의학교육연구에서 인공지능의 기본: AMEE Guide No. 156 (Med Teach, 2023)
The fundamentals of Artificial Intelligence in medical education research: AMEE Guide No. 156
Martin G. Tolsgaarda,b , Martin V. Pusicc , Stefanie S. Sebok-Syerd , Brian Gine, Morten Bo Svendsena, Mark D. Syerf , Ryan Brydgesg , Monica M. Cuddyh and Christy K. Boscardini
소개 및 목표
Introduction and aim
의학교육에서 AI를 사용하는 것은 종종 높은 희망과 비현실적인 기대를 동반합니다. 지난 10년 동안 의학 분야의 AI는 임상 적용 측면에서 기하급수적으로 성장해 왔으며, 이는 최근에서야 AI를 사용한 연구가 보고되기 시작한 의학교육과는 다른 양상입니다(Tolsgaard 외. 2020).
The use of AI in medical education is often accompanied by high hopes and unrealistic expectations. Over the past decade, AI in medicine has grown exponentially in terms of its clinical applications; this differs from medical education where only recently studies started reporting using AI (Tolsgaard et al. 2020).
이 가이드에서는 AI 접근법을 사용한 의학교육 연구를 수행하고 해석할 때 고려해야 할 실질적인 사항을 설명합니다. 먼저 기본 용어를 소개하고 어떤 문제와 데이터가 다양한 AI 방법을 사용하기에 적합한지 파악하는 것으로 시작합니다. 또한 방법론적 엄격성을 평가하는 방법과 AI 중심 연구 프로젝트를 시작하는 데 필요한 전제 조건을 고려하는 방법도 다룹니다. 의학교육에서 AI 연구의 잠재력을 극대화하기 위한 모범 사례를 제공합니다. 부록 A에서는 이 가이드에서 사용된 용어에 대한 용어집을 제공합니다.
In this guide, we describe the practical considerations involved in conducting and interpreting medical education studies using AI approaches. We start by introducing basic terminology and identifying which problems and data are well-suited for the use of different AI methods. We also address how to evaluate methodological rigor and consider prerequisites needed to initiate an AI-focused research project. We offer best practices to maximize the potential of AI research in medical education. Supplementary Appendix A provides a glossary of terms used in this guide.
용어 및 기본 개념
Terminology and basic concepts
AI라는 용어는 '지능형 기계, 특히 지능형 컴퓨터 프로그램을 만드는 과학 및 공학'으로 정의한 존 매카시가 처음 사용했습니다. (McCarthy 외. 1956). 그 이후로 AI 분야는 통계적 방법과 계산 능력의 기술적 발전에 힘입어 큰 변화를 겪었으며, 대량의 데이터를 처리하여 명시적으로 프로그래밍하지 않고도 분류와 예측을 할 수 있게 되었습니다. 이 가이드에서는 AI를 보다 현대적인 정의에 초점을 맞추고 있습니다.
- '시각 인식, 음성 인식, 의사 결정 등
일반적으로 인간의 지능을 필요로 하는 작업을 수행할 수 있는
컴퓨터 시스템의 이론과 개발'(옥스퍼드 사전 2022)
The term AI was first coined by John McCarthy who defined it as ‘the science and engineering of making intelligent machines, especially intelligent computer programs.’ (McCarthy et al. 1956, https://jmc-stanford-edu.access.hanyang.ac.kr:8443/artificial-intelligence/what-is-ai/). Since then, the field of AI has gone through significant changes, largely driven by technical developments in statistical methods and computational power, enabling the processing of large amounts of data to make classifications and predictions without being explicitly programmed to do so. In this guide, we focus on a more contemporary definition of AI and view it as the
- ‘theory and development of computer systems able to perform tasks normally requiring human intelligence, such as visual perception, speech recognition, [and] decision-making…’ (Oxford Dictionary 2022).
이 정의를 의학교육 분야에 적용하여, 우리는 AI를 컴퓨터 과학, 수학/통계학 및 도메인 전문 지식의 상호 연관성으로 개념화하여 의학교육자 및 학습자에게 관련성이 있고 상당한 인적 자원이 필요한 작업을 수행할 수 있도록 합니다(그림 1).
By applying this definition to the field of medical education, we conceptualize AI as the interrelatedness of computer science, mathematics/statistics, and domain expertise that can enable the performance of tasks relevant for medical educators and learners and would otherwise require significant human resources (Figure 1).
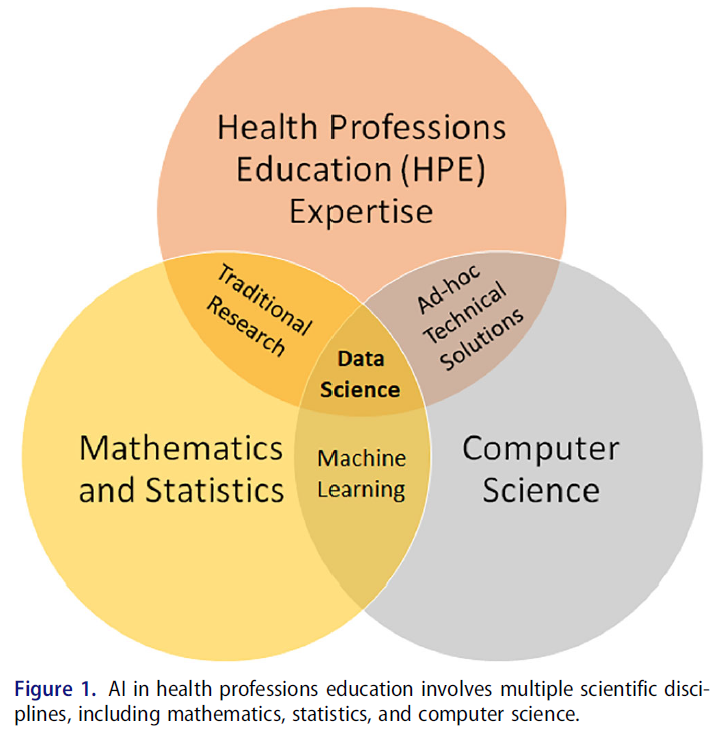
분석 도구로서의 AI
AI as an analytic tool
AI는 하나의 방법이 아니며, 데이터 마이닝(데이터에서 패턴을 추출하고 발견하는 것) 및 분석에 대한 접근 방식으로서 대규모의 복잡한 데이터 세트에 대해 다양한 통계 기법을 사용합니다. 표 1에서 볼 수 있듯이, 주성분 분석(PCA) 및 다중 비교에 불이익을 주는 고급 방법을 사용하는 회귀 모델과 같이 AI와 관련된 일부 통계적 방법은 수십 년 동안 의학 교육에서 사용되어 왔습니다(Parsell and Bligh 1999; Reed et al. 2007). 데이터 마이닝이나 이미징 데이터 분석에 일반적으로 사용되는 심층 신경망(DNN)과 같이 기존 통계와 거의 유사하지 않은 다른 AI 방법도 있습니다. DNN은 컴퓨터 비전 발전의 기반이며 영상의학, 피부과, 병리학 분야에서 초인적인 진단 성능을 보여주는 놀라운 혁신의 원동력입니다(Topol 2019).
AI is not one method but rather an approach to data mining (extracting and discovering patterns in data) and analysis using a wide range of statistical techniques on large and often complex data sets. As illustrated in Table 1, some statistical methods associated with AI have been used in medical education for decades – such as Principal Component Analysis (PCA) and regression models with advanced methods for penalizing multiple comparisons (Parsell and Bligh 1999; Reed et al. 2007). Other AI methods share little resemblance with traditional statistics, for example deep neural networks (DNNs), which are commonly used for data mining or analyzing imaging data. DNNs are the basis for advancements in computer vision and are responsible for remarkable breakthroughs demonstrating super-human diagnostic performance within radiology, dermatology, and pathology (Topol 2019).
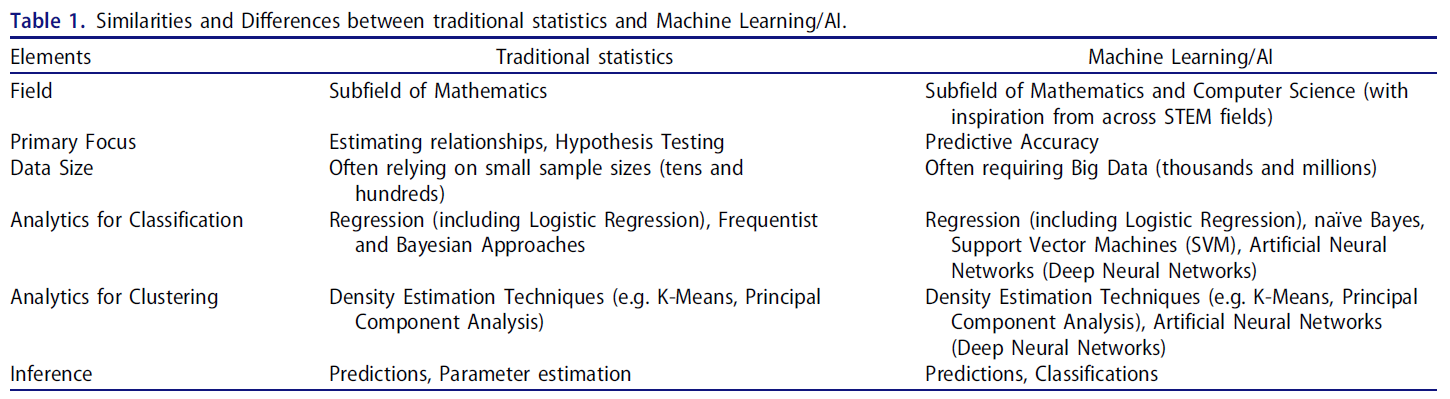
생물학적 신경망에서 영감을 얻은 DNN은 여러 노드(또는 뉴런)로 구성된 일련의 숨겨진 레이어를 통해 연결된 데이터의 입력 레이어에 의존하여 출력 레이어의 결과에 도달할 수 있도록 상호 작용합니다(그림 2).
- 연구에 따르면 피부과에 적용된 DNN은 악성 흑색종을 진단할 때 전문 피부과 의사만큼 정확했으며(Esteva 외. 2017), 병리학에 적용된 DNN은 병리 슬라이드에서 림프절의 미세 침범을 감지할 때 대부분의 병리학자보다 더 정확했습니다(Ehteshami Bejnordi 외. 2017; Golden 2017). 대장 내시경 검사 중 용종을 발견하거나 안과에서 당뇨병성 망막증을 발견할 때, 심장 영상에서 유사한 결과가 관찰되었습니다(Topol 2019).
Inspired by biological neural networks, DNNs rely on an input layer of data, connected through a series of hidden layers that consist of multiple nodes (or neurons) which interact in a way that allows them to arrive at an outcome in the output layer (Figure 2).
- Research shows that DNNs, applied in dermatology performed as well as expert dermatologists when diagnosing malignant melanoma (Esteva et al. 2017), and DNNs applied in pathology were more accurate than most pathologists in detecting microinvasion of lymph nodes in pathology slides (Ehteshami Bejnordi et al. 2017; Golden 2017). Similar results have been observed when detecting polyps during colonoscopies, when detecting diabetic retinopathy in ophthalmology, and in cardiac imaging (Topol 2019).
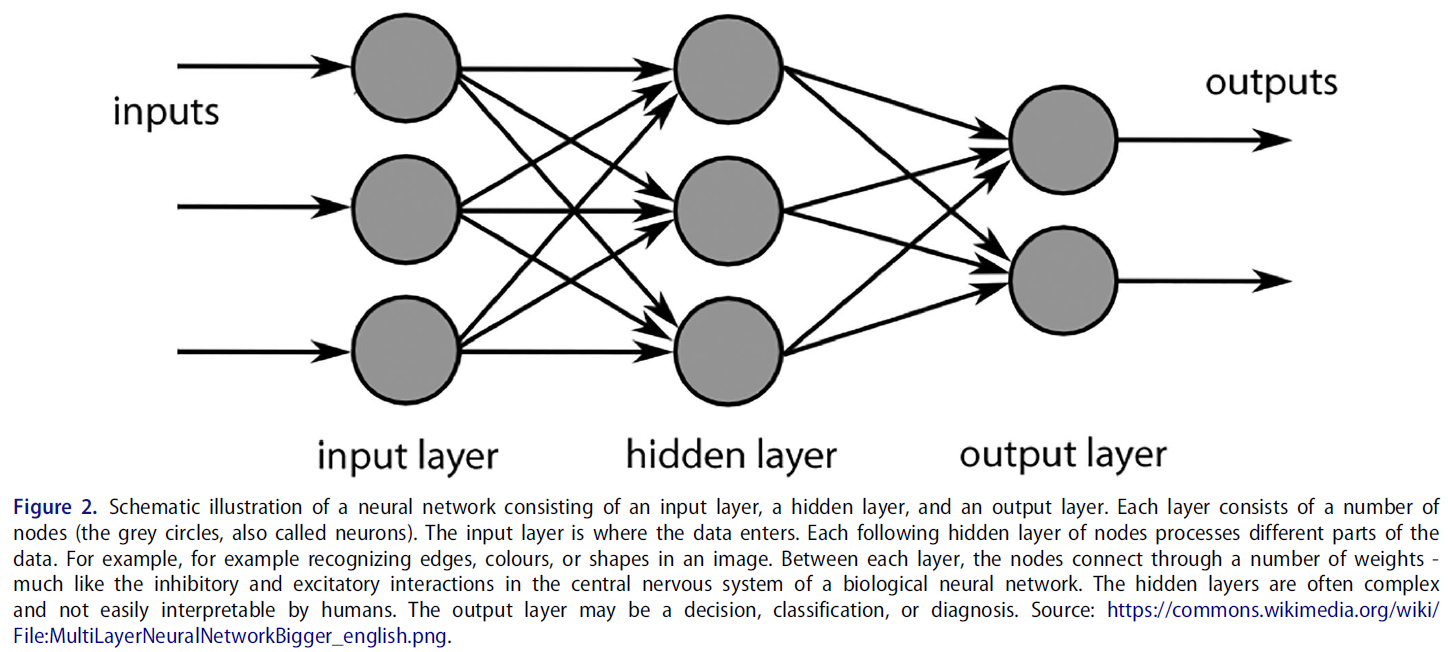
임상 연구에 적용된 이러한 AI 분석 기술은 음성, 텍스트, 이미지, 동영상 등 다양한 데이터 소스에서 일반적으로 사람이 수행하는 작업(예: 평가, 지시, 피드백, 선택)을 자동화하기 위해 의학교육 연구에도 활용될 수 있습니다.
These AI analytical techniques applied in clinical studies can also be utilized in medical education research to automate tasks usually performed by humans (e.g. assessment, instruction, feedback, selection) across a variety of data sources including voice, text, images, and videos.
의대 교육에서의 AI: 최신 연구 현황
AI in medical education: Current state-of-the-science
의학교육에서 AI를 활용한 독창적인 연구는 아직 부족하지만, AI 기술을 활용한 의학교육 연구가 증가하는 추세를 보이고 있습니다. 지금까지 많은 경험적 연구는 학습 지원을 위한 AI 사용(Chan and Zary 2019) 또는 임상 술기의 자동화된 평가에 초점을 맞추었지만, 이론이나 개념적 프레임워크의 사용은 제한적이었습니다(Tolsgaard 외. 2020). 최근 몇 년 동안 AI 입문서(Masters 2019), 인력에 미치는 영향에 대한 권장 사항(Reznick 외. 2020), 의료 커리큘럼에 AI 통합(Lee 외. 2021) 등 여러 가이드, 성명서 및 권고안이 발표되었습니다. 또한 AI의 잠재력과 가능성에 대한 논평과 사설도 다수 발표되었습니다. 자동화된 평가, 자연어 처리를 사용한 자동 채점, 의료 영상 피드백을 위한 딥 러닝의 활용에 대한 경험적 연구가 서서히 나타나고 있습니다(아래 예시 참조). 이는 AI의 기술적으로 복잡한 특성과 AI 연구에 대규모 팀, 대규모 자금, 데이터 및 시간이 필요한 경우가 많다는 사실을 반영하는 것일 수 있습니다. 이 가이드는 잠재적인 장벽을 완화하고 학자들이 의학교육에서 AI 연구에 참여할 수 있도록 돕기 위해 고안되었습니다.
Despite the scarcity of original research using AI in medical education, we are starting to see an upward trend of studies in Medical Education using AI techniques. Until now many empirical studies have focused on the use of AI for learning support (Chan and Zary 2019) or automated assessments of clinical skills, however often with limited use of theory or conceptual frameworks (Tolsgaard et al. 2020). Several guides, statement papers, and recommendations have been published in recent years, including primers to AI (Masters 2019), recommendations for workforce implications (Reznick et al. 2020), and integration of AI in medical curricula (Lee et al. 2021). Additionally, a large number of commentaries and editorials have been published about the potential and promise of AI. Empirical studies are slowly emerging in the use of automated assessments, automated scoring using natural language processing, and deep learning for medical imaging feedback (see examples below). This may reflect the technically complex nature of AI and the fact that research in AI often requires larger teams, scales of funding, data and time. This guide is designed to help mitigate potential barriers and facilitate scholars ability to engage in AI research in medical education.
AI 연구, 특히 의학교육에서 어떤 종류의 데이터가 필요합니까?
What kind of data are needed for AI studies, specifically in medical education?
AI 연구에 사용되는 데이터 유형은 광범위할 수 있으며, 영상 데이터(예: 방사선 데이터), 수치 학습 과정 데이터(예: 작업장 기반 평가) 또는 텍스트 데이터(예: 내러티브 피드백 코멘트)가 포함될 수 있습니다. 필요한 데이터의 유형은 개발 또는 테스트 중인 AI 시스템의 목적과 용도에 따라 달라집니다. 데이터 분석을 위해 데이터를 준비해야 하는 경우가 많습니다. 데이터 준비에는 데이터 세트 큐레이팅, 여러 데이터 소스 구성 및 통합, 데이터 주석 달기 등이 포함될 수 있습니다. 데이터 주석은 AI가 알려진 결과를 가진 일부 데이터를 학습하여 결과를 예측하도록 '학습'하는 지도 학습(예: 이미지의 다양한 객체에 주석을 달거나 문장의 특정 단어를 분류하는 것)에 필요합니다. 목적에 따라 데이터 주석 달기에 사용할 수 있는 무료 소프트웨어 프로그램이 여러 가지 있습니다(예: 행동 관찰 연구용 대화형 소프트웨어 BORIS, boris.unito.it에서 제공).
The types of data used in AI studies can be wide-ranging and may involve imaging data (e.g. radiology data), numerical learning process data (e.g. workplace-based assessments), or text data (e.g. narrative feedback comments). The type of data needed depends on the aim and purported use of the AI system that is being developed or tested. Often, data needs to be prepared for data analysis. The data preparation may involve curating data sets, organizing and integrating multiple data sources, as well as annotating data. Data annotation is needed for supervised learning, where an AI ‘learns’ to predict an outcome by training on some data with known outcomes (for example, annotating different objects in images or classifying particular words in a sentence). There are several free software programs available for data annotation depending on the purpose (for example, Behavioural Observation Research Interactive Software BORIS, available from boris.unito.it among other sources).
학습 분석에 다양한 데이터 소스와 형식을 통합하는 것은 의학교육에서 AI와 점점 더 관련성이 높아지는 사례가 되고 있습니다. '학업 진도를 평가하고, 미래의 성과를 예측하고, 잠재적인 문제를 발견하기 위해 학생이 생성하고 학생을 대신하여 수집한 광범위한 데이터의 해석'으로 정의되는 학습 분석은 학습자가 시간이 지남에 따라 어떻게 발전하는지 파악하는 데 점점 더 많이 사용되고 있으며, 교육 중 특정 이정표에 언제 얼마나 잘 도달하는지 모니터링하는 데도 사용될 수 있습니다(Johnson 외. 2011). 이러한 분석에는 종단 평가, 사무원 평가 점수, 시뮬레이터 점수, 필기 시험 점수 등 디지털화할 수 있는 모든 학습 과정 데이터가 포함될 수 있습니다(Holmboe 외. 2020). AI는 학습자의 기술 능력에 대한 AI 기반 자동 채점(Hung 외. 2018) 또는 크고 복잡한 데이터 세트를 사용한 팀 협업(Andersen 외. 2020) 등 학습 분석과 관련된 다양한 데이터 형식을 효율적으로 관리할 수 있는 접근 방식을 제공합니다.
The integration of a variety of data sources and formats in learning analytics is becoming an increasingly relevant case for AI in medical education. Learning analytics - defined as ‘the interpretation of a wide range of data produced by and gathered on behalf of students to assess academic progress, predict future performance, and spot potential issues’ - are increasingly used to determine how learners develop over time and can also be used to monitor when and how well they reach certain milestones during their training (Johnson et al. 2011). These analytics may include longitudinal assessments, clerkship assessment scores, simulator scores, and written exam scores – any learning process data that can be digitized (Holmboe et al. 2020). AI affords an efficient approach to managing a variety of data formats that are often associated with learning analytics, including AI-based automated scoring of learners’ technical skills (Hung et al. 2018) or team collaboration (Andersen et al. 2020) using large and complex data sets.
AI로 어떤 종류의 작업을 해결할 수 있나요?
What kind of tasks can be solved using AI?
AI는 분석 도구와 접근 방식을 확장하는 데 도움을 주는 등 현재 연구 및 교육 팀에서 어려움을 겪고 있는 작업의 효율성을 높이는 데 도움이 될 수 있습니다.
- 연구 설계 단계에서는 AI가 제공하는 데이터 소스의 유연성과 데이터 추출의 자동화를 통해 다른 질문, 방법, 접근 방식에 대한 가능성을 열어줄 수 있습니다.
- 데이터 준비 단계에서 AI는 여러 소스에 걸친 데이터 집계, 빅 데이터의 저장 및 검색을 통해 효율성을 높이고 데이터 탐색을 지원할 수 있습니다.
- 분석 단계에서 신경망은 일반적으로 많은 인력과 시간이 필요한 분석을 효율성과 정확성을 높여 신속하게 제공할 수 있습니다. 또한, 신경망은 기존 분석으로는 쉽게 확인할 수 없는 데이터 내부 및 데이터 전반의 복잡한 연관성을 포착하고 강조 표시할 수 있습니다.
AI can help increase efficiency around tasks that are currently challenging for research and education teams, including helping to expand analytical tools and approaches.
- During the research design phase, flexibility of data sources and automaticity of data extraction afforded by AI may open-up possibilities for alternative questions, methods and approaches.
- In the data preparation phase, AI can increase efficiency with data aggregation across multiple sources, storage and retrieval of big data, and assist in data exploration.
- During the analysis phase, neural networks can provide expedited analytics that would typically require many hours of human resources and time with increased efficiency and accuracy. Additionally, neural networks can capture and highlight complex associations within and across data that may not be easily ascertained by traditional analysis.
아래에서는 의학교육과 관련된 두 가지 데이터 유형에 사용되는 데이터 분석 유형을 설명하기 위해 두 가지 예를 제공합니다.
Below, we provide two examples to illustrate the types of data analysis used with two different data types relevant to medical education.
예제
Examples
예시 1. 의학 교육에서 텍스트 분석을 위한 자연어 처리 및 심층 신경망 사용
Example 1. Using Natural language processing and deep neural Networks for text analysis in medical education
AI에 관한 한, 텍스트 분석과 텍스트 마이닝은 모두 대규모 텍스트 데이터 세트(예: 인터뷰 필사본)에서 패턴을 찾아 의미를 추출하기 때문에 동의어라고 할 수 있습니다. 텍스트 분석 접근 방식인 자연어 처리(NLP)는 인간의 언어 처리를 시뮬레이션하여 기계가 텍스트 데이터를 이해하고 분석하는 데 도움을 줍니다. 하지만 인간과 달리 NLP는 체계적이고 매우 효율적인 방식으로 무제한의 데이터를 분석할 수 있습니다. 의학 교육에서의 NLP 연구의 예로는
- 필기 시험(예: 에세이) 채점,
- 서술형 피드백에서 위탁 평가와 관련된 요인 파악(Stahl 외. 2021; Solano 외. 2021),
- 서술형 피드백 품질 평가(Gin 외. 2021; Neves 외. 2021),
- 교정이 필요한 학습자의 조기 식별(Tremblay 외. 2019),
- 시험 항목의 자동화된 생성(Chary 외. 2019) 등을 들 수 있습니다.
As far as AI is concerned, text analysis and text mining are synonyms since they both look for patterns in large sets of text data (e.g. transcriptions of interviews) to extract meaning. Natural language processing (NLP), a text analysis approach, helps the machines understand and analyse textual data by simulating human language processing. However, unlike humans, NLP can analyse unlimited amounts of data in a systematic, highly efficient way. Examples of NLP studies in medical education include
- scoring of written exams (e.g. essays; Zhang et al. 2012),
- identification of factors tied to entrustment ratings in narrative feedback (Stahl et al. 2021; Solano et al. 2021),
- assessment of narrative feedback quality (Gin et al. 2021; Neves et al. 2021),
- early identification of learners in need of remediation (Tremblay et al. 2019), and
- the automated generation of test item distractors (Chary et al. 2019).
방법
Methods
NLP에서 분류기는 텍스트의 의미적 의미를 인식하고 레이블을 지정할 수 있는 알고리즘입니다. NLP 분류기는 사람이 놓치기 쉬운 기능어(예: 그녀, 또는, of)는 물론 내용어(예: 환자, 가슴, 심장)와 구문(n-그램)을 빠르게 식별하여 보다 체계적으로 분류함으로써 인간 코더에 의한 기존 텍스트 분류보다 효율성을 제공합니다. 자동화된 에세이 채점이나 특정 루브릭 또는 등급과 관련된 서술형 의견의 검토에는 텍스트 분류 접근 방식을 사용하는 경우가 많습니다.
In NLP, a classifier is an algorithm that is capable of recognizing and labelling semantic meaning of text. NLP classifiers provide efficiency over traditional text classification by human coders by quickly identifying both function words (e.g. she, or, of), which we humans often miss, as well as content words (e.g. patient, chest, or heart) and phrases (n-grams) for classification more systematically. Automated essay scoring or examination of narrative comments associated with specific rubrics or ratings often use text classification approaches.
작업 예
Worked example
의학교육에서 NLP를 활용하는 가상의 예로, 수련의의 현재 병력(HPI)의 질에 대한 자동 채점을 생각해 보겠습니다. 임상의 교육자가 수작업으로 교육생의 HPI를 채점할 수도 있지만, 이 프로세스를 자동화하면 교육생이 노트를 작성할 때 실시간으로 형성적인 피드백을 제공하고, 정교화가 필요할 수 있는 부분(예: 환자 불만 사항, 타임라인 등)을 강조하는 데 도움이 될 수 있습니다. 전문가 평가자를 위한 채점 가이드가 개발되었는데, 이 예에서 예시적으로 사용한 것은 Lewin 등의 환자 프레젠테이션 평가(PPR, Lewin 등, 2013)입니다. 이 도구는 DNN을 사용하여 NLP 분류기로 자동화할 수 있습니다. 여기에서는 DNN을 사용하여 NLP 분류기를 구현하여 HPI를 채점하는 방법을 간략하게 설명합니다:
As a hypothetical example of NLP in medical education, consider the automated scoring of the quality of a trainee’s history of present illness (HPI). While a trainee’s HPI could be scored manually by clinician educators, automating the process could be helpful for giving real-time, formative feedback to trainees when writing notes, highlighting where elaboration (e.g. on patient complaints, timelines, etc.) may be necessary. Scoring guides for expert raters have been developed such as Lewin et al.’s Patient Presentation Rating (PPR, Lewin et al. 2013), which we use illustratively in this example. This tool could be automated as an NLP classifier using DNNs. Here, we outline how to implement an NLP classifier using a DNN to score HPIs:
- 선택한 평가 도구(이 경우 PPR)에서 평가한 다양한 품질을 나타내는 서술 형식(즉, 서면 또는 필사본)의 교육생 HPI 데이터베이스를 수집합니다.
Collect a database of trainee HPIs in narrative form (i.e. written or transcribed) that represent a range of quality as assessed by the rating instrument of choice (in this case the PPR). - 인간 평가자가 PPR을 사용하여 HPI 서술의 하위 집합에 점수를 매깁니다(예: PPR의 두 번째 항목인 '입원 사유가 포함된 명확한 환자 소개'를 사용하여 1~5점 척도를 사용합니다). 이렇게 수동으로 분류(라벨링)된 하위 집합은 DNN을 훈련하고 검증하는 데 사용됩니다.
Score a subset of HPI narratives by human raters using the PPR – for example, using the second item of the PPR: ‘a clear patient introduction with reason for admission’ – using a scale of 1-5. This manually classified (labelled) subset will be used to train and validate the DNN. - DNN을 설계합니다(그림 2 참조). NLP 분류의 경우, DNN의 입력 레이어는 일반적으로 텍스트를 숫자 표현으로 변환합니다. 중간 계층에는 특정 단어, 단어 패턴, 내러티브 테마 등 텍스트의 더 복잡한 '특징'을 점진적으로 인식하는(훈련을 통해) 스택형 인공 뉴런이 포함될 수 있습니다. 그런 다음 출력 레이어는 이러한 특징을 사용하여 원본 내러티브가 어느 범주에 속하는지(이 예에서는 1~5점 사이의 점수)를 예측합니다.
Design the DNN (see Figure 2). For NLP classification, a DNN’s input layer typically converts text into numerical representations. The intermediate layers may involve stacked artificial neurons that progressively recognize (through training) more complicated ‘features’ of the text – specific words, patterns of words, and narrative themes. The output layer then uses these features to predict which category the original narrative belongs to – in this example a score between 1-5. - 2단계에서 수동으로 레이블이 지정된 HPI 내러티브 하위 집합의 일부(즉, '훈련 세트')를 사용하여 DNN을 학습시킨 다음, 학습된 DNN을 사용하여 수동으로 레이블이 지정된 나머지 HPI 내러티브 하위 집합(즉, '검증 세트')의 점수를 예측합니다. 타당성 검사 세트만 사용하여 예측된 점수를 수동으로 라벨링된 점수와 비교하여 분류기의 성능을 결정합니다(아래 평가 기준 참조). 앞서 설명한 DNN을 구현하고 훈련할 수 있는 몇 가지 패키지가 있으며, 가장 일반적으로 사용되는 패키지는 오픈 소스 TensorFlow 플랫폼입니다.
Train the DNN using a portion of the manually labelled subset of HPI narratives from Step 2 (i.e. the ‘training set’), then use the trained DNN to predict the scores of the remaining manually labelled subset of HPI narratives (i.e. the ‘validation set’). Using the validation set only, compare the predicted scores to the manually labelled scores to determine the performance of the classifier (see Evaluation Criteria below). There are several packages that could implement and train the DNN outlined; the most commonly used package is the open-source TensorFlow platform. - 이제 NLP 분류기를 사용하여 수동으로 레이블이 지정되지 않은 내러티브의 점수를 예측할 수 있지만, 설명 가능한 AI를 사용하여 예측 점수의 기반이 되는 잠재적 구조/요소를 탐색하는 것이 통찰력이 될 수 있습니다. 이러한 방법은 현재 분류 범주와 관련된 핵심 단어와 구문을 강조하는 '히트맵' 접근법을 개발하고(Stahl 외. 2021; Pryzant 외. 2018, 2020; Danilevsky 외. 2020), NLP 분류기에 의해 식별된 잠재적 특징(즉, 분류의 기반이 되는 내러티브 주제)을 추론하기 위해 모색되고 있습니다. 최근 연구에서는 DNN 기반 NLP 분류기가 사전 분류된 피드백 내러티브에서 위탁 수준을 예측하기 위해 훈련하는 동안 '학습'한 잠재적 피드백 특성(절차적 대 인지적 기술, 건설적 대 강화적 등)을 확인했습니다(그림 3, Gin 외. 2021).
While the NLP classifier may now be used to predict the scores of narratives that have not been manually labelled, it may be insightful to explore the latent constructs/factors that underlie the predicted scores using explainable AI. Such methods are currently being explored to develop a ‘heatmap’ approach highlighting key words and phrases pertaining to the classification categories (Stahl et al. 2021; Pryzant et al. 2018, 2020; Danilevsky et al. 2020), and also to infer latent features (i.e. narrative themes underlying classification) identified by an NLP classifier. Recent work identified latent feedback characteristics (procedural vs cognitive skills, constructive vs reinforcing, etc) that a DNN-based NLP classifier ‘learned’ during training to predict entrustment levels from pre-classified feedback narratives (Figure 3, Gin et al. 2021).
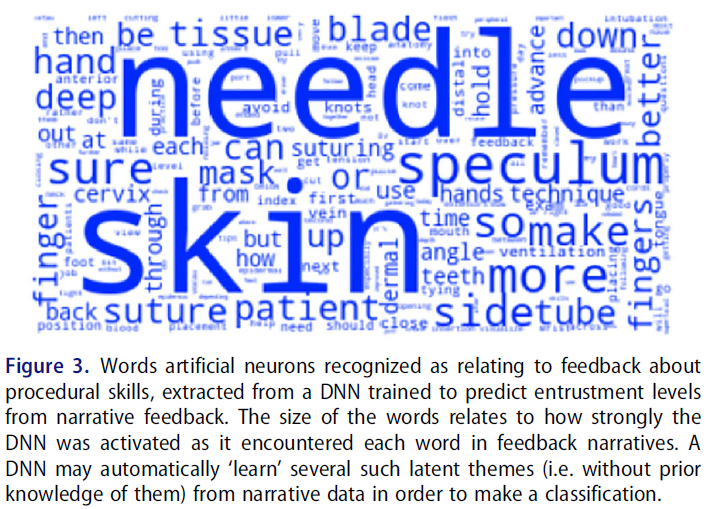
요약하자면, 텍스트 분석은 DNN의 훌륭한 응용 분야로, 대규모 텍스트 코퍼스에서 주제를 효율적으로 드러낼 수 있는 방법을 보여줍니다. 교육생이 생성하거나 교육생을 대신하여 생성하는 방대한 양의 텍스트가 감독자의 검토 능력을 압도할 수 있다는 점을 고려할 때, 이 애플리케이션은 특히 HPE에서 장점이 있습니다.
In summary, text analysis is an excellent application of DNNs, showing how they can efficiently surface themes from a large corpus of text. Given that the voluminous texts generated by and on behalf of trainees can overwhelm the capacity of their supervisors to review them, this application has particular merit in HPE.
다음 예에서는 텍스트 분석에서 이미지 및 비디오 분석으로 넘어갑니다.
In the next example, we move from text analysis to analysing images and videos.
예제 2. 영상 피드백에 컨볼루션 신경망 사용
Example 2. Using convolutional neural Networks for imaging feedback
많은 전문 분야에서는 환자 진단 및 관리를 위해 의료 이미지에 의존합니다. 방사선과, 병리과, 피부과와 같은 전통적인 영상 전문 분야 외에도 여러 임상 전문 분야에서 의료 이미지가 현장 진료 검사의 일부로 점점 더 많이 사용되고 있습니다(Topol 2019). 예를 들어 산부인과 의사는 초음파로 태아를 스캔하여 태아의 건강 상태를 확인하거나 안과 의사는 안과 검사의 일상적인 부분으로 망막 초음파에 의존할 수 있습니다. 문제는 의료 영상에서 시각적 진단을 위한 학습 곡선이 길고 임상의가 필요한 빈도로 특정 진단을 접하지 못하기 때문에 전문가 수준의 성능에 도달하지 못할 수 있다는 것입니다(Tolsgaard 외. 2019). 의료 영상 분야에서 AI를 사용하면 임상 교육 및 평가에 잠재적으로 큰 영향을 미칠 수 있는 시각적 단서뿐만 아니라 진단 제안을 포함하여 의료 전문가에게 피드백 및 의사 결정 지원을 제공할 수 있습니다(Tschandl et al. 2020).
Many specialties rely on medical images for patient diagnosis and management. Besides the traditional imaging specialties such as radiology, pathology, and dermatology, medical images are now increasingly used as part of point-of-care examinations in multiple clinical specialties (Topol 2019). For example, an obstetrician may scan the fetus using ultrasound to determine fetal well-being or an ophthalmologist may rely on retinal ultrasound as a routine part of an eye examination. The problem is that the learning curves for visual diagnosis in medical imaging are often long, and clinicians may never attain expert-level performance because they do not encounter specific diagnoses with the frequency needed (Tolsgaard et al. 2019). The use of AI in the field of medical images enables feedback and decision-support to the health care professional including diagnostic suggestions as well as visual cues which can potentially have significant impact on clinical instruction and assessment (Tschandl et al. 2020).
방법
Methods
의료 이미지 분석에 가장 자주 사용되는 AI 기술은 신경망(특히 합성곱 신경망)으로, 현재 컴퓨터 비전의 선도적인 기술입니다(Krohn 2019). 신경망 사용의 기본 아키텍처와 방법론은 위 섹션에서 설명했습니다. 의료 이미지의 분류 또는 분할을 위해 새로운 AI 모델을 훈련할 때 사전 훈련된 네트워크가 시작점으로 사용되는 경우가 많으며, 이는 공개적으로 사용 가능한 대규모 이미지 데이터베이스(예: Image-Net.org)에서 사전 훈련된 일반 모델 또는 관심 있는 이미징 절차에 대한 이전 출판물에서 제공된 특정 모델을 기반으로 할 수 있습니다. 아래의 작업 예는 산부인과 초음파 검사를 수행할 때 수련의를 지원하기 위한 AI 모델 개발을 목표로 하는 실제 연구 프로젝트에 대해 설명합니다.
The AI technique most often used in the analysis of medical images is neural networks (specifically convolutional neural networks), which are now the leading technology in computer vision (Krohn 2019). The basic architecture and methodology behind the use of neural networks have been described in the sections above. Often, a pre-trained network is used as a starting ground when training a new AI model for classification or segmentation of medical images - this may be based on pretrained generic models from large publicly available image databases (e.g. Image-Net.org) or specific models that have been made available from previous publications on the imaging procedure of interest. The worked example below describes an actual research project that aims to develop AI models for supporting trainees when performing obstetric ultrasound examinations.
작업 예시
Worked example
임상의, 데이터 과학자 및 의학교육 과학자로 구성된 그룹(Tolsgaard 외. 2021)은 산부인과 초음파 검사 중 성능에 대한 자동화된 피드백을 제공하기 위해 이미지 품질에 대한 AI 기반 채점 시스템 개발에 착수했습니다. 이 초음파는 태아의 생체 인식 및 태아 기형의 존재 여부와 같은 결과를 보고합니다. 처음부터 끝까지 프로세스는 다음과 같습니다:
To provide automated feedback on performance during screening obstetric ultrasound examinations, a group consisting of clinicians, data scientists and medical education scientists (Tolsgaard et al. 2021) set out to develop an AI-based scoring system for image quality. These ultrasounds report findings such as fetal biometries and the presence of any fetal malformations. The process from start to finish is described below:
- 먼저, 관련 국가 및 지역 보건 당국에 데이터 액세스와 관련된 규제 및 법적 권한을 확인했습니다.
First, regulatory and legal permissions regarding data access were verified with relevant national and regional health authorities. - 임상 전문가가 일상적인 초음파 검사 데이터에 수동으로 주석을 달았습니다(이미지에 다양한 해부학적 구조를 나타내는 라벨을 붙임). 이를 통해 어떤 이미지의 품질이 높은지 낮은지를 판단했습니다. 이러한 작업에는 다양한 오픈 소스 주석 소프트웨어 솔루션을 사용할 수 있습니다. 평가자 간 신뢰도를 측정한 결과, 한 명의 평가자가 각 이미지에 계속 주석을 달 수 있을 만큼 충분히 높은 것으로 판정되었습니다.
Data from routine ultrasound examinations were manually annotated (labels provided onto images denoting different anatomical structures) by clinical experts to determine which images were of high versus low quality. A variety of open-source annotation software solutions are available for these tasks (https://github.com/taivop/awesome-data-annotation). Inter-rater reliability was determined and was deemed high enough for a single assessor to continue to annotate each image. - 2,000개 이상의 이미지에 주석을 단 후, 신경망의 첫 번째 반복은 다른 장비로 다른 모집단으로 개발된 이전에 발표된 네트워크를 기반으로 훈련되었습니다. 그 결과 기존 연구와 비슷한 성능이 나타났습니다. 사전 훈련된 다양한 신경망을 자유롭게 사용할 수 있습니다.
After having annotated more than 2000 images, the first iteration of the neural network was trained based on a previously published network that was developed in a different population with different equipment. Similar performance was found as in the original study. A variety of pre-trained neural networks are freely available. - 임상의에게 신경망이 특정 분류에 어떻게 도달했는지에 대한 시각적 피드백을 제공하기 위해 히트맵을 사용했습니다. 히트맵은 이미지 분류에서 AI가 특히 중요하게 생각한 픽셀을 강조 표시합니다(예는 그림 4 참조).
To provide visual feedback to the clinicians on how the neural network arrived at a certain classification, heatmaps were used. The heatmap highlights pixels that were particularly important for the AI in the classification of an image, see Figure 4 for an example. - 이 신경망을 시뮬레이션 학습 환경에서 평가 목적으로 사용할 수 있는지 알아보기 위해 컴퓨터 애니메이션 그래픽이 포함된 가상현실 시뮬레이터에서 연습하는 대규모 훈련생 그룹의 퍼포먼스에 신경망을 적용했습니다. 안타깝게도 신경망은 이러한 성능을 완전히 분류할 수 없었고 시뮬레이션 환경의 데이터를 기반으로 완전히 새로운 모델을 학습시켜야 했습니다(모델 성능을 평가하는 방법의 예는 그림 5 참조). 신경망은 훈련 데이터와 유사한 데이터를 사용하여 좁은 문제를 해결하는 데 가장 효과적이며, 훈련과 전달 문제 간에 큰 차이가 있을 때 스킬을 평가하는 것과 같이 보다 광범위한 문제에는 적합하지 않습니다.
To explore whether this neural network could be used for assessment purposes in a simulation learning environment, the neural network was applied to performances from a large group of trainees practicing on virtual reality simulators with computer-animated graphics. Unfortunately, the neural network was completely unable to classify these performances and an entirely new model had to be trained based on data from the simulated setting (see Figure 5 for example of how to evaluate model performance). This is a frequent finding: neural networks work best to solve narrow problems using data that resembles the training data and are ill-equipped for more broad problems, such as evaluating a skill when there are large differences between training and transfer problem.
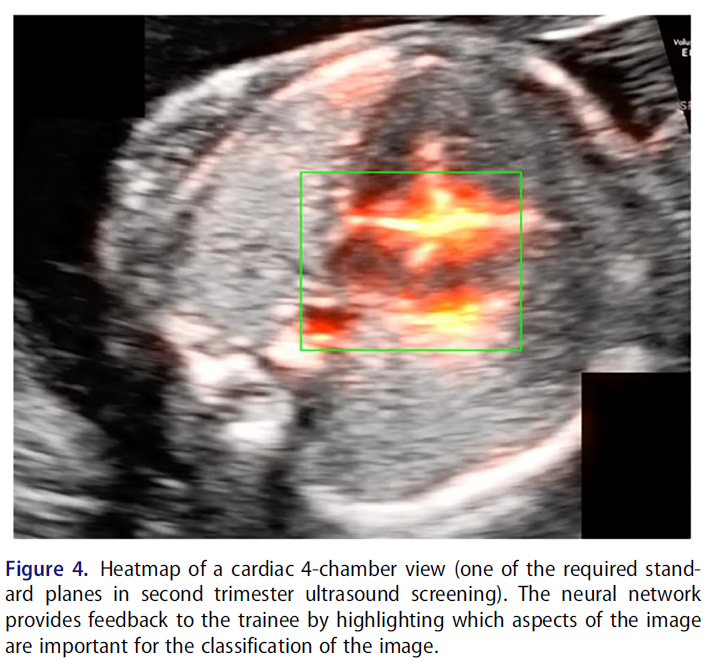
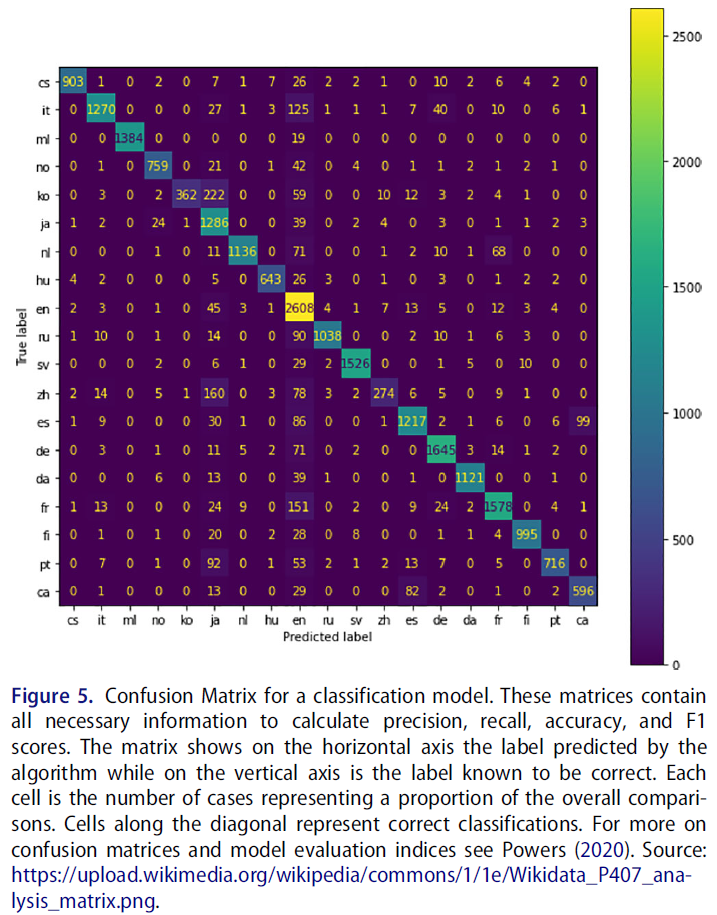
AI 결과물 이해 - 과제와 앞으로의 방법
Understanding AI outputs - challenges and ways forward
신경망에 의존하는 많은 AI 시스템에 내재된 한 가지 문제는 그 결정을 설명하기 어렵다는 것입니다. 신경망의 여러 계층에 있는 뉴런 간의 수많은 상호 작용을 데이터 과학자를 포함한 사람이 쉽게 해독할 수 없기 때문입니다. 기술적 관점에서 설명 가능성은 항상 쉬운 것은 아니지만, 여러 가지 유망한 접근 방식이 등장하기 시작했습니다(Linardatos 외. 2021). 예를 들어, 설명 가능한 AI(XAI)라는 개념은 지난 몇 년 동안 AI 커뮤니티 내에서 성장해 왔습니다.
One problem inherent in many AI systems that rely on neural networks is that their decisions are difficult to explain. This is because the many interactions between neurons in the multiple layers of a neural network are not easily deciphered by humans (including data scientists). Explainability is not always easy from a technical point of view but different promising approaches are beginning to emerge (Linardatos et al. 2021). For instance, the concept of explainable AI (XAI) has grown within the AI community over the past few years.
설명 가능성에 대한 또 다른 접근 방식은, 새로운 AI 시스템을 학습하는 동안 특정 개념을 사용하여 교육적 관점에서 관련성이 있을 수 있는 선택된 기능 클래스를 기반으로 피드백을 제공하는 시각화를 사용하여 AI가 개념적 결과물을 제공하도록 강제하는 것입니다(Koh et al. 2020). 선택한 기술적 접근 방식에 관계없이, 설명 가능성은 기술적 복잡성이 추가됨에도 불구하고 의학교육 관점에서 AI 시스템의 유용성을 평가할 때 종종 바람직합니다.
Another approach to explainability is forcing the AI to provide conceptual outputs by using visualizations, where certain concepts are used during the training of new AI systems to enable feedback based on selected classes of features that may be relevant from an educational perspective (Koh et al. 2020). Regardless of the technical approach selected, explainability is often desirable when evaluating the utility of AI systems from a medical education perspective despite its added technical complexity.
마지막으로, 그림 4와 같이 AI 분류 또는 회귀에 중요한 픽셀을 시각적으로 표현하는 히트 맵을 사용하는 간단한 접근 방식이 있습니다. 그러나 이러한 접근 방식은 초보 학습자가 특정 기능이 중요한지 아닌지를 식별하는 데 도움이 되는 개념적 인사이트를 제공하지 못할 수 있습니다.
Finally, a simplistic approach is to use heat maps as shown in Figure 4, which provide visual representations of the pixels that are important for AI classification or regression. However, these approaches may still fail to provide conceptual insights that help novice learners to identify why certain features are or are not important.
AI 연구를 위한 평가 기준
Evaluation Criteria for AI studies
AI 연구에 대한 평가 기준은 일반적으로 모델 개발과 모델 검증이라는 두 가지 주요 범주로 나뉩니다. AI 연구와 가장 관련성이 높은 가이드라인은 EQUATOR(건강 연구의 품질 및 투명성 향상) 네트워크에서 제공하며, 이 네트워크는 특히 AI 연구를 위한 TRIPOD-ML(개인 예후 또는 진단을 위한 다변량 예측 모델의 투명한 보고) 표준을 개발했습니다(콜린스 외. 2015). 의학교육에서 AI 연구를 평가하는 데 사용할 수 있는 포괄적인 모델 설명에는 모델 개발 및 검증에 사용된 접근 방식에 대한 세부 정보가 포함됩니다.
Evaluation criteria for AI studies is commonly broken down into two main categories: model development and model validation. The most relevant set of guidelines for AI studies comes from the EQUATOR (Enhancing Quality and Transparency of Health Research) Network, and they have developed the TRIPOD-ML (Transparent Reporting of a Multivariable Prediction Model for Individual Prognosis or Diagnosis) standards specifically for AI studies (Collins et al. 2015): https://www.equator-network.org/reporting-guidelines/tripod-statement/. A comprehensive model description that can be used to evaluate AI studies in Medical Education will include details about the approaches used in both developing and validating the model.
예를 들어, AI 연구에 대한 일반적인 접근 방식은 하나 이상의 소스에서 데이터를 수집하고, 모델링을 위해 데이터를 준비하고, 알고리즘을 개발하고, 모델을 훈련한 다음(예: 데이터의 70%로) 나머지 데이터(이 예에서는 30%)로 모델을 테스트하는 것입니다. 대부분의 경우 첫 번째 단계는 미세 조정된 초기 모델을 개발하는 것이며, 모델 구축 절차를 설명할 때 모델 사양 및 성능과 같은 개선 사항에 대한 감사를 포함하는 것이 좋은 보고 관행입니다.
For example, a common approach for AI studies is to gather data from one or more sources, prepare the data for modelling, develop an algorithm and train a model (e.g. with 70% of the data), then test the model with the remaining data (i.e. 30% in this example). More than likely, the first step is to develop an initial model that is fine-tuned, and good reporting practice is to include an audit of the refinements made (e.g. model specification and performance) as the model building procedures are described.
모델 개발 기준
Model development Criteria
AI 모델을 개발할 때는 데이터 소스와 샘플링 방법(예: 평가 데이터, 오픈 소스 데이터)을 설명해야 합니다. 모든 사례 유형을 설명하면 데이터의 완전성과 대표성을 평가할 수 있을 뿐만 아니라 모델이 개발되고 학습된 특성과 조건을 이해할 수 있는 정보를 얻을 수 있습니다.
- 데이터 설명은 데이터가 모델 예측의 대상이 될 수 있는 더 많은 인구(예: 연령, 성별, 인종)를 대표한다는 증거를 제공해야 합니다.
- 다른 절차와 마찬가지로 샘플링은 모델 개발 및 선택에 큰 영향을 미칠 수 있으므로 데이터에 시스템적 편향이 존재하지 않도록 노력해야 합니다.
- 또한 데이터 설명에는 알고리즘 개발 시 편향성을 유발할 수 있는 누락된 데이터를 구체적으로 언급해야 합니다. 누락된 데이터를 대입하는 경우 대입imputation 방법을 설명해야 합니다.
- 또한 예측자와 수행된 '피쳐 엔지니어링'(예: 예측자의 규모 조정, 표준화 또는 분류 또는 주성분 분석과 같은 접근 방식을 사용하여 새로운 예측자 생성)을 설명하는 것도 중요합니다.
- 마지막으로, 결과 측정값을 명시하고 결과를 평가한 방법에 대한 세부 정보를 제공해야 합니다.
When developing an AI model, one must describe the data sources and how they were sampled (e.g. assessment data, open-source data). Describing all case types provides information to assess not only the completeness and representativeness of the data, but also an understanding of the characteristics and conditions under which the model was developed and trained.
- The data description should provide evidence that the data are representative of the larger population who may be subject to the model predictions (e.g. age, gender and race). Similar to other procedures, sampling can greatly impact model development and selection, requiring efforts to ensure that systemic biases do not exist in the data.
- Data descriptions should also specifically reference any missing data, which has the potential to contribute bias in the development of an algorithm. If missing data are imputed the imputation approach must be described.
- It is also crucial to describe predictors and any ‘feature engineering’' that was performed (e.g. scaling, standardizing or categorizing the predictors or creating new predictors using approaches such as principal component analysis).
- Finally, the outcome measure must be specified, with details about how the outcome was assessed.
모델 개발에 대해 보고할 때는 모델이 어떻게 개발되었는지, 어떤 예측 변수를 제외할지 또는 다른 예측 변수에 더 많은 가중치를 부여할지 등 어떤 결정을 내렸는지 설명해야 합니다.
When reporting on model development, one must describe how the model was developed and any decisions that were made, such as whether to exclude one predictor or to assign more weight to another predictor.
개발 중 모델 검증
Model validation during development
모델 개발에 대한 일반적인 접근 방식은 데이터를 학습, 테스트, 유효성 검사의 세 가지 데이터 세트로 분할하는 것입니다.
- 첫 번째 데이터 세트인 학습 세트는 모델을 학습시키는 데 사용됩니다.
- 두 번째 데이터 세트인 테스트 세트는 모델의 성능을 평가하여 반복적으로 테스트하는 데 사용됩니다. 모델의 성능이 좋지 않으면 다른 결정을 내리고, 모델을 다시 학습시키고(학습 데이터에서), 모델을 다시 테스트(테스트 데이터에서)함으로써 모델을 반복적으로 개선해야 할 수 있습니다.
- 세 번째 세트는 모델을 검증할 준비가 될 때까지 따로 보관합니다. 모델이 '본 적이 없는' 데이터, 즉 학습이나 반복 테스트에 포함되지 않은 데이터에 대해 성능을 평가합니다. 이 세 번째 세트는 최종 평가 지표 세트를 보고하는 데 한 번만 사용되며, 특히 모델이 전반적으로 잘 작동하고 학습 및/또는 테스트 데이터에 과도하게 적합하지 않도록 알고리즘을 반복적으로 수정하는 데는 사용되지 않습니다.
A common approach to model development is to split the data into three different data sets: training, test and validation.
- The first data set, the training set, is used to train the model.
- The second set, the test set, is used to iteratively test the model by assessing its performance. If the model performs poorly then it may be necessary to iteratively improve the model by making different decisions, re-training the model (on the training data), and re-testing the model (on the test data).
- The third set is set aside until the model is ready for validation. Where its performance is assessed on data that the model has never ‘seen’ meaning that it was not part of either the training or iterative testing. This third set is used only once for reporting a final set of evaluation metrics, and specifically NOT used to iteratively modify the algorithm ensuring that the model performs well overall and does not overfit on the training and/or test data.
단일 데이터 세트가 학습, 테스트 및 유효성 검사 데이터 세트로 분할되는 경우 이를 내부 타당성 검사라고 합니다. 그러나 아직 수집되지 않은 데이터(알고리즘을 일반화/적용하고자 하는 데이터)를 사용하여 외부 타당성 검사을 수행하는 것이 이상적입니다. 예를 들어, 2020년 대학 입학을 설명하는 데이터를 수집하고 이 데이터를 학습, 테스트 및 내부 검증 데이터 세트로 분할한 다음 2021년 대학 입학을 설명하는 데이터를 수집하여 외부 검증을 수행할 수 있습니다(Baron 외. 2020).
When a single set of data are split into training, testing and validation data sets we refer to the validation as an internal validation. However, ideally you would also perform an external validation using data that has not yet been collected (and to which you wish to generalize/apply your algorithm). For example, one might collect data describing university admissions in 2020 and split this data into training, testing and internal validation data sets and then collect data describing university admissions in 2021 for external validation (Baron et al. 2020).
모델 검증 보고 메트릭
Model validation Reporting metrics
최종 모델 검증 지표를 보고할 때는 모델의 성능을 평가하기 위해 어떤 측정값을 사용했는지 설명해야 합니다. AI 연구에 사용되는 일반적인 모델 적합도 지표는 다음과 같습니다:
- R-제곱(R2), 평균 제곱 오차(MSE), 평균 제곱 오차(RMSE).
When reporting the final model validation metrics, one must describe what measures were used to assess the performance of the model. Common model fit metrics used in AI studies include:
- R-Squared (R2), Mean Squared Error (MSE), and Root Mean Squared Error (RMSE).
혼동 행렬(모델 예측과 참조 표준(예: 정밀도, 리콜, 정확도 및 수신기 작동 특성 곡선 아래 면적)을 비교하는 행렬)에서 파생된 측정값도 범주형 결과 측정값의 예측을 평가하는 데 사용됩니다.
Measures derived from the confusion matrix – a matrix comparing model predictions with a reference standard (e.g. precision, recall, accuracy and area under the receiver operating characteristics curve) are also used to evaluate the predictions of categorical outcome measures.
최종 모델을 제시할 때 대부분의 보고서는 가능한 경우 모든 회귀 계수를 신뢰 구간과 함께 포함하지만, 복잡한 모델(특히 신경망)의 경우 불가능할 수 있습니다. 대부분의 모델 유형에 대해 허용되는 보고 규칙이 존재합니다. 의학 교육자는 도메인 전문가와의 협업을 통해 다른 사람들이 모델을 정확하게 평가할 수 있는 방식으로 모델에 대한 설득력 있는 논거를 개발하는 데 도움을 받을 수 있습니다.
When presenting the final model, most reports include all regression coefficients with confidence intervals, if possible, although this may not be feasible for complex models (especially neural networks). Accepted reporting conventions exist for most model types. Collaborations with domain experts can help medical educators develop a compelling argument for their model in a way that allows others to accurately appraise it.
관심 있는 결과에 따라 응답을 구분하는 모델의 능력(변별력) 및 편향되지 않은 응답을 하는 모델의 능력(보정) 외에도, 교육 실무에서 제안된 모델의 사용법을 고려해야 합니다.
- 예를 들어, 모델이 실시간으로 의사 결정에 정보를 제공해야 하는 경우 모델의 응답 시간(즉, 모델이 예측을 내리는 데 필요한 시간)이 중요합니다.
- 다른 예로는 모델 예측의 투명성 및 설명 가능성과 같이 모델이 학습자 또는 교육자에게 정보를 제공하는 방식이 있습니다.
- 일반적으로 모델은 원래 설계된 목적 이외의 용도로 사용해서는 안 됩니다.
In addition to the model’s ability to separate out responses according to the outcome of interest (discrimination) and the model’s ability to make unbiased responses (calibration), the proposed uses of the model in educational practice must be taken into consideration.
- For example, if the model is to inform decisions in real-time then the model’s response time (i.e. the time needed for the model to make a prediction) is important.
- Other examples include how the models are supposed to inform learners or educators, such as transparency and explainability in the model predictions.
- In general, models should not be used for purposes beyond those for which they were originally designed.
의학교육에서의 AI 연구에 대한 추가 평가 고려 사항
Additional evaluation considerations for AI studies in Medical Education
의학교육에서 AI를 사용할 때는 특별히 고려해야 할 사항이 있습니다. 학자들은 모델 자체를 평가하는 것 외에도 교육 연구자가 이론을 AI 알고리즘의 근거와 필요성에 어떻게 통합했는지 먼저 평가하여 연구 전체를 고려해야 합니다. AI가 사용되는 많은 경우(예: 임상 의학)에서 목표는 일반화 가능성입니다. 그러나 의학교육에서는 항상 그렇지는 않을 수 있습니다. 예를 들어, 특정 의과대학이나 레지던트 프로그램에 대한 지원자의 적합성 평가를 지원하기 위한 AI 알고리즘을 개발하는 것은 해당 기관이나 프로그램에는 유용할 수 있지만 다른 기관이나 프로그램에 반드시 일반화할 수는 없습니다(Baron 외. 2020).
There are particular considerations for AI in medical education. Beyond evaluating the model itself, scholars must also consider the study as a whole by first evaluating how the education researcher incorporated theory into the rationale and need for an AI algorithm. In many instances where AI is used (e.g. clinical medicine) the goal is generalizability. However, within medical education this may not always be the case. For example, developing an AI algorithm to support the assessment of the suitability of applicants to a particular medical school or residency program may prove useful for that institution or program, but may not necessarily generalize to other institutions or programs (Baron et al. 2020).
의학교육에 AI 기술을 사용할 때는 개인이 자신의 데이터가 어떻게 사용되는지 이해하는 정도(데이터 사용 및 개인정보 보호 기대치)도 고려해야 합니다. 임상 환경에서 진단과 적절한 치료 계획을 결정하기 위해 AI를 사용하는 경우, 의사는 환자에게 AI 모델의 목적과 의학적 결정에 AI가 어떤 정보를 제공했는지 설명할 수 있습니다. 연구 환경에서는 특히 기존 데이터 세트를 사용하고 소급하여 동의를 받을 수 없는 경우, 이러한 유형의 정보에 입각한 동의를 얻기가 더 어려울 수 있습니다.
When employing AI techniques in medical education, one must also consider the extent to which individuals understand how their data will be used (data usage and privacy expectations). In clinical settings, when AI is used to determine diagnoses and appropriate treatment plans, physicians can communicate to their patients the purpose of the AI models and how medical decisions were informed by them. In a research environment, it may be more difficult to obtain this type of informed consent, especially when pre-existing data sets are used and consent cannot be obtained retroactively.
토론
Discussion
이 가이드에서는 기본 정의부터 실제 사례, 평가 접근 방식에 이르기까지 의학교육 연구에서 AI를 개발하고 사용할 때 고려해야 할 실질적인 사항을 제공합니다. AI에 대한 관심이 높아지고 적용 범위가 확대되는 상황에서 이 가이드는 의료 전문직 교육 분야의 얼리어답터를 위한 실용적인 가이드 역할을 합니다. 제공된 예시에서 알 수 있듯이 의료 교육에서 AI는 에세이 채점이나 대규모 이미지 데이터에 기반한 피드백 제공과 같은 작업을 처리하는 데 이상적입니다. 또한 대부분의 AI는 데이터에 특화되어 있고 일반화가 잘 되지 않으며 광범위한 추가 통계 모델링이 필요한 경우가 많기 때문에 현재의 AI 모델에는 한계가 있음을 인정합니다.
In this guide, we provide practical considerations for the development and use of AI in medical education research, from basic definitions to worked examples to evaluation approaches. Given the growing interest and increased application of AI, this guide serves as an practical guide for early adopters in health professions education. As highlighted by the examples provided, AI in medical education is ideal for handling tasks such as essay scoring or providing feedback based on large-scale image data. We also acknowledge that current AI models are limited; most AI are data-specific, generalize poorly, and often require extensive additional statistical modelling.
AI는 임상의와 연구자로서 우리가 일하는 방식에 점점 더 많은 영향을 미치고 있으며, 유능한 임상의가 된다는 것의 의미에도 영향을 미치고 있습니다. 이에 따라 캐나다 왕립 의사 및 외과의사 대학은 최근 미래 의학 커리큘럼에 AI에 대한 지식을 구축하는 것이 중요하다고 강조했습니다(Royal College 2022). 의료 교육자, 학습자, 연구자가 AI 시스템의 강점, 특히 한계를 이해하려면 새로운 기술이 필요합니다. 예를 들어, 초보 학습자는 좋은 AI 피드백을 통해 가장 많은 것을 얻을 수 있지만(Tschandl 외. 2020), AI 시스템이 제공하는 잘못된 조언에 전문가보다 더 취약할 수 있습니다(Gaube 외. 2021).
Since AI is increasingly affecting how we work as clinicians and researchers, it also affects what it means to be a competent clinician. Accordingly, the Royal College of Physicians and Surgeons of Canada recently stressed the importance of building knowledge about AI into future medical curricula (Royal College 2022). New skills are needed to understand the strengths - and in particular the limitations - of AI systems for medical educators, learners, and researchers. For example, while novice learners may gain the most from good AI feedback (Tschandl et al. 2020), they may be more susceptible than experts to incorrect advice provided by AI systems (Gaube et al. 2021).
학습 및 임상 수행을 지원하기 위한 AI 사용이 증가함에 따라 AI 모델 및 예측의 정확성과 신뢰성뿐만 아니라 한계를 이해하는 것이 중요해질 것입니다. 일부 AI 시스템은 매우 높은 통계적 정밀도를 보여줄 수 있지만 내장된 시스템적 편향으로 인해 여전히 부정확한 결과를 생성할 수 있습니다. 예를 들어, 많은 피부과 AI 지원 진단의 정확도는 연구 참여자의 피부색을 샘플링하는 것으로 제한되어 있어 더 다양한 환자 집단에 사용할 경우 잠재적으로 부정확하거나 편향된 결정을 내릴 수 있습니다(Ternov 외. 2022).
Understanding the limitations as well as the accuracy and reliability of AI models and predictions will be critical with the increased use of AI for supporting learning and clinical performances. Some AI systems may demonstrate extremely high statistical precision but still produce inaccurate results due to built-in systematic biases. For example, the accuracy of many dermatological AI-supported diagnoses is limited to sampling of the available skin colour of the study participants leading to potentially inaccurate or biased decisions when used on a more diverse patient population (Ternov et al. 2022).
의학 교육의 맥락에서, 학습 분석 및 AI에 대한 비평가들은 개인정보 보호 문제, 효율성에 대한 바람직하지 않은 초점을 포함하여 결과물의 의미 부족에 대해 경고했습니다(10 Cate 외. 2020). 이러한 우려 중 일부(예: 개인 정보 보호 및 자율성 문제)는 일반적인 지지를 얻고 있는 것으로 보이지만(쿨라세가람 2022), 빅데이터와 AI의 사용이 교육 환원주의로 이어질 것이라는 우려(반 데르 니트 및 블레이클리 2020)와 같은 다른 우려는 AI 기반 학습 분석에 빅데이터를 사용하는 것과 관련하여 의학교육 커뮤니티 내에서 더 깊은 공리학적 및 인식론적 균열을 나타낼 수 있습니다.
In the context of medical education, critics of learning analytics and AI have warned about privacy issues, lack of meaningfulness in outputs, including an undesirable focus on efficiency (ten Cate et al. 2020). While some of these concerns (for example, privacy and autonomy concerns) seem to gather general support (Kulasegaram 2022), other concerns such as the worry that the use of big data and AI will lead to educational reductionism (van der Niet and Bleakley 2020) may represent deeper axiological and epistemic clefts within the medical education community around the use of big data for AI-powered learning analytics.
AI 시스템에 대한 과도한 의존은 무인 자동차 산업의 오토파일럿 실패 사례에서 알 수 있듯이 잘못된 신뢰와 잠재적인 오류 증가로 이어질 수 있습니다(New York Times 2021). 실제로 의료 교육 맥락에서 상용화된 대부분의 임상 AI 시스템은 임상의의 학습을 개선하기 위한 것이 아니라 임상의의 성과를 지원하기 위해 개발되었습니다. 무인 자동차에 비유하자면, 의료 분야의 기존 AI 시스템은 운전 중 수행을 지원하는 것을 목표로 하지만 운전자의 기술을 향상시키지는 못합니다. 성과를 개선하는 개입이 학습에 부정적인 영향을 미칠 수 있고 그 반대의 경우도 마찬가지이기 때문에 학습과 성과는 종종 상충되는 경우가 많습니다(Schmidt 외. 1990). 지도guidance 가설에서 강조한 바와 같이, 수행 중에 임상의에게 동시에 피드백을 제공하는 기존의 상용 AI 시스템은 수행 후 요약된 피드백을 통해 학습을 지원하도록 설계된 시스템에 비해 학습에 부정적인 영향을 미칠 수 있습니다(Schmidt 외. 1989). 이러한 과제는 새로운 AI 시스템을 개발할 때 학습 과학을 임상 과학 및 데이터 과학과 통합하여 AI 시스템에 대한 과도한 의존과 학습자의 임상 기술에 대한 잠재적인 부정적인 영향을 방지하는 동시에 전문가 수준의 자동화된 지침 및 피드백에 대한 액세스의 이점을 누리는 것이 중요하다는 점을 강조합니다.
Over-reliance on AI systems can lead to false confidence and potentially increased errors as demonstrated from the autopilot failures in the driverless car industry (New York Times 2021). Indeed, in medical education contexts, most commercially available clinical AI systems are developed to support clinicians’ performances and not to improve their learning. To use the driverless car analogy, existing AI systems in healthcare aim to support performances while driving, but fail to improve drivers’ skills. Learning and performance are often at odds (Schmidt et al. 1990) as interventions that improve performance can negatively affect learning and vice versa. As highlighted by the guidance hypothesis, existing commercially available AI systems that provide concurrent feedback to clinicians during performances may negatively influence learning as compared to systems that are designed to support learning - for example through summarized feedback that is provided post-performance (Schmidt et al. 1989). These challenges highlight the importance of integrating learning sciences with clinical science and data science when developing new AI systems to prevent an overreliance on AI systems and potential negative effects on learners’ clinical skills, while also reaping the benefits of access to expert-level automated guidance and feedback.
이를 위해서는 AI 시스템 기술 개발 초기에 교육 이론과 개념을 통합하는 데 더 중점을 두어야 합니다. 기존의 AI 연구는 현재 임상 저널에 기술된 응용 연구와 학회 회의록에 보고된 기술 보고서 사이에 양극화되어 있습니다(Topol 2019). 의학교육에서는 다양한 맥락에서 일반화하고자 하는 욕구 때문에 이러한 양극화가 문제가 될 수 있습니다(Tolsgaard). 이러한 의미에서 의학교육에서의 AI 연구는 10~20년 전 시뮬레이션 문헌에서 관찰된 것과 동일한 단점, 즉 AI가 언제, 왜, 누구에게 가치를 제공할 수 있는지, 위험과 과제를 탐구하려는 연구보다 'AI가 효과가 있다'는 정당화 연구가 과대 대표되는 것을 반복할 위험이 있습니다{Issenberg}.
The key to doing this may involve a greater focus on integrating educational theory and concepts earlier within the technical development of AI systems. Existing AI research is currently polarized between applied work described in clinical journals and technical reports reported in conference proceedings (Topol 2019). In medical education, this may constitute a problem because of the desire to generalize across different contexts (Tolsgaard). In this sense, AI research in medical education risks repeating some of the same shortcomings as observed in the simulation literature 10-20 years ago: the overrepresentation of justification studies demonstrating that ‘AI works’ over work that intends to explore when, why and for whom AI may provide value, risks and challenges.{Issenberg}
요약하자면, AI는 교육 제공과 교육 연구 모두에서 점점 더 많이 사용되고 있습니다. 학습 과학을 의료 분야의 AI 시스템 개발 및 사용에 통합하는 것은 앞으로 몇 년 동안 계속 도전 과제가 될 것입니다. AI는 의학교육 커뮤니티에 특별한 기회를 제공할 수 있지만, 의학교육자, 교육 연구자, 데이터 과학자, 임상의 간의 긴밀한 학제 간 협업을 필요로 합니다. 의학교육에서 AI에 신중하고 주의 깊게 접근하면 학습과 성과는 물론 설명 가능성과 투명성을 지원하는 AI 시스템을 개발할 수 있습니다.
To summarize, AI is increasingly being used in both the provision of education and in education research. Integrating learning sciences into the development and uses of AI systems in healthcare is likely going to continue to be a challenge in the years to come. While potentially offering unique opportunities for the medical education community, AI requires close interdisciplinary collaborations between medical educators, education researchers, data scientists, and clinicians. In approaching AI in medical education with caution and care, we can enable the development of AI systems that support learning and performance as well as explainability and transparency.
The fundamentals of Artificial Intelligence in medical education research: AMEE Guide No. 156
PMID: 36862064
Abstract
The use of Artificial Intelligence (AI) in medical education has the potential to facilitate complicated tasks and improve efficiency. For example, AI could help automate assessment of written responses, or provide feedback on medical image interpretations with excellent reliability. While applications of AI in learning, instruction, and assessment are growing, further exploration is still required. There exist few conceptual or methodological guides for medical educators wishing to evaluate or engage in AI research. In this guide, we aim to: 1) describe practical considerations involved in reading and conducting studies in medical education using AI, 2) define basic terminology and 3) identify which medical education problems and data are ideally-suited for using AI.
Keywords: Artificial Intelligence; assessment; big data; machine learning; medical education.