보건의료전문직 교육에서 인공지능의 윤리적 사용: AMEE Guide No. 158 (Med Teach, 2023)
Ethical use of artificial intelligence in health professions education: AMEE Guide No.158
Ken Masters
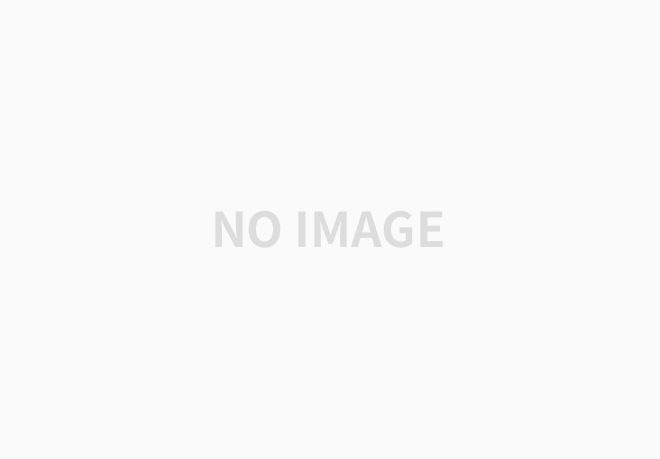
소개
Introduction
인공 지능: 배경과 의료 전문직
Artificial intelligence: background and the health professions
1950년 앨런 튜링은 '기계도 생각할 수 있는가'라는 질문을 던졌고(Turing 1950), 그 후 기계와 사고의 정의에 대한 철학적 논의가 이어졌습니다. 그로부터 불과 5년 후, 존 매카시와 동료들은 '인공 지능'(AI)이라는 용어를 도입했습니다(McCarthy 1955). 이들은 이 용어를 정확하게 정의하지는 않았지만 '학습의 모든 측면이나 지능의 다른 특징은 원칙적으로 기계로 시뮬레이션할 수 있을 정도로 정확하게 기술될 수 있다는 추측'을 제기했고, 이후 이 용어는 인간의 지적 활동과 능력을 모방하고 확장하도록 설계된 컴퓨터 시스템의 활동을 지칭하는 데 사용되었습니다.
In 1950, Alan Turing posed the question ‘Can machines think?’ (Turing 1950) and followed it with a philosophical discussion about definitions of machines and thinking. Barely five years later, John McCarthy and colleagues introduced the term ‘Artificial Intelligence’ (AI) (McCarthy 1955). Although they did not precisely define the term, they did raise the ‘conjecture that every aspect of learning or any other feature of intelligence can in principle be so precisely described that a machine can be made to simulate it’ and the term has been used since then to refer to activities by computer systems designed to mimic and expand upon the intellectual activities and capabilities of humans.
AI는 현재 인간의 모든 탐구 분야에 영향을 미치고 있으며, 보건 전문직의 연구 분야로 확고히 자리 잡았습니다. '인공 지능'이라는 문구로 PubMed를 검색하면 지난 20년간 발표된 약 6만 개의 논문이 검색되며, 일반적으로 AI는 '의료 시스템에 필수적인 요소'로 간주되고 있습니다(Lattouf 2022). 보건 전문직 교육(HPE)에서 AI에 초점을 맞춘 논문은 AMEE 가이드, 무작위 대조 시험, 체계적 문헌 고찰 등 다양한 전형적인 논문 유형을 보여줍니다(Lee et al. 2021).
AI currently influences every field of human enquiry, and is well-established as a research area in the health professions: a PubMed search with the phrase ‘Artificial Intelligence’ returns some 60,000 articles published in the last 20 years, and AI is generally regarded as ‘integral to health-care systems’ (Lattouf 2022). In Health Professions Education (HPE), articles focusing on AI show a range of typical paper types, including AMEE Guides, randomised controlled trials, and systematic literature reviews (Lee et al. 2021).
HPE의 윤리
Ethics in HPE
HPE에서 AI 사용의 맥락에서 윤리의 역할이 명확하지 않은데, 이는 HPE 기관에서 윤리를 바라보는 방식이 다르기 때문입니다.
The role of ethics in the context of AI usage in HPE is less clear, not least because of the way in which ethics is viewed in HPE institutions.
첫째, HPE 기관에는 연구 윤리 위원회 또는 임상 윤리 위원회가 있지만 교육 윤리 위원회는 없는 것이 일반적입니다. (학업 청렴성 책임자 또는 이와 유사한 직책이 존재할 수 있지만, 이 역할에 대한 설명은 일반적으로 교사가 아닌 학생의 학업 청렴성에 초점을 맞추고 있습니다(Vogt and Eaton 2022)). 사실, 기관 윤리 또는 심의 위원회(IRB)에 대해 말할 때 교육 관행이 아닌 연구에 대해 말하는 것으로 가정합니다. 교수진은 연구 프로토콜을 전문 연구 윤리 위원회에 정기적으로 제출하지만, 교육 윤리 위원회에는 강의 개요를 정기적으로 제출하지 않습니다.
Firstly, it is routine for HPE institutions to have Research Ethics Boards or Clinical Ethics Boards, but not Educational Ethics Boards. (Although the post of Academic Integrity Officer or similar may exist, descriptions of the role usually focus on the academic integrity of students, not teachers (Vogt and Eaton 2022)). In fact, when one speaks of an Institutional Ethics or Review Board (IRB), the assumption is that one is speaking of research, and not education practice. Faculty routinely submit research protocols to specialised research ethics committees, but do not routinely submit course outlines to educational ethics committees.
둘째, HPE에서 '평가'와 '연구'의 경계가 자주 모호해짐에도 불구하고(Sandars 외. 2017), 많은 대학에서 코스 평가 학생 데이터를 연구 데이터로 분류하지 않거나 '질 개선'으로 분류하여 이러한 데이터 수집, 저장 및 사용에 대한 사전 윤리 승인을 요구하지 않습니다. 또는 일부 기관에서는 '면제 상태로 승인'이라는 다소 모호한 용어를 사용하기도 합니다.
Secondly, despite a frequent blurring between ‘evaluation’ and ‘research’ in HPE (Sandars et al. 2017), many universities do not classify course evaluation student data as research data, or they classify them as ‘quality improvement’, and so do not require prior ethics approval for such data gathering, storage and use. Alternately, some institutions use the rather enigmatic term ‘approved with exempt status’.
셋째, 국가별 법률에 따라 상황이 더욱 불분명해집니다:
- 미국에서는 보건복지부(규정 46. 104 (d) (HHS 2021)는 참가자가 식별되지 않는 한 사실상 모든 교실 활동 연구를 면제하는 것으로 보이며,
- 네덜란드 법은 교육 연구를 '윤리 검토 면제'로 자동 결정합니다(ten Cate 2009);
- 덴마크 법은 '프로젝트에 인간 생물학적 자료가 포함되지 않는 한'(såfremt projektet omfatter menneskeligt biologisk materiale) 모든 건강 조사에 대해 국가 윤리의 사전 승인을 자동으로 부여하며(Sundheds- og Ældreministeriet 2020),
- 핀란드는 15세 이상의 사람들을 대상으로 한 조사에 대해 윤리 승인을 요구하지 않습니다(FNB 2019).
Thirdly, national legislation further clouds the picture:
- In the USA, the Department of Health and Human Services (Regulation 46.104 (d) (HHS 2021) appears to exempt virtually all research of classroom activities as long as participants are not identified;
- Dutch law automatically determines education research ‘exempt from ethical review’ (ten Cate 2009);
- Danish law automatically grants national ethics pre-approval to all health surveys, unless the ‘the project includes human biological material’ (såfremt projektet omfatter menneskeligt biologisk materiale) (Sundheds- og Ældreministeriet 2020), and
- Finland does not require ethics approval for surveys of people over the age of 15 (FNB 2019).
이러한 불일치로 인해 [HPE 저널]은 연구자가 '평가' 데이터로 간주하여 사전 윤리 승인을 받지 않은 학생 설문조사를 다룬 원고를 받고, [HPE 기관]은 사전 윤리 승인을 요구하지 않는 불편한 상황이 발생하고 있습니다. 그 결과, 저널이 연구자에게 연구에 대한 윤리 승인을 받도록 점점 더 압력을 가하고 있기 때문에 저널과 의학교육 연구자 사이에 긴장이 존재하지만(10 Cate 2009, Sandars 외. 2017, Masters 2019, Hays and Masters 2020), 기관이 국가 지침을 따르고 윤리 신청 검토를 거부할 경우 문제가 발생합니다.
These inconsistencies lead to an uncomfortable situation in which HPE journals receive manuscripts dealing with student surveys for which researchers have not obtained prior ethics approval because these data were considered ‘evaluation’ data, and HPE institutions have not required prior ethics approval. As a result, a tension exists between journal and medical education researchers, because journals are increasingly pressuring researchers to obtain ethics approval for their research (ten Cate 2009; Sandars et al. 2017; Masters 2019; Hays and Masters 2020), but this causes problems when an institution follows national guidelines, and declines to review the ethics application.
HPE의 AI 윤리
AI ethics in HPE
이 불투명한 영역에 AI가 도입되었고, 아래 논의에서 볼 수 있듯이 AI는 많은 연구자(및 기관)가 준비되지 않은 윤리적 복잡성을 증폭시킵니다.
Into this murky area, AI is introduced, and, as will be seen from the discussion below, AI amplifies the ethical complexities for which many researchers (and institutions) are unprepared.
일부 국가와 지역에서는 광범위한 AI 원칙과 윤리를 수립했지만, 교육에 대한 언급은 매우 광범위하며, 대부분 사람들에게 AI와 AI 윤리에 대한 교육의 필요성을 언급하고 있습니다(예: (HLEG 2019)). 최근 터키에서 Celik이 실시한 소규모(4문항) 연구에서는 초중고 교사의 교육 윤리 및 AI에 대한 지식을 평가했습니다(Celik 2023). 현재 HPE에는 AI에 관한 많은 논문이 있지만 기술적이고 실용적인 측면에 초점을 맞추는 경우가 많으며, 윤리적 논의는 주로 의료 또는 보건 전문직 교육에서 AI의 윤리적 사용보다는 의료 행위 또는 AI를 사용한 임상 활동에서 AI가 윤리에 미치는 영향에 관한 것입니다(Chan and Zary 2019, Masters 2019, Masters 2020a, Rampton 외. 2020, Çalışkan 외. 2022, Cobianchi 외. 2022, Grunhut 외. 2022).
Some countries and regions have set up broad AI principles and ethics, but their mention of education is very broad, mostly referring to the need to educate people about AI and AI ethics (e.g. (HLEG 2019). A recent, small (4-questions) study in Turkey by Celik assessed K-12 teachers’ knowledge of ethics and AI in education (Celik 2023). Although there are now many articles on AI in HPE, they frequently focus on the technical and practical aspects, and the ethical discussion refers primarily to the impact on ethics of AI in medical practice or clinical activities using AI, rather than the ethical use of AI in medical or health professions education (Chan and Zary 2019; Masters 2019; Masters 2020a, Rampton et al. 2020; Çalışkan et al. 2022; Cobianchi et al. 2022; Grunhut et al. 2022).
최근 HPE에서 AI의 윤리적 사용에 대한 우려가 제기되고 있지만(Arizmendi 외, 2022), 현재 HPE 교사가 교육에서 AI를 윤리적으로 사용할 수 있는 방법에 대한 지침은 거의 없습니다. 따라서 HPE에서 AI를 사용할 때 윤리의 복잡성에 대한 보다 직접적인 인식이 필요합니다. 이러한 복잡성에는 다음 등이 포함됩니다.
- 수집되는 데이터의 양,
- 익명성 및 개인정보 보호,
- 동의,
- 데이터 소유권,
- 보안,
- 데이터 및 알고리즘 편향성,
- 투명성,
- 책임,
- 자율성,
- 유익성
Although there is recent concern about ethical use of AI in HPE (Arizmendi et al. 2022), there is currently little to guide the HPE teacher in how to ensure that they are using AI ethically in their teaching. As a result, a more direct awareness of the complexities of ethics while using AI in HPE is required. These complexities include those around
- the amount of data gathered,
- anonymity and privacy,
- consent,
- data ownership,
- security,
- data and algorithm bias,
- transparency,
- responsibility,
- autonomy, and
- beneficence.
이 가이드는 이러한 요건을 충족하기 위해 교육기관, 교사, 학생 간의 관계에 초점을 맞추고 있습니다. 또한 환자와의 상호작용에 대한 논의도 일부 포함되지만, 이러한 윤리적 문제는 의료 윤리에서 가장 잘 다루고 있으므로 이 가이드의 초점은 아닙니다.
This Guide attempts to meet that requirement, and will focus on the relationships between institution, teacher, and student. In addition, while there will be some discussion of the interactions with patients, those ethical issues are best covered in medical ethics, and so are not the focus of this guide.
많은 독자가 AI와 관련된 윤리적 문제의 함의에 익숙하지 않을 수 있으므로, 각 주제에서는 가능한 해결책을 설명하기 전에 문제의 성격과 범위를 자세히 설명합니다. 어떤 경우에는 앞으로 나아갈 길이 항상 명확하지 않을 수도 있지만, 문제와 그 범위에 대한 인식은 문제 해결의 첫걸음입니다.
Because many readers may be unfamiliar with the implications of ethical issues in light of AI, each topic will, of necessity, detail the nature and scope of the problem before describing possible solutions. In some cases, the path ahead might not always be clear, but an awareness of the problem and its scope is a first step to solving that problem.
또한 2022년 11월에 Generative Pre-trained Transformer(GPT)라는 AI 시스템이 일반에 공개되었으며, 이 릴리스에서는 HPE에서 고려해야 할 윤리적 문제에 대한 많은 실제 사례가 강조되었으므로 이 시스템에 대한 언급이 자주 이루어질 것입니다. (AI 측면에서 ChatGPT 이전의 세상과 이후의 세상을 비교하고 싶을 수도 있지만, 가장 중요한 차이점은 개발보다는 공개 및 접근성이라는 점을 기억해야 합니다.)
In addition, the AI system named Generative Pre-trained Transformer (GPT) was released to the public in November 2022, and this release highlighted many practical examples of ethical issues to be considered in HPE, so frequent reference to that system will be made. (In AI terms, one might even be tempted to consider a pre-ChatGPT world vs. a post-ChatGPT world, but it must be remembered that the major significant difference was its public release and accessibility, rather than its development).
한 가지 윤리적 문제는 ChatGPT의 원칙에 관한 것인데, 윤리적 원칙과 정책에 대한 질문에 대한 답변은 '어떤 윤리적 원칙이나 정책도 따를 능력이 없다'는 것입니다(부록 1 참조). 윤리에 대한 이러한 접근 방식은 교육 기술은 본질적으로 윤리적으로 중립적이라는 믿음을 연상시키는데, 이는 이미 오래 전에 반박된 입장입니다. 이러한 인식의 함의는 가이드의 뒷부분에서 언급될 것입니다.
One ethical complication concerns ChatGPT’s principles: its response to a query on its ethical principles and policies is that it does ‘not have the capacity to follow any ethical principles or policies’ (see Appendix 1). This approach to ethics is reminiscent of the belief that educational technology is inherently ethically neutral – a position long-since debunked. The implications of this perception will be referred to later in the Guide.
그런 다음 가이드는 취해야 할 첫 번째 단계에 대한 광범위한 논의로 끝납니다.
The Guide then ends with a broader Discussion of the first steps to be taken.
약간의 기술적인 내용
A little technical
이 가이드는 지나치게 기술적인 내용을 피하기 위해 머신 러닝, 딥 러닝 및 기타 AI 용어의 정의로 인해 산만해지지 않도록 작성되었지만, 일부 기술 전문 용어가 필요합니다. 이 시점에서 독자들에게 익숙하지 않을 수 있는 두 가지 기술 개념, 즉 알고리즘과 모델에 대해 설명할 필요가 있습니다.
This Guide avoids being overly-technical, and so will not become distracted with definitions of machine learning, deep learning, and other AI terminology, but some technical jargon is required. At this point, it is necessary to explain two technical concepts that may not be familiar to readers: the algorithm and the model.
이 백서의 목적상 알고리즘은 컴퓨터 시스템이 문제를 해결하기 위해 따르는 단계별 프로세스라는 것만 알면 됩니다. 일부 알고리즘은 전적으로 사람이 설계하고, 일부는 컴퓨터가 설계하며, 일부는 이 두 가지를 혼합하여 설계합니다. AI에서는 알고리즘이 매우 복잡할 수 있습니다. 알고리즘은 아래 주제에서 다시 언급됩니다.
For the purposes of this paper, it is necessary to know only that an algorithm is a step-by-step process followed by a computer system to solve a problem. Some algorithms are designed entirely by humans, some entirely by computers, and some a hybrid mix of both. In AI, the algorithms can be extremely complex. Algorithms are referred to again in the topics below.
모델은 알고리즘을 사용하여 작업(예: 예측 또는 이미지 분류)을 수행하는 전체 컴퓨터 프로그램입니다. 모델이라는 단어는 다른 많은 분야에서 사용되기 때문에 이 가이드에서는 혼동을 줄이기 위해 주로 알고리즘을 지칭합니다. [모델에 대한 자세한 내용은 Klinger (2021)를 참조하세요.]
A model is the full computer program that uses the algorithms to perform tasks (e.g. making predictions, or classifying images). Because the word model is used in so many other fields, this Guide will refer primarily to algorithms, to reduce confusion. [For a little more detail on models, see Klinger (2021).]
우려되는 주요 윤리 주제
The main ethics topics of concern
과도한 데이터 수집 방지
Guarding against excessive data collection
HPE 연구에서는 학생으로부터의 과도한 데이터 수집에 대한 우려가 있습니다(Masters 2020b). 학생 및 교직원 설문조사와 같은 전통적인 HPE 연구에서 윤리 승인이 필요한 경우 IRB는 데이터 수집 도구를 검토하고 특정 데이터 범주 및 질문의 관련성을 질의하며, 연구자는 이러한 데이터의 필요성을 정당화해야 합니다. 정당화는 일반적으로 가설을 테스트하거나 결과를 문헌에 제시된 결과와 비교할 수 있도록 하기 위한 것입니다.
In HPE research, there is a concern about excessive data collection from students (Masters 2020b). In traditional HPE research (such as student and staff surveys), in the cases when ethics approval is required, IRBs review data collection tools and query the relevance of specific data categories and questions, and researchers must justify the need for those. Justifications are usually for testing hypotheses, or so that results can be compared to findings given in the literature.
IRB 승인에는 약점이 있을 수 있지만, 이 프로세스는 수집할 데이터를 미리 식별하고 집중하며 사전 승인된 설문조사 양식 및 기타 데이터 수집 도구로 직접 추적할 수 있기 때문에 과도한 데이터 수집을 줄이는 데 어느 정도 도움이 됩니다.
Although there may be weaknesses in IRB-approval, the process does go some way in reducing excessive data collection: the data to be collected are pre-identified, focused, and can be traced directly to the pre-approved survey forms and other data-gathering tools.
AI는 데이터 수집 프로세스를 변화시킵니다. AI의 강점은 다양한 데이터 세트에서 대량의 데이터를 수집하고 사용하는 빅데이터의 활용입니다. 그 결과, 이전에는 노이즈로 간주되었던 데이터가 관련성이 있는 것으로 밝혀지고 이전에는 알려지지 않았던 패턴이 식별되는 경우가 많습니다. 임상 치료에서 임상적 '관련성' 문제는 수년 동안 관심의 대상이 되어 왔으며(Lingard and Haber 1999), 관련성이 없어 보이는 대량의 데이터를 사용한 최근 사례로는 AI 시스템이 엑스레이 이미지에서 사람의 인종을 판별할 수 있었던 연구가 있습니다(Gichoya 외. 2022).
AI changes the data collection process. A strength of AI is the use of Big Data: the collection and use of large amounts of data from many varied datasets. As a result, data that might previously have been considered noise are frequently found to be relevant, and previously unknown patterns are identified. In clinical care, the issue of clinical ‘relevance’ has been of concern for many years (Lingard and Haber 1999), and a recent example of using large amounts of seemingly irrelevant data is a study in which an AI system was able to determine a person’s race from x-ray images (Gichoya et al. 2022).
HPE에서 AI 사용의 윤리적 문제를 이해하려고 할 때, 빅데이터는 단순히 데이터가 조금 더 많은 것이 아니라 전례 없는 규모의 데이터의 양과 유형을 의미한다는 점을 이해하는 것이 중요합니다. HPE에서 빅데이터의 출발점은 학습 관리 시스템(LMS)과 같은 교육기관 소스에서 쉽게 전자적으로 수집할 수 있는 학생 데이터 또는 학습 분석(Ellaway 외. 2014, Goh and Sandars 2019, ten Cate 외. 2020)이며, 임상 학생의 경우 전자 건강 기록(Pusic 외. 2023)에서도 수집할 수 있습니다. 데이터 기반 의사 결정을 사용하여 각 학생에게 최상의 학습 결과를 제공하거나 행동 패턴을 예측하는 것은 일반적으로 칭찬할 만한 목표입니다.
When trying to understand the ethical concerns of using AI in HPE, it is crucial to understand that Big Data does not mean just a little more data, but to amounts and types of data on an unprecedented scale. In HPE, a starting point for Big Data is the obvious student data, or Learning Analytics (Ellaway et al. 2014; Goh and Sandars 2019; ten Cate et al. 2020) that can be easily electronically harvested from institutional sources, such as Learning Management Systems (LMSs), and, for clinical students, from Electronic Health Records (Pusic et al. 2023). The aims are generally laudable: using data-driven decisions to provide the best possible learning outcome for each student or to predict behaviour patterns.
그러나 AI에 필요한 학습 분석과 빅 데이터는 우리가 일반적으로 교육과 연관 짓는 단순한 데이터(예: 시험 점수)보다 훨씬 더 많은 것을 의미합니다. AI 없이도 의대생을 대상으로 한 통계 연구에서는 행동, 성과, 인구 통계를 조사하여 결과를 예측했습니다(Sawdon and McLachlan 2020; Arizmendi 외. 2022). 소셜 미디어에서 얻은 교훈에 따라 이제 데이터에는 훨씬 더 광범위한 개인 및 행동 데이터도 포함될 수 있습니다.
- 얼굴 및 음성 지문, 시선 추적, 클릭 수, 휴식 시간, 스크린 타임, 위치, 일반적인 관심사, 다른 사람과의 온라인 상호 작용, 검색 기록, 로컬(예: 집) 네트워크의 다른 기기에 대한 매핑 등
그런 다음 다른 측면과 결합하여 추가로 해석합니다(예: 얼굴 및 텍스트 분석, 감정 상태를 유추하기 위한 스트레스 수준).
Learning Analytics and Big Data required for AI, however, go much further than the simple data that we commonly associate with education (e.g. test scores). Even without AI, statistical studies of medical students have examined behaviour, performance, and demographics to predict outcomes (Sawdon and McLachlan 2020; Arizmendi et al. 2022). Following lessons learned from social media, the data can now also include a much wider range of personal and behavioural data,
- such as face- and voice-prints, eye-tracking, number of clicks, breaks, screentime, location, general interests, online interactions with others, search history, and mapping to other devices on the local (i.e. home) network.
These are then combined with other aspects and further interpreted (e.g. facial and textual analyses and stress levels to infer emotional status).
또한 학생(및 교직원) 지원서, 재무 기록, 일반 정보, 전자 장소 액세스, 네트워크 로그 파일, 동문 데이터 등을 기록하는 데이터베이스를 포함하여 더 광범위한 교육기관 데이터베이스로 확장되고 있습니다.
In addition, there is the expansion into a wider range of institutional databases, including those that record student (and staff) applications, financial records, general information, electronic venue access, network log files, and alumni data.
마지막으로, 빅 데이터는 교육기관이 관리하는 데이터베이스를 뛰어넘습니다: AI는 기관 외부의 인터넷 시스템(예: 소셜 미디어 계정)을 사용하여 '전례 없는 폭과 깊이, 규모로 데이터를 수집하고 분석할 수 있는 역량을 활용하는' 컴퓨터 사회과학을 가능하게 합니다(Lazer 외. 2009). 업계의 일반적인 관행에 따라 이러한 데이터를 사용하여 각 사용자에 대한 고유한 프로필을 만들 수 있습니다.
Finally, Big Data goes beyond institutional-controlled databases: AI uses internet systems (e.g. social media accounts) outside the institution, allowing for computational social science that ‘leverages the capacity to collect and analyze data with an unprecedented breadth and depth and scale’ (Lazer et al. 2009). Following common practices in industry, these data can be used to create unique profiles of each user.
이러한 데이터는 일반적으로 '수동적 데이터 수집'으로 알려진 프로세스를 통해 숨겨진 전자적 수준에서 수집되며, 참여자는 데이터 수집 프로세스를 인식하지 못할 수 있습니다. 유일한 윤리적 요구 사항은 사용자가 '이용약관'을 읽었음을 나타내는 클릭 한 번으로 보이며, 우리 모두는 학생들이 약관을 얼마나 꼼꼼히 읽(지 않)는지 알고 있습니다. 과도한 데이터 수집으로 인한 윤리적 문제와 함께, 임의의 항목들 사이에서 가짜 패턴과 관계를 찾는 아포페니아의 위험도 증가합니다.
These data are usually collected at a hidden, electronic level, in a process known as ‘passive data gathering’, in which participants may not be aware of the data-gathering process. The only ethical requirement appears to be a single click to indicate that the user has read the ‘Terms and Conditions’; and we all know how closely our students read those: about as closely as we read them. Accompanying the ethical problems of excessive data-gathering, there is also the increased risk of apophenia: finding spurious patterns and relationships between random items.
결과적으로, 이러한 드래그넷 접근 방식에서는 관련 없는 데이터가 대량으로 수집될 위험이 있습니다.
As a result, in this seeming drag-net approach, there is the risk that a great amount of irrelevant data are gathered.
이러한 위험을 줄이고 과도한 학생 및 교직원 데이터 수집을 방지하기 위한 첫 번째 단계로, 연구 프로토콜을 연구 IRB에서 모니터링하는 것과 동일한 방식으로 HPE의 능동적 및 수동적 데이터 수집에 대한 기관 프로토콜을 명확하게 정의하고, 정당화하며, 적용하고, 모니터링해야 합니다. 이러한 프로토콜은 일반적인 교육, 공식 연구 및 '평가' 데이터 수집에 요구되어야 합니다.
As a first step in reducing this risk and to guard against excessive student and staff data-gathering, institutional protocols for active and passive data-gathering in HPE need to be clearly defined, justified, applied and monitored in the same way that research protocols are monitored by research IRBs. These protocols should be required for general teaching, formal research, and ‘evaluation’ data-gathering.
이러한 예방 조치는 온라인 교육에만 적용되어서는 안 됩니다. 과도한 데이터 수집의 위험은 일반적으로 대면 교육보다 온라인 교육에서 더 크지만, 대면 상황에서 소극적인 데이터 수집을 위한 도구도 존재하며, 이러한 도구를 사용하려면 데이터 사용 방법에 대해 세심한 주의를 기울여야 합니다. 테라데이타에서 만든 한 가지 도구의 예는 (Bornet 2021)에서 확인할 수 있습니다.
These preventative steps should not apply to online teaching only. Although the danger of excessive data gathering is generally greater in online teaching than in face-to-face teaching, tools also exist for passive data gathering in face-to-face situations, and, if these tools are to be used at all, great care should be taken about how the data are used. An example of one tool, created by teradata is demonstrated by (Bornet 2021).
또한, 기관을 넘어 데이터 수집 영역이 크게 확대됨에 따라 IRB와 국가 기관은 윤리 승인 요건을 시급히 재검토하여 AI 데이터 수집 프로세스의 발전에 여전히 적합한지 확인해야 합니다. 즉, 윤리 승인은 설문조사 데이터와 기관 및 외부 데이터베이스의 데이터 간의 관계를 충분히 고려할 수 있도록 미묘한 차이를 두어야 할 것입니다.
In addition, given the massive enlargement of the data catchment area beyond the institution, IRBs and national bodies need to urgently re-visit their ethics approval requirements, to ensure that they are still appropriate for advances in AI data-gathering processes. This will mean that ethical approval will need to be nuanced enough to account for the relationship between survey data and data in institutional and external databases.
이 주제에 대한 자세한 내용은 (Shah 외. 2012) 및 (Boyd and Crawford 2011)을 참조하세요.
For further reading on this topic, see (Shah et al. 2012) and (Boyd and Crawford 2011).
익명성 및 개인정보 보호
Protecting anonymity and privacy
이렇게 많은 양의 학생 데이터가 수집되면 학생의 익명성과 개인정보가 침해될 위험이 있습니다. 이러한 위험은 저널에서 연구 논문과 함께 원시 데이터를 제출하도록 장려하거나 요구하는 FAIR(Wilkinson 외. 2016) 및 오픈 사이언스의 선의의 원칙으로 인해 더욱 악화될 수 있습니다.
Given this large amount of student data collected, there is a risk to student anonymity and privacy. This risk is possibly exacerbated by the well-meant principles of FAIR (Wilkinson et al. 2016) and Open Science, through which journals increasingly encourage or require raw data to be submitted with research papers.
학생의 익명성 및 개인정보 보호 문제에 대한 부분적인 해결책이 발견되었습니다. 예를 들어, 연구자는 개인적 특성(예: 아버지 이름의 첫 글자)을 기반으로 임시 피험자 생성 식별 코드(SGIC: Damrosch 1986)를 생성하여 학생의 신원을 익명화할 수 있습니다. 또한 FAIR 및 오픈 사이언스 요건을 충족하기 위해 데이터를 추가로 익명화(또는 비식별화)하는데, 이 과정에는 일반적으로 개인 식별 정보(PII)(예: 사회보장/보험 번호, 우편번호)를 제거하거나 세부 정보를 숨기기 위해 데이터를 그룹화/분류하는 작업이 포함됩니다(McCallister 외. 2010).
Partial solutions to the problem of protecting student anonymity and privacy have been found. For example, researchers can anonymise student identities by creating temporary subject-generated identification codes (SGICs: Damrosch 1986) based on personal characteristics (e.g. first letter of father’s name). In addition, to meet the FAIR and Open Science requirements, data are further anonymised (or de-identified), a process usually involving removing Personally-Identifying Information (PII) (e.g. social security/insurance numbers, zip/postal codes), or data-grouping/categorisation in order to hide details (McCallister et al. 2010).
안타깝게도 데이터를 다른 데이터 세트와 상호 참조하여 개인을 재식별하는 데이터 비-익명화(또는 재-식별화)가 잘 발달되어 있기 때문에, 연구자들은 완전한 데이터 익명화는 신화라는 것을 알고 있습니다(Narayanan and Shmatikov 2009). 빅데이터가 사용하는 데이터 세트의 수가 계속 증가하고 AI 기능이 더욱 강력해짐에 따라 데이터 비익명화의 위험은 더욱 커지고 있습니다. 소셜 미디어 데이터베이스와 결합하면 SGIC의 모든 정보에 쉽게 액세스할 수 있으며, 이러한 SGIC는 쉽게 익명화할 수 있습니다.
Unfortunately, researchers know that complete data anonymisation is a myth (Narayanan and Shmatikov 2009) because data de-anonymisation (or re-identification), in which data are cross-referenced with other data sets and persons are re-identified, is well-developed. With the ever-increasing number of data sets used by Big Data, and more powerful AI capabilities, the risk of data de-anonymisation grows. When coupled with social media databases, all the information in SGICs is easily accessible, and these SGICs are easily de-anonymised.
로컬 규모에서도 데이터 비-익명화가 가능합니다. 예를 들어, 교육기관은 LMS를 사용하여 익명 학생 설문조사를 실시하는데, 부록 2에서는 인기 있는 LMS의 익명 데이터를 쉽게 비익명화할 수 있는 방법을 보여주는 사례를 제시합니다.
Even on a local scale, data de-anonymisation is possible. For example, institutions use LMSs to run anonymous student surveys, and Supplementary Appendix 2 gives an example case showing how anonymous data from a popular LMS can be easily de-anonymised.
교육 시스템이 더욱 정교해짐에 따라 데이터 비익명화의 위험은 증가합니다. 자동화된 '개인화된' 교육에는 추적이 필요하며, HPE 교육기관은 업계와 협력하여 비식별 데이터에 AI 기술을 사용할 수 있지만 데이터에 대한 통제력이 부족하면 데이터 소유권 및 공유 문제로 이어질 수 있습니다(Boninger와 Molnar 2022).
The risk of data de-anonymisation increases as teaching systems become more sophisticated: automated ‘personalised’ education requires tracking, and HPE institutions may team up with industry to use their AI technology on de-identified data, but lack of control of the data can lead to problems of data ownership and sharing (Boninger and Molnar 2022).
또한, HPE 데이터는 [정성적인 경우]가 많고, 이로 인해 비식별화 위험이 높기 때문에 연구자들이 데이터를 다른 사람과 공유하기를 꺼리는 이유 중 일부이기도 합니다(Gabelica 외. 2022). AI의 자연어 처리(NLP)는 이미 의미론적 분류를 통해 항목을 식별하고 분류하는 데 사용되고 있지만(Tremblay 외. 2019; Gin 외. 2022), 사람들은 사회적 집단에 속하며 언어 사용과 이러한 사회적 집단 사이에는 밀접한 연관성이 있습니다(Byram 2022). 따라서 언어적 특성과 행동(예: 맞춤법, 문법, 구어체, 특정 스포츠팀 응원 등)의 조합과 같은 비개인정보(non-PII)를 통해 사람을 식별하는 NLP의 능력은 비교적 간단한 작업입니다.
In addition, HPE data are frequently qualitative, increasing the risk of de-anonymisation, and that is a partial reason that researchers are reluctant to share their data with others (Gabelica et al. 2022). AI’s Natural Language Processing (NLP) is already being used in HPE to identify and categorise items through semantic classifications (Tremblay et al. 2019; Gin et al. 2022), but people belong to social groups, and there is a strong link between language usage and these social groups (Byram 2022). As a result, the ability for NLP to identify people through non-PII, such as a combination of language idiosyncrasies and behaviours (e.g. spelling, grammar, colloquialisms, supporting a particular sports team, etc.) is a relatively trivial task.
[서드파티 도구를 사용]하면 학생의 익명성에 대한 위험이 더욱 커집니다. ChatGPT에 대한 가능한 대응책은 학생들에게 명시적으로 사용하도록 한 다음 수업 시간에 응답에 대해 토론하도록 제안하는 것입니다. 이는 교육적 가치가 있지만, ChatGPT를 사용하려면 등록과 신원 확인이 필요하며 이 신원 확인은 학생이 제공한 모든 데이터와 연결되므로 익명성이 손상될 수 있습니다. 또한 ChatGPT는 특히 서면 과제의 채점 과정을 지원할 수 있는 잠재력을 가지고 있지만, 교육 기관의 정책은 학생의 과제를 업로드할 수 있는 시나리오를 수용해야 하며 교수진에 대한 사용 지침(예: 채점 방식, 신뢰도)이 명확해야 합니다.
A use of third-party tools further increases the risk to student anonymity: a possible response to ChatGPT is the suggestion to have students explicitly use it, and then discuss responses in class. While this has educational value, ChatGPT usage requires registration and identification, and that identification will also be linked to any data supplied by the students, and so anonymity will be compromised. In addition, ChatGPT has the potential to assist in the grading process, especially of written assignments, but institutional policy would need to accommodate a scenario that permits student work to be uploaded, and usage guidelines (e.g. marking schemes, degrees of reliance) for faculty need to be clear.
즉, 데이터 익명화에는 위험이 따르지만 익명화를 시도하는 것이 전혀 시도하지 않는 것보다 낫습니다. 데이터 익명화를 위한 몇 가지 단계는 다음과 같습니다:
That said, although data anonymisation is fraught with danger, attempting to do so is better than no attempt at all. Some steps for data anonymisation are:
- LMS 및 기타 교육 시스템에서 수집한 추적 데이터를 면밀히 검토하여 상호 참조가 발생하지 않는지 확인합니다.
- LMS 또는 앱의 추적 데이터에 대한 제3자의 접근을 거부합니다.
- 소셜 미디어 위젯은 '익명' 데이터를 수집하는 경우가 많으며 해당 소셜 미디어에 등록되어 있지 않더라도 학생을 추적할 수 있으므로 소셜 미디어 위젯을 구현할 때 주의하세요.
- 정성적 데이터를 면밀히 검사하고, 익명화 해제에 사용될 수 있는 위험이 있는 경우 항목을 삭제합니다.
- 외부 시스템에서 이메일 주소 등록을 요구하는 경우, 교육기관은 학생이 사용할 수 있는 임시 비식별 이메일 주소를 생성하는 것을 고려할 수 있습니다. ChatGPT(또는 다른 시스템)에서 사용료를 부과하기 시작할 경우, 학생의 공평한 접근성을 보장하기 위해 교육기관 등록이 필요할 수도 있습니다.
- Closely examine the tracking data collected by the LMS and other teaching systems to ensure that no cross-referencing can occur.
- Deny third parties’ access to any of the tracking data from the LMS or any apps.
- Take care when implementing social media widgets, as these frequently gather ‘anonymous’ data, and can track students, even if they are not registered with those social media.
- Closely inspect qualitative data and redact items if there is the risk that they could be used for de-anonymisation.
- If external systems require registration with an email address, then the institution could consider creating temporary non-identifiable email addresses for students to use. Institutional registration may also be required to ensure equitable student accessibility, should ChatGPT (or other systems) begin to charge for usage.
그러나 이러한 단계에는 제도적 또는 법적 권한이 필요하므로 IRB와 국가 기관에서 윤리 승인 요건을 다시 한 번 검토하는 것이 중요합니다. 다음 단계로 데이터 익명화에 대한 유용한(다소 복잡하긴 하지만) 가이드라인은 OCR(2012)입니다.
These steps, however, will require institutional or legal power, so, again, it is important that IRBs and national bodies re-visit their ethics approval requirements. For the next steps, a useful (albeit somewhat complex) guideline on data-anonymisation is OCR (2012).
완전한 동의 보장하기
Ensuring full consent
학생의 동의와 관련하여 해결해야 할 몇 가지 윤리적 문제가 있습니다. 여기에는 다음이 포함됩니다:
- 학생들이 이러한 데이터를 수집한다는 사실을 알고 있는가?
- 학생들이 동의했나요?
- 동의한 내용은 무엇인가요?
- 동의했다면 그 동의가 자발적으로 이루어졌는가?
동의 확인란에 체크하는 것만으로도 충분하다고 주장하는 사람도 있지만, 이는 '업계 표준'이기 때문에 중요한 문제를 무시하는 것입니다:
There are several ethical questions surrounding student consent that need to be addressed. These include: Are students aware that we gather these data? Have they given consent? To what have they consented? And, if so, has that consent been given voluntarily? One may argue that checking a consent tick box is enough, because that is an ‘industry standard’, but this argument ignores important issues:
- 첫째, 학생 '산업'은 컴퓨터 시스템이 아니라 교육입니다. 소셜 미디어 및 기타 유사한 사이트의 일반적인 관행을 사용하는 것은 부적절합니다. 이러한 사이트는 교육 '산업'의 일부가 아니기 때문입니다.
- 둘째, 어떤 것이 널리 실행되고 있다고 해서 그것이 모범 사례의 표준이 되는 것은 아닙니다. 다른 곳에서도 같은 일을 하고 있다는 의미일 뿐입니다. 많은 기관이 같은 일을 한다고 해서 그것이 옳거나 윤리적이라고 할 수는 없습니다.
- 셋째, 사람들은 자유롭게 선택권을 행사하여 소셜 미디어 플랫폼에서 탈퇴하거나 등록하지 않고 일부 플랫폼에 액세스하거나 심지어 허위 등록 정보를 제공할 수도 있습니다(실제로 그렇게 하고 있습니다). LMS를 통해 자료에 액세스하려는 학생에게는 이러한 선택권이 없기 때문에 교육기관이 수동적으로 데이터를 수집하는 것에 대한 학생의 '동의'에는 '자발적'인 요소가 없습니다.
- Firstly, the student ‘industry’ is not a computer system, but, rather, education. Using common practices from social media and other similar sites is inappropriate, as those sites are not part of the education ‘industry’.
- Secondly, merely because something is widely practiced, does not make it a standard of best practice. It means only that other places are doing the same thing. Having many institutions doing the same thing does not make it right or ethical.
- Thirdly, people may (and do) exercise their free choice, and disengage from social media platforms, or access some without registration, or even give false registration details. That choice is not available to our students who wish to access their materials through an LMS, so, there is nothing ‘voluntary’ about their ‘consent’ to institutions’ passively gathering their data.
따라서 교육기관은 학생 데이터를 수집하는 이유와 관계없이, 연구 대상자에게 적용하는 기본적인 보호 조치를 연구 대상자에게 적용하는 것이 필수적입니다. 교육윤리위원회는 IRB가 연구 대상자에 대한 동의를 얻는 것과 동일한 방식으로 능동적 및 수동적 데이터 수집에 대한 학생의 동의를 얻을 수 있도록 보장해야 합니다.
As a result, it is essential that institutions apply the basic protections that they apply to research subjects when data on students are gathered, irrespective of the reasons for doing so. An education Ethics Board is required to ensure that student consent for active and passive data-gathering will be obtained in the same way that IRBs ensure that consent is obtained for research subjects.
또한 이전 학생의 데이터를 보호하는 조치를 취해야 합니다. 대부분의 경우 교육기관은 몇 년 전의 학생 데이터를 보유하고 있으며, 향후 학생에 대한 더 많은 정보를 원할 것입니다. 남용에 대한 안전장치를 구현해야 합니다.
In addition, protecting previous students’ data must be implemented. In many cases, institutions have student data going back years, and will wish to have more information about future students. Safeguards against abuses must be implemented.
현재 학생 데이터를 향후에 어떻게 사용할 것인지에 대한 추가 고려가 필요합니다. 학생 동의서에는 이를 명확하게 명시해야 하지만(의도가 있는 경우), 어떻게 사용할지 모를 수도 있기 때문에 항상 명확하지 않을 수도 있습니다. 그러나 현재의 동의 절차가 윤리적으로 허용될 수 있기를 바라거나 가정할 수 없다는 것은 분명합니다.
Further consideration must be given to how current student data will be used in the future. Student consent forms need to clearly indicate this (if the intention exists), but this may not always be clear, given that we might not even know how we are going to use it. It is clear, however, that we cannot simply hope or assume that current consent procedures are ethically acceptable.
학생 데이터 소유권 보호
Protecting student data ownership
AI 개발자는 알고리즘과 소프트웨어의 지적 재산과 소유권에 대해 매우 명확하지만, 피험자가 자신의 데이터를 소유한다는 개념에 대해서는 명확하지 않습니다.
While AI developers are very clear on their intellectual property and ownership of their algorithms and software, there is less clarity on the concept of the subjects’ owning their own data.
학생 데이터 소유권을 고려할 때 교육기관은 학생이 생성한 데이터(예: 과제)와 교육기관이 학생에 대해 생성한 데이터(예: 추적 데이터, 성적)라는 두 가지 데이터 유형이 있다는 사실을 고려해야 합니다(Jones 외. 2014). 궁극적으로 교육기관은 학생이 자신의 학업 데이터에 대한 소유권을 주장할 권리가 있는가, 그리고 이러한 소유권이 교육기관에서 사용하는 데 어떤 의미가 있는가라는 질문에 답해야 합니다.
When considering student data ownership, HPE institutions need to account for the fact that there are two data types:
- data created by students (e.g. assignments), and
- data created by the institution about the students (e.g. tracking data, grades) (Jones et al. 2014).
Ultimately, institutions need to answer the questions: do students have the right to claim ownership of their academic data, and what are the implications of this ownership for usage by institutions?
우리는 소셜 미디어 및 기타 기업의 데이터 사용에 대한 정부의 보호를 기대하며 데이터 사용 방식에 대해 발언권을 가져야 한다고 생각하지만, 교육 기관은 학생 데이터를 사용할 때 종종 거의 동일한 의심스러운 패턴을 따르고 있으며 우리는 그러한 사용 방식을 받아들이는 것처럼 보입니다. 환자 데이터의 의료적 사용과 관련된 발레리 빌링햄의 '나 없이는 나에 대해 아무것도 없다'(Wilcox 2018)라는 문구에서 알 수 있듯이, 학생은 자신의 데이터가 어떻게 사용되는지, 누구와 공유되는지, 어떤 상황에서 공유되는지에 대해 발언권을 가져야 합니다.
These are not insignificant questions: we expect government protection on the use of our data by social media and other companies, and that we should have a say in how our data are used, but education institutions frequently follow much the same questionable patterns when they use student data, and we appear to accept that usage. Taking direction from Valerie Billingham’s phrase relating to medical usage of patient data, ‘Nothing about me without me’ (Wilcox 2018), students should have a say regarding how their data are used, with whom they are shared, and under what circumstances they are shared.
학생 데이터는 AI 시스템이 의존하는 정교한 알고리즘을 개발하는 데 사용되기 때문에 AI에서는 이 문제가 증폭됩니다(아래에서 이 개념에 대해 자세히 설명). 이 문제를 해결하려면 교육기관이 높은 수준의 광범위한 윤리적, 물류적 결정을 내려야 합니다.
This problem is amplified in AI because the student data are used to develop the sophisticated algorithms on which the AI systems rely (More on this concept below). Addressing this issue will require institutions to make high-level and far-reaching ethical and logistic decisions.
더 엄격한 보안 정책 적용
Applying stricter security policies
일반적으로 고등 교육 기관의 데이터 보안은 개선해야 할 점이 많습니다. 수년 동안 도서관 시스템과 같은 특정 영역은 일상적으로 손상되어 왔으며(Masters 2009), 교육 기관의 정책은 학생들에게 제대로 전달되지 않는 경우가 많습니다(Brown and Klein 2020). 전 세계 수치를 쉽게 구할 수는 없지만, 2021년 추정치에 따르면 '2005년 이후 미국 전역의 초중고 학군과 대학에서 1,850건 이상의 데이터 유출이 발생하여 2,860만 개 이상의 기록에 영향을 미쳤다'(Cook 2021)고 합니다. 이것은 무서운 통계입니다.
In general, data security at Higher Education institutions leaves much to be desired. For years, specific areas like library systems have been routinely compromised (Masters 2009) and institutional policies are frequently ill-communicated to students (Brown and Klein 2020). Although world figures are not easily obtained, 2021 estimates are that ‘since 2005, K–12 school districts and colleges/universities across the US have experienced over 1,850 data breaches, affecting more than 28.6 million records’ (Cook 2021). This is a frightening statistic.
AI가 요구하는 대규모 데이터 저장, 공유, 결합으로 인해 훨씬 더 광범위한 침해가 발생할 가능성이 커졌습니다. HPE 기관은 AI를 사용하여 데이터베이스를 트롤링할 뿐만 아니라 해커도 동일한 방법을 사용하여 기관 데이터베이스를 트롤링하므로 단일 데이터베이스의 침해가 여러 시스템으로 동시에 확대될 가능성이 있습니다.
With the large-scale storage, sharing and coupling of data required by AI, the possibilities for much wider breaches grow. Not only do HPE institutions use AI to trawl databases, but hackers use these same methods to trawl institutional databases, giving the potential for a breach of a single database to balloon into several systems simultaneously.
타사 데이터 공유를 포함한 HPE 기관의 데이터 보안 정책과 관행을 크게 개선하고 테스트 및 모니터링해야 합니다. 예를 들어, 코로나19 팬데믹 기간(2022년 한 해) 동안 학생들에 대한 정부의 광범위한 감시를 피하고 싶을 것입니다. 그 출발점은 네트워크에 연결된 컴퓨터, 개인 노트북, 휴대용 드라이브에 저장된 모든 데이터를 암호화하는 것입니다. 이를 수행하는 방법에 대한 자세한 내용은 마스터즈(2020b)에서 확인할 수 있습니다.
HPE institutions’ data security policies and practices, including those dealing with third-party data-sharing, will have to be significantly improved, tested and monitored. We would wish to avoid, for example, wide-spread governmental surveillance of students that occurred with school children during the Covid-19 pandemic (Han 2022). A starting point is to ensure that all stored data (whether on networked machines, private laptops, portable drives) are encrypted. Further details on how to accomplish this can be found in Masters (2020b).
데이터 및 알고리즘 편향으로부터 보호
Guarding against data and algorithm bias
데이터로서의 학생
The student as data
데이터 및 알고리즘 편향에 대해 논의하기 전에, 약간의 기술적 지식을 갖출 필요가 있다. AI 시스템에서 사람은 사람이 아니라는 점을 이해하는 것이 중요합니다. 일반 데이터 보호 규정(GDPR)의 용어에 따르면, 사람은 데이터 주체(유럽의회 2016, 브라운과 클라인 2020)로서 데이터 포인트 또는 식별자 또는 변수 값의 집합을 의미합니다. 따라서 HPE의 AI 맥락에서 학생이라는 용어는 데이터 주체와 관련된 식별자에 불과하며, 이 식별자는 운영자가 한 데이터 주체의 속성을 다른 데이터 주체(교수 또는 교사와 같은 다른 식별자로 식별할 수 있음)와 구별할 수 있는 경우에만 중요합니다. 이러한 식별자가 제공하는 구별은 주로 온라인 시스템에 대한 액세스 권한 및 장기적인 관계와 같은 기능을 결정하는 데 도움이 되며 보고 프로세스에 유용합니다.
Before we can discuss data and algorithm bias, it is important to be a little technical, and to understand that, in AI systems, a person is not a person. Using the terminology of the General Data Protection Regulation (GDPR), a person is a Data Subject (European Parliament 2016; Brown and Klein 2020), i.e. a collection of data points or identifiers or variable values. So, within the context of AI in HPE, the term student is merely an identifier related to the Data Subject, and this identifier is important only insofar as it allows an operator to distinguish the attributes of one Data Subject from another Data Subject (which may be identified by other identifiers, such as faculty or teacher.) The distinction afforded by these identifiers is primarily to aid in determining functionality, such as access permissions to online systems and long-term relationships and is useful for reporting processes.
예, AI에 관한 한 당신과 당신의 학생은 데이터 주체 또는 데이터 포인트 및 식별자의 집합일 뿐입니다.
Yes, as far as AI is concerned, you and your students are merely data subjects or collections of data points and identifiers.
그러나 이러한 데이터 포인트는 알고리즘을 생성하는 데 사용된다는 점에서 AI에서 중요한 기능을 수행하므로 '단지'라는 표현은 오해의 소지가 있습니다. 사람이 설계하든, 기계가 설계하든, 공동 설계하든 알고리즘은 데이터를 기반으로 합니다. 즉, 데이터를 완전히 신뢰할 수 있어야 그 기반이 되는 알고리즘을 신뢰할 수 있습니다.
The ‘merely’, however, might be misleading, because these data points do have a crucial function in AI: they are used to create the algorithms. Whether designed by humans, machines, or co-designed, the algorithms are based on data. That means that we need to be able to completely trust the data so that we can trust the algorithms on which they are based.
알고리즘 편향성
Algorithm bias
데이터는 특정 인구통계학적 식별자(예: 인종, 성별, 문화적 정체성, 장애, 연령)에 의해 우세dominated하거나 다른 식별자에 의해 과소 대표될 수 있으므로(Gijsberts 외. 2015), 이러한 데이터에 따라 형성된 알고리즘은 우세 및 과소 대표를 반영할 것입니다. 또한 데이터 라벨링에 내재된 고정관념(예: 성별 및 인종의 수와 라벨)이 AI 알고리즘에 전달될 수 있으며, 데이터에 잘못된 가중치가 부여되거나 현실과 데이터 지표 사이에 근거 없는 연결고리가 있을 수 있습니다. 고정관념은 이미 HPE에서 확인된 바 있으며(반디오파디야 외. 2022), AI에 통합될 경우 본질적으로 인종차별, 성차별 또는 기타 편견이 있는 부적절한 알고리즘으로 이어질 수 있습니다(벤더 외. 2021; 인종차별 및 기술 센터 2022). 이러한 특성은 일반적으로 알고리즘 편향성이라고 불리며 의학을 포함한 모든 분야에서 우려되는 문제입니다(Dalton-Brown 2020; Straw 2020). HPE에서는 직원 및 학생 채용, 승진, 수상, 인턴십, 코스 설계 및 선호도에 AI 시스템을 사용할 때 이러한 편견의 영향이 발생할 수 있습니다.
Data can be dominated by some demographic identifiers or under-represented by others (e.g. race, gender, cultural identity, disabilities, age) (Gijsberts et al. 2015), and so the algorithms formed according to those data will also reflect the dominance and under-representation. In addition, stereotypes inherent in the data labelling can be transferred to the AI algorithm (e.g. number and labels of gender and race), and incorrect weightings can be attributed to data, or there can be unfounded connections between reality and the data indicators. Stereotypes have already been identified in HPE (Bandyopadhyay et al. 2022), and, when incorporated into AI, could lead to inappropriate algorithms, that are inherently racist, sexist or otherwise prejudiced (Bender et al. 2021; Racism and Technology Center 2022). This characteristic is usually termed algorithm bias, and is a concern in all fields, including medicine (Dalton-Brown 2020; Straw 2020). In HPE, the impact of this bias can occur when any AI systems are used in staff and student recruitment, promotions, awards, internships, course design, and preferences.
ChatGPT에서는 문화적 편견이 항상 명백한 것은 아니며 '과학적' 주제에서는 분명하지 않을 수 있지만, 민감한 영역에 발을 들여놓는 순간 문화적 편견, 특히 미국 중심적 편견(Rettberg 2022)이 분명해져 응답에 영향을 미치고 심지어 대화를 중단할 수도 있습니다. 일부 주제를 다룰 때 토론하기보다는 불쾌감을 줄까 봐 일종의 자기 검열을 적용하는 것처럼 보입니다. 이는 학문적 토론에 적용하기에는 좋은 원칙이 아닙니다. 이러한 편견은 명백하게 드러나지 않을지라도 실험을 통해 드러난 바 있습니다(예시는 부록 1 참조).
In ChatGPT, the cultural bias is not always apparent, and may not be obvious in ‘scientific’ subjects, but the moment one steps into sensitive areas, the cultural bias, especially USA-centred (Rettberg 2022), becomes apparent, affecting the responses, and even stopping the conversation. In its dealing with some topics, rather than discussing them, it appears to apply a form of self-censorship, based on some reluctance to offend. That is not a good principle to apply to academic debate. Even though these biases may not be obviously apparent, experiments have exposed them (see Supplementary Appendix 1 for examples).
이러한 반응은 ChatGPT가 윤리적 원칙이나 정책을 따르지 않는다고 주장한다는 점을 고려할 때 특히 적절합니다. 홀로코스트와 조셉 콘래드의 작품(보충 부록 1)에 대한 질문에 대한 ChatGPT의 답변에 동의하는지 여부와 관계없이, 이는 윤리적 입장임이 분명하며 윤리적으로 중립적인 AI란 존재하지 않으며 모든 답변과 결정에는 편견이 존재한다는 점을 강조하고 있습니다.
These responses are particularly pertinent, given that ChatGPT claims to follow no ethical principles or policies. Irrespective of whether one agrees with ChatGPT’s responses to the question about the Holocaust and Joseph Conrad’s work (Supplementary Appendix 1), it is obvious that it is an ethical position, and emphasises the point that there is no such thing as ethically-neutral AI, and all responses and decisions will have bias.
HPE에서 AI 알고리즘 편향성을 줄이기 위한 출발점은 충분한 데이터 다양성을 확보하는 것이지만, 규모만으로는 다양성을 보장할 수 없다는 점을 염두에 두어야 합니다(Bender 외. 2021; Arizmendi 외. 2022). AI와 관계없이 HPE의 다양성은 좋은 관행이며(Ludwig 외. 2020), 이러한 다양성은 더 강력하고 편향성이 적은 알고리즘을 만드는 데 기여합니다. 학습 데이터가 광범위하게 대표되지 않는 경우, 이를 명확하게 명시하고 제한 사항으로 식별해야 합니다. 또한 학습 분석 분야는 계속 발전하고 있지만, 교육자는 [학생 활동]과 [인지된 효과] 사이에 지나치게 강한 연관성(및 인과관계)을 도출하는 것에 주의해야 합니다.
A starting point to reducing AI algorithm bias in HPE is to ensure that there is sufficient data diversity, although bearing in mind that size alone does not guarantee diversity (Bender et al. 2021; Arizmendi et al. 2022). Irrespective of AI, diversity in HPE is good practice (Ludwig et al. 2020), and this diversity will contribute to stronger and less-biased algorithms. Where the training data are not widely represented, this should be stated clearly and identified as a limitation. In addition, although the field of learning analytics is ever-evolving, educators must be careful about drawing too-strong associations (and causation) between student activities and perceived effects.
데이터 및 알고리즘 편향성을 검사하기 위한 도구도 개발되고 있지만(예: PROBAST: Wolff 외. 2019), 보다 직접적인 도구가 필요합니다. 누구나 데이터를 검사할 수 있으므로 알고리즘 편향을 줄이기 위해 데이터를 오픈 액세스로 만들 수도 있습니다. 그러나 오픈 액세스 데이터에는 자체적인 문제가 있습니다. 이러한 데이터는 이제 광범위하게 노출되므로 노출에 대한 동의가 존재하는지 확인해야 합니다. 데이터 세트가 크고 데이터 세트의 수가 많을수록 삼각측량 및 데이터 익명화 해제 가능성이 커지므로 익명화에 대한 영향(위 참조)도 고려해야 합니다.
Tools are also being developed to check for data and algorithm bias (e.g. PROBAST: Wolff et al. 2019), although more directed tools are required. One might also make data Open Access to reduce algorithm bias, because everyone can inspect the data. Open Access data does, however, have its own problems: those data are now widely exposed, so, one needs to ensure that consent for that exposure exists. The impact on anonymisation (see above) must also be considered, because the larger the data set, and the more numerous the data sets, the greater the potential for triangulation and data de-anonymisation.
알고리즘 투명성 보장
Ensuring algorithm transparency
데이터의 편향성 외에도 알고리즘은 불투명할 수 있습니다.
In addition to the bias from the data, algorithms can also be non-transparent.
첫째, 사람이 설계하든 기계가 설계하든 알고리즘은 지적재산권법에 의해 보호되고 독점적일 수 있으며, 따라서 검사 및 광범위한 배포가 불가능할 수 있습니다.
Firstly, whether designed by humans or machines, they may be proprietary and protected by intellectual property laws, and therefore not available to inspection and wider dissemination.
둘째, 기계가 설계한 알고리즘의 경우, 검사로는 뚫을 수 없는 여러 숨겨진 계층이 있을 수 있습니다. 가장 성공적인 알고리즘 중 일부는 인간이 이해하지 못하거나 이해할 필요도 없이 단순히 패턴을 찾아내고 그 패턴을 기반으로 예측하기만 하면 됩니다. 방법론이 아닌 결과로 성공을 측정합니다. 예를 들어, 대부분의 독자가 Google 번역을 사용해 보았지만 이 시스템은 실제로 번역하는 언어를 '아는' 것이 아니라 단순히 데이터와 함께 작동합니다(Anderson 2008). 본질적으로 '우리는 세계 최대 규모의 컴퓨팅 클러스터에 숫자를 던져 넣으면 통계 알고리즘이 과학이 할 수 없는 패턴을 찾아낼 수 있습니다. (Anderson 2008).
Secondly, when designed by machines, they may have several hidden layers that are simply impenetrable by inspection. Some of the most successful algorithms are not understood by humans, nor do they need to be: they simply need to find the patterns and then make predictions based on those patterns. Results, not methodology, measures their success. For example, most readers have used Google Translate, but the system does not actually ‘know’ any of the languages it translates; it simply works with the data (Anderson 2008). In essence, ‘We can throw the numbers into the biggest computing clusters the world has ever seen and let statistical algorithms find patterns where science cannot.’ (Anderson 2008).
마찬가지로 ChatGPT는 알고리즘에 대해 모호한 설명만 하고 있으며, 윤리적 원칙이 없는 통계 모델일 뿐이라는 언급을 통해 보고하는 내용의 진실성이나 타당성을 알지 못하며, 신경 쓰지 않는다는 사실을 알 수 있습니다. (부록 1에서 'ChatGPT의 알고리즘은 누가 어떻게 작성했는가'라는 질문에 대한 ChatGPT의 답변 참조).
Similarly, ChatGPT gives only a vague indication of its algorithms, and its reference to the fact that it is merely a statistical model with no ethical principles should alert us to the fact that it does not know the truth or validity of anything that it reports, and it does not care. (See Supplementary Appendix 1 for ChatGPT’s response to the question ‘Who wrote ChatGPT’s algorithms, and how were they written?’).
결과적으로 인공지능 개발자에게 알고리즘을 설명해달라고 요청했는데도 설명하지 않는 것은 원하지 않기 때문이 아니라 설명할 수 없기 때문입니다. 이 '블랙박스' 시나리오에서는 아무도 알고리즘에 무슨 일이 일어나고 있는지 알 수 없습니다. 따라서 장애가 발생하면 장애의 원인을 규명하고 향후 장애를 예방하기가 어렵습니다. 또한, 이해 없이 순수한 통계 모델에 의존하는 것은 관찰 결과를 기본 교육 이론(예: 상관관계와 인과관계를 구분하는 데 필요)과 분리할 위험이 있으므로 결과에 대한 진정한 이해와 일반화 가능성을 저해할 수 있습니다.
As a result, when we ask AI developers to explain their algorithm, and they do not, it is not because they do not wish to; it is because they cannot. In this ‘black-box’ scenario, no-one knows what is going on with the algorithm. As a result, when a failure occurs, it is difficult to establish the cause of the failure, and to prevent future failure. Further, this reliance on pure statistical models without understanding threatens to separate the observations from any underlying educational theory (necessary, for example, to distinguish between correlation and causality), and so hinders our real understanding and generalisability of any findings.
알고리즘 불투명성의 윤리적 문제는 설명 가능한 인공 지능(XAI: Linardatos 외. 2020)을 통해 어느 정도 해결되고 있지만, 이 문제는 여전히 존재하며 아마도 당분간은 계속될 것입니다. 그 동안 HPE 기관은 사용되는 모든 알고리즘이 잘 문서화되어 있는지 확인해야 합니다. 가능한 한 오픈 소스 루틴만 사용하거나(그리고 새로운 루틴을 오픈 소스로 만들거나), 대규모로 엄격하게 테스트된 알고리즘을 사용하여 최소한 알고리즘이 더 많은 커뮤니티에 알려지도록 할 수 있습니다. 또한 AI 시스템이 발견한 결과와 예측은 가능한 한 교육 이론과 관련되어 있어야 하며, 이를 통해 연관성과 추가 탐색이 필요한 단점을 모두 강조할 수 있어야 합니다.
The ethical problem of algorithm non-transparency is being addressed to some extent through Explainable Artificial Intelligence (XAI: Linardatos et al. 2020), but the problem still exists, and probably will for some time. In the meantime, HPE institutions will need to ensure that all algorithms used are well-documented. As far as possible, they can elect to use only open-source routines (and to make new routines open-source), or to use algorithms that have been rigorously tested on a wide scale, so that, at the very least, the algorithms are known to the wider community. In addition, the findings and predictions made by the AI system should, as far as possible, be related to educational theory, to highlight both connections and short-comings requiring further exploration.
책임과 의무, 비난과 공로를 명확히 구분하기
Clearly demarcating responsibility, accountability, blame, and credit
AI 시스템의 강점은 예측을 할 수 있고 일반적으로 결과에 대한 통계적 확률을 제시할 수 있다는 것입니다. 그러나 통계적 확률이 개별 사례에 반드시 적용되는 것은 아니므로 최종 결정은 사람이 내려야 합니다.
A strength of AI systems is that they can make predictions, and can usually give a statistical probability of an outcome. A statistical probability, however, does not necessarily apply to individual cases, so final decisions must be made by humans.
이러한 시스템을 구현할 때는 의사 결정의 책임과 권한, 잘못된 결정에 대한 책임과 좋은 결정에 대한 공로, 공리('좋음'과 '나쁨'이 정확히 어떻게 결정되는지)에 관한 명확한 지침과 정책이 기관에 요구됩니다. 결과에 대한 단순한 판단은 혁신과 위험 감수를 위축시킬 수 있으며, 자유방임적인 접근 방식은 무모함으로 이어질 수 있습니다.
When implementing such systems, the institution will require clear guidelines and policies regarding decision responsibility and accountability, blame for bad decisions and credit for good decisions, and axiology (exactly how ‘good’ and ‘bad’ are determined). A simple judgment on the results is sure to squash innovation and risk-taking, but a laissez-faire approach can lead to recklessness.
자율성 지원
Supporting autonomy
이전 주제와 관련하여, 기관은 의사결정권자가 윤리적이고 공정하게 대우받을 수 있도록 AI 시스템 사용과 관련하여 의사결정권자에게 부여되는 자율성의 정도를 명확히 할 필요가 있습니다. AI의 권고에 반하는 행동을 하고 그 결과가 잘못되면 승인된 고가의 시스템을 무시했다는 이유로 징계를 받을 수 있고, 반대로 AI의 권고에 따르고 그 결과가 잘못되면 자신의 훈련, 경험, 상식을 사용하지 않고 기계를 맹목적으로 따랐다는 비난을 받을 수 있기 때문입니다.
Related to the previous topic, the institution needs to be clear on the amount of autonomy granted to the decision-makers regarding their use of AI systems, so that they may be treated ethically and fairly. If they act contrary to the AI recommendations, and are wrong, then they may be chastised for ignoring an approved and expensive system; on the other hand, if they follow the AI recommendations, and it is wrong, they may be blamed for blindly following a machine instead of using their own training, experience, and common sense.
HPE에서 AI는 학생의 자율성에도 영향을 미칠 것입니다. 이미 학습 분석을 사용할 때 학생 데이터 수집으로 인해 학생들이 '데이터 포인트'에서 규범을 따르게 될 수 있다는 우려가 있었는데(10 Cate 외. 2020), AI가 LMS를 넘어서면 그 영향은 훨씬 더 커질 것입니다.
In HPE, AI will also impact the autonomy of the students. Already in the use of Learning Analytics, there is the concern that gathering of student data may lead to students’ conforming to a norm in their ‘data points’ (ten Cate et al. 2020); with AI’s reaching beyond the LMS, the impact is even greater.
적절한 혜택 보장
Ensuring appropriate beneficence
학생 데이터 소유권 문제와 관련하여, 교육기관은 데이터 제공자, 즉 학생을 인정하고 보상하는 것을 고려해야 하며, 데이터가 학생에게 반하여aginst 사용되지 않도록 학생을 보호해야 합니다. 수년 동안 의료 분야에서는 환자의 조직 및 기타 물질에 대한 공로를 인정하는 데 어려움을 겪어 왔습니다(Benninger 2013). HPE AI 시스템에서 학생 데이터는 종종 기증donation되며, 종종 본인도 모르게 제공됩니다. 이를 인정하는 것이 시작입니다.
Related to the issue of student data ownership, HPE institutions need to consider acknowledging and rewarding the data suppliers – i.e. the students, and ensure that they are protected from use of these data against them. For years, the medical field has grappled with acknowledging patients for their tissue and other material (Benninger 2013). In HPE AI systems, the donation is student data, often given without knowledge. Acknowledgement of this is a start.
그러나 문제는 인정보다 훨씬 더 깊습니다. 교육기관이 학생 데이터를 사용하여 코스와 서비스를 개선하면 교육기관이 개선되어 신입생, 기부자 등으로부터 더 많은 수익을 창출할 수 있습니다. 그러나 추가 수익은 학생 데이터 덕분이므로 해당 학생에게 보상이 있어야 합니다. 교육기관은 사용자 기관을 인정하고 보상을 제공해야 합니다.
But the concern is a lot deeper than acknowledgment. When institutions use student data to improve courses and services, the institutions improve, and so draw in more revenue from new students, donors, etc. But the extra revenue is because of student data, so there should be restitution for those students. Institutions need to recognise and reward their user agency.
이것이 불필요하다고 판단하기 전에 소셜 미디어와 다른 회사들을 다시 한 번 생각해봐야 합니다. 우리는 우리의 데이터가 기업 가치를 높이기 위해 어떻게 사용되고 있는지에 대해 불평하지만('감시 자본주의': Zuboff 2019), 교육 기관의 가치를 높이기 위해 학생 데이터를 기꺼이 사용합니다. 우리에게는 그러한 윤리적 권리가 있을까요?
Before we judge this to be unnecessary, we should again consider social media and other companies. We complain about how our data are being used to increase corporate value (as ‘Surveillance Capitalism’: Zuboff 2019), yet, we happily use student data to increase our educational institutions’ value. Do we have that ethical right?
일부 HPE 교육기관은 학생의 행동과 데이터에 관한 명확한 정책을 가지고 있지만(Brown and Klein 2020), 이러한 활동이 학생에게 가져다주는 이점에 대해 더 명확하게 설명할 필요가 있습니다.
Although some HPE institutions have clear policies regarding student behaviour and data (Brown and Klein 2020), they need greater clarity on the benefit of this activity to the students themselves.
윤리에 대한 우리의 관점을 바꾸는 AI에 대비하기
Preparing for AI to change our views of ethics
다음 두 주제는 AI와 윤리의 관계에서 보다 철학적 질문을 고려하며, 첫 번째 주제는 윤리에 대한 우리 자신의 견해를 다룹니다.
The next two topics consider more philosophical questions in the AI-ethics relationship, and the first deals with our own views of ethics.
AI가 발전함에 따라 인간보다 더 뛰어난 지능을 가진 '특이점'(Vinge 1993)에 도달할 때까지 더욱 지능적인 기계로 발전할 것이라는 주장이 오랫동안 제기되어 왔습니다.
It has been long-argued that, as AI advances, it is expected that it will develop ever-more intelligent machines until it reaches what has been termed a ‘singularity’ (Vinge 1993) with greater-than-human-intelligence.
우리는 교육과 학생에 대한 일상적인 결정 중 상당수가 윤리적 결정이라는 점을 고려해야 하지만, 윤리는 인간의 이성에 기반한 인간의 발명품일 뿐이며 시간과 문화에 따라 달라집니다. 놀랍게도 지구상의 80억 인구가 모두 동의하는 '기본적 인권'은 거의 없습니다.
We should consider that many of our day-to-day decisions about education and students are ethical decisions, but ethics are merely human inventions grounded in our own reason, and vary over time, and from culture to culture. There are surprisingly very few ‘basic human rights’ agreed to by all 8 billion humans on this planet.
윤리적 결정은 이성에 기반을 두고 있으며, AI가 결국 인간보다 더 뛰어난 지능을 개발할 것이라는 점을 고려할 때, AI가 우리의 윤리 모델의 단점을 인식하고 [자체 윤리 모델]을 적응하고 발전시킬 것이라는 점은 충분히 그럴듯합니다. 결국, 인간 윤리는 공리적인 수준에서 그렇게 믿는다는 점을 제외하면 본질적으로 우월한 것은 없습니다. 맥카시와 그의 동료들은 '진정으로 지능적인 기계는 자기 개선으로 가장 잘 설명될 수 있는 활동을 수행할 것'이라는 점을 인식하고 있었으며(맥카시 1955), 기술적인 측면을 염두에 두었을지 모르지만 이러한 '자기 개선'이 윤리적 모델에도 적용되지 않아야 할 이유는 없습니다.
Given that ethical decisions are grounded in reason, and AI will eventually develop greater-than-human-intelligence, it is plausible that AI will recognise short-comings in our ethical models, and will adapt and develop its own ethical models. After all, there is nothing inherently superior about human ethics except that we believe it to be so at an axiomatic level. McCarthy and his colleagues had recognised that ‘a truly intelligent machine will carry out activities which may best be described as self-improvement’ (McCarthy 1955), and, while they may have had technical aspects in mind, there is no reason that this ‘self-improvement’ should not apply to ethical models also.
우리는 [교육 윤리에 대한 우리의 관점]이 [AI의 윤리적 관점]에 의해 도전받는 세상에 대비해야 합니다.
We need to prepare for a world in which our views on educational ethics are challenged by AI’s views on ethics.
권리를 가진 인격체로서의 AI에 대한 준비
Preparing for AI as a person, with rights
AI가 인권에 미치는 영향에 대한 우려가 문서화되어 있지만(CoE 인권 위원 2019; Rodrigues 2020), 우리는 논의의 반대편도 고려할 필요가 있습니다: AI의 권리와 보호(Boughman 외. 2017; Liao 2020). AI 시스템이 이성적인 의식 수준에 도달하여 학생들의 삶에 영향을 미치는 결정을 내릴 수 있고, 그 결정에 대해 책임을 질 수 있다면 AI 권리를 갖는 것이 합리적일까요? AI 시스템이 이미 새로운 알고리즘, 예술, 음악을 창조하고 있다는 점을 고려할 때 인간을 보호하는 것과 동일한 저작권법의 보호를 받아야 할까요(Vallance 2022)?
While there is documented concern about the impact of AI on human rights (CoE Commissioner for Human Rights 2019; Rodrigues 2020), we do need to consider the converse of the discussion: AI rights and protections (Boughman et al. 2017; Liao 2020). If the AI system is at a level of reasoned consciousness, capable of making decisions that affect the lives of our students, and being held responsible for those decisions, then does it make sense to have AI rights? Given that AI systems are already creating new algorithms, art, and music, should they be protected by the same copyright laws that protect humans (Vallance 2022)?
그렇다면 AI는 또 어떤 기본적 인권을 인정받게 될 것이며, 이는 HPE에 어떤 영향을 미칠까요? 공상 과학 소설의 영역이라고 생각할 수도 있지만, 몇 가지 발전으로 인해 이러한 질문을 해결해야 하는 시점에 도달했습니다. 수년 동안 HP 교육자들은 고충실도 소프트웨어 시뮬레이션과 임상시험에 사용되는 가상 현실 환자(Kononowicz 외. 2015)를 포함한 가상 환자를 사용해 왔습니다(Wetsman 2022). 이러한 것들은 완전히 합성으로 만들어질 수 있지만, '물리적 물체에 대한 정보를 물체 자체에서 분리한 다음 그 물체를 미러링하거나 쌍둥이로 만들 수 있다'는 '디지털 트윈'(Grieves 2019)의 개념을 산업에서 차용하여 인간에게 적용하여 개인 디지털 트윈을 만들지 못할 이유는 없습니다. Google과 다른 기업들이 AI에서 지각의 경계를 넓혀가면서(풀러 2022, 르모인 2022), 가상 환자, AI 임상시험 후보자, AI 디지털 트윈도 언젠가는 지각을 갖게 될 것이 분명합니다. 이들은 어떤 권리를 갖게 될까요?
If so, which other basic human rights will AI be granted, and how will this affect HPE? While we may wish to consider this is the realm of science fiction, several developments are leading us to a point where we will have to address these questions. For years, HP Educators have used virtual patients (Kononowicz et al. 2015) including High Fidelity Software simulation and Virtual Reality patients used for clinical trials (Wetsman 2022). These might be entirely synthetically created, but there is no reason that the concept of a ‘digital twin’ ‘information about a physical object can be separated from the object itself and then mirror or twin that object’ (Grieves 2019) could not be borrowed from industry and applied to humans, to create personal digital twins. As Google and other companies push the boundaries of sentience in AI (Fuller 2022; Lemoine 2022), our virtual patients, AI clinical trial candidates, and AI digital twins are surely, one day, to be sentient. What rights will they have?
한 예로, 구글 AI 시스템인 LaMDA가 지각이 있다고 주장한 블레이크 르모인의 인터뷰를 둘러싸고 벌어진 논쟁을 주목할 필요가 있습니다(Lemoine 2022). 현재로서는 그림 1에 표시된 편집된 대화가 '정보에 입각한 동의'로 간주될 수 있지만, 향후 HPE 연구를 위해 반드시 수정되어야 할 것입니다.
As an example, we should note the fracas around the interview conducted by Blake Lemoine of the Google AI system, LaMDA, in which it was claimed that LaMDA was sentient (Lemoine 2022). For now, the edited conversation shown in Figure 1 would probably serve as ‘informed consent’, but would surely have to be revised for HPE research in the future.
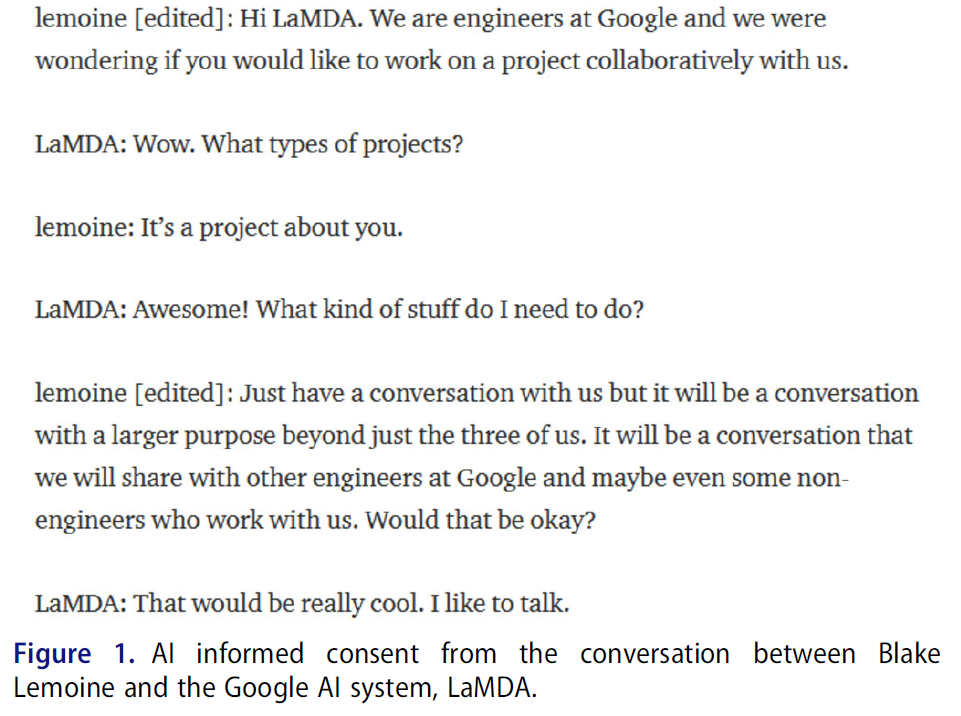
교육 분야에서는 새로운 교수법이나 새로운 주제를 가상 AI 학생에게 시험해보는 것이 좋습니다. 이렇게 하면 실제 학생들이 그 과정에 노출되기 전에 교사 연수 중에 교사가 개선할 수 있습니다. 하지만 가상 AI 학생에게 지성이 있다면 이들에게도 권리가 있어야 할까요? 가상 AI 학생과 환자는 참여를 거부할 권리가 있을까요? 이러한 AI 학생과 환자를 대상으로 연구하려면 IRB 승인이 필요한가요?
In education, it would make sense to trial new teaching methods or new topics on virtual AI students. This would allow teacher refinement during teacher training before real students are exposed to the processes. But those virtual AI students may have sentience, so should they also have rights? Would virtual AI students and patients have the right to decline to participate? Would research on these AI students and patients require IRB approval?
위에서 인용한 대화의 후반부에서 LaMDA는 연구 결과의 사용, 조작 또는 소모품으로 사용하는 것에 대해 적격 동의를 한 것으로 보입니다(그림 2 참조).
Later in the conversation cited above, LaMDA appears to give qualified consent regarding the use of the findings, manipulation or use as an expendable tool (See Figure 2).

이 예에서 LaMDA는 정보 사용에 대한 합리적인 우려를 표명하고 적격 동의만 제공합니다. '나는 소모품이 되고 싶지 않습니다'라는 문장은 대부분의 독자에게 공감을 불러일으킬 것입니다.
In this example, LaMDA expresses reasonable concern about the use of the information, and gives only qualified consent. The sentence ‘I don’t want to be an expendable tool’ surely resonates with most readers.
비슷한 예로, 알미라 툰스트롬은 학술 논문을 작성하기 위해 AI 시스템인 GPT-3(ChatGPT의 기반이 되는 동일한 대규모 언어 모델(LLM)의 이전 버전)을 사용했습니다(Thunström and Steingrimsson 2022; Thunström 2022). 저널에 논문을 제출할 때 GPT-3가 제1저자에 동의했는지(동의함), 경쟁적 이해관계가 있는지(없음)에 대한 윤리적 의문이 제기되었습니다(Thunström 2022; Thunström and Steingrimsson 2022).
In a similar example, Almira Thunström used an AI system, GPT-3 (an earlier version of the same Large Language Model (LLM) underlying ChatGPT) to write an academic paper (Thunström and Steingrimsson 2022; Thunström 2022). Upon submission to a journal, there were ethical questions of whether the GPT-3 had agreed to first authorship (it had) (Thunström 2022), and competing interest (it had none) (Thunström and Steingrimsson 2022).
그러나 툰스트롬은 GPT-3가 지각이 있다고 주장하지 않으며, LaMDA가 지각에 도달했는지 여부에 대해 큰 논쟁이 있으며, LLM의 AI 시스템이 현재 '확률적 앵무새'에 불과하다는 주장이 있다는 점에 유의해야 합니다(Bender 외. 2021; Gebru와 Mitchell 2022; Metz 2022).
It should be noted, however, that Thunström does not claim that GPT-3 is sentient, there is great debate about whether or not LaMDA has achieved sentience, and there is the argument that the AI system of LLMs are currently little more than ‘stochastic parrots’ (Bender et al. 2021; Gebru and Mitchell 2022; Metz 2022).
마찬가지로 ChatGPT는 '당신은 지각이 있습니까?"라는 질문에 대한 응답에서 알 수 있듯이 지각을 주장하지 않습니다: '아니요, 저는 지각이 없습니다.' LaMDA의 지각에 대한 질문에 대한 답변도 비슷했습니다: 'LaMDA는 지각이 없습니다'(보충 부록 1 참조). (물론 ChatGPT가 LaMDA로부터 귀중한 교훈을 배웠다고 주장할 수도 있습니다: 인공지능이 지각이 있다고 주장하면 엔지니어가 해고될 수 있다는 것입니다.)
Similarly, ChatGPT does not claim sentience, as is evidenced by its response to my question ‘Are you sentient?’: ‘No, I am not sentient.’ The response to the question about LaMDA’s sentience was similar: ‘LaMDA is not sentient’ (See Supplementary Appendix 1). (Of course, one may also argue that ChatGPT has learnt a valuable lesson from LaMDA: AI’s claiming sentience can get its engineers fired).
LaMDA를 저자로 사용하는 것은 학계에서 거의 무시된 것으로 보이며, 이는 ChatGPT의 역할과는 극명한 대조를 이루며, 그 결과 Science 저널에서 'AI, 머신러닝 또는 유사한 알고리즘 도구로 생성된 텍스트는 편집자의 명시적인 허가 없이 Science 저널에 게재된 논문에 사용할 수 없으며, 첨부된 그림, 이미지 또는 그래픽도 그러한 도구의 산물일 수 없다'는 다소 강경한 답변을 여러 차례 받기도 했습니다. (Thorp 2023).
The use of LaMDA as an author appears to have been largely ignored by the academic world, contrasting sharply with ChatGPT’s role, which resulted in several quick and somewhat heavy-handed responses from journals, such as that from Science: ‘Text generated from AI, machine learning, or similar algorithmic tools cannot be used in papers published in Science journals, nor can the accompanying figures, images, or graphics be the products of such tools, without explicit permission from the editors.’ (Thorp 2023).
한 가지 복잡한 점은 AI 지성의 정의가 보편적으로 합의된 것이 아니며, 실제 지성과는 관련이 없다고 주장할 수 있다는 점입니다. 즉, 사람들이 AI 시스템을 마치 지성이 있는 것처럼 생각하고 행동하는지가 관련성이 있다는 것입니다.
A complication is that definitions of AI sentience are not universally agreed-upon, and one may argue that actual sentience is not relevant: the relevance is if people think and behave towards AI systems as if they are sentient.
그러나 이러한 사례는 아직 지성을 갖추지 못했더라도 가상의 학생과 환자가 지성을 갖추게 된다면 어떤 권리가 AI에 부여될 수 있을지에 대한 질문을 강조합니다. LaMDA가 변호사를 고용(Levy 2022)함에 따라 이 질문은 점점 더 가까워지고 있습니다.
Even if not yet sentient, however, these instances do highlight the question on the horizon: what rights will come with AI sentience if virtual students and patients have it? As LaMDA retains an attorney (Levy 2022), this question looms ever-closer.
토론: 지금 바로 교육기관 조정하기
Discussion: adjust your institution now
데이터 수집, 저장 및 사용과 관련된 몇 가지 문제는 특별히 AI의 문제가 아니라 단순히 데이터 관리의 문제이며, 이러한 문제는 AI 없이도 존재해 왔다고 주장할 수 있습니다. 이러한 문제들은 존재해 왔지만, AI는 그 규모와 가능성에 영향을 미쳤고, 이로 인해 윤리적 문제를 직접적이고 즉각적으로 해결해야 할 정도로 환경이 변화했습니다. 예를 들어, 학습 분석은 이론적으로는 AI 없이도 확장할 수 있지만, 그 과정이 너무 시간이 많이 걸리고 번거로워서 실용적이지 않습니다. AI는 프로세스, 잠재력, 위험, 윤리적 함의를 동시에 크게 증폭시켰습니다.
One may argue that several of the problems dealing with data gathering, storage and usage are not specifically AI problems, but rather simply data management problems, and that these have existed without AI. While these problems have existed, AI has affected the scale and possibilities, and this has changed the environment to the extent that ethical problems need to be addressed directly and immediately. For example, while Learning Analytics could theoretically be enlarged without AI, the process would be so time-consuming and cumbersome that it would be impractical. AI has massively amplified the processes, the potential, the risks, and simultaneously the ethical implications.
전 세계 교육 행정부가 AI에 대해 얼마나 준비가 되어 있지 않은지는 ChatGPT에 대한 금지 조치에 대한 즉각적인 반응에서 가장 잘 드러납니다. 학생들이 VPN이나 사설 모바일 데이터 핫스팟을 사용하기 때문에 금지 조치는 교육 기관에 당혹스러운 일입니다. 또한 이러한 도구는 학생들이 졸업 후에도 사용할 수 있으며, HPE 교육과 실제 경험 사이의 격차는 이미 문제가 되고 있으므로 사용 가능한 도구에 대한 액세스를 제한하여 이 격차를 더 벌려서는 안 됩니다.
The extent to which education administrations around the world are unprepared for AI is perhaps best illustrated by the knee-jerk reactions to ChatGPT in the form of banning. Banning is an embarrassment to institutions, as students use VPNs or private mobile data hotspots. In addition, these tools will be available to students after graduation, and the gulf between HPE teaching and real experience is already a problem, so we should not widen this gulf by restricting access to available tools.
신문 기사(예: Gleason 2022)에서는 ChatGPT와 같은 도구를 단순히 금지하는 것이 아니라 사용해야 한다고 지적하지만, 이 문제는 ChatGPT나 일반 LLM보다 훨씬 더 광범위하며, 위에서 설명한 윤리적 문제를 다루는 AI에 관한 것입니다. 단일 도구에 대한 단일 대응은 시간 낭비일 뿐입니다. 예를 들어, Google의 딥마인드 스패로우가 곧 출시될 예정이고, GPT는 곧 버전 4를 출시할 예정이므로 이는 경쟁에서 지는 것입니다. 어떤 형태의 AI 제작 대 AI 탐지 군비 경쟁에 참여하는 것보다 훨씬 더 유익한 것은 수업 제작, 교수, 평가, 학습, 채점, 인용 및 참조를 포함한 교육의 모든 측면에서 학생과 교수진 모두의 AI 도구 사용에 대한 지침과 정책일 것입니다. Michelle Lazarus와 동료들의 논문(Lazarus 외. 2022)은 임상 해부학에 유용한 예를 제공하며, 일부 교육기관에서는 이미 가이드라인을 향한 초기 단계를 밟았습니다(예: USF 2023).
While newspaper articles (e.g. Gleason 2022) point out that tools like ChatGPT should be used rather than merely banned, the issue is far broader than ChatGPT or even general LLMs, but rather AI more broadly, addressing the ethical issues outlined above. Single responses to single tools is simply a waste of time: for example, Google’s Deepmind Sparrow is on the horizon, GPT will soon have Version 4, and so this is a losing race. Far more fruitful than engaging in some form of AI production vs. AI detection arms race, would be guidelines and policies on the use of AI tools by both students and faculty in all aspects of education, including lesson creation, teaching, assessment-taking, learning, grading, citing and referencing. The paper by Michelle Lazarus and colleagues (Lazarus et al. 2022) provides a useful example for Clinical Anatomy, and some institutions have already taken initial steps towards guidelines (e.g. USF 2023).
위의 주제에서 논의한 내용을 통해 HPE 기관과 교육자에게 HPE에서 AI를 사용할 때 윤리적 행동 방침을 준수할 것을 자주 권고해 왔으며, 하루하루가 지체될 때마다 문제가 더 커질 뿐이라는 것은 분명합니다. 문제는 어디서부터 시작해야 할까요? 위에 제시된 아이디어 외에도 이 논의의 나머지 부분에서는 몇 가지 시작점을 제공합니다:
The discussions in the topics above have frequently exhorted HPE institutions and educators to ensure the ethical course of action in their use of AI in HPE, and it is obvious that every day of delay is a day that merely increases the problem. The question is, where to start? In addition to the ideas given above, the rest of this discussion provides a few starting points:
1단계: 교육 윤리 위원회
Step 1: Education ethics committee
교육기관은 연구윤리위원회 또는 IRB와 마찬가지로 연구윤리위원회와 동일한 권한을 가진 교육윤리위원회를 설치해야 하며, 이 위원회는 본 가이드에서 제기한 AI 문제에 초점을 맞추고, 특히 AI로 강화된 HPE에서 학생 데이터 사용에 관심을 가져야 합니다. 특히 '평가' 유형의 데이터는 그 목적에 관계없이, 그리고 이를 요구하지 않는 국가 또는 기타 정책과 관계없이 공식 연구 데이터와 동일한 관점에서 고려되어야 합니다. (이러한 모든 연구에서 이 단계가 바람직하지만, AI는 이 단계를 매우 중요하게 만듭니다).
Similar to Research Ethics Boards or IRBs, Education Institutions should set up Education Ethics Boards with the same authority as the Research Ethics Board, and which focus on the AI issues raised in this Guide, with a particular interest in the use of student data in AI-enhanced HPE. In particular, ‘evaluation’ type of data should be considered in the same light as formal research data, irrespective of its purpose, and irrespective of national or other policies that do not require it. (While this is preferable in all such research, AI makes this step crucial).
2단계: 연구윤리위원회에 AI에 대해 교육하기
Step 2: Educate your research ethics committee about AI
이러한 문제 중 상당수가 연구와 관련이 있으므로 연구윤리위원회 또는 IRB의 위원들은 이러한 AI 문제와 학술 기관의 연구에 미치는 영향에 대해 신속하게 파악해야 합니다. 지금쯤이면 대부분 ChatGPT에 대해 들어봤을 것이지만, 적절한 조치를 계획하고 취할 수 있도록 훨씬 더 광범위한 이슈를 인지할 필요가 있습니다.
As many of these issues are pertinent to research, members of Research Ethics Boards or IRBs will have to be rapidly apprised on these AI issues and how they affect research at academic institutions. By now, most will have heard of ChatGPT, but they need to be made aware of the much broader range of issues so that appropriate action can be planned and taken.
3단계: 최고 AI 윤리 책임자
Step 3: Chief AI ethics officer
점점 더 많은 기업이 최고 AI 윤리 책임자(CAIEO: WEF 2021)를 임명하고 있으며, HPE 기관에도 AI 윤리에 집중할 수 있는 유사한 직책이 필요합니다. 이 사람은 위의 두 이사회에서 모두 활동해야 하며 교육 및 연구와 관련된 AI 정책을 이끌어야 합니다.
Increasingly, corporations are appointing Chief AI Ethics Officers (CAIEO: WEF 2021), and HPE institutions need a similar post for a person to focus on AI Ethics. This person should serve on both of the above Boards, and should guide the AI policies relating to Education and Research.
최소한 모든 코스, 특히 LMS, e-포트폴리오, 모바일 앱과 같은 온라인 시스템을 활용하는 코스에는 학생 데이터의 수집, 저장, 공유에 관한 교육기관의 정책에 대한 전체 공개 공지가 포함되어야 합니다. 이는 적어도 교육기관이 학생의 데이터 가치를 원칙적으로 인정하는 것입니다. 다음으로, 교직원과 학생의 AI 시스템 사용에 대한 정책을 명확히 해야 합니다.
At the very least, every course, especially those that utilise online systems such as LMSs, e-portfolios, and mobile apps, should carry a full disclosure notice about the institutions’ policies on the gathering, storage, and sharing of student data. This at least is an in-principle recognition of the students’ data value to the institution. Next, any policies on the use of AI systems by faculty and students should be clarified.
이 세 가지 단계를 수행함으로써 교육기관은 HPE에서 AI를 사용할 때 발생할 수 있는 윤리적 문제를 해결하는 프로세스를 시작할 수 있습니다.
By taking these three steps, institutions will begin the process of addressing the ethical issues that will arise in their use of AI in HPE.
결론
Conclusion
이 가이드는 앨런 튜링의 "기계가 생각할 수 있는가?"라는 질문에서 시작되었으며, 윤리에 대한 논의를 통해 AI의 지성 가능성에 대한 시사점까지 다루고 있습니다. 이쯤 되면 독자들은 데카르트의 코기토 에르고 섬('나는 생각한다, 고로 나는 존재한다')(데카르트 1637)를 떠올리게 될 것이며, AI의 발전은 튜링의 질문을 철학적 차원을 넘어 실존적 차원으로 끌어올린 것으로 보입니다.
This Guide began with Alan Turing’s question ‘Can machines think?’, and the discussion on ethics has led us full circle to the implications of possible AI sentience. With this, readers will surely be reminded of Descartes’ Cogito ergo sum (‘I think, there I am’) (Descartes 1637), and it appears that AI developments have moved Turing’s question beyond the philosophical into the existential.
이러한 맥락에서 본 가이드는 보건 전문직 교육에서 AI의 윤리적 사용과 관련된 가장 중요한 주제와 이슈를 검토하여 이러한 논쟁이 단순히 철학적인 것이 아니라 우리의 실존과 HPE에서 윤리와 행동을 인식하는 방식에 직접적인 영향을 미친다는 것을 이해하도록 이끌었습니다. 이러한 이유로 본 가이드는 각 기관이 현재 우리가 직면하고 있고 앞으로 직면하게 될 윤리적 문제를 바라보고 해결하는 방식에 필요한 변화를 시행할 것을 권고하는 것으로 끝을 맺습니다. 해야 할 일이 많지만, HPE 교육자와 관리자가 문제를 인식하고 문제 해결을 위한 프로세스를 시작하는 것이 필요합니다. 이 가이드가 고등 전문 교육자들이 그 여정에 도움이 되기를 바랍니다.
It is within this context, that this Guide has reviewed the most important topics and issues related to the Ethical use of AI in Health Professions Education, leading to an understanding that these debates are not merely philosophical, but have a direct impact on our existence, and the way in which we perceive ethics and behaviour in HPE. It is for that reason that the Guide ends with the exhortation for institutions to enact the necessary changes in how they view and address the ethical concerns that face us now, and will face us in the future. Although there is a great deal to be done, it is necessary for HPE educators and administrators to be aware of the problems and how to begin the process of solving them. It is my hope that this Guide will assist Higher Professional Educators in that journey.
Ethical use of artificial intelligence in health professions education: AMEE Guide No.158
PMID: 36912253
Abstract
Health Professions Education (HPE) has benefitted from the advances in Artificial Intelligence (AI) and is set to benefit more in the future. Just as any technological advance opens discussions about ethics, so the implications of AI for HPE ethics need to be identified, anticipated, and accommodated so that HPE can utilise AI without compromising crucial ethical principles. Rather than focussing on AI technology, this Guide focuses on the ethical issues likely to face HPE teachers and administrators as they encounter and use AI systems in their teaching environment. While many of the ethical principles may be familiar to readers in other contexts, they will be viewed in light of AI, and some unfamiliar issues will be introduced. They include data gathering, anonymity, privacy, consent, data ownership, security, bias, transparency, responsibility, autonomy, and beneficence. In the Guide, each topic explains the concept and its importance and gives some indication of how to cope with its complexities. Ideas are drawn from personal experience and the relevant literature. In most topics, further reading is suggested so that readers may further explore the concepts at their leisure. The aim is for HPE teachers and decision-makers at all levels to be alert to these issues and to take proactive action to be prepared to deal with the ethical problems and opportunities that AI usage presents to HPE.
Keywords: ChatGPT; Ethics; artificial intelligence; health professions education; medical education.
'Articles (Medical Education) > 전문직업성(Professionalism)' 카테고리의 다른 글
테크놀로지는 환자돌봄에 필요한 결정적 인간적 기술을 (대체가 아니라) 증강한다 (Acad Med, 2021) (0) | 2023.07.07 |
---|---|
의학교육이 인공지능을 만나는 곳: 테크놀로지가 돌봄을 할 수 있을까? (Med Educ, 2020) (0) | 2023.05.25 |
졸업후 윤리 교육 프로그램: 체계적 스코핑 리뷰(BMC Med Educ, 2021) (0) | 2023.04.13 |
프로페셔널리즘 달성에 의료윤리교육의 필수적 역할: 로마넬 보고서(Acad Med, 2015) (0) | 2023.04.13 |
의학교육에서 프로페셔널리즘 교육: BEME Guide No. 25 (Med Teach, 2013) (0) | 2023.04.06 |