강력한 학습자 중심의 AI를 추가하는 두 가지 접근 방식을 옹호합니다.
With emerging innovations in artificial intelligence (AI) poised to substantially impact medical practice, interest in training current and future physicians about AI is growing1. Alongside this interest comes the question of what, precisely, medical students should learn2. While competencies for the clinical usage of AI are broadly similar to those for any other novel technology in medicine, there are qualitative differences of critical importance to concerns regarding explainability, health equity, and data security3,4,5. We advocate for a dual-focused approach: combining robust, learner-centered AI additions to baseline curricula and extracurricular programs to cultivate leadership in this space.
의사가 임상 맥락에서 AI에 대해 이해해야 할 사항은 무엇인가요?
What do physicians need to understand about AI in the clinical context?
가장 직접적으로, 의사는 임상 의사 결정에 영향을 미치는 모든 기술을 이해하는 것과 같은 방식으로 AI를 이해해야 합니다. 예를 들어 MRI를 사용하는 의사는 T1과 T2 가중 스캔을 구분하는 입자 스핀 물리학을 이해할 필요는 없지만, 이해는 할 수 있어야 합니다:
Most directly, physicians need to understand AI in the same way that they need to understand any technology impacting clinical decision-making. A physician utilizing MRI, for example, does not need to understand the particle spin physics differentiating T1 and T2 weighted scans, but they do need to be able to:
- (i)사용 - 해당 기술이 주어진 임상 상황에 적합한 경우와 의미 있는 결과를 얻기 위해 어떤 입력이 필요한지 식별합니다.
(i)Use it—identify when the technology is appropriate for a given clinical context, and what inputs are required to receive meaningful results. - (ii)해석하기 - 오류, 편향 또는 임상적 부적합성에 대한 인식을 포함하여 합리적인 수준의 정확도로 결과를 이해하고 해석합니다.
(ii)Interpret it—understand and interpret the results with a reasonable degree of accuracy, including awareness of sources of error, bias, or clinical inapplicability. - (iii)설명하기 - 다른 사람(예: 관련 의료 전문가 및 환자)이 이해할 수 있는 방식으로 결과와 그 근간이 되는 과정을 전달할 수 있어야 합니다.
(iii)Explain it—be able to communicate the results and the processes underlying them in a way that others (e.g. allied health professionals and patients) can understand.
이러한 기술은 AI의 맥락에서 특별한 뉘앙스를 띠게 됩니다. (i)와 (ii)의 경우, 의사는 AI의 고도로 맥락에 따른 특성과 제한된 단일 맥락에서의 성과가 항상 이전 가능하지 않을 수 있다는 사실을 이해하는 것이 중요합니다. 또한 특정 환자 그룹에 대한 알고리즘의 성능을 저하시킬 수 있는 요인을 인식하는 것도 중요합니다3.
These skills take on particular nuances in the context of AI. For (i) and (ii), it is critical for physicians to appreciate the highly context-specific nature of AI, and the fact that performance in a single restricted context may not always be transferable. It is also important to be aware of factors which may decrease the performance of algorithms for specific patient groups3.
AI는 일반적으로 '블랙박스' 효과, 즉 모델이 어떤 결정에 도달하는 메커니즘을 해독할 수 없다는 비판을 받아왔습니다1. 그러나 이러한 기술적 '설명 가능성'이 부족하다고 해서 (iii)의 의무가 면제되는 것은 아닙니다. 정보에 입각한 동의 및 임상 협력 요건을 충족하기 위해 의사는 알고리즘 결과의 출처, 특성 및 정당성에 대한 이해를 환자, 가족 및 동료에게 전달해야 할 수 있습니다.
AI has been commonly criticized for the “black box” effect—that is, the mechanism by which a model arrives at a decision may be indecipherable1. This lack of technical “explainability”, however, does not discharge the obligations of (iii). To satisfy requirements of informed consent and clinical collaboration, a physician may be called upon to communicate their understanding of the origin, nature, and justification of an algorithm’s results to patients, families, and colleagues.
의사는 보다 광범위한 전문적 맥락에서 AI에 대해 무엇을 이해해야 할까요?
What do physicians need to understand about AI in the broader professional context?
의사의 전문적 의무는 임상적 역할을 넘어 리더십과 건강 옹호까지 확장됩니다. 의료 분야에서 AI의 파괴적인 전망은 의사들이 환자 복지를 위해 공동으로 참여할 준비가 되어 있어야 하는 중대한 윤리적, 운영적 과제를 제기합니다.
The professional obligations of physicians extend beyond the clinical role into leadership and health advocacy. The disruptive prospects of AI in healthcare raise significant ethical and operational challenges which physicians must collectively be prepared to engage with for the sake of ensuring patient welfare.
소수 집단을 대표하지 않는 데이터 세트 사용3, 알고리즘이 기존의 편견을 학습하고 영속화할 가능성4 등의 요인으로 인해 알고리즘적 임상 의사 결정 지원이 건강 형평성에 미치는 영향에 대해 상당한 우려가 존재합니다. 데이터 보안 및 개인정보 보호와 관련된 위험도 빠르게 드러나고 있습니다5. 그러나 AI 자체가 편견과 불공정성에 대한 의학의 기존 문제를 일부 완화할 수 있는 잠재력도 있습니다6. 의사는 두 가지 가능성을 모두 인식하고 윤리적이고 공평한 시스템의 개발과 배포를 지지할 준비가 되어 있어야 합니다. 마지막으로, 의사는 환자 데이터에 대한 책임 있는 관리자로서 의료진과 환자 간의 기본적인 신뢰가 침해되지 않도록 행동해야 합니다.
Substantial concerns exist regarding the impact of algorithmic clinical decision support on health equity, due to factors such as the use of datasets lacking representation from minority populations3, and the possibility for algorithms to learn from and perpetuate existing biases4. Risks around data security and privacy are also becoming rapidly apparent5. There is also, however, the potential for AI itself to alleviate some of medicine’s existing problems with bias and unfairness6. Physicians should be aware of both possibilities and be equipped to advocate for the development and deployment of ethical and equitable systems. Finally, physicians must act as responsible stewards for patient data to ensure that the foundational trust between provider and patient is not violated.
의대생이 배워야 할 내용을 어떻게 배울 수 있을까요?
How might medical students learn what they need to learn?
AI와 의학에 모두 능통한 의사 리더를 양성하기 위한 공동의 노력을 기울여야 합니다. 의학에서 임상적으로 관련성이 있고 계산적으로 실현 가능한 AI 대상을 선택하는 것은 간단한 일이 아니기 때문에 이러한 이중 역량이 중요합니다. 사일로화된 접근 방식은 명확한 임상 목표가 눈에 띄지 않게 되고 기술적인 '문제 해결을 위한 솔루션'의 생산이 악화될 수 있습니다7. 학습에 대한 다학제적이고 통합적인 접근 방식은 이러한 목표를 달성하는 데 도움이 될 것입니다.
Concerted efforts should be taken to cultivate physician-leaders who are fluent in both AI and medicine. Such dual competence is important, as it is no simple task to select clinically relevant and computationally feasible targets for AI in medicine. A siloed approach may lead to clear clinical targets going unnoticed and worsen the production of technical “solutions in search of problems”7. A multidisciplinary, integrated approach to learning will serve to facilitate this goal.
이러한 복잡한 주제에 접근할 때는 모든 의사가 일상적인 진료를 위해 반드시 알아야 하는 것과 일부 의사가 혁신을 추진하기 위해 알아야 하는 것을 구분하는 것이 중요합니다. 커리큘럼 구성 요소는 전자를 다루기 위한 것이어야 하며, 강력한 과외 프로그램은 후자를 목표로 삼을 수 있습니다. 두 가지 요소 모두 AI와 의학의 융합이 현재 의사의 정체성에 어떤 영향을 미치고 있고 앞으로도 계속 영향을 미칠지에 대한 논의를 촉진하는 역할을 합니다. 이는 핵심 지식의 틀을 확립하는 동시에 특정 주제 영역에 대해 심층적으로 탐구하고자 하는 학생들을 지원하는 '재구상된 의과대학'의 개념과도 일치합니다8.
When approaching such a complex topic, it is critical to distinguish between that which all physicians must know for everyday practice, and that which some physicians should know to drive innovation. Curricular components should be targeted to address the former, while robust extracurricular programs can be targeted toward the latter. Both components serve to promote discussions on how the convergence between AI and medicine is currently impacting and will continue to impact the physician’s identity. This aligns with the concept of the “reimagined medical school”, which establishes a framework of core knowledge while supporting students who seek deep dives into specific subject areas8.
이 접근 방식은 토론토 대학교(UofT) 의과대학에서 시범 운영되었으며, 학부의 전략 계획의 중요한 부분으로 행정부에서 채택했습니다8.
- 전임상 커리큘럼의 강의는 모든 학생에게 이러한 개념을 소개하며,
- 2년 과정의 '의학용 컴퓨팅' 자격증 프로그램은 특히 관심 있는 학생들에게 실용적인 프로그래밍 기술과 임상 데이터 과학 프로젝트에 대한 몰입을 제공합니다9.
- 또한, "의학 분야의 AI" 학생 관심 그룹은 이 주제에 대한 과외 세미나를 주최하고 의대생과 도시 내 광범위한 AI 생태계(학계 및 산업계) 간의 연결을 촉진하는 데 도움을 줍니다(지난 2년간 제공된 의학 분야의 AI 목록은 부록 표 1 참조).
This approach has been piloted at the University of Toronto (UofT) Faculty of Medicine and has been embraced by administration as an important part of the Faculty’s strategic plan8.
- Lectures in the preclinical curriculum introduce all students to these concepts, and
- the 2-year-long “Computing for Medicine” certificate program provides particularly interested students with practical programming skills and immersion into clinical data science projects9.
- Additionally, an “AI in Medicine” student interest group hosts extracurricular seminars on the subject and helps to facilitate connections between medical students and a city’s broader AI ecosystem (in academia and industry) (see Supplementary Table 1 for a list of AI in Medicine offerings in the last two years).
하버드 의대도 비슷한 접근 방식을 채택하여 의대생에게 임상 정보학 교육을 선택 과목으로 제공하고 있습니다10. 이 선택 과목에서 학생들은 관심 분야의 교수진 멘토와 짝을 이루어 교훈적인 학습과 실습을 혼합하여 정보학이 의료 시스템에 어떻게 내장되는지 탐구합니다. 또한 MIT 크리티컬 데이터 그룹과 협력하여 의학 분야의 데이터 과학에 대한 프로젝트 기반 과정을 제공하고 있습니다11. 과외 활동으로 MIT 크리티컬 데이터 그룹은 '데이터톤'(컴퓨터 과학자와 임상의가 협력하여 임상 문제를 해결하기 위해 데이터를 사용하는 짧은 경연 대회)12을 통해 AI에 대한 관심을 촉진하기 위해 노력해 왔습니다. 이러한 협업은 의대생 교육을 강화하기 위해 비의대 학부와의 협업 가능성을 보여주는 상징적인 사례입니다.
Harvard Medical School has engaged in a similar approach, offering clinical informatics training as an elective for medical students10. During this elective, students are paired with faculty mentors in their area of interest and engage in a mix of didactic and hands-on learning to explore how informatics is embedded into health systems. The School has also collaborated with the MIT Critical Data group to offer a project-based course on data science in medicine11. Extracurricularly, the MIT Critical Data Group has worked to spur interest in AI through “datathons” (brief competitions wherein computer scientists and clinicians work together to use data to solve clinical problems)12. These collaborations are emblematic of the possibilities for collaboration with non-medical faculties to enrich the education of medical students.
이러한 경험에서 얻은 인사이트를 바탕으로 우리는 커리큘럼 및 과외 활동 영역에서 일련의 중요한 기회를 발견했습니다(표 1에 요약되어 있음). 학습 목표와 학습 목표 전달 간의 시너지 효과를 찾고, 수동적인 지식 전달이 아닌 학생 참여에 중점을 둔 학습자 중심의 정신을 유지하는 것이 중요하다는 점을 강조하고자 합니다. AI를 효과적으로 활용하는 데 필요한 역량은 옹호, 리더십, 커뮤니케이션 등 의사 역할의 다른 핵심 측면을 수행하는 데 필요한 역량과 겹치는 경우가 많으므로 이러한 개념은 적절한 경우 커리큘럼의 다른 측면(예: 윤리적 임상 의사 결정에 대한 워크숍에 AI 사례 연구 포함)과 통합되어야 합니다. 의과대학은 학생들의 학습을 도울 뿐만 아니라 학문적 관심을 키우고 미래 리더십의 씨앗을 뿌리는 데에도 중요한 역할을 담당하고 있습니다. 이러한 권장 사항은 각 의과대학, 파트너십 및 학생 단체의 상황과 강점에 맞게 조정될 수 있으며 조정되어야 합니다.
With insight from these experiences, we identify a series of important opportunities in both the curricular and extracurricular realms (outlined in Table 1). We wish to emphasize the importance of finding synergy between the learning objectives and their delivery, and of maintaining a learner-centered ethos with a focus upon student engagement rather than passive knowledge transfer. These concepts should be integrated with other aspects of the curriculum wherever appropriate (such as the inclusion of an AI case study in a workshop about ethical clinical decision-making), as the competencies required to effectively work with AI will often overlap with those required to fulfil other core aspects of the physician role such as advocacy, leadership, and communication. Medical schools have a critical role to play not only in helping their students learn but also in nurturing their academic interests and sowing the seeds of future leadership. These recommendations can and should be tailored to the context and strengths of each medical school, its partnerships, and its student body.
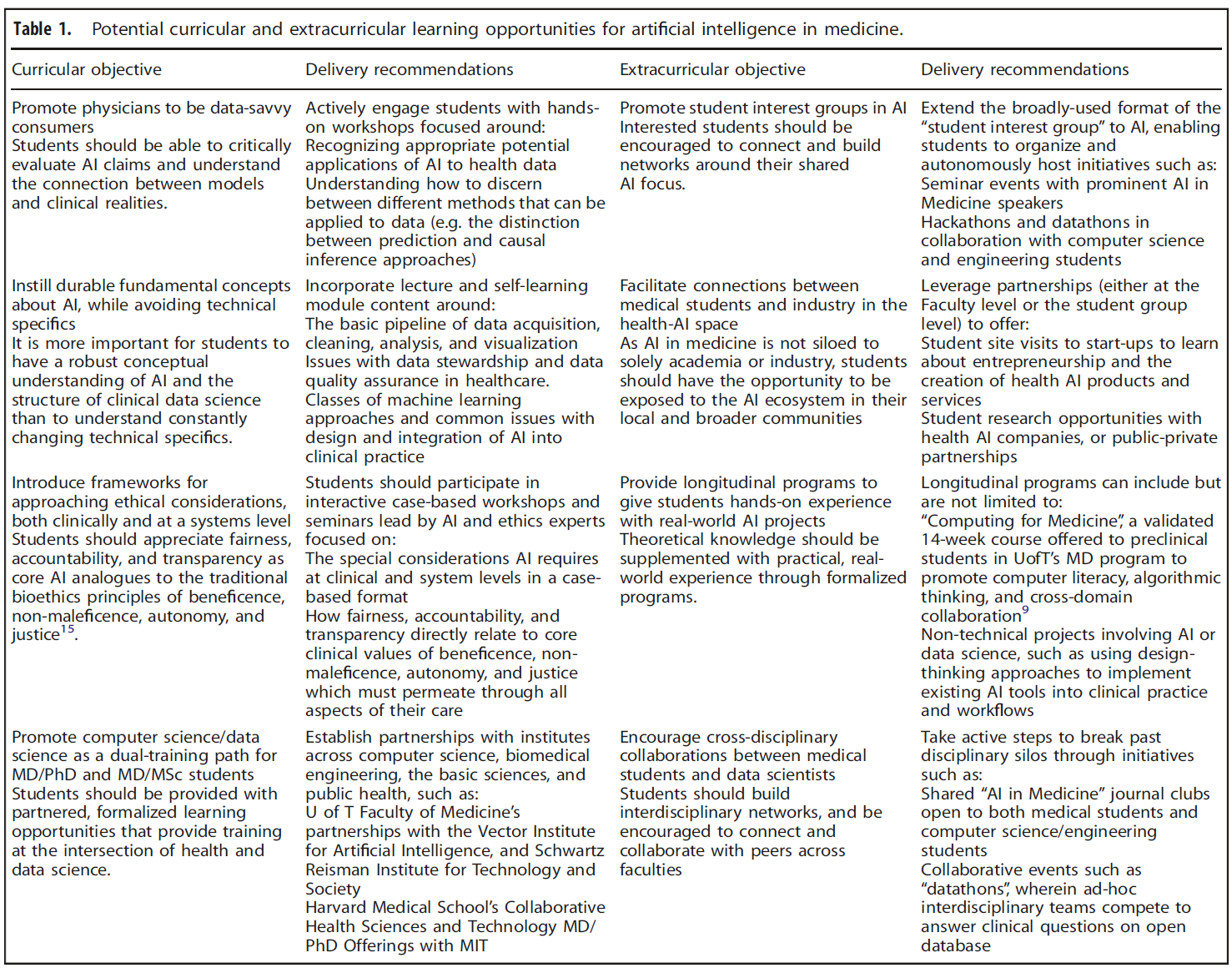
의과대학 졸업 후에는 어떻게 되나요?
What about after medical school?
의학전문대학원 교육(PGME)과 평생 의학 교육(CME)에 대한 자세한 논의는 이 연구의 범위를 벗어나지만, 의학 교육은 평생 추구해야 하는 것으로 간주되며 이후 경력 단계에 있는 학습자에게도 관심을 기울여야 한다는 점을 고려하는 것이 중요합니다13. AI 관련 역량은 기존 연구 또는 품질 개선(QI) 블록의 PGME 커리큘럼에 통합될 수 있습니다. 의학 또는 외과 수련의를 위한 연구 교육은 데이터 과학이나 생의학 공학과 같은 기술 분야뿐만 아니라 윤리, 의료 서비스 연구, 의학교육 분야에서도 이루어질 수 있습니다. QI는 검증된 혁신을 진료에 적용하고 평가하는 데 중점을 둡니다. 온라인 또는 대면 워크숍을 통한 CME 제공은 임상의가 경력을 쌓는 동안 자신의 역량을 새롭게 할 수 있을 뿐만 아니라 기존 의료진도 이 분야를 따라잡을 수 있는 기술과 지식을 갖출 수 있도록 지원합니다14. 표 1의 다양한 커리큘럼 측면은 경력 단계에 따라 학습자에게 적합하도록 수정할 수 있습니다.
While detailed discussion on postgraduate medical education (PGME) and continuing medical education (CME) is outside the scope of this work, it is important to consider that medical education is viewed as a life-long pursuit and attention needs to be provided to learners at later career stages13. Competencies around AI could be integrated in PGME curricula in existing research or Quality Improvement (QI) blocks. Research training, for medical or surgical trainees, could be in technical areas such as data science or biomedical engineering but also in ethics, health services research, and medical education. QI would focus on translating and evaluating proven innovations into care. CME offerings through online or in-person workshops can not only allow clinicians to refresh their competencies over the course of their career but also empower established practitioners with the skills and knowledge to keep up with this field14. The various curricular aspects in Table 1 can be modified to suit learners at different stages in their careers.
결론
Conclusion
궁극적으로 의과대학은 인공지능이 중요한 역할을 하게 될 미래에 대비하여 의사를 양성해야 하는 과제를 안고 있습니다. 이 과제를 성공적으로 완수하기 위해서는 학생들이 인공지능 도구의 임상적 사용, 기술적 한계, 윤리적 의미에 대해 교과과정 및 과외 학습 기회를 갖는 것이 필수적입니다. 이 기술의 중요성과 잠재적 영향을 고려할 때, 우리는 의사들 전반의 인공지능 이해력 기반을 확보하고 이 분야에서 혁신을 주도할 미래 리더들의 기술과 관심을 육성하기 위해 행동해야 합니다.
Ultimately, medical schools are tasked with training physicians for a future in which artificial intelligence is poised to play a significant role. In order to succeed at this task, it will be essential for students to have curricular and extracurricular learning opportunities around the clinical usage, technical limitations, and ethical implications of the tools at their disposal. Given the importance and potential impact of this technology, we must act both to ensure a base of artificial intelligence literacy among physicians at-large and to nurture the skills and interests of the future leaders who will drive innovation in this space.
What do medical students actually need to know about artificial intelligence?
PMID: 32577533
PMCID: PMC7305136
DOI: 10.1038/s41746-020-0294-7
Free PMC article
Abstract
With emerging innovations in artificial intelligence (AI) poised to substantially impact medical practice, interest in training current and future physicians about the technology is growing. Alongside comes the question of what, precisely, should medical students be taught. While competencies for the clinical usage of AI are broadly similar to those for any other novel technology, there are qualitative differences of critical importance to concerns regarding explainability, health equity, and data security. Drawing on experiences at the University of Toronto Faculty of Medicine and MIT Critical Data's "datathons", the authors advocate for a dual-focused approach: combining robust data science-focused additions to baseline health research curricula and extracurricular programs to cultivate leadership in this space.
Keywords: Health care; Medical ethics.
© The Author(s) 2020.
'Articles (Medical Education) > Miscellaneous' 카테고리의 다른 글
상호주관성* - 정신분석 패러다임의 변화 - (Psychoanalysis, 2008) (0) | 2019.01.02 |
---|---|
전문직간학습의 공통역량 정의(Med Teach, 2017) (0) | 2018.07.29 |