질적연구를 위한 프레임워크분석방법을 사용: AMEE Guide No. 164 (Med Teach, 2023)
Using framework analysis methods for qualitative research: AMEE Guide No. 164
Sonja Klingberga , Renee E. Stalmeijerb and Lara Varpioc
소개
Introduction
보건 전문직 교육(HPE) 연구자가 선택할 수 있는 다양한 질적 연구 방법론과 방법이 있으며, 각 방법론은 고유한 목적에 맞게 맞춤화되어 있습니다.
- 직장 기반 학습에 관한 새로운 이론을 개발하는 데 관심이 있으신가요? 구성주의적 근거 이론(예: Helmich 외. 2018)을 찾아볼 수 있습니다.
- 의료 교육에서 수치심에 대한 생생한 경험을 탐구하는 데 관심이 있으신가요? 해석학적 현상학(예: Bynum 외. 2021)을 살펴볼 수 있습니다.
구성주의적 근거 이론과 해석학적 현상학을 포함한 대부분의 질적 연구 방법론은 현상을 깊이 이해하거나 설명하기 위해 해석의 힘을 활용합니다. 이러한 해석적(주요 용어에 대한 용어집은 표 1 참조) 질적 연구(Finlay 2021)는 종종 소규모 데이터 세트에 의존합니다. 소규모 데이터 세트는 연구 데이터 말뭉치를 반복적으로 읽고 다시 읽고, 연구팀과 함께 진화하는 이해에 대해 순환적인 방식으로 반복해서 논의하여 연구 인사이트를 개발하는 분석을 가능하게 합니다.
There are many different qualitative research methodologies and methods for health professions education (HPE) researchers to choose from, each tailored to unique purposes.
- Are you interested in developing a new theory about workplace-based learning? You might reach for constructivist grounded theory (e.g. Helmich et al. 2018). Are
- you interested in exploring the lived experience of shame in medical training? You might turn to hermeneutic phenomenology (e.g. Bynum et al. 2021).
Most qualitative research methodologies—including constructivist grounded theory and hermeneutic phenomenology—harness the power of interpretation to deeply understand or explain a phenomenon. Such interpretive (see Table 1 for a glossary of key terms) qualitative research (Finlay 2021) often relies on small datasets. Smaller data sets enable analyses that involve iteratively reading and re-reading the corpus of study data, discussing evolving understandings with the research team, again and again in a cyclical fashion, to develop research insights.
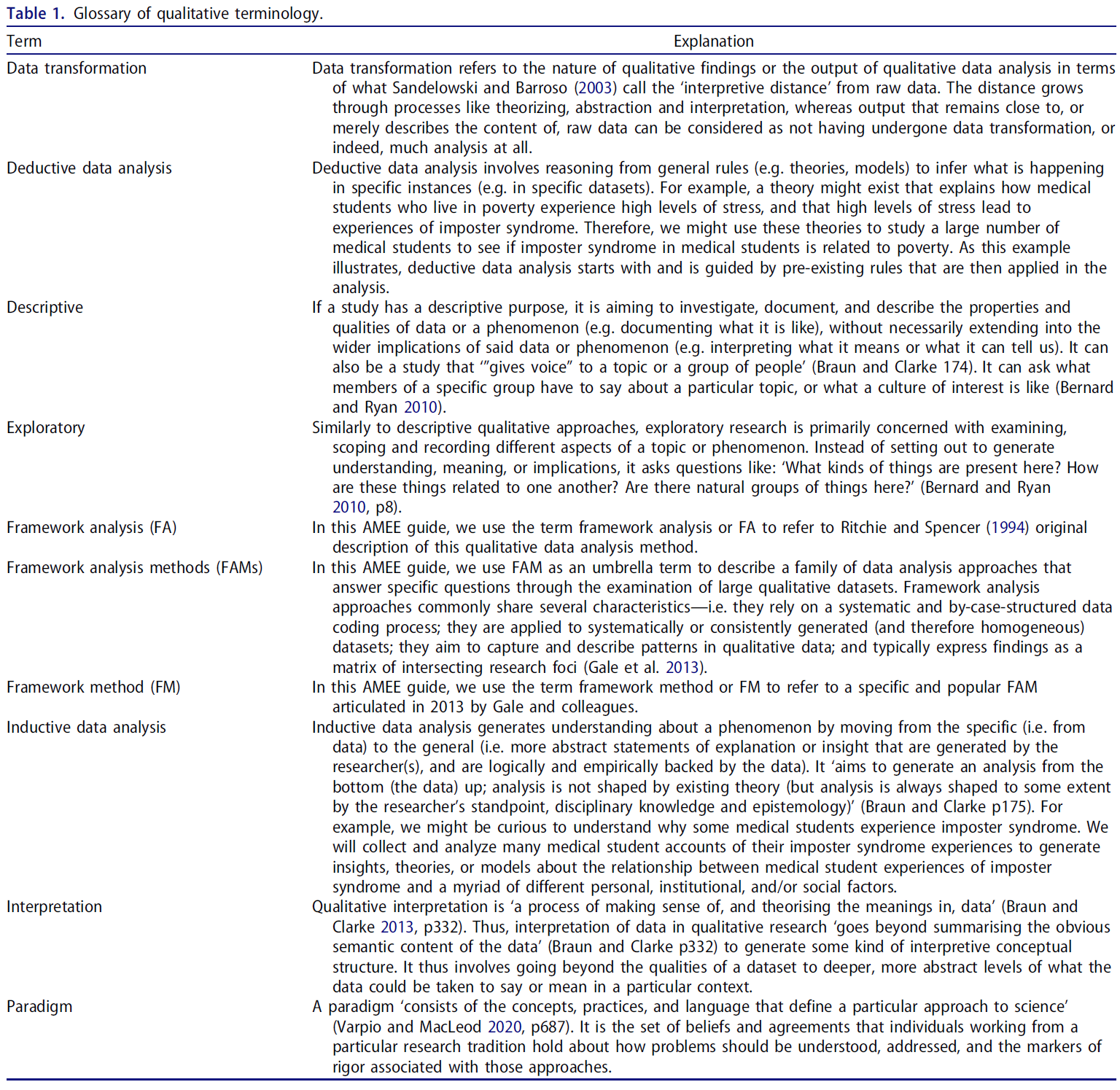
데이터 변환: 데이터 변환은 샌델로우스키와 바로소(2003)가 원시 데이터와의 '해석적 거리'라고 부르는 관점에서 질적 연구 결과 또는 질적 데이터 분석의 결과물의 성격을 말합니다. 이 거리는 이론화, 추상화, 해석과 같은 과정을 통해 커지는 반면, 원시 데이터에 가깝거나 단순히 원시 데이터의 내용을 설명하는 출력물은 데이터 변환을 거치지 않았거나 실제로 분석이 전혀 이루어지지 않은 것으로 간주할 수 있습니다.
Data transformation: Data transformation refers to the nature of qualitative findings or the output of qualitative data analysis in terms of what Sandelowski and Barroso (2003) call the ‘interpretive distance’ from raw data. The distance grows through processes like theorizing, abstraction and interpretation, whereas output that remains close to, or merely describes the content of, raw data can be considered as not having undergone data transformation, or indeed, much analysis at all.
연역적 데이터 분석: 연역적 데이터 분석은 일반적인 규칙(예: 이론, 모델)을 추론하여 특정 인스턴스(예: 특정 데이터 집합)에서 일어나는 일을 추론하는 것을 포함합니다. 예를 들어, 가난하게 사는 의대생이 높은 수준의 스트레스를 경험하고, 높은 수준의 스트레스가 사기꾼 증후군을 경험하게 된다는 이론이 존재할 수 있습니다. 따라서 이러한 이론을 사용하여 의대생의 사기꾼 증후군이 빈곤과 관련이 있는지 알아보기 위해 많은 수의 의대생을 연구할 수 있습니다. 이 예에서 알 수 있듯이, 연역적 데이터 분석은 기존의 규칙으로 시작하여 그 규칙에 따라 분석에 적용됩니다.
Deductive data analysis: Deductive data analysis involves reasoning from general rules (e.g. theories, models) to infer what is happening in specific instances (e.g. in specific datasets). For example, a theory might exist that explains how medical students who live in poverty experience high levels of stress, and that high levels of stress lead to experiences of imposter syndrome. Therefore, we might use these theories to study a large number of medical students to see if imposter syndrome in medical students is related to poverty. As this example illustrates, deductive data analysis starts with and is guided by pre-existing rules that are then applied in the analysis.
설명적: 설명적 목적의 연구는 데이터 또는 현상의 속성과 특성을 조사, 문서화 및 기술하는 것을 목표로 하며(예: 어떤 것인지 문서화), 해당 데이터 또는 현상의 더 넓은 의미(예: 데이터의 의미 또는 우리에게 무엇을 알려줄 수 있는지 해석)로 확장할 필요는 없습니다. 또한 어떤 주제나 집단에 '목소리를 부여'하는 연구일 수도 있습니다(브라운과 클라크 174). 특정 그룹의 구성원이 특정 주제에 대해 어떤 의견을 가지고 있는지 또는 관심 있는 문화가 어떤지 물어볼 수 있습니다(Bernard and Ryan 2010).
Descriptive: If a study has a descriptive purpose, it is aiming to investigate, document, and describe the properties and qualities of data or a phenomenon (e.g. documenting what it is like), without necessarily extending into the wider implications of said data or phenomenon (e.g. interpreting what it means or what it can tell us). It can also be a study that ‘”gives voice” to a topic or a group of people’ (Braun and Clarke 174). It can ask what members of a specific group have to say about a particular topic, or what a culture of interest is like (Bernard and Ryan 2010).
탐색적: 설명적 질적 접근 방식과 마찬가지로, 탐색적 연구는 주로 주제나 현상의 다양한 측면을 조사하고, 범위를 정하고, 기록하는 데 중점을 둡니다. 이해, 의미 또는 시사점을 도출하기 위해 시작하는 대신 다음과 같은 질문을 던집니다: '여기에는 어떤 종류의 것들이 있는가? 이 사물들은 서로 어떻게 관련되어 있는가? 여기에 자연스러운 사물의 그룹이 있는가?'(Bernard and Ryan 2010, p8).
Exploratory: Similarly to descriptive qualitative approaches, exploratory research is primarily concerned with examining, scoping and recording different aspects of a topic or phenomenon. Instead of setting out to generate understanding, meaning, or implications, it asks questions like:
- ‘What kinds of things are present here?
- How are these things related to one another?
- Are there natural groups of things here?’ (Bernard and Ryan 2010, p8).
프레임워크 분석(FA): 이 AMEE 가이드에서는 프레임워크 분석 또는 FA라는 용어를 사용하여 이 질적 데이터 분석 방법에 대한 Ritchie와 Spencer(1994)의 원래 설명을 참조합니다.
Framework analysis (FA): In this AMEE guide, we use the term framework analysis or FA to refer to Ritchie and Spencer (1994) original description of this qualitative data analysis method.
프레임워크 분석 방법(FAM): 이 AMEE 가이드에서는 대규모 정성적 데이터 집합을 조사하여 특정 질문에 답하는 데이터 분석 접근법의 제품군을 설명하기 위해 포괄적인 용어로 FAM을 사용합니다. 프레임워크 분석 접근법은 몇 가지 특징을 일반적으로 공유합니다(Gale et al. 2013).
- 체계적이고 사례별로 구조화된 데이터 코딩 프로세스에 의존하고,
- 체계적으로 또는 일관되게 생성된(따라서 동질적인) 데이터 세트에 적용되며,
- 질적 데이터의 패턴을 포착하고 설명하는 것을 목표로 하고,
- 일반적으로 교차하는 연구 초점의 매트릭스로 결과를 표현한다
Framework analysis methods (FAMs): In this AMEE guide, we use FAM as an umbrella term to describe a family of data analysis approaches that answer specific questions through the examination of large qualitative datasets. Framework analysis approaches commonly share several characteristics—i.e.
- they rely on a systematic and by-case-structured data coding process;
- they are applied to systematically or consistently generated (and therefore homogeneous) datasets;
- they aim to capture and describe patterns in qualitative data; and
- typically express findings as a matrix of intersecting research foci (Gale et al. 2013).
프레임워크 방법(FM): 이 AMEE 가이드에서는 프레임워크 방법 또는 FM이라는 용어를 사용하여 Gale과 동료들이 2013년에 설명한 구체적이고 널리 알려진 FAM을 지칭합니다.
Framework method (FM): In this AMEE guide, we use the term framework method or FM to refer to a specific and popular FAM articulated in 2013 by Gale and colleagues.
귀납적 데이터 분석: 귀납적 데이터 분석은 구체적인 것(즉, 데이터로부터)에서 일반적인 것(즉, 연구자가 생성하고 데이터에 의해 논리적이고 경험적으로 뒷받침되는 설명이나 통찰에 대한 보다 추상적인 진술)으로 이동하여 현상에 대한 이해를 생성합니다. '아래로부터(데이터) 위로 분석을 생성하는 것을 목표로 하며, 분석은 기존 이론에 의해 형성되지 않습니다(그러나 분석은 항상 연구자의 관점, 학문적 지식 및 인식론에 의해 어느 정도 형성됨)'(브라운과 클라크 p175). 예를 들어, 우리는 왜 일부 의대생들이 사기꾼 증후군을 경험하는지 이해하고 싶을 수 있습니다. 우리는 의대생의 사기 증후군 경험에 대한 많은 의대생의 설명을 수집하고 분석하여 의대생의 사기 증후군 경험과 무수히 많은 개인적, 제도적, 사회적 요인 사이의 관계에 대한 통찰력, 이론 또는 모델을 생성할 것입니다.
Inductive data analysis: Inductive data analysis generates understanding about a phenomenon by moving from the specific (i.e. from data) to the general (i.e. more abstract statements of explanation or insight that are generated by the researcher(s), and are logically and empirically backed by the data). It ‘aims to generate an analysis from the bottom (the data) up; analysis is not shaped by existing theory (but analysis is always shaped to some extent by the researcher’s standpoint, disciplinary knowledge and epistemology)’ (Braun and Clarke p175). For example, we might be curious to understand why some medical students experience imposter syndrome. We will collect and analyze many medical student accounts of their imposter syndrome experiences to generate insights, theories, or models about the relationship between medical student experiences of imposter syndrome and a myriad of different personal, institutional, and/or social factors.
해석: 질적 해석은 '데이터의 의미를 이해하고 그 의미를 이론화하는 과정'입니다(Braun and Clarke 2013, p332). 따라서 질적 연구에서 데이터 해석은 '데이터의 명백한 의미적 내용을 요약하는 것을 넘어'(브라운과 클라크 p332) 일종의 해석적 개념 구조를 생성하는 것입니다. 따라서 데이터 집합의 품질을 넘어 특정 맥락에서 데이터가 말하거나 의미할 수 있는 더 깊고 추상적인 수준으로 나아가는 것이 포함됩니다.
Interpretation: Qualitative interpretation is ‘a process of making sense of, and theorising the meanings in, data’ (Braun and Clarke 2013, p332). Thus, interpretation of data in qualitative research ‘goes beyond summarising the obvious semantic content of the data’ (Braun and Clarke p332) to generate some kind of interpretive conceptual structure. It thus involves going beyond the qualities of a dataset to deeper, more abstract levels of what the data could be taken to say or mean in a particular context.
패러다임: 패러다임은 '과학에 대한 특정 접근 방식을 정의하는 개념, 관행 및 언어로 구성'됩니다(Varpio와 MacLeod 2020, p687). 특정 연구 전통에서 일하는 개인이 문제를 어떻게 이해하고 해결해야 하는지, 그리고 그러한 접근 방식과 관련된 엄격함의 지표에 대해 가지고 있는 신념과 합의의 집합입니다.
Paradigm: A paradigm ‘consists of the concepts, practices, and language that define a particular approach to science’ (Varpio and MacLeod 2020, p687). It is the set of beliefs and agreements that individuals working from a particular research tradition hold about how problems should be understood, addressed, and the markers of rigor associated with those approaches.
그러나 때로는 질적 연구에 참여하는 이유가 더 지시적directed 때도 있습니다. 사전에 결정된 특정 정보 요구를 충족하기 위한 연구인 경우, 데이터 수집 및 분석의 범위가 좁아질 수 있습니다. 이러한 상황에서는 기존의 이론, 모델 또는 문헌의 증거가 데이터 수집과 분석 방법의 선택을 지시할 수 있습니다. 예를 들어, 실행 및 프로세스 평가 연구에서 이러한 경우가 많습니다(Klingberg 외. 2021; Draper 외. 2022). 이러한 지시적 목표directed goals와 관련된 질적 연구에서 연구자는 종종 대규모 데이터 세트를 수집하고 보다 집중적이고 구조화된 데이터 분석에 참여합니다(Davidson et al. 2019). 이러한 종류의 연구에 참여하는 HPE 연구자는 프레임워크 분석 방법(FAM)을 유용하게 사용할 수 있습니다. FAM은 데이터로부터 추상적인 해석을 위해 귀납적으로 작업하는 대신 연역적 접근 방식을 사용하는 경우가 많으므로 미리 설정된 목적과 목표에서 시작합니다(Pope 외. 2000). 보다 구조화된 형태의 데이터 수집(예: 개방형 설문조사 질문 또는 구조화된 인터뷰)을 포함하며, 데이터 분석은 분석이 시작되기 전, 때로는 데이터 수집이 시작되기도 전에 설정된 이론, 모델, 문헌 조사 결과 및 목적에 따라 기초적으로 형성되고 방향이 정해집니다.
However, sometimes our reasons for engaging in qualitative research are more directed. Sometimes the study is aimed towards fulfilling specific pre-determined information needs; this focus narrows the scope of data collection and analysis. In these situations, pre-existing theories, models, or evidence from the literature can direct data collection and the choice of analysis methods. For example, this is frequently the case in implementation and process evaluation studies (Klingberg et al. 2021; Draper et al. 2022). In qualitative studies that involve such directed goals, researchers often collect large datasets and engage in more focused and structured data analysis (Davidson et al. 2019). HPE researchers engaging in this kind of research might usefully rely on a framework analysis method (FAM). Rather than working inductively from the data towards abstract interpretation, FAMs often employ a deductive approach, and so begin with pre-set aims and objectives (Pope et al. 2000). They involve more structured forms of data collection (e.g. open ended survey questions or structured interviews), and data analysis is foundationally shaped and directed by theories, models, literature findings, and/or purposes that are established before analysis—and sometimes even before data collection—begins.
FAM의 우산 아래에는 다른 방법과 차별화되는 유용성과 힘을 가진 많은 분석 방법이 있습니다. 이 가이드에서는 FAM과 정성 분석 방법 그룹에 속하는 다양한 방법에 대해 명확하게 설명하고자 합니다. 먼저 오늘날 존재하는 다양한 FAM의 개발을 촉진한 1994년 Ritchie와 Spender가 설명한 프레임워크 분석 접근법과 FAM을 정의하는 것으로 시작합니다. HPE 연구자가 자신의 연구 요구 사항에 가장 적합한 FAM 유형을 결정할 수 있도록 FAM 범주에 속하는 가장 일반적인 방법 몇 가지를 간략히 검토합니다. 그런 다음 일반적으로 사용되는 다른 질적 분석 방법과의 관계에 대해 설명하여 독자가 연구 목적에 맞는 FAM을 파악할 수 있도록 돕습니다. 다음으로, 일반적으로 널리 사용되는 FAM인 프레임워크 방법의 일부인 단계에 대해 설명합니다. FAM이 이미 HPE 연구에서 어떻게 유용하게 활용되었는지를 설명하기 위해 FAM이 어떻게 활용될 수 있는지를 보여주는 몇 가지 발표된 연구 사례를 제공합니다. 마지막으로 정성적 분석에 FAM을 사용할 때 피해야 할 잠재적 함정에 대해 경고하는 것으로 마무리합니다.
There are many analysis methods that can sit beneath the FAM umbrella—each with utility and power that differentiates it from other methods. In this guide, we aim to provide clarity around FAMs and the variety of methods that are part of this group of qualitative analysis methods. We begin by defining FAMs and the framework analysis approach described in 1994 by Ritchie and Spender which catalyzed the development of the many varieties of FAMs that exist today. We offer a short review of some of the most common methods under the FAM umbrella so that the HPE researcher can decide which type of FAM best suits their research needs. We then describe how FAMs sit in relation to other commonly used qualitative analysis methods to help readers know when FAMs might fit their research purposes. Next, we explain the phases that are typically part of a popular FAM, the framework method. To illustrate how FAMs have already been useful in HPE research, we offer some examples of published research that demonstrate how FAMs might be harnessed. We end by warning readers of potential pitfalls to avoid when using FAMs for qualitative analysis.
FAM이란 무엇인가요?
What are FAMs?
질적 연구의 맥락에서 FAM의 역사는 1980년대 후반 영국에서 수행된 대규모 정책 연구 중 제인 리치와 리즈 스펜서가 만든 프레임워크 분석(FA)으로 거슬러 올라갑니다(Ritchie and Spencer 1994; Gale 외. 2013). FA는 대규모 질적 데이터 세트를 사용하여 특정 질문에 답하고 실행 가능한 인사이트를 창출할 수 있는 질적 데이터 분석 접근 방식에 대한 응용 정책 연구자들의 필요성에서 탄생했습니다(Ritchie and Spencer 1994; Srivastava and Thomson 2009). FA가 시작된 이래로 다양한 변형과 파생물이 개발되었습니다. 이러한 변형과 파생은 원래의 FA와 마찬가지로 FAM의 범주에 속하며, 여러 특징을 공유합니다(Gale et al. 2013).
- 체계적이고 사례별로 구조화된 데이터 코딩 프로세스에 의존하고,
- 비교적 동질적인 데이터 세트(즉, 주제나 주요 문제를 어느 정도 일관되게 다루기 위해 수집된 데이터 세트)에 적용되며,
- 정성적 데이터의 패턴을 주제(예: 공통 경험 및 경험 간 변화)로 포착하고 설명하는 것을 목표로 하고,
- 일반적으로 사례와 교차하는 코드로 구성된 프레임워크 매트릭스를 생성한다는
글로벌 보건(Klingberg 외. 2022), 보건 서비스 연구(Heath 외. 2012), HPE(Kumar 외. 2011, Howman 외. 2016, Balmer 외. 2021) 등 다양한 분야의 학자들이 다양한 FAM의 힘을 활용하고 있습니다.
In the context of qualitative research, the history of FAMs trace back to framework analysis (FA) which was created by Jane Ritchie and Liz Spencer in the late 1980s during large-scale policy research carried out in the United Kingdom (Ritchie and Spencer 1994; Gale et al. 2013). FA was born out of applied policy researchers’ need for a qualitative data analysis approach that enabled answering specific questions and creating actionable insights using large qualitative datasets (Ritchie and Spencer 1994; Srivastava and Thomson 2009). Since the inception of FA, different variants and offshoots have been developed. These variants and offshoots, as well as the original FA, sit under the FAM umbrella and so share several characteristics:
- they rely on a systematic and by-case-structured data coding process;
- they are applied to relatively homogenous datasets (i.e. datasets collected to address topics or key issues somewhat consistently);
- they aim to capture and describe patterns in qualitative data as themes (e.g. common experiences and variation across experiences); and
- typically produce framework matrices consisting of codes that intersect with cases (Gale et al. 2013).
The power of various FAMs have been harnessed by scholars working in a wide range of fields, including global health (Klingberg et al. 2022), health services research (Heath et al. 2012), and HPE (Kumar et al. 2011; Howman et al. 2016; Balmer et al. 2021).
표 2에는 FAM 우산 아래에 있는 몇 가지 연구 접근법이 나열되어 있습니다. 이 표에서 알 수 있듯이 몇 가지 미묘한 차이점만 있을 뿐, 각 접근법에는 고유하지는 않더라도 특징적인 특징이 있습니다. 특정 연구의 정확한 목적에 따라 HPE 연구자는 정성적 데이터 분석에 가장 적합한 FAM을 선택할 수 있습니다.
In Table 2, we list some research approaches that sit beneath the FAM umbrella. As this table makes clear, only a few nuanced differences separate some of the approaches under the umbrella; nevertheless, each approach has certain characterizing, if not unique, features. Depending on the precise purpose of a particular study, HPE researchers can choose the FAM best suited for analyzing their qualitative data.
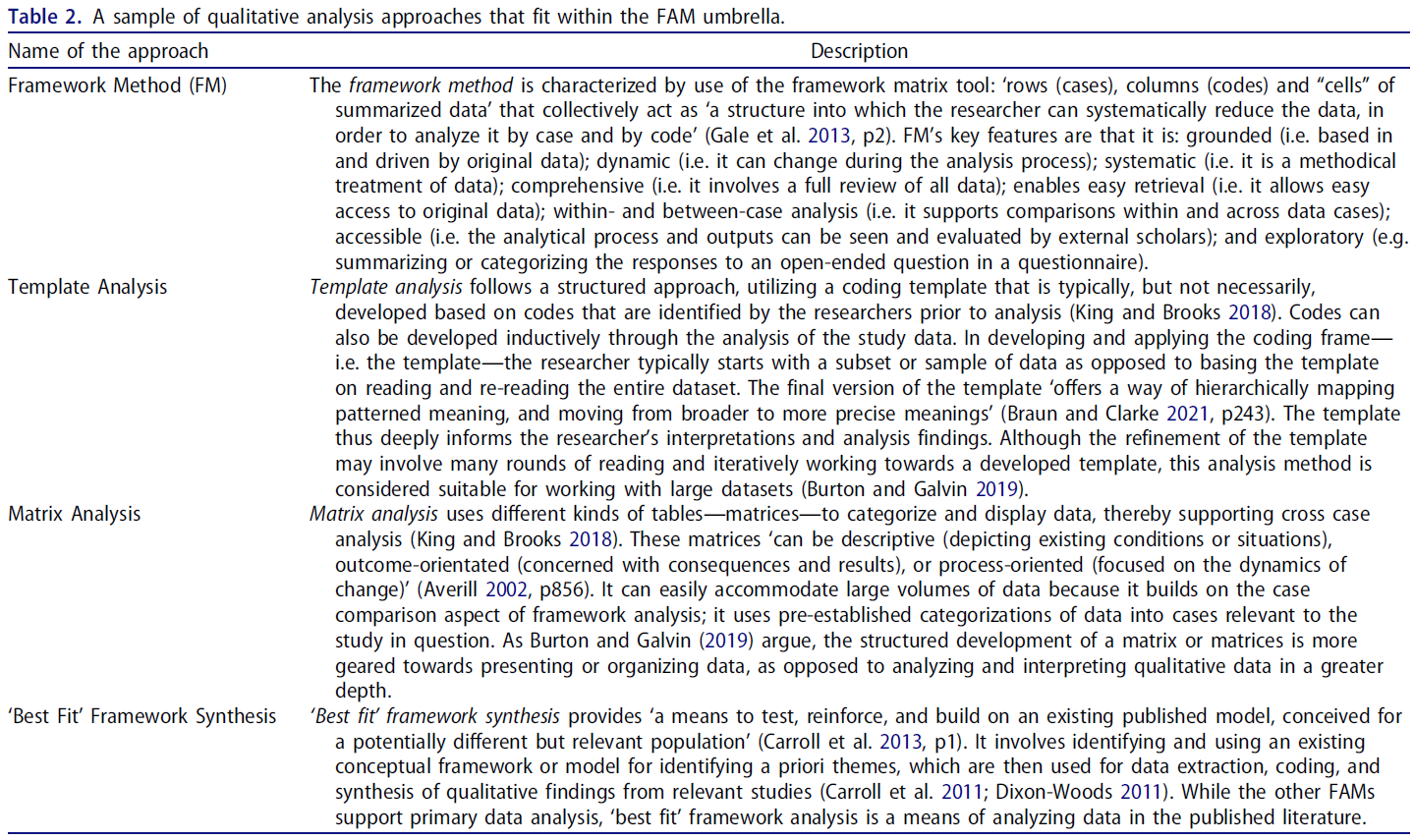
프레임워크 방식(FM)
Framework Method (FM)
프레임워크 방식은 '행(사례), 열(코드), 요약된 데이터의 '셀'로 구성된 프레임워크 매트릭스 도구를 사용하는 것이 특징이며, 이는 '연구자가 데이터를 체계적으로 축소하여 사례별, 코드별로 분석할 수 있는 구조'로 작용합니다(Gale 외. 2013, p2). FM의 주요 특징은 다음과 같습니다:
- 근거 기반(즉, 원본 데이터에 기반하고 이에 의해 구동됨);
- 동적(즉, 분석 프로세스 중에 변경될 수 있음);
- 체계적(즉, 데이터를 체계적으로 처리합니다);
- 포괄적(즉, 모든 데이터에 대한 전체 검토가 포함됨);
- 쉽게 검색할 수 있음(즉, 원본 데이터에 쉽게 액세스할 수 있음);
- 사례 내 및 사례 간 분석(즉, 데이터 사례 내 및 사례 간 비교를 지원합니다);
- 접근성(즉, 외부 학자가 분석 과정과 결과를 보고 평가할 수 있음); 그리고
- 탐색적(예: 설문지의 개방형 질문에 대한 응답을 요약하거나 분류).
The framework method is characterized by use of the framework matrix tool: ‘rows (cases), columns (codes) and “cells” of summarized data’ that collectively act as ‘a structure into which the researcher can systematically reduce the data, in order to analyze it by case and by code’ (Gale et al. 2013, p2). FM’s key features are that it is:
- grounded (i.e. based in and driven by original data);
- dynamic (i.e. it can change during the analysis process);
- systematic (i.e. it is a methodical treatment of data);
- comprehensive (i.e. it involves a full review of all data);
- enables easy retrieval (i.e. it allows easy access to original data);
- within- and between-case analysis (i.e. it supports comparisons within and across data cases);
- accessible (i.e. the analytical process and outputs can be seen and evaluated by external scholars); and
- exploratory (e.g. summarizing or categorizing the responses to an open-ended question in a questionnaire).
템플릿 분석
Template Analysis
템플릿 분석은 구조화된 접근 방식을 따르며, 일반적으로 연구자가 분석 전에 식별한 코드를 기반으로 개발되는 코딩 템플릿을 활용하지만 반드시 그렇지는 않습니다(King and Brooks 2018). 코딩은 연구 데이터의 분석을 통해 귀납적으로 개발할 수도 있습니다. 코딩 프레임, 즉 템플릿을 개발하고 적용할 때 연구자는 일반적으로 전체 데이터 집합을 읽고 다시 읽는 것을 기반으로 템플릿을 만드는 것이 아니라, 데이터의 하위 집합 또는 샘플로 시작합니다. 템플릿의 최종 버전은 '패턴화된 의미를 계층적으로 매핑하고 더 넓은 의미에서 더 정확한 의미로 이동할 수 있는 방법을 제공합니다'(Braun and Clarke 2021, 243쪽). 따라서 템플릿은 연구자의 해석과 분석 결과를 깊이 있게 알려줍니다. 템플릿을 다듬는 데는 여러 차례의 읽기와 개발 템플릿을 향한 반복적인 작업이 필요할 수 있지만, 이 분석 방법은 대규모 데이터 세트 작업에 적합한 것으로 간주됩니다(버튼과 갤빈 2019).
Template analysis follows a structured approach, utilizing a coding template that is typically, but not necessarily, developed based on codes that are identified by the researchers prior to analysis (King and Brooks 2018). Codes can also be developed inductively through the analysis of the study data. In developing and applying the coding frame— i.e. the template— the researcher typically starts with a subset or sample of data as opposed to basing the template on reading and re-reading the entire dataset. The final version of the template ‘offers a way of hierarchically mapping patterned meaning, and moving from broader to more precise meanings’ (Braun and Clarke 2021, p243). The template thus deeply informs the researcher’s interpretations and analysis findings. Although the refinement of the template may involve many rounds of reading and iteratively working towards a developed template, this analysis method is considered suitable for working with large datasets (Burton and Galvin 2019).
매트릭스 분석
Matrix Analysis
행렬 분석은 다양한 종류의 테이블, 즉 행렬을 사용하여 데이터를 분류하고 표시함으로써 사례 간 교차 분석을 지원합니다(King and Brooks 2018). 이러한 행렬은 '설명적(기존 조건 또는 상황 묘사), 결과 지향적(결과 및 결과 관련) 또는 과정 지향적(변화의 역학에 초점)'일 수 있습니다(Averill 2002, p856). 프레임워크 분석의 사례 비교 측면을 기반으로 하기 때문에 대량의 데이터를 쉽게 수용할 수 있으며, 해당 연구와 관련된 사례로 미리 설정된 데이터 분류를 사용합니다. Burton과 Galvin(2019)이 주장한 것처럼, 매트릭스 또는 행렬의 구조화된 개발은 질적 데이터를 심층적으로 분석하고 해석하는 것이 아니라, 데이터를 제시하거나 조직화하는 데 더 적합합니다.
Matrix analysis uses different kinds of tables—matrices—to categorize and display data, thereby supporting cross case analysis (King and Brooks 2018). These matrices ‘can be descriptive (depicting existing conditions or situations), outcome-orientated (concerned with consequences and results), or process-oriented (focused on the dynamics of change)’ (Averill 2002, p856). It can easily accommodate large volumes of data because it builds on the case comparison aspect of framework analysis; it uses pre-established categorizations of data into cases relevant to the study in question. As Burton and Galvin (2019) argue, the structured development of a matrix or matrices is more geared towards presenting or organizing data, as opposed to analyzing and interpreting qualitative data in a greater depth.
'최적 적합' 프레임워크 합성
‘Best Fit’ Framework Synthesis
'최적 적합' 프레임워크 합성은 '잠재적으로 다르지만 관련성이 있는 모집단을 위해 고안된 기존의 공개된 모델을 테스트, 강화 및 구축하는 수단'을 제공합니다(Carroll 외. 2013, p1). 여기에는 선험적 주제를 식별하기 위해 기존의 개념적 프레임워크 또는 모델을 식별하고 사용하는 것이 포함되며, 이는 관련 연구의 질적 연구 결과의 데이터 추출, 코딩 및 종합에 사용됩니다(Carroll 외. 2011; Dixon-Woods 2011). 다른 FAM은 1차 데이터 분석을 지원하지만, '최적 적합' 프레임워크 분석은 출판된 문헌의 데이터를 분석하는 수단입니다.
‘Best fit’ framework synthesis provides ‘a means to test, reinforce, and build on an existing published model, conceived for a potentially different but relevant population’ (Carroll et al. 2013, p1). It involves identifying and using an existing conceptual framework or model for identifying a priori themes, which are then used for data extraction, coding, and synthesis of qualitative findings from relevant studies (Carroll et al. 2011; Dixon-Woods 2011). While the other FAMs support primary data analysis, ‘best fit’ framework analysis is a means of analyzing data in the published literature.
독자들이 이러한 선택을 할 수 있도록 돕기 위해, 이제 FAM의 정의적 특징에 대해 좀 더 자세히 설명하겠습니다. 다른 정성적 분석 방법과 관련하여 FAM을 고려함으로써 FAM의 범주에 속하는 접근법을 하나로 묶는 일관성을 더 잘 이해할 수 있습니다.
To support readers in making these selections, we next go into more detail about the defining features of FAMs. By considering FAMs in relation to other qualitative analysis methods, we can further understand the coherence that unites approaches that fall underneath the FAM umbrella.
다른 정성적 분석 방법과 FAM 구별하기
Distinguishing FAMs from other qualitative analysis methods
정성적 분석 방법과 다른 정성적 분석 방법의 차이점을 이해하는 한 가지 방법은 분석 프로세스 중에 데이터가 변형되는 정도를 고려하는 것입니다. Sandelowski와 Barroso(2003)는 데이터 변환의 연속선상에서 질적 연구에 대한 다양한 접근 방식을 찾는 데 도움이 됩니다. 그림 1은
- 이 연속체의 한쪽 끝에서 원시 데이터에 가깝게 유지하고자 하는 연구가 어떻게 데이터를 최소한의 변형(예: 설문지의 개방형 질문에 대한 응답 요약)을 거치는지 보여줍니다.
- 이와는 대조적으로, 해석적 설명에 중점을 두는 연구에서는 데이터가 원본 데이터에서 많은 변형 과정을 거칩니다(예: 감정적 경험의 본질에 대한 현상학적 분석).
이러한 양극 사이에는 다양한 연구가 존재하며, 이는 분석이 데이터 내용과 특성에 대한 단순한 목록을 넘어 보다 추상적인 해석 및/또는 인사이트를 생성하는 데까지 나아갈 것으로 기대되는 정도를 반영합니다. 이 연속체는 다양한 정성적 접근 방식과 관련된 분석의 종류를 개념화하는 데 도움이 될 수 있습니다.
One way of understanding what differentiates one qualitative analysis method from another is to consider the extent to which the data is transformed during the analysis process. Sandelowski and Barroso (2003) helpfully located different approaches to qualitative research on a continuum of data transformation. Figure 1 demonstrates
- how, at one end of this continuum, research studies seek to stay close to raw data and so the data undergo minimal transformation (e.g. summarizing the responses to an open-ended question in a questionnaire). In contrast,
- at the other end of the spectrum, studies engage in interpretive explanation and so the data undergo many transformative moves away from the original data (e.g. a phenomenological analysis of the essence of an emotional experience).
There is a spectrum of research that exists between these poles, reflecting the degree to which analyses are expected to move beyond simple inventories of data content and characteristics, to generate more abstract interpretations and/or insights. This continuum can serve as a helpful conceptualization of the kind of analysis involved in different qualitative approaches.
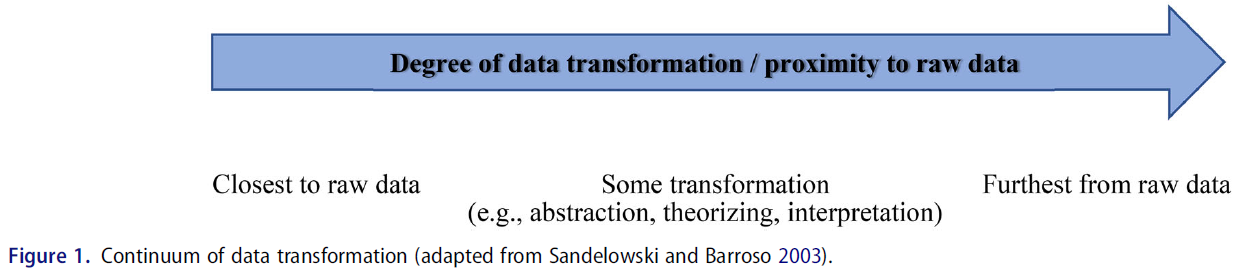
FAM은 일반적으로 연구자가 심층 데이터 변환에 참여하지 않는다는 점을 감안할 때, 일반적으로 Sandelowski와 Barroso의 연속체에서 원시 데이터에 가까운 쪽에서 작업하는 연구를 지원합니다. 따라서 FAM은 특정 상황에서 사용하기에 적합한 방법입니다. FAM을 사용하면 연구자가 데이터에 근접하여 설명, 범주 및/또는 유형을 제공하는 분류, 조직화 및 요약된 데이터의 고도로 구조화된 결과물을 생성할 수 있습니다. 모든 질적 연구가 사회 현상에 대한 새로운 이론이나 고도로 정교한 설명을 만들어내야 하는 것은 아닙니다. 따라서 설명적이고 실용 지향적인 연구를 촉진하기 위해 FAM과 같은 특정 방법의 가치를 인식하는 것이 도움이 됩니다. FAM의 설명적 또는 탐색적 경향은 다양한 참여자 그룹을 포함하고 서로 다른 그룹 간의 결과를 비교하는 것이 분석적 관련성이 있는 질적 데이터 세트를 분석하는 데 특히 유용합니다. 이는 다양한 연령대, 직업 또는 특정 현상에 대한 관계/경험(예: 만성 질환이 있는 의료 전문가와 없는 의료 전문가)과 같이 잠재적으로 관심 있는 자연스러운 하위 집합을 포함하는 대규모 데이터 세트의 경우에 해당할 수 있습니다.
Given that FAMs do not typically engage the researcher in deep data transformation, they generally support research working at the close to raw data end of Sandelowski and Barroso’s continuum. As such, FAMs are methods that lend themselves to be used in specific circumstances. FAMs enable the researcher to stay close to the data to generate highly structured outputs of categorized, organized, and summarized data offering descriptions, categories, and/or typologies. Not all qualitative research needs to produce new theories or highly sophisticated explanations of social phenomena. It is therefore helpful to recognize the value of specific methods—like FAMs—for facilitating descriptive and for-practical-use oriented research. The descriptive or exploratory tendencies of FAMs are also particularly useful for analyzing qualitative datasets that include distinct participant groups and where a comparison of findings between different groups is of analytical relevance. This may well be the case with larger datasets that potentially contain natural subsets of interest, such as different age groups, professions, or relationships with/experiences of a given phenomenon (e.g. health care professionals with and without chronic illness).
FAM과 달리 다른 질적 방법론과 데이터 분석 방법에는 상당한 변형이 수반됩니다. 데이터 변환의 연속선상에서 다양한 질적 연구 접근법이 어떻게 위치할 수 있는지를 고찰함으로써, 어떤 접근법은 데이터에 대한 심층적인 해석과 현상에 대한 설명을 수반하는 반면, 어떤 접근법은 해석을 거의 수반하지 않는다는 점을 강조합니다. 표 3은 프레임워크 방법, FAM을 포함한 네 가지 접근 방식을 이 연속체에 따라 정리하고 각 접근 방식이 일반적으로 사용되는 연구 목적의 측면에서 비교를 제공합니다.
In contrast to FAMs, other qualitative methodologies and data analysis methods involve considerable transformation. By reflecting on how different qualitative research approaches can be located along the continuum of data transformation, we highlight how some approaches involve deep interpretation of data and explanations of phenomena, while others engage in very little interpretation. Table 3 organizes four different approaches—including framework method, an FAM—along this continuum and offers comparisons in terms of the kind of research purposes each approach is typically used to address.
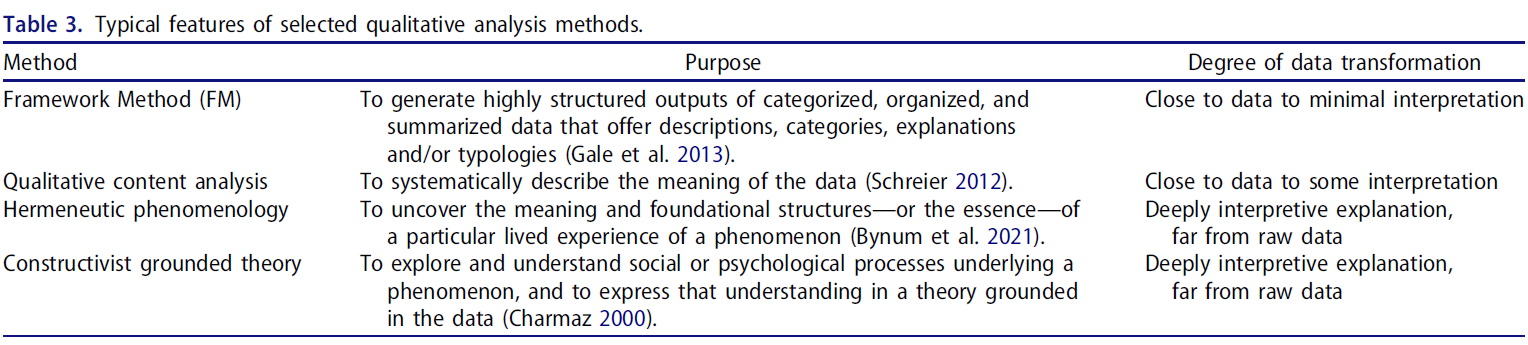
그렇다고 해서 FAM이 해석이나 데이터 변환이 필요 없다는 뜻은 아니며, 대신 발생하는 변환은 원시 데이터에 가깝게 유지됩니다. FAM의 힘 중 하나는 대량의 데이터를 의도적으로 구성된 사례(예: 분석 단위, 참가자 인터뷰)와 코드(예: '원시 데이터 발췌에 할당된 설명적 또는 개념적 레이블')로 축소하는 능력에 있습니다(Gale 외. 2013, 2페이지). 이러한 사례와 코드는 전체 데이터 세트에 적용되는 코딩 프레임을 구성하며, 코딩은 본질적으로 데이터 발췌에 적절한 코드로 라벨을 붙이는 과정입니다. 모든 데이터가 프레임워크에 코딩되었다고 해서 분석이 완료된 것은 아닙니다. 대신 연구자가 연구의 특정 목표로 돌아가 사례와 코드가 이러한 목표를 충족하는지 확인하고, 관심 있는 현상의 범위 또는 성격을 매핑하고, 유형학을 개발하고, 연관성 또는 설명을 식별해야 합니다(Ritchie와 Spencer 1994). 이 작업에는 분명히 데이터에 대한 해석이 포함되지만, 분석은 데이터에 가깝게 유지되며 일반적으로 추상화 수준이 제한적인 인사이트를 제공합니다.
This is not to suggest that FAMs are free of any interpretation or data transformation; instead, the transformation that takes place stays close to the raw data. Part of the power of FAMs rests in their ability to reduce large amounts of data into a purposefully constructed set of cases (i.e. the units of analysis; e.g. a participant interview) and codes (i.e. ‘a descriptive or conceptual label that is assigned to excerpts of raw data’ (Gale et al. 2013, p2). These cases and codes make up the coding frame which is applied to the entire dataset; this application is essentially a process of labelling data excerpts with the appropriate code. When all the data is coded into the framework, the analysis is not complete. Instead, as Ritchie and Spencer explain, this is when the researcher: returns to the study’s specific objectives and ensures that the cases and codes address these objectives; maps the range and/or nature of the phenomenon of interest; develops typologies; and identifies associations and/or explanations (Ritchie and Spencer 1994). While this work clearly involves interpretations of the data, the analysis stays close to the data and offers insights that are typically limited in their level of abstraction.
FAM을 사용하여 정성적 분석에 참여하는 방법
How to engage in qualitative analysis using a FAM
FAM을 사용하는 방법을 설명하기 위해, 우리는 이 우산 아래에서 프레임워크 방법(FM) 중 하나를 선택합니다. FM은 패턴 기반의 구조화된 기법을 사용하여 데이터를 사례와 코드가 교차하는 매트릭스로 구성하고, 결국 프레임워크 매트릭스의 도움으로 주제를 개발합니다(Gale 외. 2013). 사례(즉, 분석 단위)는 종종 개별 참여자이지만 사례는 참여자 그룹, 조직 또는 다른 범주일 수도 있습니다. 이 접근 방식은 사례를 비교하고 대조하는 것을 장려하기 때문에 데이터 세트가 비교적 균질하고 일관되게 수집되는 것이 중요합니다(Gale 외. 2013).
To illustrate how to use FAMs, we select one from underneath this umbrella: the framework method (FM). FM uses a pattern-based and structured set of techniques to organize data into a matrix where cases intersect with codes, and eventually developing themes with the help of the framework matrix (Gale et al. 2013). The case (i.e. the unit of analysis) is often an individual participant, but a case could also be a participant group, an organization, or another categorization. Since this approach encourages the comparing and contrasting of cases, it is important that the dataset be relatively homogenous and consistently collected (Gale et al. 2013).
연역적 접근법에서는 기존 이론, 선행 연구 또는 특정 연구 목표를 기반으로 주제 및/또는 코드를 미리 선택하고, 귀납적 접근법에서는 데이터에서 코드와 주제를 생성한 후 분석이 진행됨에 따라 구체화합니다. FM은 전사, 익숙화, 코딩, 작동하는 분석 프레임워크 개발, 분석 프레임워크 적용, 프레임워크 매트릭스에 데이터 차트화, 데이터 해석의 7단계로 구성됩니다(Gale 외. 2013). 아래에서 각 단계를 설명합니다. 이러한 단계는 독립적인 연구자가 수행하거나 연구팀이 공동으로 수행할 수 있습니다. 특히 대규모 데이터 세트로 작업하는 경우, 데이터 관리 및 분석을 돕기 위해 정성적 데이터 분석 소프트웨어를 7단계 중 일부 또는 모두에 활용하는 것이 적절할 수 있습니다. 여기서 설명하는 단계는 FM에 국한된 것이지만, 이 단계에 관련된 작업은 다른 FAM에서 사용되는 많은 프로세스와 유사하다는 점에 유의하는 것이 중요합니다.
FM can be used both deductively and inductively: in the deductive approach, themes and/or codes are pre-selected based on existing theories, prior research or specific study objectives; in the inductive approach, codes and themes are generated from the data and refined as analysis progresses. FM involves seven stages: transcription; familiarization; coding; developing a working analytical framework; applying the analytical framework; charting data into the framework matrix; and interpretating the data (Gale et al. 2013). We describe each of these below. These stages can be carried out by an independent researcher or collaboratively by a research team. Qualitative data analysis software may be relevant to utilize in many or all seven stages to aid data management and analysis, especially if working with a large dataset. It is important to note that the stages we describe are specific to FM; however, the work involved in these stages parallels many of the processes used in other FAMs.
1단계: 전사
Stage 1: Transcription
대부분의 정성적 분석 방법과 마찬가지로, FM을 사용한 데이터 분석 작업은 전사 작업으로 시작되며, 특히 연구자가 전사 작업을 수행하는 경우 더욱 그렇습니다. 따라서 단어 하나하나를 그대로 옮긴(즉, 축어체) 트랜스크립트를 작성하는 것은 데이터에 대한 첫 번째 검토 역할을 합니다. 그러나 외부 계약업체가 전사 작업을 수행하더라도 FM 연구자는 원본 녹음과 대조하여 전사본을 확인하여 정확성을 보장해야 합니다. 이 과정에서 연구자는 데이터에 몰입하여 연구 목적 및 질문과 관련하여 데이터를 고려해야 합니다. 질적 설문조사 응답을 사용하는 경우처럼 녹음된 데이터의 필사본이 필요하지 않은 경우(Palermo 외. 2019), 2단계가 첫 번째 분석 단계가 됩니다.
As with most qualitative analysis methods, the work of data analysis using FM begins with transcription—especially if that transcription work is being done by the researcher. As such, the creation of a word-for-word (i.e. verbatim) transcript acts as a first review of the data. However, even if the transcription work is being conducted by an external contractor, the FM researcher should verify the transcript against the original recording to ensure accuracy. This process requires the researcher to immerse themselves in the data and consider them in relation to the research purposes and questions. If no transcription of recorded data is required, as in the case of using qualitative survey responses (Palermo et al. 2019), stage 2 becomes the first analysis phase.
2단계: 익숙화
Stage 2: Familiarization
다양한 질적 데이터 분석 방법에서 여전히 일반적인 이 단계에서는 연구자가 데이터 세트의 범위와 다양성에 익숙해져야 합니다. 이 단계는 본질적으로 데이터에 더 깊이 몰입하는 작업입니다. 익숙해지기 위해서는 기록이나 응답을 읽거나 녹음을 듣는 것이 포함됩니다. 이 숙지 과정에서 연구자는 핵심 아이디어, 생각, 인상, 반복되는 주제에 대한 맥락 및 반성적 메모를 작성해야 합니다.
This phase of FM, which is still typical for many different qualitative data analysis methods, requires the researcher to become familiar with the range and diversity of the dataset. It is essentially the work of deeper immersion in the data. Familiarization can involve reading of transcripts or responses, and/or listening to recordings. During this familiarization, the researcher should write contextual and/or reflective memos of key ideas, thoughts, impressions, and recurrent themes that they notice.
3단계: 코딩
Stage 3: Coding
코딩의 어떤 형태는 많은 질적 분석 방법에서 공통적이지만, 이 단계에서는 FM이 다른 방법과 더욱 뚜렷해지기 시작하고 FM의 변형(예: 귀납적 또는 연역적)도 서로 달라지기 시작합니다. 이는 질적 방법론과 분석 방법마다 코딩에 접근하는 방식이 매우 다르기 때문입니다. 이 FM 단계에서 연구자는 간단한 설명 코드 또는 의역된 데이터 발췌문으로 데이터 세그먼트에 레이블을 지정합니다. 이 라인별 작업의 목표는 데이터를 조사할 수 있는 주요 문제와 개념을 식별하는 것입니다(Ritchie and Spencer 1994). 코드를 개발할 때 연구자는 데이터 세트의 모든 트랜스크립트(즉, 모든 사례)로 작업하거나 일부만 사용하여 코드를 개발하기로 결정할 수 있습니다.
While some form of coding is common to many qualitative analysis methods, this is the phase where FM starts to become more distinct from other methods, and where variations of FM (e.g. inductive or deductive) also differ from each other. This is because different qualitative methodologies and analysis methods approach coding in very different ways. During this FM stage, the researcher labels data segments with brief descriptive codes or paraphrased data excerpts. The goal of this line-by-line work is to identify key issues and concepts according to which the data can be examined (Ritchie and Spencer 1994). When developing codes, it may be that the researcher decides to develop codes by working with all transcripts (i.e. all cases) in the dataset or by using only a few.
귀납적 분석을 지원하기 위해 FM을 사용할 때 Gale 등(2013)은 다음과 같이 제안합니다:
When using FM to support inductive analysis, Gale et al. (2013) suggest that:
코드는 실체적인 것(예: 특정 행동, 사건 또는 구조), 가치(예: 근거 기반 의학 또는 환자 선택에 대한 믿음과 같이 특정 진술을 알리거나 뒷받침하는 것), 감정(예: 슬픔, 좌절, 사랑) 및 보다 인상적/방법론적 요소(예: 인터뷰 대상자가 설명하기 어려운 것을 발견함, 인터뷰 대상자가 감정적이 됨, 인터뷰자가 불편함을 느낌)를 나타낼 수 있습니다. (p. 4)
Codes could refer to substantive things (e.g., particular behaviours, incidents or structures), values (e.g., those that inform or underpin certain statements, such as a belief in evidence-based medicine or in patient choice), emotions (e.g., sorrow, frustration, love), and more impressionistic/methodological elements (e.g., interviewee found something difficult to explain, interviewee became emotional, interviewer felt uncomfortable). (p. 4)
이와는 대조적으로, 순수 연역적 FM 연구에서는 주제가 미리 정의되어 있고, 이론, 모델 또는 문헌의 기존 연구 결과를 바탕으로 코드에 정보를 제공합니다(Onyura 외. 2017). 귀납적-연역적 결합 접근 방식을 결합한 경우, 코딩은 미리 식별되거나 개발된 프레임워크에 의해 부분적으로 정보를 얻을 수 있으며, 귀납적 코딩은 연역적으로 결정된 주제에 대한 하위 주제를 개발하는 데 사용될 수 있습니다(Redman et al. 2017).
In contrast, in a purely deductive FM study, themes will have been pre-defined, informed by a theory, a model, or existing findings from the literature, which then informs codes (Onyura et al. 2017). In combined inductive-deductive approaches, the coding can be partly informed by pre-identified or developed frameworks, and inductive coding can be used to develop sub-themes to deductively determined themes (Redman et al. 2017).
코드가 인사이트를 개발하는 기반이 되기 때문에 코드 개발은 FM에서 특히 중요한 측면입니다. Gale 등(2013)은 다음과 같이 설명합니다: '코딩은 모든 데이터를 분류하여 데이터 세트의 다른 부분과 체계적으로 비교할 수 있도록 하는 것을 목표로 합니다'(4페이지). 연구의 성공에 있어 코드의 중요성을 고려할 때, 연구자는 코드가 '적합하지 않거나' 데이터와 잘 맞지 않을 때를 인식하는 것이 필수적입니다. 이러한 상황에서는 연구자가 코드를 재고하고 개선할 준비가 되어 있어야 한다고 Ritchie와 Spencer(1994)는 제안합니다. 이는 연구팀에서 논의함으로써 이루어질 수 있으며, 이러한 관행은 데이터 분석을 더욱 견고하게 만들 것입니다.
The development of codes is a particularly important aspect of FM since codes are the foundations from which insights are developed. As Gale et al. (2013) explain: ‘coding aims to classify all of the data so that it can be compared systematically with other parts of the dataset’ (p. 4). Given the centrality of codes to the success of the study, it is essential that the researcher recognizes when codes are not a good ‘fit’ or not well aligned with the data. In these situations, Ritchie and Spencer (1994) suggest that the researcher be ready to reconsider and refine codes. This might be done by discussing them in the research team—a practice that should make the data analysis more robust.
4단계: 작업용 분석 프레임워크 개발하기
Stage 4: Developing a working analytical framework
코드가 개발되면 연구자는 작업용 분석 프레임워크를 구축하여 보다 고유하고 정의적인 FM 단계로 진행합니다. 프레임워크는 명확하게 정의되고 설명된 전체 코드 집합으로 구성되며, 일부 코드는 카테고리로 묶여 있습니다. 팀으로 작업하는 경우 이 단계에서는 서로 다른 팀원들의 코딩 접근 방식을 조율해야 하며, 정확한 프로세스는 특정 연구에 대해 취하는 실용적이고 철학적 접근 방식과 분석 프로세스의 목표에 따라 달라집니다. 예를 들어
- 귀납적 접근 방식으로 작업하는 경우, 연구팀은 코드의 개념화 전반에 걸친 차이를 어떻게 수용할지 결정해야 합니다(예: 한 연구자의 코드 'X'가 다른 연구자의 코드 'Y'와 충분히 겹쳐서 서로 병합할 수 있는가?). 이와 대조적으로
- 연역적 접근 방식에서는 연구자가 기존의 개념적 틀을 활용할 수 있지만(예: Onyura 외. 2017 및 표 4 참조), 연구자는 기존 이론, 모델 및/또는 문헌 기반 코드에 대한 각 코더의 해석이 일치하는지를 확인해야 합니다.
Once codes have been developed, the researcher progresses to the more unique and defining stages of FM by constructing a working analytical framework. This framework consists of the full set of codes—clearly defined and described—and with some codes being clustered together into categories. If working in a team, this phase will involve harmonization of different team members’ coding approaches; the exact process for that will depend on the practical and philosophical approach taken for a specific study, as well as the aims of the analysis process. For instance,
- if working from an inductive approach, the research team will need to determine how to accommodate differences across the conceptualizations of codes (e.g. does one researcher’s code ‘X’ overlap sufficiently with another researcher’s code ‘Y’ so that they can be merged together?). In contrast,
- in a deductive approach, researchers may draw on an existing conceptual framework (see e.g. Onyura et al. 2017 and Table 4), but the researchers will then need to confirm that each coder’s interpretation of the pre-existing theory, model, and/or literature-based codes are aligned.
연구자가 프레임워크가 연구 질문에 답할 수 있는 모든 관련 데이터를 포착하고 있다고 판단하기까지 여러 번의 반복이 필요하기 때문에 (귀납적 접근 방식과 연역적 접근 방식 모두) 프레임워크 개발 작업은 힘들 수 있습니다. Gale 등(2013)이 지적한 것처럼, 분석 프레임워크가 완성될 때까지 '기타' 또는 '기타'와 같은 자리 표시자 코드를 사용하여 점진적으로 개선할 수 있는 공간을 확보하는 것이 좋습니다. 연구자가 귀납적으로 데이터에 접근할수록 분석에 더 많은 시간이 소요될 수 있다는 점에 유의할 필요가 있습니다. 이는 분석 방법이나 접근 방식을 선택하기 전에, 그리고 분석 프로세스를 시작할 때 고려해야 할 실용적이고 방법론적인 고려 사항입니다.
This work of developing the framework can be laborious since—for both inductive and deductive approaches—several iterations will be required before the researcher decides that the framework is capturing all the relevant data that will enable them to answer their research question(s). As Gale et al. (2013) point out, it is worth making space for this gradual refinement by using placeholder codes such as ‘other’ or ‘miscellaneous’ until the analytical framework can be finalized. It is worth noting that the more inductively the researcher approaches the data, the more time the analysis is likely to take. This is both a practical and methodological consideration worth giving some thought to prior to selecting an analysis method or approach, and when starting the analysis process.
5단계: 분석 프레임워크 적용
Stage 5: Applying the analytical framework
이전 단계에서 개발한 분석 프레임워크를 적용하기 위해 분석 프레임워크에 나열되고 설명된 코드를 사용하여 데이터 세트의 모든 트랜스크립트를 코딩합니다(즉, 코드와 관련된 모든 데이터는 수동으로 또는 소프트웨어의 태그를 사용하여 표시합니다). 연구 데이터 세트의 크기에 따라 질적 데이터 분석 소프트웨어를 사용하여 프레임워크를 적용하는 작업을 신속하게 처리할 수 있습니다. 데이터 세트에 많은 기록(예: 사례)이 있는 경우, 소프트웨어는 연구자가 모든 데이터를 코딩하고 정리할 뿐만 아니라 데이터 발췌문을 독립형 문서로 내보내 사례 간 비교를 용이하게 하는 데 도움을 줄 수 있습니다.
To apply the analytical framework developed in the previous phase, all transcripts in the dataset will be coded (i.e. all data pertaining to a code will be marked, either manually or using tags in a software) using the codes listed and described in the analytical framework. Depending on the size of the study’s dataset, the work of applying the framework can be expedited using qualitative data analysis software. If the dataset has many transcripts (i.e. cases), software can help the researcher not only to code and organize all data, but also to export data excerpts into stand-alone documents, thereby facilitating cross case comparisons.
6단계: 프레임워크 매트릭스에 데이터 차트화
Stage 6: Charting data into the framework matrix
데이터를 코딩하는 데 사용된 분석 프레임워크를 기반으로 코드(또는 카테고리나 주제와 같은 상위 수준의 구성 요소, 예: Klingberg 외, 2022)와 사례(또는 사례 그룹, 예: Klingberg 외, 2022)를 스프레드시트 구조(예: 열은 코드를 나타내고 행은 사례를 나타내는 구조)로 결합하여 프레임워크 매트릭스를 구성합니다. 각 사례 및 코드에 대해 해당 코드와 관련된 성적표의 측면에 대한 요약 설명을 포함하거나 대표적인 인용문을 매트릭스에 도표로 표시할 수 있습니다. 이렇게 하면 여러 사례 또는 그룹에서 패턴과 뉘앙스를 추적할 수 있습니다.
Based on the analytical framework used to code the data, a framework matrix is constructed by combining codes (or higher-level constructs, such as categories or themes, e.g. Klingberg et al. 2022) and cases (or groups of cases, e.g. Klingberg et al. 2022) into a spreadsheet structure (e.g. where columns represent codes and rows represent cases). For each case and code, a summary description of the aspects of the transcript that relate to that code can be included, or a representative quote can be charted into the matrix. This allows for patterns and nuances to be traced across the different cases or groups.
7단계: 데이터 해석
Stage 7: Interpreting the data
코드를 결정하고 메모를 작성할 때 분석의 초기 단계에서 이미 어느 정도의 해석이 이루어졌다면, 보다 공식적인 데이터 해석 및 테마 생성 단계는 데이터를 프레임워크 매트릭스에 차트화한 후에 이루어집니다. 이 단계에서는 연구자가 연구 목적과 연구를 주도하는 연구 질문을 검토합니다. Ritchie와 Spencer(1994)는 이 단계의 기본 프로세스로 연구자가 차트화된 데이터를 검토하고, 연구 노트/메모를 검토하고, 사례 간 비교 및 대조하고, 패턴과 연관성을 찾고, 연구 질문과 관련된 결과를 밝히는 데 도움이 되는 설명을 찾아야 한다고 언급했습니다. Gale 등(2013)은 다음과 같이 잠재적인 해석 방법을 설명합니다:
While some level of interpretation already takes place during the earlier phases of analysis when deciding on codes and writing memos, the more formal phase of data interpretation and theme generation occurs after charting data into the framework matrix. This is when the researcher reviews the study purposes and research question(s) driving the research. Ritchie and Spencer (1994) noted that the basic processes for this stage require the researcher: to review the charted data; to examine research notes/memos; compare and contrast across cases; search for patterns and connections; and to seek out descriptions that help to illuminate findings relevant to the research question(s). Gale et al. (2013) describe potential interpretive avenues as follows:
점진적으로 데이터의 특징과 데이터 간의 차이점을 파악하여 유형학을 생성하고, 이론적 개념(이전 개념 또는 데이터에서 나온 개념)을 질문하거나, 범주 간의 연결을 매핑하여 관계 및 인과관계를 탐색합니다. 데이터가 충분히 풍부하다면 이 과정을 통해 도출된 결과는 특정 사례에 대한 설명을 넘어 현상의 출현 이유, 조직이나 기타 사회적 행위자가 상황을 어떻게 유발하거나 대응할지 예측하거나 조직이나 시스템 내에서 제대로 작동하지 않는 영역을 식별하는 데까지 확장될 수 있습니다(5페이지).
Gradually, characteristics of and differences between the data are identified, perhaps generating typologies, interrogating theoretical concepts (either prior concepts or ones emerging from the data) or mapping connections between categories to explore relationships and/or causality. If the data are rich enough, the findings generated through this process can go beyond description of particular cases to explanation of, for example, reasons for the emergence of a phenomena, predicting how an organisation or other social actor is likely to instigate or respond to a situation, or identifying areas that are not functioning well within an organisation or system (p. 5).
또한 데이터 해석은 종종 작성 과정에서 개발되고 개선되므로, 연구 원고를 작성하는 과정도 분석 과정의 일부로 간주된다는 점을 기억하는 것이 중요합니다. Gale 등(2013)은 주제를 분석의 최종 결과물로 설명하지만, 해석의 정확한 과정과 결과는 연구 목적, 분석 접근법(귀납적 또는 연역적), 해당 연구의 설계에 따라 달라집니다.
It is also important to remember that data interpretations are often developed and refined throughout the writing process and so the process of writing the research manuscript is considered part of the analytic process. While Gale et al. (2013) describe themes as the final output of analysis, the exact process and outcome of interpretation will depend on the purpose, analysis approach (i.e. inductive or deductive), and design the study in question.
HPE 연구에서의 FAM 예시
Examples of FAMs in HPE research
과거에 HPE 학자들이 FAM을 어떻게 성공적으로 사용했는지 설명하기 위해 표 4에는 각각 약간 다른 방식(예: 귀납적, 연역적 또는 두 접근법의 조합)으로 FAM을 활용하는 현장에서 발표된 사례가 요약되어 있으며, 질적 또는 혼합 방법 HPE 연구에서 다양한 목적에 맞는 FAM의 다용도성을 보여 줍니다.
To illustrate how HPE scholars have successfully used FAMs in the past, Table 4 summarizes published examples from the field, each utilizing FAMs in slightly different ways (e.g. inductively, deductively or a combination of the approaches), illustrating the versatility of FAMs for different purposes in qualitative or mixed methods HPE research.
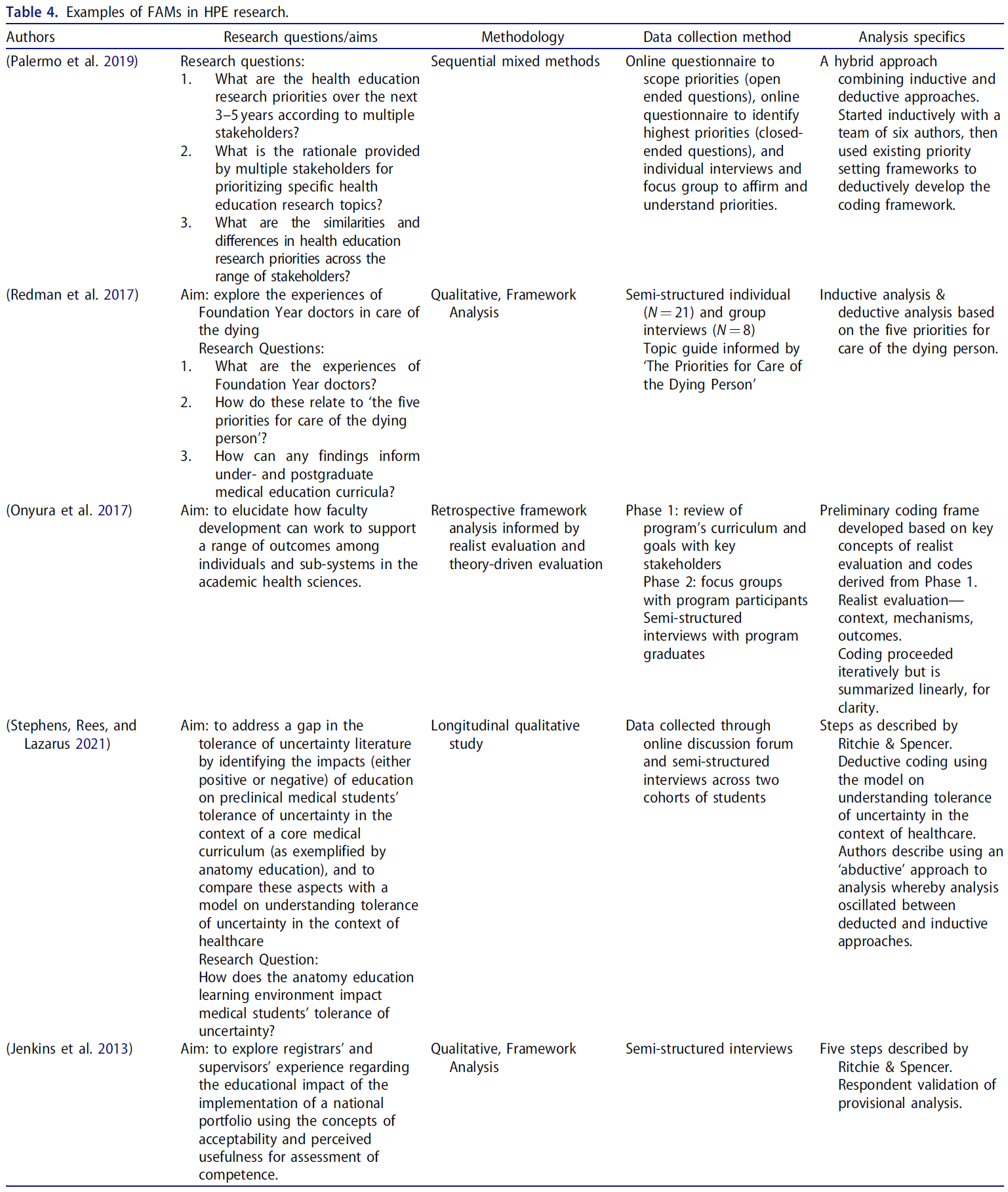
피해야 할 함정
Pitfalls to avoid
귀납적 및 연역적으로 모두 적용 가능하고, 대규모 정성적 데이터 세트를 분석할 수 있는 수단을 제공하며, 데이터 분석 프로세스에 비교적 구조화된 접근 방식을 제공하는 등 FAM은 상당한 이점을 제공하지만, 연구자가 피해야 할 몇 가지 함정도 있습니다. 첫 번째 함정은 특정 패러다임 내에서 연구를 진행하지 않고 FAM을 사용하는 것입니다(표 1 참조). HPE의 연구자들은 개별 학자들이 제기하는 질문, 개발할 수 있는 지식의 종류, 유용한 방법의 종류, 기대되는 엄격성의 표준을 개념화하는 방식을 형성하는 다양한 연구 전통에 따라 연구에 참여합니다. 이러한 전통 또는 패러다임은 HPE 연구자가 개별 FAM을 활용하는 방식을 변화시킬 것입니다. 따라서 연구자는 단순히 FAM의 단계를 따른다고 가정해서는 안 되며, 패러다임적 방향과 연구의 품질 및 엄격성에 대한 기본 기대치에 맞게 FAM 사용을 조정해야 합니다(Varpio 및 MacLeod 2020).
Although FAMs offer significant benefits (e.g. being applicable both inductively and deductively; offering means of analyzing large qualitative datasets; and offering relatively structured approach to the data analysis process), there are also certain pitfalls that researchers will want to avoid. The first pitfall is using FAMs without situating their research within a specific paradigm (see Table 1). Researchers in HPE engage in their studies from different research traditions that shape how individual scholars conceptualize the questions being asked, the kind of knowledge that can be developed, the kinds of methods that are useful, and the standards of rigor that are to be expected. These traditions—or paradigms—will change the way the HPE researcher harnesses individual FAMs. Therefore, researchers should not assume that they simply follow the stages of an FAM; instead, they must tailor their FAM use to align with their paradigmatic orientation and the underlying expectations of quality and rigor for the study (Varpio and MacLeod 2020).
또 다른 함정은 [해석]과 [FAM의 보다 구조화된(그리고 잠재적으로 더 따르기 쉬운) 요소] 사이의 균형을 맞추는 문제입니다. 예를 들어, Gale 외(2013)의 FM에서 테마는 전체 데이터 집합을 분석한 최종 결과물로 설명되지만, 분석 단계가 프레임워크 매트릭스에서 테마를 정확히 어떻게 개발해야 하는지를 지시하지는 않습니다. 이는 연구의 접근 방식(예: 귀납적, 연역적 또는 혼합)과 목적(예: 설명적 또는 탐색적)에 따라 달라집니다. 이때 연구 도구로서 질적 연구자의 역할이 강조되어야 하며, 각 연구자는 자신이 선호하는 방식으로 주제를 개발하는 작업에 참여하게 됩니다.
- 귀납적 접근법의 경우, 프레임워크 매트릭스 구조를 사용하여 코드, 범주, 사례 비교를 점진적으로 읽고 다시 읽으면서 이러한 요소들 간의 연결고리를 만들어내는 주제에 대한 분석 메모를 생성하는 등 주제 개발을 지원하는 것이 도움이 될 수 있습니다.
- 연역적 접근법의 경우, 테마는 분석의 결과라기보다는 입력이므로 해석 작업은 관련 테마에 맞춰 코딩 및 프레임워크 개발을 수행하는 데 중점을 둡니다.
연구를 뒷받침하는 접근 방식과 목표에 관계없이 모든 연구 과정과 결과가 목표, 목적 및 수행된 관행에 따라 투명하고 일관되게 보고되는 것이 중요합니다.
Another pitfall is the challenge of balancing interpretation with the more structured (and potentially easier to follow) elements of an FAM. For example, in Gale et al. (2013) FM, themes are described as the final output of analyzing the entire dataset, but the stages of analysis do not dictate how exactly to develop themes from the framework matrix. This will depend on the approach (i.e. inductive, deductive, or mixed) and aim (e.g. descriptive or exploratory) of the study. This is where the role of the qualitative researcher as a research instrument must be emphasized; each researcher will engage in the work of developing themes in their own preferred way.
- In an inductive approach to FM, it may be helpful to use the framework matrix structure to support the development of themes by, for example, gradually reading and re-reading codes, categories, and case comparisons to generate analytic memos of themes they identity that create connections between these elements.
- In the case of deductive approaches, themes are inputs rather than outputs of the analysis and, as such, the work of interpretation centers on carrying out coding and framework development in line with the relevant themes.
Regardless of the approach and aim that underpins the study, it is important that all research processes and findings are reported transparently and consistently with aims, objectives, and undertaken practices.
결론
Conclusion
이 AMEE 가이드에서는 FAM과 이 포괄적인 용어 아래에 포함될 수 있는 다양한 접근법을 명확하게 설명하기 위해 노력했습니다. 귀납적 접근법과 연역적 접근법을 모두 사용할 수 있다는 점에 주목하여 FAM의 유연성을 강조했습니다. 또한 FM을 예로 들어, 독자들이 이러한 방법을 사용하는 연구와 관련된 분석 작업의 종류를 이해할 수 있도록 특정 FAM에 참여하는 단계에 대해 설명했습니다. 다른 질적 분석 접근 방식과 마찬가지로 FAM에는 몇 가지 위험과 함정이 수반되지만, 우리가 제공한 예와 권장 사항이 더 많은 HPE 연구자가 해당 분야에서 이러한 방법을 사용하는 데 도움이 되기를 바랍니다.
In this AMEE guide, we have endeavored to provide clarity around FAMs and the variety of approaches that can sit beneath that umbrella term. We have highlighted the flexibility of FAMs, noting that they can be used both inductively and deductively. Using FM as an example, we have also described the stages involved in engaging in a particular FAM to provide readers with an understanding of the kind of analytical work involved in research using these methods. As with any qualitative analysis approach, FAMs involve some risks and pitfalls but we hope that the examples and recommendations we have provided can help more HPE researchers use these methods in our field.
Med Teach. 2023 Sep 21:1-8. doi: 10.1080/0142159X.2023.2259073. Online ahead of print.
Using framework analysis methods for qualitative research: AMEE Guide No. 164
Affiliations
1SAMRC/Wits Developmental Pathways for Health Research Unit, Faculty of Health Sciences, University of the Witwatersrand, Johannesburg, South Africa.
PMID: 37734451
Abstract
Framework analysis methods (FAMs) are structured approaches to qualitative data analysis that originally stem from large-scale policy research. A defining feature of FAMs is the development and application of a matrix-based analytical framework. These methods can be used across research paradigms and are thus particularly useful tools in the health professions education (HPE) researcher's toolbox. Despite their utility, FAMs are not frequently used in HPE research. In this AMEE Guide, we provide an overview of FAMs and their applications, situating them within specific qualitative research approaches. We also report the specific characteristics, advantages, and disadvantages of FAMs in relation to other popular qualitative analysis methods. Using a specific type of FAM-i.e. the framework method-we illustrate the stages typically involved in doing data analysis with an FAM. Drawing on Sandelowski and Barroso's continuum of data transformation, we argue that FAMs tend to remain close to raw data and be descriptive or exploratory in nature. However, we also illustrate how FAMs can be harnessed for more interpretive analyses. We propose that FAMs are valuable resources for HPE researchers and demonstrate their utility with specific examples from the HPE literature.
Keywords: Qualitative research; framework analysis; framework method; qualitative analysis; qualitative methods.
'Articles (Medical Education) > 의학교육연구(Research)' 카테고리의 다른 글
서사 탐구: 의학교육의 관계적 연구 방법론(Med Educ, 2017) (0) | 2023.12.06 |
---|---|
해석적 기술: 의학교육연구를 위한 유연한 질적방법론(Med Educ, 2021) (0) | 2023.12.02 |
대관절 누구의 문제인가? 보건의료전문직 교육에서 '문제'의 신화 직면하기 (Med Educ, 2023) (0) | 2023.10.14 |
혼합방법연구에서 데이터를 통합하는 세 가지 테크닉(BMJ. 2010) (0) | 2023.09.18 |
왜 개방형 설문 질문이 강건한 질적 인사이트를 지지하기 어려운가(Acad Med, 2018) (0) | 2023.09.18 |