보건의료전문직 교육에서 인공지능: 스코핑 리뷰(JMIR Med Educ. 2021)
Artificial Intelligence Education Programs for Health Care Professionals: Scoping Review
Rebecca Charow1,2, MSc; Tharshini Jeyakumar2, MHI; Sarah Younus2, MPH; Elham Dolatabadi1,3, MESc, PhD;
Mohammad Salhia4, MEd; Dalia Al-Mouaswas4, HBSc; Melanie Anderson2, MLiS; Sarmini Balakumar1,4, BSc;
Megan Clare4, HBSc; Azra Dhalla3, MBA; Caitlin Gillan1,2,5, MEd; Shabnam Haghzare2,3,6, BSc; Ethan Jackson3,
PhD; Nadim Lalani3, BA; Jane Mattson4, BSc, MLT; Wanda Peteanu4, MHSc; Tim Tripp2, MLiS; Jacqueline Waldorf4,
EMBA; Spencer Williams2, BSc; Walter Tavares1,2,5,7, PhD; David Wiljer1,2,5,8, PhD
서론
Introduction
배경
Background
보건 과학, 교육 및 실습에서 인공지능(AI) 기술의 광범위하고 신속한 채택은 환자 치료를 제공하는 새로운 방법을 도입한다[1]. AI는 복잡한 기계에 인간과 같은 인식, 지능 및 문제 해결을 통합할 수 있는 기술을 포함하는 컴퓨터 과학 내에서 더 넓은 용어를 포함한다[2]. 의료 분야의 빅데이터는 고성능 컴퓨팅 파워와 함께 AI, 머신러닝(ML) 및 딥 러닝의 활용을 가능하게 하여 특히 임상 의사 결정 및 보건 분야 효율성을 향상시켰다[3]. 보다 최근에는 방사선과, 마취과, 피부과, 외과 및 약학 분야에서 AI 지원 기술이 계속 등장하고 있다[4-7]. AI가 임상 추론을 대체할 가능성은 낮지만, Mesko[8]는 AI가 관행의 특성(예: 관련된 반복적인 작업의 정도 및 작업이 데이터 기반인지 여부)에 따라 다양한 정도로 모든 전문성에 영향을 미칠 것으로 예측한다. 그러나 의료에서 AI 지원 기술의 효율성은 이러한 기술을 개발하고 검증하는 데 있어 의료 전문가(HCP)의 참여에 달려 있다. 따라서 HCP는 이러한 전환에서 역할을 해야 하며 AI 채택이 그들의 전문성과 조직에 어떤 영향을 미칠지 형성하는 모든 측면에 관여해야 한다.
The widespread and rapid adoption of artificial intelligence (AI) technologies in health sciences, education, and practices introduces new ways of delivering patient care [1]. AI encompasses a broader term within computer science, which includes technologies that can incorporate human-like perception, intelligence, and problem-solving into complex machines [2]. Big data in health care, along with high-performance computing power, has enabled the use of AI, machine learning (ML), and deep learning, in particular, to improve clinical decision-making and health sector efficiency [3]. More recently, AI-enabled technologies have continued to emerge, predominantly in the medical fields of radiology, anesthesiology, dermatology, surgery, and pharmacy [4-7]. Although AI is not likely to replace clinical reasoning, Mesko [8] predicts that AI will influence all specialties in varying degrees, depending on the nature of the practice (eg, the degree of repetitive tasks involved and whether the tasks are data driven). However, the efficacy of AI-enabled technologies in health care depends on the involvement of health care professionals (HCPs) in developing and validating these technologies. Therefore, HCPs should play a role in this transformation and be involved in every aspect of shaping how AI adoption will affect their specialties and organizations.
HCP 참여에 대한 권고안이 대두되고 있다. 예를 들어, 의료 영상 분야에서 웨스트와 앨런[9]은 HCP가 다음과 같이 관여할 것을 권고한다.
- (1) 데이터 표준을 구현하고 실제로 이를 준수
- (2) 의학에서 AI의 사용 사례 우선 순위 지정
- (3) 잠재적 알고리즘의 임상적 영향을 결정
- (4) 데이터 과학자와 연구자를 위한 직업의 필요성을 설명하고 명확하게 설명
- (5) 인간 언어에서 기계 언어로 연습 요구를 번역하는 데 참여
이러한 기술이 등장함에 따라, HCP와 교육자들은 이러한 변화를 신속하게 개발하고 그들의 관행과 분야에 통합하는 데 필요한 역량을 갖추는 것이 필수적이다.
Recommendations for HCP involvement are emerging. For instance, in the field of medical imaging, West and Allen [9] recommend that HCPs be involved in
- (1) implementing data standards and following them in practice,
- (2) prioritizing use cases of AI in medicine,
- (3) determining the clinical impact of potential algorithms,
- (4) describing and articulating the needs of the profession for data scientists and researchers, and
- (5) participating in the translation of practice needs from human language into machine language.
As these technologies emerge, it is essential for HCPs and educators to have the competencies required to rapidly develop and incorporate these changes into their practices and disciplines.
개인 차원에서 [AI 리터러시 부족]은 다양한 의료 전문 분야에서 AI 지원 기술을 최대한 활용하고 채택하는 데 상당한 장벽이다. 특히 AI 교육 프로그램에서는 다양한 수준의 의학 교육(학부, 대학원, 실습 기반 교육 또는 지속적인 전문성 개발)에서 구현에 장벽이 존재한다. 예를 들어,
- 건강 정보학은 현대 의학에서 중요한 역할을 하지만, 대부분의 의과대학 커리큘럼의 초점은 아니다[2].
- 전자 임상 도구 사용에 대한 교육을 제공하기 위해 테크놀로지 전문가와 상담하는 경우는 많지만, 그렇다고 해서 전자electronic 임상 도구 사용이 환자 상호 작용 향상과 치료 개선에 어떻게 사용될 수 있는지를 이해하는 데 필요한 기술skill 수준을 지원하지 않는다[10].
- 또 다른 예는 방사선 레지던트 프로그램 내에 존재하며, 여기에는 AI 구현 및 사용에 대한 인식 부족이 채택의 장벽으로 언급되었다[11,12].
At the individual level, a lack of AI literacy is a significant barrier to the adoption and use of AI-enabled technologies to their full capacity in various medical specialties. In AI education programs specifically, there are barriers to implementation at various levels of medical education (undergraduate, postgraduate, practice-based education, or continuing professional development). For instance,
- health informatics plays a valuable role in modern medicine; yet, it is not the focus of most medical school curricula [2].
- Technology experts are often consulted to provide training on the use of electronic clinical tools, but this does not support the level of skill required to understand how it could be used to enhance patient interactions and improve care [10].
- Another example exists within radiology residency programs, where the lack of awareness as well as lack of knowledge of implementing and using AI were cited as barriers to its adoption [11,12].
보건 전문가의 커리큘럼에 [AI 기초]를 통합하는 것은 필수적이며, 미래의 HCP가 자신의 임상 판단의 맥락에서 AI를 고려할 수 있도록 권한을 부여함empowering으로써, [이러한 지식]과 [환자 중심 치료를 제공하는 것]의 균형을 맞추는 것이 유용할 것이다. [스스로의 판단에 대한 신뢰]와 [기본적인 통계 지식]의 조합은 임상 실습에서 새로운 AI 기반 기술을 가장 잘 적용하는 방법을 이해하는 데 유용할 것이다[13]. AI는 의료 분야에서 HCP의 광범위한 기술 세트, 우선순위 및 궁극적인 목표의 맥락에서 고려되어야 한다. 여기에는 임상 실무에서 환자 중심적이고 동정적인 치료를 장려하는 것이 포함된다[13,14].
Incorporating AI fundamentals into health professionals’ curricula is essential, and it would be useful to balance this knowledge with providing patient-centered care by empowering future HCPs to consider AI in the context of their own clinical judgment. The combination of trust in their own judgment and basic statistical knowledge will be useful in understanding how to best apply new AI-driven technologies in clinical practice [13]. AI needs to be considered within the context of HCPs’ broader skill sets, priorities, and ultimate goals in health care; this includes encouraging patient-centered, compassionate care in clinical practice [13,14].
[Martec의 법칙]은 이러한 [기술을 채택하는 조직의 능력]에 비해 [기술 변화]가 훨씬 더 빠르게, 그리고 실제로 기하급수적으로 일어난다는 생각을 말합니다[1]. 따라서 조직은 혁신 기술을 적극적으로 홍보하고 전문 인력이 AI 기반 도구를 실제로 성공적으로 구현할 수 있도록 적절한 교육을 받을 수 있도록 해야 합니다[1]. 이러한 신기술을 [개인의 수준]과 [조직의 문화 및 운영 범위] 내에서 효과적이고 동정적으로 통합하기 위해서는 [일치되고 신중한 접근 방식]이 필요하다[1].
Martec’s Law refers to the idea that technology changes occur much more rapidly, and in fact exponentially, compared with the ability of organizations to adopt these technologies [1]. Therefore, organizations need to promote innovative technologies proactively and empower their professionals to be adequately trained to successfully implement AI-based tools in their practice [1]. A concerted, deliberate approach is required to incorporate these new technologies, both effectively and compassionately, at an individual level and within the culture and operations of an organization [1].
이러한 기술을 구현하는 데 있어 많은 [잠재적 장벽]이 존재합니다. 확인된 세 가지 주요 제한 사항에는 규제, 경제 및 조직 문화 문제가 포함됩니다[15].
- 임상 환경에서 AI 기술을 [채택]하려면 규제 승인[16]이 필요하며, 이러한 기술을 환자 치료에 사용하는 데 있어 잠재적 책임뿐만 아니라 임상 의사 결정을 위한 AI 알고리듬의 안전성, 유효성 및 투명성을 고려해야 한다[17,18].
- AI 채택을 위해 [데이터에 액세스]하는 경우에도 규제 문제가 발생할 수 있습니다. 알고리즘 개선 및 검증과 함께 수반되는 연구 윤리 위원회 및 규제 승인을 위해 다중 기관 데이터 공유가 필요합니다 [18].
- 채택을 더욱 개선하기 위해, 이러한 기술은 또한 [경제적]이어야 하고 적절한 자금 지원을 받아야 하며 [18] 조직 자체에도 가치가 있는 것처럼 보여야 합니다.
- 조직 수준에서 AI의 사용은 조직의 [목표와 전략계획]과 일치해야 한다. 조직은 AI 기술이 데이터 웨어하우스 및 전자 건강 기록을 포함한 [기존 시스템에 얼마나 잘 통합]될지 평가해야 한다[18].
- 여전히 원활하게 작동하고 개별 수준에서 임상적으로 유용하면서 조직 수준에서 가치가 입증될 정도로 다양한 임상 상황에 걸쳐 특정 AI 모델을 일반화하는 것은 어려울 수 있다[15].
- 또한, AI 기술을 채택하기로 선택할 때, 조직은 외부 공급업체와 협력하거나 사내에서 기술을 개발할 수 있으며, 이는 추가적인 인적 및 물적 자원을 사용해야 한다[15].
A number of potential barriers to implementing these technologies exist; the 3 main limitations identified include regulatory, economic, and organizational culture issues [15].
- Regulatory approval [16] is needed to adopt AI technologies in clinical settings, and potential liabilities in using these technologies for patient care must be considered, as well as the safety, efficacy, and transparency of AI algorithms for clinical decision-making [17,18].
- Regulatory issues can also come into play when it comes to accessing data for AI adoption; multi-institution data sharing is required for algorithm improvement and validation, as well as the accompanying research ethics board and regulatory approvals [18].
- To further improve adoption, these technologies will also have to be economical, supported by adequate funding [18], and seem as valuable to the organization itself. At an organizational level, the use of AI should align with the goals and strategic plans of an organization; organizations will need to assess how well the AI technology will integrate into existing systems, including data warehouses and electronic health records [18].
- It may be difficult to generalize a particular AI model across different clinical contexts to a degree that would prove valuable at an organizational level while still working seamlessly and being clinically useful at the individual level [15].
- Furthermore, when choosing to adopt AI technologies, organizations can either collaborate with outside vendors or create the technologies in-house, which will require the use of additional human and material resources [15].
목적
Objective
AI 교육의 적자는 국립 의학 아카데미의 5중 목표 모델의 일부로서 AI 통합에 대한 요구에도 불구하고 환자 치료를 개선하기 위해 AI 기술을 완전히 통합하고 채택할 수 있는 의료 시스템의 용량capacity 부족에 기여할 수 있다[19]. 의료 기관 및 이해 관계자가 의료 전달을 강화하고 최적화하는 데 AI를 활용할 수 있는 인지, 정신 운동 및 정서적 기술을 갖추도록 하는 것이 중요하다. 여기에는 또한 모든 유형의 HCP에 널리 이용 가능한 AI 교육 이니셔티브를 지원하는 것도 포함될 것이다. 향후 AI 교육 개발, 보급, 평가를 지원하기 위해서는 보건의료에서 AI 도입 내 현황을 평가하고 AI 교육 실시 대상자, 대상 콘텐츠는 무엇이며 전달 방식, 전문가들이 AI 교육 커리큘럼이 포함되어야 한다고 믿는 것이 반영되는지 여부 등 AI 교육 시행의 범위를 더욱 파악하는 것이 중요하다. 본 범위 검토는 HCP를 위한 AI 교육 프로그램에 대한 기초적 이해를 확립하는 것을 목표로 한다.
Deficits in AI education may be contributing to a lack of capacity in health care systems to fully integrate and adopt AI technologies to improve patient care, despite calls for AI integration as part of the National Academy of Medicine’s Quintuple Aim Model [19]. It is important to equip health care organizations and their stakeholders to have the cognitive, psychomotor, and affective skills to harness AI in enhancing and optimizing the delivery of care. This will also involve supporting AI education initiatives that are widely available for all types of HCPs. To support future AI education development, dissemination, and evaluation, it is important to assess the current situation within AI adoption in health care and further understand the extent of AI education implementation, including who is receiving AI training or education, what content is covered, how it is delivered, and whether this reflects what experts believe that AI education curricula should include. Therefore, this scoping review aims to establish a foundational understanding of education programs on AI for HCPs by determining the following:
- HCP가 의료 서비스 제공을 개선하고 최적화하는 데 AI를 활용할 수 있도록 하는 가장 효과적인 교육 접근 방식은 무엇이었습니까?
- 어떤 커리큘럼 콘텐츠가 제공되었습니까?
- 전달해야 할 콘텐츠의 범위는 어느 정도였습니까?
- Bloom [20]에 의해 공식화된 학습을 위한 분류법을 사용하여 이러한 접근법에 사용된 학습 목표는 무엇인가?
- 어떤 커리큘럼 콘텐츠가 제공되었습니까?
- 이러한 프로그램의 성공과 의료 교육 프로그램에서 AI 커리큘럼의 구현에 기여 요인 또는 장애 요인은 무엇이었습니까?
- Kirkpatrick-Barr Framework [21]를 사용하여 교육 프로그램의 효과를 평가하기 위해 어떤 성과가 사용되었는가?
- What were the most effective educational approaches to enabling HCPs to harness AI in enhancing and optimizing health care delivery?
- What curricular content was delivered?
- What was the scope of content that should be delivered?
- What learning objectives were used in these approaches, using the taxonomy for learning formulated by Bloom [20]?
- What were the enablers or barriers that contributed to the success of these programs and the implementation of AI curricula in health care education programs?
- What outcomes were used to assess the effectiveness of the education programs, using the Kirkpatrick-Barr Framework [21]?
방법들
Methods
개요
Overview
이 범위 검토는 Arcsey 및 O'Malley [22] 지침과 범위 검토용 PRISMA(체계적 검토 및 메타 분석을 위한 선호 보고 항목) 확장 체크리스트 [23,24]를 따랐다. 이 범위 검토의 목적은 HCP에 대한 AI 교육 및 훈련에 대한 현존하는 문헌을 검토하고 요약하는 것이다.
This scoping review followed the Arksey and O’Malley [22] guidelines and the PRISMA (Preferred Reporting Items for Systematic Reviews and Meta-Analyses) Extension for Scoping Reviews checklist [23,24]. The objective of this scoping review is to examine and summarize the extant literature on AI education and training for HCPs.
1단계: 검색 전략
Stage 1: Search Strategy
보건과학 사서(MA)는 AI 및 보건 전문직 교육을 위해 적절한 주제 제목과 키워드를 사용하여 전략을 개발했다. 보건과학 문헌에서 보건직업과 교육과 관련된 용어가 널리 사용된 결과, 결과 집합의 소음을 줄이기 위해 보건직업 교육 개념에 검색을 집중하기로 결정했다. 이러한 제목에 대한 검색은 주요 제목(항목에 대한 데이터베이스 기록에서 가장 중요한 제목)인 위치로 제한되었습니다. 이러한 개념에 대한 키워드는 데이터베이스의 내용과 필드 가용성에 따라 연구 제목, 저자 지정 키워드, 제목 단어, 저널 제목에서만 검색되었다. 언어 또는 날짜 제한이 적용되지 않았습니다. 검색은 실행되었고 결과는 2020년 7월 7일에 다운로드되었다. 전체 전략은 멀티미디어 부록 1을 참조하십시오. 검색 결과에 학회 초록과 프로시딩 내용이 포함된 경우 해당 후속 연구를 찾기 위한 검색이 Google Scholar에서 수행되었습니다. 마지막으로, 초기 선별부터 포함된 연구에서 인용된 모든 논문이 2단계 선별 과정(제목 및 추상 스캔은 물론 전체 텍스트 검토)을 거친 상태에서 핸드 빗 프로세스로도 알려진 진주 재배가 수행되었다.
A health sciences librarian (MA) developed strategies for Ovid MEDLINE All, Ovid Embase, Ovid APA PsycINFO, Ovid Emcare Nursing, Ovid Cochrane Database of Systematic Reviews, Ovid Cochrane Central Register of Controlled Trials, EBSCO ERIC, and Clarivate Web of Science using appropriate subject headings and keywords for AI and health professions education. As a result of the widespread use of terms relating to health professions and education in health sciences literature, the decision was made to focus the searches on health professions education concepts to reduce noise in the results sets. Searches for these subject headings were limited to where they were the major subject heading (the most important subject heading in the database record for an item). Keywords for these concepts were only searched in the study titles, the author-assigned keywords, heading words, and journal titles, depending on the content and field availability of the database. No language or date limits were applied. The searches were run and the results were downloaded on July 7, 2020. For the complete strategies, see Multimedia Appendix 1. If the search results included conference abstracts and proceedings, a subsequent search to find any corresponding follow-up studies was conducted in Google Scholar. Finally, pearl growing, also known as a hand comb process, was conducted where all cited works in the included studies from the initial screening underwent a 2-stage screening process (title and abstract scan as well as full-text review).
2단계: 스터디 선택
Stage 2: Study Selection
2단계 심사 과정은 (1) 제목 및 초록 스캔과 (2) 전문 검토로 구성되었다. 연구 적격성은 두 명의 독립 검토자에 의해 결정되었으며, 두 검토자 간에 합의에 도달하지 못한 경우 충돌을 해결하기 위해 세 번째 검토자가 참여했습니다. 전체 텍스트 검토를 위해 스터디가 포함되고 후속 포함을 위해 선택되기 위해서는 각 단계의 제목과 요약이 다음과 같은 속성을 가져야 합니다.
- 실제 훈련 프로그램이나 교육 개입, 잠재적 훈련 프로그램이나 교육 개입, 그리고 다루어야 할 바람직한 내용에 대해 논의하였다.
- AI에 초점을 맞췄다.
- HCP를 위해 설계되거나 의도되었다.
The 2-stage screening process consisted of (1) title and abstract scan and (2) full-text review. Study eligibility was determined by 2 independent reviewers, and a third reviewer was involved to resolve any conflict when consensus was not reached between the 2 reviewers. For a study to be included for full-text review and to be chosen for subsequent inclusion, the title and abstract at each stage needed to have the following attributes:
- It discussed an actual training program or educational intervention or potential training program or educational intervention and the desired content to be covered.
- It focused on AI.
- It was designed or intended for HCPs (at any stage of their career).
MEDLINE 인용문 중 20%(595/2973)에 대한 파일럿 검토를 수행하여 상호간 신뢰성이 확립되었다. 중간자 신뢰도 임계값은 0.70의 Cohen θ 값을 가지며, 이는 상당한 일치성을 나타낸다. 임계값이 충족될 때까지 50개의 추가 인용 배치를 검토했다.
A pilot review of 20% (595/2973) of the MEDLINE citations was conducted to establish interrater reliability. The interrater reliability threshold had a Cohen κ value of 0.70, indicating substantial agreement. Additional batches of 50 citations were reviewed until the threshold was met.
3단계: 데이터 수집
Stage 3: Data Collection
표준화된 차트 작성 양식은 기사 세부 정보, 연구 세부 정보(출판이 경험적 연구인 경우), 교육 프로그램 세부 정보 및 구현 요소를 포착하기 위해 개발되었다. 데이터 추출을 위한 도메인의 세분화가 표 1에 요약되어 있습니다.
A standardized charting form was developed to capture the following domains: article details, study details (if publication was an empirical study), education program details, and implementation factors. The subdivisions of the domains for the data extraction are outlined in Table 1.
4단계: 결과 종합 및 보고
Stage 4: Synthesizing and Reporting the Results
본 검토에 포함된 연구를 정리하고 요약하며 보고하기 위해 서술적 합성 접근법이 사용되었다[25]. 여기에는 각 영역(기사 상세, 연구 상세, 교육 프로그램 상세, 구현 요소)을 보고하기 위해 기술 통계를 이용한 수치 요약이 포함되었다. 교육 프로그램 세부사항에 따른 프로그램 커리큘럼의 경우 커리큘럼 주제를 귀납적으로 코딩하였다. 일단 주제 목록이 생성되면 블룸이 공식화한 학습을 위한 분류법을 사용하여 도메인별로 그룹화되었다. 3개의 도메인이 있습니다.
- (1) 인지, 즉 학습자가 가져야 할 지식을 말한다.
- (2) 사이코모터, 학습자가 보여주고 익혀야 하는 기술을 가리킴
- (3) 정동, 학습자가 개발하고 실습에 통합해야 하는 태도를 나타냄
To collate, summarize, and report on the included studies in this review, a narrative synthesis approach was used [25]. This included a numeric summary using descriptive statistics to report each domain (article details, study details, education program details, and implementation factors). For program curriculum under education program details, curriculum topics were inductively coded. Once a list of topics was generated, they were then grouped by domain using the taxonomy for learning formulated by Bloom. There are 3 domains:
- (1) cognitive, which refers to knowledge that learners should have,
- (2) psychomotor, which refers to skills learners should demonstrate and master, and
- (3) affective, which refers to attitudes learners should develop and incorporate into their practice [20].
[20] 연구 결과는 교육 성과의 Kirkpatrick-Barr 프레임워크[21]를 사용하여 연역적으로 코딩되었다. 이 프레임워크는 각 연구에서 보고된 교육적 성과 유형을 분류하는 표준화된 방법을 제공했기 때문에 선택되었다. 구현 요소 하위 도메인은 선험적 코드를 사용하여 2명의 독립 검토자에 의해 주제적으로 분석되었다. 검토자들은 코딩 체계를 비교하고 주요 주제를 반복적으로 결정하여 결과를 프레임화하였다. 콘텐츠 검증을 위해 프로젝트 팀원과 환자, 의료교육 및 AI 분야 전문가가 주제 분석에 대한 피드백을 제공했다.
The study outcomes were deductively coded using the Kirkpatrick-Barr Framework [21] of educational outcomes. This framework was selected because it provided a standardized method of categorizing the type of educational outcomes reported by each study. The implementation factors subdomain was thematically analyzed by 2 independent reviewers using a priori codes. The reviewers compared coding schemes and iteratively determined overarching themes to frame their findings. For content validation, the project team members, patients, and experts in the fields of medical education and AI provided feedback on the thematic analysis.
결과.
Results
검색 결과
Search Results
초기 데이터베이스 검색 결과 13,449개의 결과가 나왔으며, 중복이 제거된 후에는 10,094개(75.05%)의 고유 인용 제목과 요약이 확인되었다. 10,094개의 고유 인용문 중에서 기존 13개 고유 프로그램[32,35,39,43,49,50,59,61-63]이 10개(24%) 기사에 언급된 41개(0.41%) 기사[2,5,13,26-31,34,36-36,40-42,44-48,51,50] 기사에 언급된 나머지 31개(76%) 기사[2,5,35,13,49,50,61-63]는 바람직한 교육내용에 대해 논의하였다. 기사 선택 프로세스는 그림 1에 나와 있습니다. 기존 프로그램에 대해 논의한 10개의 기사 중 8개(80%)는 논평[32,43,49,50,59,61-63]이었고, 1개(10%)는 사례 보고서[39]였으며, 1개(10%)는 실증 연구[35]였다. 표 2와 3은 이 검토에 포함된 기사와 프로그램의 특성을 설명한다.
The initial database search yielded 13,449 results; once duplicates were removed, the titles and abstracts of 10,094 (75.05%) unique citations were identified. From the 10,094 unique citations, we identified 41 (0.41%) articles [2,5,13,26-63], where 13 unique, existing programs [32,35,39,43,49,50,59,61-63] were mentioned in 10 (24%) articles, and the remaining 31 (76%) articles [2,5,13,26-31,33,34,36-38,40-42,44-48,51-58,60] discussed the desired or recommended curricular content. The article selection process is presented in Figure 1. Of the 10 articles that discussed an existing program, 8 (80%) were commentaries [32,43,49,50,59,61-63], 1 (10%) was a case report [39], and 1 (10%) was an empirical study [35]. Tables 2 and 3 describe the characteristics of the articles and programs included in this review.
전달 방식은 무엇이었습니까?
What Was the Mode of Delivery?
개별 프로그램의 요약은 표 4에서 확인할 수 있습니다. 13개 프로그램 중 8개(62%)가 미국[32,35,49,50,59,61-63]에서, 1개(8%)가 캐나다[43]에서, 1개(8%)가 프랑스[59]에서, 1개(8%)가 멕시코[39]에서 나왔다. Strosahl [64]이 기술한 유형학은 교육 방법을 분류하는 데 사용되었다. 13개 프로그램 중 9개 프로그램(69%)은 강의식 접근[32, 35, 43, 43, 43, 43, 43, 50, 50, 59, 59, 59, 59, 59]과 결합된 토론 [62](1/13, 8%), 웹 기반 [39,61](2/13, 15%), 워크숍 및 사례 기반 [50](1/13, 8%), 경험적 학습 [43](1/13, 8%)을 가지고 있었다. 13개 프로그램 중 10개(77%)가 학술적 환경에서 교육되었습니다 [32,35,39,43,59,61,62].
Summaries of the individual programs can be found in Table 4. Of the 13 programs, 8 (62%) originated from the United States [32,35,49,50,59,61-63], 1 (8%) from Canada [43], 1 (8%) from France [59], and 1 (8%) from Mexico [39]. The typology described by Strosahl [64] was used to classify the educational method. Of the 13 programs, 9 (69%) had a didactic approach [32,35,39,43,50,59,61,62] in combination with discussions [62] (1/13, 8%), web-based [39,61] (2/13, 15%), workshop and case-based [50] (1/13, 8%), and experiential learning [43] (1/13, 8%). Of the 13 programs, 10 (77%) were taught in an academic setting [32,35,39,43,59,61,62].
대상 독자
Target Audience
검토된 41개 논문에서 확인된 HCP의 유형은 의사 [41, 43, 46, 52, 59, 63](6/41, 15%)와 간호사 [31, 52](2/41, 5%) 및 방사선 기술자 [5](1/41, 2%)이다. 또한 의료영상[5,26,32-34,37,42,48,50,51,55,58,63] (13/41,32%)과 심장내과[56,61] (2/41,51,31,35,36,38-43,43-47,49-52,57,60,60,26] (2/41,63)의 두 가지 특정 전문성이 확인되었다. 그림 2는 임상의의 학습 연속체에서 다루는 커리큘럼 주제 유형을 보여주고 있으며, 여기에는 학부 의료 교육 [2,13,28-30,33,35-37,39-41,44,47,53,57,61,62](20/41,49%), 대학원 의료 교육 [5,26,32-35,42,42,42,42,42,41,54-58,63,63](19/41,41,41,416%), 전문성 개발 [51,51,2] 등이 포함된다. 5%). 기타 비임상 전문가로는 연구자[5,27,33,59,62](5/41,12%), 보건관리자[27,33,45,52,62](5/41,12%), 컴퓨터 및 데이터 과학자[27,33,52,63](4/41,10%) 등이 있습니다.
There were 3 types of HCPs identified in the 41 reviewed papers: physicians [41,43,46,52,59,63] (6/41, 15%), nurses [31,52] (2/41, 5%), and radiology technologists [5] (1/41, 2%). In addition, 2 specific specialties were identified: medical imaging [5,26,32-34,37,42,48,50,51,55,58,63] (13/41, 32%) and cardiology [56,61] (2/41, 5%), with others not being specified [2,13,27-31,35,36,38-41,43-47,49,52-54,57,59,60,62] (26/41, 63%). Figure 2 illustrates the type of curriculum topics covered in the continuum of learning for clinicians, which includes undergraduate medical education [2,13,28-30,33,35-37,39-41,43,44,47,53,57,61,62] (20/41, 49%), postgraduate medical education [5,26,32-35,41,42,48-51, 54-58,62,63] (19/41, 46%), and continuing professional development [5,57] (2/41, 5%). Other nonclinical professionals include researchers [5,27,33,59,62] (5/41, 12%), health care administrators [27,33,45,52,62] (5/41, 12%), and computer and data scientists [27,33,52,63] (4/41, 10%).
어떤 내용이 다루어졌습니까?
What Content Was Covered?
이러한 논문에서 언급한 프로그램 커리큘럼과 희망 또는 추천 콘텐츠는 맥코이 외 연구진[43]에 의해 프레임화된 AI의 사용, AI 해석 및 결과 설명에 대한 주제를 포함했다. 각 커리큘럼 주제에 대한 설명은 표 5에서 확인할 수 있다. 이들 16개 교육과정 주제 중 인지영역(56%)은 9개, 심리운동영역(68%)은 6개, 정서영역(6%)은 1개였으며, 현 교육프로그램을 논한 논문과 HCP가 무엇을 배워야 하는지에 대한 논평을 통해 대부분 언급되었다. 커리큘럼 주제는 Bloom[20]이 공식화한 학습을 위한 분류법에서 식별된 3가지 영역으로 분류되었다. 표 6은 AI 프로그램이 현재 가르치는 내용을 기술한 논문[32,35,39,43,49,50,59,61-63]의 24%(10/41)에 고유한 커리큘럼 주제를 보여주고 있으며, AI 프로그램이 커리큘럼의 일부로 무엇을 가르쳐야 하는지를 기술한 논문[2,5,13,26-33,34,36-38,40-42,44-48,51-58,60]의 76%(31/41)에 해당하는 커리큘럼 주제를 보여주고 있다. 가르쳐야 할 것과 가르쳐야 할 것.
From these papers, the program curriculum and desired or recommended content mentioned included topics on using AI, interpreting AI, and explaining results from AI, as framed by McCoy et al [43]. A description of each curricular topic can be found in Table 5. Of these 16 curricular topics, 9 (56%) fell under cognitive domain, 6 (38%) under psychomotor domain, and 1 (6%) under affective domain, and most of them were mentioned both by papers that discussed current education programs and commentaries that discussed what HCPs should be learning. The curricular topics were categorized into the 3 domains identified in the taxonomy for learning formulated by Bloom [20]. Table 6 displays the curricular topics
- that were unique to 24% (10/41) of the papers [32,35,39,43,49,50,59,61-63] that described what AI programs currently teach,
- 76% (31/41) of the papers [2,5,13,26-31,33,34,36-38,40-42,44-48,51-58,60] that described what AI programs should teach as part of their curriculum, and
- those that outlined both what was taught and what should be taught.
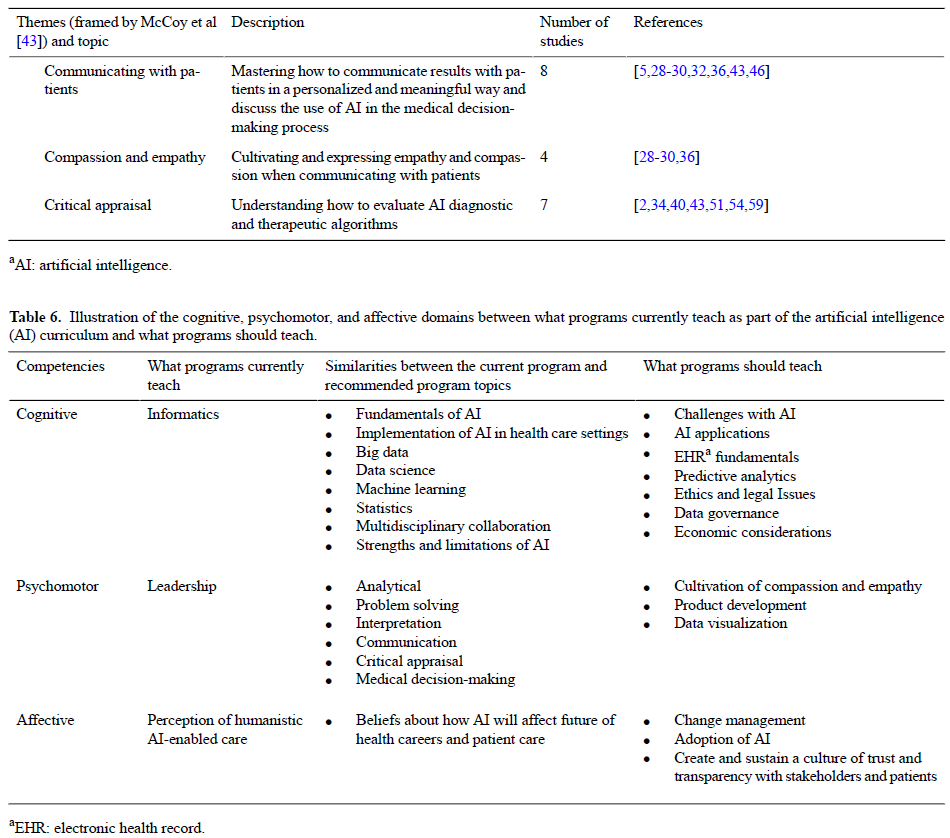
인지 영역
Cognitive Domain
41편의 논문 중 20편(49%), [5,26,32,34,36,37,39-41,43,45-47,50,52,55,57-59,62]은 HCP에게 AI에 대한 기본적 이해를 제공하는 것의 중요성을 강조했고 10편(24%)은 AI 응용을 가르치라고 권고했다. 그 연구는 진단 시스템, 데이터 수집, 평가 및 사용, 임상 응용, 개인 맞춤형 치료 등 AI의 다양한 응용에 초점을 맞췄다. 또한, 많은 논문은 의료 교육과정이 빅데이터와 생물정보학을 포함하되 이에 국한되지 않는 건강관리 데이터 과학의 기초[5,13,26-36,42,45,49,50,52,54](19/41,46%)를 통합해야 한다고 보고했다. 매서니 외 연구진[45]은 데이터 과학 커리큘럼은 AI의 가치를 향상시키고 현재 작동 중인 윤리, 형평성, 다양성 및 포용 원칙과 AI 구현에서 발생할 수 있는 우발적 결과를 인지하기 위해 다학제적 개발팀을 구성하는 방법을 포함해야 한다고 언급했다. 이 연구는 또한 통계, 모델 개발을 포함한 ML, 임상 지식에서의 모델 번역 및 사용, 데이터 추출, 환자의 시각화를 위한 응용 프로그램에 초점을 맞췄다. ML 어휘에 대한 익숙함과 방법론(알고리즘과 기계 수집 및 데이터 프로세스)에 대한 기본적인 이해는 이 급부상하는 분야를 이해하는 데 중요하다고 여겨졌다.
Of the 41 papers, 20 (49%) [5,26,32,34,36,37,39-41,43, 45-47,50,52,55,57-59,62] highlighted the importance of providing HCPs with a baseline understanding of AI and 10 (24%) [32,35,39,43,49,50,59,61-63] recommended teaching them AI applications. The studies focused on various applications of AI, including diagnostic systems, data gathering, assessment and use, clinical applications, and personalized care. In addition, many of the papers reported that medical curricula should integrate fundamentals of health care data science [5,13,26-36,42,45,49,50,52,54] (19/41, 46%), including, but not limited to, big data and bioinformatics. Matheny et al [45] stated that data science curricula should encompass how to form multidisciplinary development teams to improve the value of AI and to be aware of the ethics, equity, diversity, and inclusion principles at play and the inadvertent ramifications that may result from AI implementation. The studies also focused on statistics, ML with model development, model translation and use in clinical knowledge, data extraction, and applications for visualization of patients. Familiarity with ML vocabulary and a basic understanding of the methodology (algorithms and machine gathering and process of data) were deemed important to understand this rapidly emerging field.
사이코모터 도메인
Psychomotor Domain
대부분의 논문은 추세와 효율성 상관관계를 파악하기 위해 [2,5,31,34,35,40,43,46,50,54,55,58,59,61,63](15/41,37%) [임상의가 데이터를 효과적으로 분석할 수 있도록 하는 것]에 초점을 맞추었다. 발타자르 외 연구진[63]과 포니와 맥브라이드[55]가 강조했듯이, AI 애플리케이션의 효율성과 정밀도를 평가하는 방법을 배우는 것이 필수적이다. 이 점은 의대생이 알고리즘의 임상 정확도를 검증할 수 있어야 한다는 박 외 연구진[40]의 검토에서 강화되었다. HCP는 실시간 건강 정보를 수용하는 방법에 익숙해져야 하며, 실천 환경에서 의사 결정을 내릴 수 있다[61]. 41편의 논문 중 8편(20%)은 출처 오류, 편향 또는 임상적 무관성에 대한 인식을 포함하여 합리적인 정확도로 연구 결과를 이해하고 해석하는 것의 중요성을 논의하였다[13,28-31,39,47,50]. 또한, 연구 결과는 문제 해결[35,38,60](3/41,7%)을 중요한 기술로 설명했으며, 여러 개별 자원의 관리와 적용을 수반했다. 임상의는 결과 및 프로세스[5,28-30,32,36,43,46](8/41,20%)를 개인화되고 의미 있는 방식으로 환자와 전달하는 데 능숙해야 한다. 환자와 의사소통할 때 공감과 동정심을 배양하고 표현하는 것[28-30,36](4/41, 10%)이 여러 연구에서 강조되었다.
Most of the papers focused on clinicians being able to effectively analyze the data [2,5,31,34,35,40,43,46,50,54,55,58,59,61,63] (15/41, 37%) to identify trends and efficiency correlations. As highlighted by Balthazar et al [63] and Forney and McBride [55], it is imperative to learn how to evaluate the efficacy and precision of AI applications. This point was reinforced in a review conducted by Park et al [40] that stated medical students should be able to validate the clinical accuracy of algorithms. HCPs will need to become accustomed and understand how to embrace real-time health information to help make decisions in their practice setting [61]. Of the 41 papers, 8 (20%) discussed the significance of understanding and interpreting the findings with a reasonable degree of accuracy, including awareness of source error, bias, or clinical irrelevance [13,28-31,39,47,50]. Moreover, the study findings described problem-solving [35,38,60] (3/41, 7%) as a critical skill, entailing the management and application of several distinct resources. Clinicians will need to become adept in communicating the results and processes [5,28-30,32,36,43,46] (8/41, 20%) with patients in a personalized and meaningful manner. Cultivating and expressing empathy and compassion [28-30,36] (4/41, 10%) when communicating with the patient was emphasized in several studies.
영향 영역
Affective Domain
41편의 논문 중 8편(20%)은 HCP가 환자와 지역사회에 대한 결과를 개선하기 위해 AI 도구를 효과적으로 활용하는 태도를 가져야 한다고 강조했다[27,30,37,52,55,58,59,62]. Wiljer와 Hakim [27]은 초기 단계로서 AI에 대한 대중적 고정관념을 깨는 것의 중요성을 주장했다. 전문가들은 AI가 의료 시스템의 다른 측면을 인수하는 것이 아니라 의료 전달을 강화하는 것으로 인식하는 것이 필수적이다[62]. 포니와 맥브라이드[55]는 임상의가 광범위한 AI 도구와 이러한 도구가 작업 흐름 및 환자 치료에 미치는 영향을 볼 수 있다면 AI를 위협으로 인식할 가능성이 없다고 밝혔다. 또한, Sit 외 연구진[37]은 의대생들이 활용 사례를 제시하고 AI 도구의 경계를 이해할 때 특정 전문 분야를 추구하는 것을 주저하지 않을 가능성이 높다고 언급했다. 응답자의 거의 절반은 [AI 때문에 방사선 전문의와 같은 특정 전문가가 가까운 미래에 쓸모없게 될 것이라는 잘못된 인식]을 믿었다. 또한, Brouillette[59]는 의대생, 컴퓨터 공학대생 및 공대생 간의 협업 프로그램의 필요성을 언급하여 서로의 학문을 더 잘 이해할 수 있도록 하였다. 몇몇 논문은 미래 AI 프로그램이 변경 관리를 통합하고 관련 이해 관계자들과 신뢰와 투명성의 문화를 구축해야 하며, 이는 조직이 의료 생태계 내에서 AI 기술을 보다 신속하게 채택하고 구현할 수 있도록 지원할 것이라고 권고했다[27,30]. 따라서, 조직이 기술의 급속한 발전에 발맞춘 속도로 변화를 관리할 수 있도록 지원하는 것이 중요합니다.
Of the 41 papers, 8 (20%) stressed that HCPs should have the attitude to harness AI tools effectively to improve outcomes for patients and their communities [27,30,37,52,55,58,59,62]. Wiljer and Hakim [27] asserted the importance of breaking the mass stereotypes about AI as an initial step. It is essential that professionals perceive AI as augmenting their delivery of care, rather than taking over different aspects of the health care system [62]. Forney and McBride [55] stated that clinicians are not as likely to perceive AI as a threat if they are able to see the wide array of AI tools and the impact these tools have on workflow and patient care. Furthermore, Sit et al [37] mentioned that medical students are not as likely to be discouraged from pursuing certain specialties when they are presented with use cases and understand the boundaries of AI tools; almost half of the respondents believed the misconception that because of AI, certain specialists such as radiologists will become obsolete in the near future. Moreover, Brouillette [59] mentioned the need for collaborative programs among medical students, computer science students, and engineering students, where they can better understand each other’s disciplines. A few papers recommended that future AI programs should integrate change management and establish a culture of trust and transparency with relevant stakeholders, which will support organizations to more rapidly adopt and implement AI technologies within the health care ecosystem [27,30]. Thus, it is vital to help organizations manage change at a rate in pace with the rapid advancement of technology.
중요한 구현 요인은 무엇이었습니까?
What Were the Critical Implementation Factors?
활성화 요인
Enablers
이러한 프로그램의 성공과 구현에 기여하거나 잠재적으로 기여하는 것으로 확인된 요인에는 단과대학 간 협력 촉진[39,54,57]과 기존 규제 구조 내에서 작업[28,37,39,57]이 포함된다. 모든 기관이 데이터 과학에 대한 경험을 가진 임상 교수진을 보유하고 있는 것은 아니다. 따라서 데이터 과학 교수진과의 협업을 위한 실습과 교육 모두에서 필요하다. 연구에 따르면, [interfaculty collaboration]의 촉진은 교수진 간의 전문성 공유로 설명되었고, 따라서 다학제적인 팀을 구성했다[39,54,57]. 임상 및 비임상 강사의 협업 교육은 향후 HCP를 준비할 때 교육 가치를 높일 수 있으며 또한 교수진에게 데이터 과학 지원을 제공할 수 있다[39,54]. 구현의 또 다른 촉진자는 [기존 규제 구조] 내에서 작업하는 것이다. 커리큘럼 변경은 기존 인증 및 규제 기관의 지원을 필요로 한다[28]. 몇 개의 논문은 의무 AI 과정 작업 및 평가를 현재 커리큘럼과 통합할 필요성을 논의하였다[39,57]. 따라서, 이것은 다양한 AI 리터러시 수준을 다룰 수 있다. AI에 대한 지식을 향상시키면 실제 환경에서 사용될 가능성이 높아질 것이다[37].
The factors identified as contributing, or potentially contributing, to the success and implementation of these programs include promoting interfaculty collaboration [39,54,57] and working within existing regulatory structures [28,37,39,57]. Not all institutions have clinical faculty who also have experience with data science; hence, there is a need in both practice and teaching for collaboration with data science faculty. Promoting interfaculty collaboration was described in the studies as the sharing of expertise among faculty members, thus creating a multidisciplinary team [39,54,57]. Collaborative teaching by clinical and nonclinical instructors may increase the educational value when preparing future HCPs and also provide data science support to faculty [39,54]. Another facilitator to implementation is working within existing regulatory structures. Curriculum changes require the support of existing accreditation and regulatory bodies [28]. A few papers discussed the need for the integration of mandatory AI coursework and assessments with the current curricula [39,57]. Hence, this could address varying AI literacy levels; enhancing knowledge of AI will increase the likelihood that it will be used in practice settings [37].
장벽
Barriers
전반적으로 조직의 구현 노력을 잠재적으로 방해할 수 있는 두 가지 주요 장벽이 확인되었습니다.
- (1) 커리큘럼 설계에서 교수진 간의 다양한 수준의 AI 리터러시[54,57] 및
- (2) AI를 현재 커리큘럼에 통합할 수 있는 인프라의 부족 [34,39,50,54].
교직원과 교육과정 지도자들 사이의 다양한 수준의 [AI 리터러시(literacy)]가 AI 프로그램의 실행을 가로막는 주요 장벽으로 논의되었다. 41편의 논문 중 2편(5%)은 교수진이 AI 기초(예: 빅 데이터 또는 데이터 과학)와 소프트웨어에 대한 지식뿐만 아니라 [가르치는 시간]도 어떻게 제한되는지 논의했다[54,57]. AI 기반 커리큘럼을 설계하기 위한 기술적 전문지식이 부족하다[49,57]. 게다가, 몇몇 연구는 AI를 교육과정에 통합할 수 있는 [인프라의 부족]에 대한 우려를 표명했다. 일부 연구에서는 기존 커리큘럼이 포괄적이고 복잡하며 AI에 대한 [추가 콘텐츠가 강의 부하]를 증가시킬 것이라고 강조했습니다 [34, 50, 54]. 학술 기관은 교직원의 퇴직, AI에 익숙하지 않은 직원, 불충분한 재정 자원과 같은 여러 가지 장애에 직면해 있다[54]. 마지막으로, AI 콘텐츠를 기존 커리큘럼에 통합하는 것은 많은 조직에 장애가 될 수 있다[39].
Overall, 2 major barriers were identified that could potentially impede an organization’s implementation efforts:
- (1) varying levels of AI literacy among faculty in designing curricula [54,57] and
- (2) lack of infrastructure to integrate AI into the current curriculum [34,39,50,54].
Varying levels of AI literacy among faculty and curriculum leaders was discussed as a major barrier that encumbers the implementation of AI programs. Of the 41 papers, 2 (5%) discussed how faculty have limited knowledge of AI fundamentals (eg, big data or data science) and software, as well as limited time to teach [54,57]. There is a lack of technical expertise to design AI-based curricula [49,57]. Moreover, a few studies voiced concerns about the lack of infrastructure to integrate AI into the curriculum. Some studies highlighted that the existing curricula are comprehensive and complex and additional content on AI will increase the course load [34,50,54]. Academic institutions are faced with several encumbrances such as faculty retirement, staff not being well-versed in AI, and inadequate financial resources [54]. Finally, integrating the AI content into existing curricula can be an impediment for many organizations [39].
교육 프로그램의 효과를 평가하기 위해 어떤 조치와 결과가 사용되었는가?
What Measures and Outcomes Were Used to Assess the Effectiveness of Education Programs?
41편의 논문 중 5편(12%)이 훈련 평가 결과를 제시했다[35,39,49,50,62]. 연구마다 교육적 접근법이 다르기 때문에, 각 접근법에 대해 간략하게 논의한 후(표 7) 각 교육 이니셔티브와 관련된 조치와 결과를 도출한다. Kirkpatrick-Barr 프레임워크에 따라 분류된 결과는 레벨 1(즉, 학습자 반응과 교육에 대한 만족도) [39,49,50], 레벨 2a(즉, 태도 변화) [49,50,62] 또는 레벨 2b(즉, 지식 또는 기술의 변화) [35,62]였다. 수준 3 또는 수준 4로 분류할 수 있는 결과가 없었으므로 프로그램 평가는 조직 수준 또는 환자 결과에 대한 행동 또는 영향의 변화에 대해 언급하지 않았다.
Of the 41 papers, 5 (12%) presented the results of their training evaluation [35,39,49,50,62]. As the educational approaches varied across studies, each approach will be briefly discussed (Table 7), followed by the measures and outcomes associated with each educational initiative. Categorized according to the Kirkpatrick-Barr Framework, the outcomes were either level 1 (ie, learner reaction and satisfaction with the education) [39,49,50], level 2a (ie, change in attitude) [49,50,62], or level 2b (ie, change in knowledge or skill) [35,62]. There were no outcomes that could be categorized as level 3 or level 4; thus, the program evaluations did not comment on the change in behavior or affect at the organizational level or on patient outcomes.
논의
Discussion
AI 교육 프로그램 현황
Current State of AI Education Programs
이 리뷰는 HCP를 위한 효과적인 AI 교육 프로그램에 대한 우리의 이해에서 중추적인 지식 격차를 확인했다. 본 검토를 통해 확인된 격차는 HCP가 이용할 수 있는 제한된 AI 교육 및 훈련 기회를 보여주었으며, 따라서 HCP를 대상으로 한 추가 AI 교육 프로그램의 큐레이션 필요성을 강조했다. 기존 프로그램은 [AI의 개발과 구현]에만 집중하는 경향이 있다.
그러나, AI와 협력할 뿐만 아니라 건강과 임상 의사 결정을 위해 AI를 발전시킬 수 있도록 HCP를 준비하는 것도 필수적이다. AI 교육 프로그램은 HCP가 이러한 기술을 안전하게 채택할 수 있을 뿐만 아니라 관련성을 유지하기 위해 practice의 범위를 조정하고 전환할 수 있도록 설계되어야 한다. 의료 분야의 AI 커리큘럼에 대한 중요하고 의미 있는 변화는 [HCP의 AI 리터러시를 높이고 관련 디지털 및 데이터 중심 의사 결정 도구를 활용할 수 있는 능력을 제공해야만] 일어날 것이다. 연구 결과 AI 관련 교육 시책의 성과를 평가하기 위한 노력이 이루어지고 있음을 알 수 있지만, 이러한 성과에 대한 종합적인 평가를 위한 조치에는 일관성이 결여되어 있다. 대부분의 논문은 자체 제작 및 검증되지 않은 도구를 사용했으며, 그 결과를 정성적인 용어로 설명했습니다. 연구에 사용된 다양한 도구를 고려할 때, 표준적이고 포괄적인 도구의 부재는 연구 전반에 걸친 발견의 통합과 합성을 방해한다. 이 검토에서 제공된 지침 원칙은 또한 이러한 프로그램의 향후 개발 및 설계에 도움이 되기를 바란다.
This review identified pivotal knowledge gaps in our understanding of effective AI education programs for HCPs. The gaps identified through this review illustrated the limited AI education and training opportunities available for HCPs and thus emphasized the necessity of curating further AI education programs targeted to HCPs. The existing programs tend to focus only on the development and implementation of AI; yet, it is essential to also prepare HCPs to not only work with AI but also to advance AI for health and clinical decision-making. AI education programs should be designed in a way that enables HCPs to not only safely adopt these technologies, but also to adapt and shift their scope of practice to stay relevant. A significant and meaningful change to AI curricula in health care will only occur by increasing AI literacy among HCPs and by providing them with the ability to leverage relevant digital and data-driven decision-making tools. Although the studies demonstrate that efforts are being made to evaluate the outcomes of AI-related education initiatives, there is a lack of consistency in the measures for a comprehensive assessment of these outcomes. Most of the papers used self-constructed and nonvalidated instruments and delineated their findings in qualitative terms. Given the variety of instruments that have been employed in the studies, the absence of a standard, comprehensive tool impedes the integration and synthesis of findings across the studies. The guiding principles provided in this review will also hopefully inform future development and design of these programs.
중요한 구현 요소
Critical Implementation Factors
AI 콘텐츠를 현재 커리큘럼에 통합하기 위한 인프라의 부족은 이러한 유형의 프로그램 개발을 방해할 수 있다. 설명된 프로그램 중 일부는 콘텐츠 개발을 용이하게 하기 위해 기존 전문 인증 기관의 인프라에 콘텐츠를 내장했다. 특히 캐나다 왕립의과대학은 이러한 규제 전략의 필요성을 더욱 강조하고 있는데, 이는 현재 진행 중이지만 아직 실제로 시행되지는 않고 있다[65,66]. 다학제적 협업의 촉진은 콘텐츠 전달의 원동력으로 지적되었다. 그러나 교수진 간 다양한 수준의 AI 리터러시는 AI 콘텐츠의 성공적인 전달을 방해할 수 있다[54,57]. [커리큘럼 적응과 AI 기술에 대한 인프라 구축]은 환자 치료를 개선하기 위해 AI를 채택하려는 HCP에게 도움이 될 수 있다. 여기에는 AI 교육에 사용할 수 있는 의료 데이터 유형의 개선이 포함된다[67].
A lack of infrastructure to integrate AI content into current curricula could hinder the development of these types of programs; some of the programs described embedded their content within existing professional certifying bodies’ infrastructure to facilitate content development. The Royal College of Physicians and Surgeons of Canada, in particular, further emphasizes the need for these regulatory strategies, which are currently in process but not yet in practice [65,66]. The promotion of multidisciplinary collaboration was indicated as an enabler of content delivery; yet, varying levels of AI literacy among faculty could impede successful delivery of AI content [54,57]. Curricular adaptations and building an infrastructure for AI technologies could be helpful to HCPs wanting to adopt AI to improve patient care; this includes improvements in the types of health care data available for AI education [67].
특히, 생성된 건강 데이터의 대부분은 연구자가 액세스할 수 없고, 기관 윤리 승인 및 데이터 공유 계약을 포함한 규제 또는 인프라 수준의 장벽에 의해 제한된다[67]. 비식별된 데이터, 보안 및 개인 정보 보호 제어의 사용은 잠재적으로 접근 범위를 넓힐 수 있다. 또한 다학제 전문가와의 광범위한 협력은 의료 데이터의 사용과 접근을 개선하기 위한 안전한 데이터 네트워크를 구축하는 데 도움이 될 수 있다[67]. 낮은 AI 리터러시는 [역량 진술을 표준화]하고, [e-러닝에 교수진을 참여]시키고 훈련함으로써 강화될 수 있다[68-70]. 세계보건기구의 디지털 건강에 대한 글로벌 전략은 AI 채택에 대한 장벽을 시스템 수준에서 해결하고 구현의 모든 측면을 고려해야 한다는 것을 추가로 시사한다.
Of note, much of the health data generated are often inaccessible to researchers and limited by regulatory or infrastructure-level barriers, including institutional ethics approvals and data-sharing agreements [67]. The use of deidentified data, security, and privacy controls could potentially widen the scope of access; broader collaboration with multidisciplinary experts could also help to establish secure data networks to improve use and access of health care data [67]. Lower levels of AI literacy could be augmented by standardizing competency statements and engaging and training faculty in e-learning, for instance [68-70]. The World Health Organization’s Global Strategy on Digital Health further suggests that the barriers to AI adoption need to be addressed at the systems level and all aspects of implementation should be considered.
우리의 권고안은 미래의 AI 커리큘럼 개발을 안내하거나 기존 커리큘럼에 AI 교육을 통합하는 데 사용될 수 있는 지침 원칙으로 형성되었다.
Our recommendations have been formed into guiding principles that could be used to guide the development of future AI curricula or to incorporate AI education into existing curricula.
지도 원칙
Guiding Principles
원칙 1: 규제 전략의 필요성
Principle 1: Need for Regulatory Strategies
기존 규제 구조 내에서 일하는 것이 AI 교육 이니셔티브의 이행을 저해할 수 있다는 연구결과가 다수 논의됐다. 교직원은 처음에 국가 보드 시험을 준비하기 위해 개발된 커리큘럼을 변경하는 데 방해가 될 수 있다[28,30]. 또한, 교육 접근 방식은 변화하는 디지털 및 AI 환경에 새로운 기술[29]을 통합하기에는 너무 구식일 수 있다. 새로운 규제 전략이 요구될 것이며, 조직은 이러한 도구를 사용하여 치료를 제공할 수 있는 지식과 기술뿐만 아니라, 신속하게 학습하고 적응할 수 있는 역량을 갖춘 인력을 개발하는 데 우선순위를 두어야 합니다. 이 연구는 또한 [인증 기관이 변화의 장애물이 될 수 있음]을 강조했습니다 [27-29]. Wartman과 Combs[28]는 AI 지원 치료를 위한 미래 의료 제공자를 준비하기 위해 인증기구가 (사실 기억 및 임상 실습을 기반으로 하는) 전통적인 모델을 넘어, 혁신하고 평생 학습에 대한 새로운 접근 방식을 고려할 필요가 있다고 밝혔다.
Many studies discussed that working within the existing regulatory structure can hinder the implementation of AI education initiatives. Faculty can be inhibitors to changing curricula that were initially developed to prepare students for their national board examinations [28,30]. In addition, teaching approaches may be too outdated to incorporate new and emerging technologies [29] into the changing digital and AI landscape. New regulatory strategies will be required, and organizations will have to prioritize developing a workforce that not only has the knowledge and skills to provide care with these tools, but also the competencies to rapidly learn and adapt. The studies also highlighted that accrediting bodies can be a roadblock to change [27-29]. Wartman and Combs [28] stated that to prepare future care providers for AI-enabled care, there is a need for accreditors to move beyond traditional models (based on fact memorization and clinical clerkships) and be willing to innovate and consider new approaches to lifelong learning.
원칙 2: 설계 및 전달에 대한 다원적 접근
Principle 2: Multidisciplinary Approach to Design and Delivery
빠르게 진화하는 현장의 특성과 역동적인 규제, 법률 및 경제 지형은 AI 커리큘럼의 구현을 방해할 수 있으며, 따라서 임상 실습에서 AI 도구의 배치에 영향을 미칠 수 있다. 이러한 영역 중 많은 부분이 여전히 상당한 연구와 발전을 수반하기 때문에 초기 AI 커리큘럼은 반복적으로 개발되어야 하며 [26] 새로운 지식 습득과 정책 변화가 커리큘럼에 반영되도록 해야 한다. 이 발견은 Wiljer와 Hakim에 의해 논문에서 강화되었다[27]. 저자들은 AI 애플리케이션이 아직 복잡성과 임상적 가치 수준으로 발전하지 못한 것은, 이들 애플리케이션 중 상당수가 현재 연구개발 단계에 있기 때문이라고 보고했다.
The rapidly evolving nature of the field and the dynamic regulatory, legal, and economic landscape may hinder the implementation of an AI curriculum and thus affect the deployment of AI tools in clinical practice. An initial AI curriculum must be developed iteratively because many of these areas still entail considerable research and advancement [26], ensuring that new knowledge gains and policy changes are reflected within the curriculum. This finding was reinforced in a paper by Wiljer and Hakim [27]. The authors reported that AI applications have not yet developed to a level of complexity and clinical value because many of these applications are currently in the research and development stages.
Wiens et al [71]은 성공적인 ML 구축은 지식 전문가, 의사 결정자 및 사용자를 포함한 다양한 분야의 전문가와 이해 관계자를 모으는 것을 수반한다고 언급했습니다. 커리큘럼 재설계에 대한 접근 방식은 여러 분야와 훈련 수준에 초점을 맞출 필요가 있다. 커리큘럼은 의료 연구자, 임상의 및 품질 개선 팀과 같은 [다양한 개인의 요구]에 특화되어야 한다[44]. 따라서 AI 기반 커리큘럼의 개발은 커리큘럼의 정확성과 임상적 관련성을 보장하기 위해 보건 시스템 리더, 최전선 제공자, 데이터 과학자, 환자 및 교육 전문가로 구성된 다학제 팀을 포함해야 한다[57,71]. 해당 분야의 모든 이해당사자와 전문가들이 잠재적 편향을 이해하고 해결하기 위해 협력하는 것이 필수적이며, 따라서 기존의 사회적 불평등을 줄이고 궁극적으로 모든 환자를 위한 최적의 치료로 이어진다[71].
Wiens et al [71] stated that successful ML deployment entails assembling experts and stakeholders from various disciplines, including knowledge experts, decision-makers, and users. The approach to curriculum redesign will need to focus on multiple disciplines and levels of training; curricula should be specialized to the needs of various individuals such as health care researchers, clinicians, and quality improvement teams [44]. Therefore, the development of an AI-based curriculum should involve a multidisciplinary team comprising health system leaders, frontline providers, data scientists, patients, and education experts to ensure accuracy and clinical relevance of the curriculum [57,71]. It is imperative for all stakeholders and experts in the field to work collaboratively to understand and address the potential biases, thus reducing the existing social inequalities and ultimately leading to optimal care for all patients [71].
원칙 3: 역량기반 교육과정 설계
Principle 3: Competence-Based Curriculum Design
[미래 practice의 발전]에 영향을 미치려면 [HCP가 기본적인 수준의 AI 역량과 기술을 보유]하는 것이 필수적이다[27]. 교육은 HCP가 임상 practice에서 사용하는 AI와 협력하고 이해하도록 교육하는 방식으로 설계되어야 한다. 또한, AI의 기준 역량 수준은 훈련생이 자신의 업무 범위와 관련된 보건 정책 결정에 상당한 기여를 할 수 있도록 해야 한다[50]. 인공지능은 미래의 의료 행위에 크게 기여할 것이다. 따라서 [AI 도구와 용어의 기본과 적용]은 의대 커리큘럼에 통합되어야 한다. 특히, 이러한 기술의 한계와 윤리적 영향을 고려하면서, 양질의 의료 서비스를 제공하기 위해 이러한 도구를 사용하는 방법에 대해 현재 및 미래의 의사를 교육하는 것이 유용할 것이다[43]. 의학 학습자 및 의사 외에도, 의학 교사는 이러한 혁신적인 AI 커리큘럼 콘텐츠를 제공하기 위해 교육을 받아야 한다. 이는 앞으로의 가파른 학습 곡선을 고려할 때 지체 없이 일어나야 할 변화이다[36]. Paranjape 외 연구진[41]은 학부생에서 지속적인 의학 교육에 이르는 AI와 의료에서의 AI의 적용에 대해 미래 의료 제공자에게 교육하기 위한 단계별 접근 방식을 권고했다.
To influence the development of their future practice, it is essential for HCPs to have a foundational level of AI competencies and skills [27]. Education should be designed in a manner that teaches HCPs to work with, and understand, the AI they use in their clinical practice. Furthermore, a level of baseline competencies in AI should allow trainees to make significant contributions to health policy decisions related to their scope of practice [50]. AI will likely contribute significantly to the medical practice of the future; therefore, fundamentals and applications of AI tools and terminologies should be integrated into medical school curricula. Specifically, training current and future physicians on how to use these tools to provide quality health care, while taking into account the limitations and ethical implications of such technologies, will be useful [43]. In addition to medical learners and physicians, medical teachers need to be trained to deliver this innovative AI curriculum content; this is a shift that needs to occur without delay, given the steep learning curve ahead [36]. Paranjape et al [41] recommended a staged approach to educating future care providers about AI and its application in health care that spans from undergraduate to continuing medical education.
이 검토 결과를 바탕으로 AI 개념의 이상적인 흐름은 옥스퍼드 메디컬이 정의한 의학교육의 3단계에 걸쳐 나눌 수 있다.
학부 의학 교육, 대학원 의학 교육 및 지속적인 전문성 개발(그림 3) [72]
- [학부 의학 교육]은 HCP가 AI 용어, ML 및 데이터 과학의 기초, AI의 역량, 건강 형평 렌즈로 AI가 적합한 건강 분야 기회와 응용 분야를 파악하는 방법에 초점을 맞춰야 한다.
- [졸업후 의학 교육] 동안, 배치deployment뿐만 아니라, 모델의 검증과 사전 평가에 참여하는 방법에 중점을 두어야 한다. 거버넌스 전략 개발을 포함한 윤리적, 법적 고려사항을 더 깊이 탐구해야 한다.
- 마지막으로, [지속적인 전문성 개발] 중에 제공자는 윤리 및 사회적 논의를 촉진하고, AI 과정을 가르치고, 새로운 AI 지식과 기술 및 교수 방법을 따라잡는 데 참여해야 한다.
On the basis of the findings of this review, an ideal flow of AI concepts could be split across the 3 stages of medical education defined by Oxford Medicine: undergraduate medical education, postgraduate medical education, and continuing professional development (Figure 3) [72].
- Undergraduate medical education should be focused on HCPs becoming familiar with AI terminology, the fundamentals of ML and data science, capabilities of AI, and how to identify opportunities and applications in health where AI would be appropriate with a health equity lens.
- During postgraduate medical education, emphasis should be placed on how to engage in validation and prospective evaluation of models, as well as deployment. Ethical and legal considerations, including governance strategy development, should be explored in more depth.
- Finally, during continuing professional development, providers should be involved in facilitating ethical and societal discussions, teaching AI courses, and keeping abreast of new AI knowledge and skills as well as teaching methods.
원칙 4: 환자-임상의 상호 작용
Principle 4: Patient-Clinician Interaction
AI 지원 의료의 시대에 HCP는 [환자와 임상의사 상호작용]의 잠재적 영향뿐만 아니라 기술 지원 환경에서 제공되는 의료 품질을 개선하기 위한 전략을 고려해야 한다[13,27]. Li et al [13]은 보건 전문직 교육은 AI 애플리케이션의 출현에 필수적인 인간에게 고유한 기술인 이타주의와 동정심을 가르치고 육성해야 한다고 말했다. 이것은 HCP가 새로운 도구에 의해 중단되지 않도록 보장할 것이다. 실제로 AI를 사용할 수 있도록 준비하기 위해, 의료 제공자는 의료 제공 시 신뢰할 수 있는 정보와 거짓 정보를 구별할 수 있는 역량을 개발해야 한다[40]. 다른 산업의 상황과 유사하게, 의료 분야에서 AI를 채택하고 구현하는 도전은 승자와 낙후자를 초래할 것이다[48]. AI의 성공적인 채택을 위해서는, HCP는 환자와 협력해야 한다. AI가 의료 환경을 변화시킬 때 이러한 상호 작용은 AI의 기술적 전문 지식을 보완하는 데 중요하기 때문이다[48].
In the age of AI-enabled care, HCPs must consider the potential impact of the patient and clinician interaction as well as the strategies for improving the quality of care delivered in a technology-enabled environment [13,27]. Li et al [13] stated that health professions education should teach and cultivate altruism and compassion, unique skills to humans that are integral to the emergence of AI applications. This will ensure that HCPs are not disrupted by novel tools. To equip themselves to use AI in practice, care providers should develop competencies that allow them to differentiate between credible and false information in their delivery of care [40]. Similar to the situation in other industries, the challenge of adopting and implementing AI in health care will lead to winners and laggards [48]. In the successful adoption of AI, HCPs should engage with their patients because these interactions will be important to complement the technical expertise of AI as AI transforms the health care milieu [48].
제한 사항
Limitations
우리의 범위 검토 결과는 다음과 같은 제한사항의 맥락에서 검토되어야 한다. 범위 검토의 특성 때문에 확인된 각 연구의 품질은 평가되지 않았다. 연구 대상의 성격상 의학 교육이나 지속적인 전문성 개발을 위한 도구로서 AI를 논의한 연구는 제외했다. 오직 영어로 된 연구만이 포함되었다. 또한, 교육적 접근은 연구에 따라 다양하여 어떤 것이 효과적인지 결정하기 위해 커리큘럼 간에 공식적인 비교를 수행할 수 없었다. 그러나 문헌 검토를 통해 현재 교육 프로그램의 차이를 확인하고 향후 교육 노력을 안내할 수 있는 통찰력과 모범 사례를 제공할 수 있었다. 본 검토는 모든 유형의 연구를 포함하고 광범위한 문헌에 초점을 맞추었기 때문에, 교육 프로그램 세부사항에 대한 보고의 깊이는 연구의 범위에 따라 일관성이 없고 다양했다.
Our scoping review findings should be examined in the context of the following limitations. Because of the nature of the scoping review, the quality of each identified study was not assessed. Given the nature of the topic being investigated, we excluded studies that discussed AI as a tool for medical education or continuing professional development. Only studies in English were included. In addition, the educational approaches varied across the studies; thus, we were unable to conduct formal comparisons among the curricula to determine which were effective. However, reviewing the literature enabled us to identify the gaps in current education programs and provide insights and best practices to guide future education efforts. As this review was inclusive of all types of studies and focused on a breadth of literature, the depth in reporting of education program details was inconsistent and varied based on the scope of the study.
결론들
Conclusions
의료 디지털화가 불가피하게 진행됨에 따라 보건 전문직 교육은 이러한 신흥 기술을 보완할 수 있는 인간 고유의 능력을 길러야 한다. 본 검토에서는 보건전문교육의 AI 현황과 향후 의료분야 AI 시대를 위한 의료제공자 준비방향에 대한 개요를 제공하였다. 향후 교육 노력은 규제 전략 개발, 커리큘럼 재설계에 대한 다학제적 접근, 역량 기반 커리큘럼 및 환자-임상의 상호 작용에 초점을 맞춰야 한다.
With the inevitable progression of health care digitization, health professions education should foster unique human abilities, which will complement these emerging technologies. This review provided an overview of the current state of AI in health professions education and future directions on preparing care providers for the era of AI in health care. Future education efforts should focus on the development of regulatory strategies, a multidisciplinary approach to curriculum redesign, a competency-based curriculum, and patient-clinician interaction.
Artificial Intelligence Education Programs for Health Care Professionals: Scoping Review
PMID: 34898458
PMCID: PMC8713099
DOI: 10.2196/31043
Free PMC article
Abstract
Background: As the adoption of artificial intelligence (AI) in health care increases, it will become increasingly crucial to involve health care professionals (HCPs) in developing, validating, and implementing AI-enabled technologies. However, because of a lack of AI literacy, most HCPs are not adequately prepared for this revolution. This is a significant barrier to adopting and implementing AI that will affect patients. In addition, the limited existing AI education programs face barriers to development and implementation at various levels of medical education.
Objective: With a view to informing future AI education programs for HCPs, this scoping review aims to provide an overview of the types of current or past AI education programs that pertains to the programs' curricular content, modes of delivery, critical implementation factors for education delivery, and outcomes used to assess the programs' effectiveness.
Methods: After the creation of a search strategy and keyword searches, a 2-stage screening process was conducted by 2 independent reviewers to determine study eligibility. When consensus was not reached, the conflict was resolved by consulting a third reviewer. This process consisted of a title and abstract scan and a full-text review. The articles were included if they discussed an actual training program or educational intervention, or a potential training program or educational intervention and the desired content to be covered, focused on AI, and were designed or intended for HCPs (at any stage of their career).
Results: Of the 10,094 unique citations scanned, 41 (0.41%) studies relevant to our eligibility criteria were identified. Among the 41 included studies, 10 (24%) described 13 unique programs and 31 (76%) discussed recommended curricular content. The curricular content of the unique programs ranged from AI use, AI interpretation, and cultivating skills to explain results derived from AI algorithms. The curricular topics were categorized into three main domains: cognitive, psychomotor, and affective.
Conclusions: This review provides an overview of the current landscape of AI in medical education and highlights the skills and competencies required by HCPs to effectively use AI in enhancing the quality of care and optimizing patient outcomes. Future education efforts should focus on the development of regulatory strategies, a multidisciplinary approach to curriculum redesign, a competency-based curriculum, and patient-clinician interaction.
Keywords: deep learning; education; health care providers; learning; machine learning; patient care.
©Rebecca Charow, Tharshini Jeyakumar, Sarah Younus, Elham Dolatabadi, Mohammad Salhia, Dalia Al-Mouaswas, Melanie Anderson, Sarmini Balakumar, Megan Clare, Azra Dhalla, Caitlin Gillan, Shabnam Haghzare, Ethan Jackson, Nadim Lalani, Jane Mattson, Wanda Peteanu, Tim Tripp, Jacqueline Waldorf, Spencer Williams, Walter Tavares, David Wiljer. Originally published in JMIR Medical Education (https://mededu.jmir.org), 13.12.2021.
'Articles (Medical Education) > 교육과정 개발&평가' 카테고리의 다른 글
머신러닝: 의학교육의 다음 패러다임 시프트(Acad Med, 2021) (0) | 2022.09.05 |
---|---|
의과대학생이 AI에 대해서 알아야 하는 것은 무엇인가? (J Educ Eval Health Prof. 2019) (0) | 2022.09.01 |
학부의학교육에서 AI: 스코핑 리뷰(Acad Med, 2021) (0) | 2022.09.01 |
When I say … 상황 (Med Educ, 2021) (0) | 2022.08.31 |
의대생들에게 가르치는 것 가르치기: 내러티브 리뷰와 문헌에 기반한 권고(Acad Med, 2022) (0) | 2022.08.24 |