미래의 의사에게 AI 교육시키기: 통합적 리뷰와 변화 제안(J Med Educ Curric Dev. 2021)
Educating Future Physicians in Artificial Intelligence (AI): An Integrative Review and Proposed Changes
Joel Grunhut1 , Adam TM Wyatt1 and Oge Marques2
서론
Introduction
의료 동향
Trends in Healthcare
미국은 2022년까지 의료 지출이 5.4% 증가하여 국내총생산의 9%를 차지할 것으로 예상된다. 1-3 의료의 확대는 의료 관행의 많은 측면에 스며들었다. 의사들에게는 정보 및 정보 기술의 성장을 포함한 의료의 동향에 관한 많은 것들이 있다. 10년 전 1950년 의학 지식의 두 배 시간은 50년이라고 예측되었다. 1980년, 7년; 그리고 2010년에는 3.5년, 그리고 2020년에는 0.2년, 단 73일로 예측되었다. 주어진 비율로, 의과대학에 입학하는 학생들은 첫 해에만 의학 지식이 다섯 배로 증가하게 될 것이다. 이미 정규 의과대학 교육과정 하에서, 적절한 도움 없이 동적으로 변화하는 정보를 축적하는 것은 의학교육과 의료경력에 있어서 전례 없는 도전이 될 것이다.
The United States is projected to see a growth in healthcare expenditure at a rate of 5.4% to reach $10 trillion by 2022 thereby constituting 9% of gross domestic product. The expansion of healthcare has permeated many aspects of medical practice. For physicians, there are many concerning trends in healthcare including the growth of information and information technology. Ten years ago it was predicted that the doubling time of medical knowledge in 1950 was 50 years; in 1980, 7 years; and in 2010, 3.5 years, and in 2020 it was projected to be 0.2 years—just 73 days.4 At the given rate, entering medical students will experience a doubling of medical knowledge 5 times in their first year alone. Already strained under a regular medical school curriculum, accumulating dynamically changing information without proper aid will present an unprecedented challenge in medical education and medical careers.
AI의 부상
Rise of AI
[인공지능(AI)]은 [기계가 인간의 지능을 모방하는 능력]으로 정의된다. AI는 시작부터 일상생활, 비즈니스 등에 스며들었고, 특히 헬스케어에 AI가 점점 더 많이 적용되고 있다. 이 기술은 진단부터 치료 옵션, 벤치워크 연구부터 제약 설계에 이르기까지 제도화된 의료와 민간 의료의 많은 측면에 영향을 미칠 수 있는 잠재력을 가지고 있다. AI는 방사선학에서 이미지 진단 및 이미지 증강과 같은 반복적인 작업을 지원하고 대체하기 위해 의료 분야에서 테스트되고 있다. AI는 자동화된 분류를 통해 의료 작업 흐름을 증가시키고, 개별 의사의 생산성을 향상시키며, 인간의 오류를 줄이고, 더 나은 환자 치료 패턴을 발견하고, 의료 비용을 지불하고, 최소 침습 수술을 수행하고, 사망률을 줄일 것으로 기대된다. 궁극적으로, 많은 확립된 의사 관행, 화학적 합성 계획 및 약물 발견을 다루는 데 있어 AI의 우수한 효율성으로 인해 의사의 역할이 변화하고 있다.
Artificial Intelligence (AI) is defined as the ability of a machine to imitate human intelligence. From its inception, AI has permeated everyday life, business and in particular, AI is increasingly applied to healthcare.7 The technology has the potential to impact many aspects of both institutionalized and private healthcare, from diagnosis to treatment options and from benchwork research to pharmaceutical design. AI is being tested in healthcare to assist and replace repetitive tasks such as image recognition in diagnosis and augmentation of images in radiology. AI is expected to augment healthcare workflow through automated triage, improve the productivity of individual physicians, reduce human errors, discover better patterns of patient care, defray medical costs, perform minimally invasive surgery, and reduce mortality rates.8–14 Ultimately, physicians roles are changing due to the superior efficacy of AI in dealing with many established physician practices, chemical synthesis planning, and drug discovery.
적응의 필요성
The Need to Adapt
과거에는 로봇공학 도입과 마찬가지로 의사 역할도 변화의 비슷한 압박을 받았다.16 이에 대해 의사는 환자 치료를 더욱 발전시키기 위해 자신의 도구에 가장 잘 맞도록 역할을 조정했다. 그러나 AI의 급속한 발전으로 의사들은 새로운 기술 세트 전체를 학습하고 기하급수적으로 증가하는 의료 지식을 관리해야 하는 전례 없는 스트레스에 직면해 있다. 다행히 AI에 대한 학습 과제를 해결하면 의료 지식 확장 문제에 유용한 해결책을 제공할 수 있다. AI는 의사들에게 의료 지식을 효율적으로 처리하고 실제로 번역할 수 있는 대안을 제공할 수 있는 능력을 갖추고 있다.
In the past, as with the introduction of robotics, physicians roles were under similar pressure from change.16 In response, physicians adapted their roles to best match their tools to further advance patient care.17 However, with the rapid advancement of AI, physicians face an unprecedented stress to learn entire new skill sets and to manage an exponential growth of medical knowledge.18 Fortunately, solving the challenge of learning about AI can provide a useful solution to the issue of expanding medical knowledge. AI has the ability to equip physicians with an alternative to efficiently deal with and translate medical knowledge into practice.19–21
교육 혁신
Transforming Education
의료는 진보하고 있으며 의료 교육을 개선할 필요가 있다. 의학 지식의 발견 속도가 증가함에 따라, 의사들은 정보를 관리하고, 구성하고, 유지하는 데 어려움을 겪을 것이다. 의료 전문가들이 이 지식을 의료 실습을 위해 효과적으로 사용할 수 있도록 하기 위해서는 AI와 같은 기술이 필요하다. 의료와 의료 제공이 AI 시대로 접어들면서 임상 의사 결정을 돕기 위한 데이터 사용을 위한 유능한 인간-기계 상호 작용의 필요성이 높아질 것이다. 의대생들은 AI가 어떻게 기능하는지, 비용 개선, 품질 향상 및 의료 접근 용이성에 대한 기본 개념을 충분히 이해할 수 있어야 한다. 마찬가지로, 학생들은 투명성과 책임과 같은 인공지능의 한계에 대해 교육을 받아야 한다. 마지막으로, 예측 가능한 미래에 변형될 기술을 간과하는 것은 의대생들을 불리하게 할 것이다.
Healthcare is advancing and along with it is the necessity to improve medical education. As the rate of discovery of medical knowledge grows, physicians will have trouble managing, organizing, and retaining information. Technologies such as AI are needed to enable healthcare professionals to effectively use this knowledge to practice medicine.22 As medicine and the delivery of healthcare enters the age of AI, the need for competent human–machine interaction for the use of data to aid clinical decision making will rise.23 Medical students need to be sufficiently proficient in understanding the fundamental concepts of how AI functions, its advantages to improve expenses, increase quality, and ease access to healthcare.24,25 Similarly, students must be educated about the shortfalls of AI such as transparency and liability.26–29 Finally, overlooking a technology that will be transformative for the foreseeable future would place medical students at a disadvantage.
목표들
Goals
우리는 커리큘럼 과목으로서 의료 교육에서 AI를 가르치는 역할을 설명하는 이전 연구에 대한 광범위한 문헌 검토를 제공하는 것을 목표로 한다. 여기에는 개념, 도구, 원리, 방법론, 시사점, 위험 및 응용 분야로서의 AI를 가르치는 것이 포함된다. 의대생들은 AI 출력 결과를 사용하고, 해석하고, 설명할 수 있어야 한다. 비록 AI 도구'를 가지고' 의대생들을 가르치는 것이 의미 있고 중요하지만, 그것은 이 논문의 범위를 벗어난다. 이 논문은 또한 의대생들에게 AI에 대해 가르치라는 요구에서 선진적인 실행 경로로 더욱 전환하는 것을 목표로 한다. 본 논문에서는 본 논문에서 주요 관심 주제, 특히 학부 의료 교육(UME)에 AI 콘텐츠 교육을 성공적으로 통합하는 데 필요한 커리큘럼, 문화 및 태도 변화를 다룬 의학교육 분야의 최근 관련 논문을 요약한다.
We aim to provide an extensive literature review of previous studies describing the role of teaching AI in medical education as a curriculum subject. This includes teaching AI as a concept, tools, principles, methodologies, implications, risks, and applications. Medical students should be able to use, interpret, and explain the results of an AI output.30 Although teaching medical students with AI tools is meaningful and important, that is beyond the scope of this paper. This paper also aims to further transition from a call to teach medical students about AI to an advanced path of implementation. In this paper, we summarize recent relevant papers in the field of medical education that have addressed the main topics of interest in this paper, particularly the curricular, cultural, and attitudinal changes required to successfully incorporate the teaching of AI contents in undergraduate medical education (UME).
방법
Method
디자인 및 검색
Design and Search
다른 유형의 연구에서 과거와 현재 문헌을 식별하기 위해 [통합 검토 접근법]이 선택되었다. [통합 검토]는 실험과 비실험을 포함한 연구에 대한 다양한 접근 방식을 결합하는 고유한 접근 방식이다. 통합 검토의 순차적 접근법은 문제 파악, 문헌 검색, 데이터 평가, 데이터 분석 및 제시이다. 확인된 문제는 의학교육에서 AI 구현의 부족을 이해하는 것이었다.
An integrative review approach was chosen to identify the past and current literature from different types of research. Integrative review is a unique approach to combining different approaches to research including experimental and nonexperimental.31 The sequential approach to integrative review is problem identification, literature search, data evaluation, data analysis, and presentation.31 The problem identified was to understand the lack of AI implementation in medical education.
검색 디자인은 모든 저자에 의해 동의되었다. 설계된 전략은 Pubmed, Medline(Ovid), Google Scholar 및 Web of Science를 검색하는 것이었습니다. 검색된 주요 용어는 ("AI" 또는 "인공지능" 또는 "기계학습"), ("의학 교육" 및 ("교육과정" 또는 "학습" 또는 "교육")이었다. Excel에서 선정기준에 대한 결과물 목록을 저장, 검토, 분석하였습니다.
A search design was agreed upon by all authors. The designed strategy was to search Pubmed, Medline (Ovid), GoogleScholar, and Web of Science. Key terms searched were (“AI” or “artificial intelligence” or “machine learning”) AND (“medical education” or “undergraduate medical education”) AND (“curriculum” or “learning” or “teaching”). Resulting article lists were saved, reviewed, and analyzed for selection criteria on Excel.
제외 및 포함 기준
Exclusion and Inclusion Criteria
이 연구는 영어로 출판된 국내외 실험 및 비실험적 연구를 포함했다. 2011년 전자건강기록과 디지털정보가 크게 성장하기 전 기간을 포함하기 위해 2010년부터의 문헌을 선정하였다. [기사 포함 기준]에는 학부 수준에서 의료 교육과정에 AI가 통합될 필요성을 논의하는 연구가 포함되었다. 문헌 검토, 교육과정 개혁에 대한 이전의 추측적 제안을 반복한 연구, 논문, 편지, 의견, 관점, 논평 등을 포함했다. 우리는 적어도 AI에 대한 교육과정 개혁, 의대생과 교직원의 태도, 계획된 교육과정 변화, 시행된 변화 보고서, 그리고 AI에서 학생들을 훈련시키기 위한 기관 수준 프로그램에 대한 추측성 제안을 포함한 기사를 포함했다.
This study included national and international experimental and nonexperimental studies published in English. We selected articles dating from 2010 in order to include the period before the major growth in electronic health records and digital information in 2011.32,33 Article inclusion criteria included studies discussing the need for AI to be incorporated in the medical curriculum either at undergraduate level. We included literature reviews, studies that repeated prior speculative suggestions of curriculum reform, dissertations, letters, opinions, perspectives, and commentaries. We included articles with at least speculative suggestions of curriculum reform, medical student and faculty attitude towards AI, planned out curriculum changes, reports of implemented changes, and institutional level programs to train students in AI.
다만 의과대학에서 [AI 교육의 필요성을 언급하지 않았거나], 의과교육에서의 AI의 실제 진행에 대한 [새로운 교육전략이나 통찰을 제시하지 않은 연구]는 제외했다. 또한, 의료 학습을 강화하기 위한 AI 도구의 통합에 초점을 맞춘 연구는 이것이 우리의 연구의 목적이 아니었기 때문에 제외하였다. 마지막으로 대학원 수준의 의학교육이나 지속 의학교육에 대한 연구는 제외하였다.
However, we excluded research that did not mention the need for education of AI in medical school or provide new educational strategies or insight into the actual progression of AI in medical education. Additionally, we excluded research focusing on incorporation of AI tools to enhance medical learning as this was not the purpose of our study. Finally, we excluded research on graduate level medical education or continued medical education.
데이터 선택
Data Selection
엑셀 기능을 이용하여 중복 연구들을 걸러냈다. 저자들은 관련성을 위해 미리 정해진 포함 및 제외 기준을 바탕으로 각 매치를 살펴보고 원고 제목과 초록을 분석하였다. 저자들 중 두 명은 포함 및 제외 기준에 대한 기사를 독립적으로 심사했다. 그들은 그들의 결과에 대해 토론했고 세 번째 저자를 사용하여 불일치를 해결했다. 그런 다음 동일한 방법으로 개별 기사를 기사 내용에 기반한 포함 기준 정확도에 대해 재분석하였다.
Using Excel functions, duplicate research studies were screened out. The authors went through each match and analyzed manuscript title and abstract based on predetermined inclusion and exclusion criteria for relevance. Two of the authors independently screened the articles for inclusion and exclusion criteria. They discussed their results and resolved any discrepancies using the third author. Individual articles were then reanalyzed for inclusion criteria accuracy based on article content in the same manner.
관련성 평가
Relevance Grading
그런 다음 이 통합 검토를 위한 관련성에 따라 기사를 코드화했다. 관련성은 4점 척도로 채점되었다.
- 1—추측적에 근거한 개혁으로 변화를 요구하는 기사,
- 2—학생 및 교직원 태도 결정
- 3—교육과정 변경에 대한 계획을 수립
- 4—실행된 커리큘럼 변경 또는 제도적 프로그램의 개요.
이것은 전체 원고를 주의 깊게 읽고 가장 적합한 범주를 결정함으로써 결정되었다. 그런 다음 점수는 이전 점수를 받은 값에 이전에 노출되지 않은 다른 저자에 의해 검증되었다. 모든 불일치는 전체적으로 논의되었고 공동으로 수정되었다.
Articles were then coded according to relevance for this integrative review. Relevance was scored on a 4-point scale:
- 1— articles call for change with speculative reform,
- 2—determining student and faculty attitudes,
- 3—thorough plans for a curriculum change,
- 4—reports of implemented curriculum change or institutional programs.
This was determined through careful reading of the full manuscript and determining the best fit category. The scores were then verified by another author who had no previous exposure to the previous values scored. Any discrepancies were discussed holistically and rescored jointly.
결과.
Results
초기 검색 결과 총 729건의 기사가 나왔다. 중복을 제거한 결과 총 588개 논문(표 1)이 나왔다. 메드라인 수색 결과 289개가 일치했다. Pubmed는 251개의 유니크 검색결과를 낳았다. 구글 스콜라 검색은 37개의 새로운 매치를 목표로 하는 그룹을 산출했다. 웹 오브 사이언스 검색 결과 11개의 새로운 일치 항목이 나왔다. 의료교육에서 AI를 언급하지 않은 제목(n = 463)은 제외했다. 저자들은 제목을 기준으로 각 기사의 중요성을 개별적으로 판단한 후 결과를 비교하여 불일치를 찾아냈다. 그런 다음 요약을 검토하고 의료 커리큘럼의 일부로 AI의 초점을 설명하지 못한 기사(n = 86)를 제외했다. 이 시점에서 저자들은 UME(n = 1)에 초점을 맞추지 않은 모든 기사를 제외했다. 이에 따라 총 39개의 기사가 체계적 검토 및 메타분석 흐름도(그림 1)에 표시되었습니다.
The initial searches performed resulted in a total of 729 articles. Removal of duplicates resulted in a total of 588 articles (Table 1). Medline search yielded 289 matches. Pubmed resulted in 251unique matches. Google Scholar search yielded a targeted group of 37 new matches. Web of Science search resulted in 11 new matches. We excluded titles that failed to mention AI in medical education (n = 463). Authors individually determined the significance of each article based on title and then compared results to find discrepancies. We then reviewed abstracts and excluded any articles that failed to describe the focus of AI as part of the medical curriculum (n = 86). At this point, the authors excluded any articles that did not focus on UME (n = 1). This resulted in 39 articles total that is displayed in a Preferred Reporting Items for Systematic Reviews and Meta-Analyses flowchart (Figure 1).
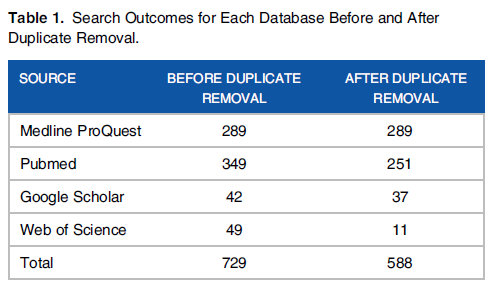
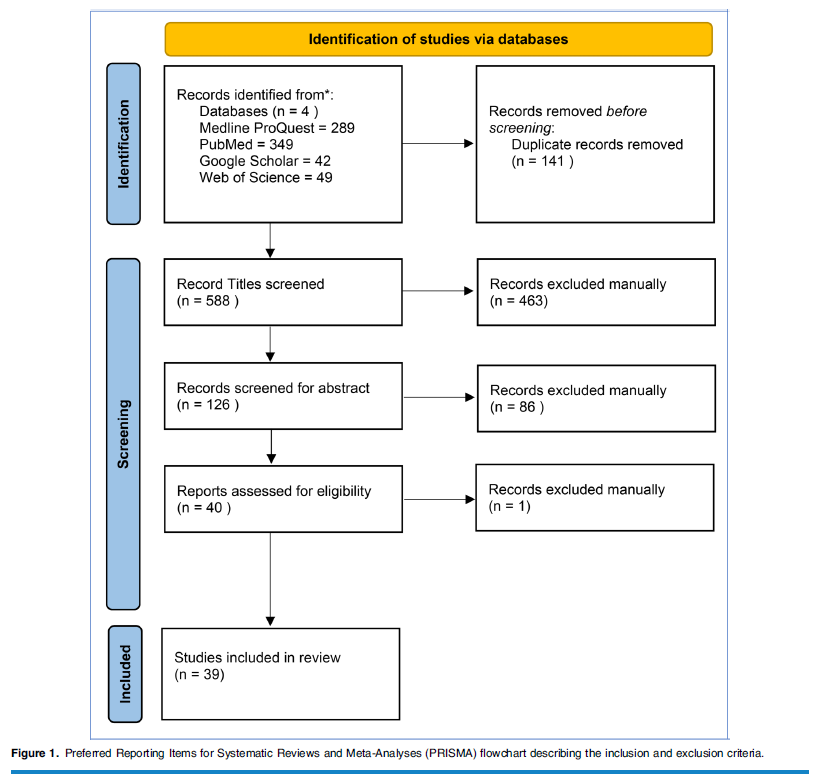
데이터 평가
Data Evaluation
통합 검토를 위한 4점 척도에 기초한 기사의 관련성이 밝혀졌다.
- 추측적 개혁으로 변화를 촉구하는 26개 논문,
- 학생과 교직원의 태도를 결정하는 5개의 논문,
- 커리큘럼 변경에 대한 철저한 계획이 있는 6개 논문 및
- 시행된 교육과정 변경 또는 제도적 프로그램에 대한 보고서 2개(표 2)
마지막 두 기사 모두 의과대학이나 의과대학 전체에 가져온 변화가 아니라 학생 개개인을 위한 시범 프로그램이었다.
The articles’ relevance based on the 4-point scale for integrative review revealed
- 26 articles calling for change with speculative reform,
- 5 articles determining student and faculty attitudes,
- 6 articles with thorough plans for a curriculum change, and
- 2 reports of implemented curriculum change or institutional programs (Table 2).
Both of these articles were not changes brought to an entire medical school or medical class but pilot programs for individual students.
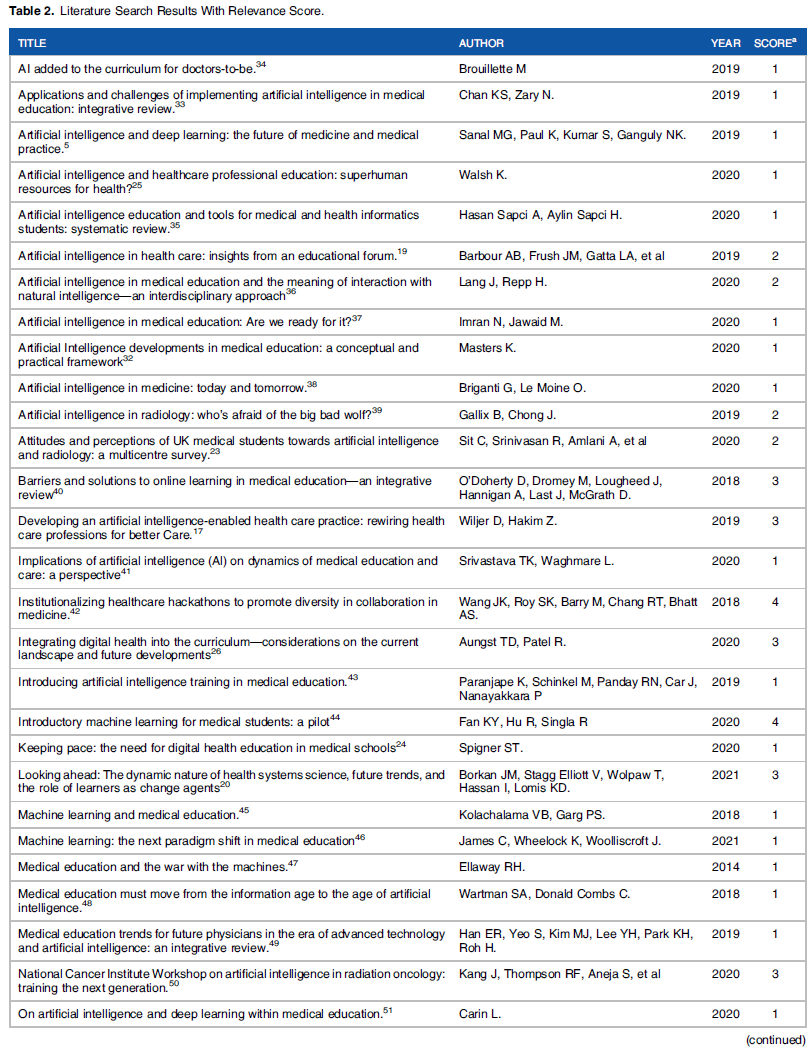

논의
Discussion
우리의 결과는 의사들 사이에서 AI에 대한 현재 지식이 놀랄 만큼 낮고 미래의 의사들에게는 불충분하다는 전반적인 합의를 보여주었다. 이전 문헌들은 의과대학 교육과정에 AI를 통합하는 것의 중요성을 선언했다. AI는 증가하는 경제적 번영, 향상된 교육 기회 및 삶의 질을 통해 미국 전역의 삶을 변화시킬 잠재력을 가지고 있다. 동시에 AI의 잠재적 능력과 복잡성은 AI에 대한 우리의 이해를 더욱 발전시키는 것을 매우 중요하게 만든다. 전 세계의 의학 교육자들은 21세기의 새로운 과제를 해결하기 위해 의학 교육과 교육과 교육과정이 "지식 습득"에서 "지식 관리 및 커뮤니케이션"에 중점을 두는 것으로의 재시동이 필요하다는 견해를 가지고 있다. 게다가, 전문 의료 분야에서 빅 데이터와 AI의 통합이 증가함에 따라, 그 문제에 대한 의사 지식의 격차가 있다. 구체적으로, 우리의 검토의 한 결과는 UME 프로그램의 학생들이 기초 및 임상 의학, 데이터 과학, 생물 통계 및 증거 기반 의학에 대한 충분한 지식을 습득해야 한다고 보고했다. 의대생으로서 AI의 기본 개념에 대한 학습을 통해 환자와 대중을 위해 철저히 검증되고 신뢰할 수 있는 정보를 만드는 법을 배워야 한다.
Our results showed an overall consensus that the current knowledge of AI among physicians is at an alarmingly low level and insufficient for future physicians.55 Previous literature has proclaimed the importance of AI incorporation into the medical school curriculum.37 AI holds the potential to transform lives across the United States through increased economic prosperity, improved educational opportunities, and quality of life. At the same time, the potential capabilities and complexities of AI make it critically important to further advance our understanding of AI.41 Medical educationists throughout the world are of the view that a reboot of medical education and curriculum shift from “Knowledge acquisition” to emphasis on “Knowledge management and communication” is needed to address the emerging challenges of 21st century.39,45 Furthermore, with the increasing integration of big data and AI in professional practice there is a gap in physician knowledge in the matter. Specifically, one result of our review reported that students in an UME program should acquire sufficient knowledge of basic and clinical medicines, data science, biostatistics, and evidence-based medicine.28 As a medical student, one should learn to create thoroughly validated, trustworthy information for patients and the public through learning about the fundamental concepts of AI.28
구현 부족
Lack of Implementation
우리의 결과는 문헌이 의료 교육 과정에서 AI 교육의 중요성에 대한 의견의 합의를 제안하지만 실제 계획 및 구현은 피한다는 것을 보여준다. 교육과정 변화 계획을 언급한 6개의 기사 중, 이러한 계획에 대한 후속 기사의 징후는 없다. 무엇이 의과대학이 이러한 변화를 시행하는 것을 막고 있는지 완전히 이해되지 않는다. 의사들이 AI가 가져올 의료 변화에 대처할 수 있는 장비를 갖추지 못하는 것은 단지 몇 년의 문제일 것이다.
Our results show that the literature suggests a consensus of opinions regarding the importance of AI education in medical curriculum but a void in actual planning and implementation of this.43,46,48 Of the 6 articles mentioning plans for curricular change, there is no indication of published articles following up on these plans.38,44,50,51 It is not fully understood what is preventing medical schools from implementing these changes. It is likely only a matter of a few years before physicians will be ill equipped to handle the change on healthcare AI will bring.
이전 문헌의 결과를 바탕으로 의과대학 교수진의 AI에 대한 지식 부족이 교육과정 중 AI를 통합하는 데 있어 가장 중요한 단일 장애임을 발견했다. 의과대학 교수들은 이 변화를 어떻게 이행해야 할지 전혀 이해하지 못하고 있다. 따라서, 우리는 이러한 변화를 추진하기 위한 선진적인 계획을 제안한다.
Based on our results of the previous literature, we found that the lack of knowledge in AI among medical school faculty is the single most important hindrance in incorporating it among the curriculum.34,47,49,55 Medical school faculty simply have no understanding of how to implement this change.35 Therefore, we propose an advanced initiative to propel this change.
앞으로 나아가는 가장 좋은 방법은 무엇인가?
What is the Best way Forward?
본 검토에서는 의료교육 환경의 변화와 도전을 예측하기 위해 의료교육에서 AI의 구현에 대한 의견과 연구 및 보고한 변화를 통합하였다. 우리의 체계적 검토 결과는 어떠한 제도적 포괄적 연구 및 연구 계획도 수행되지 않았음을 보여준다. 시행된 변화에 대한 2건의 보고 중 학생들이 그러한 변화로부터 무엇을 얻고자 하는지 또는 어떤 방법이 가장 효과적일지에 대한 연구는 이전에 보고된 바 없다. 마찬가지로 의료교육과정 변경을 제안한 6개 조항 중 이러한 제안을 뒷받침하는 근거 기반 방법이 없었다. 본 연구에서 소개된 세부 프로그램들은 의학교육자들이 커리큘럼을 개발하는 데 유용할 수 있다. 이러한 교육동향을 대학원 및 지속적인 의학교육으로 통합하고, 각 의과대학의 혁신적 교육프로그램의 현황이나 효과를 조사하기 위해서는 추가적인 연구가 필요하다.
In order to anticipate changes and challenges in medical education environments, this review integrated the opinions, studies, and reported changes of implementation of AI in medical education. The results of our systematic review show that no institutional comprehensive studied and researched initiatives were conducted. Among the 2 reports of implemented changes, there were no previous reported studies researching what students hope to gain from such changes or what methods would be most effective.36,47 Similarly, among the 6 articles with proposed changes to medical curriculum, there was no evidence-based method backing these proposals.40,54 The detailed programs introduced in this study could be useful for medical educators in the development of curricula. Further research is required to integrate the educational trends into graduate and continuing medical education, and to investigate the status or effects of innovative educational programs in each medical school.
앞으로 의료교육 교육과정에 AI를 접목해 크고 유례없는 변화를 어떻게 수행할지에 대한 종합적인 이해를 모색하는 종단적 연구를 제안한다.
Going forward, we propose a longitudinal study searching for a comprehensive understanding of how to conduct a large and unparalleled change by incorporating AI in the medical education curriculum.
의학교육의 인공지능에 대한 요구
Call for Research of AI in Medical Education
AI에 대한 의료진의 [지식 격차의 노출]에 대한 연구를 진행 중이지만, 이러한 격차를 해소하기 위해 교수직을 재구성할 필요가 있다는 결론을 내릴 가능성이 높다. 이러한 격차는 인공지능 응용 프로그램에 대한 [적극적인 관심은 있지만], 이해, 접근 및 적용을 돕는 [지식 있는 교수진에 대한 노출이 부족한 학습자]를 저해할 수 있다. 2015년 한 출판물은 조사 대상 미국 의대생 중 30%가 임상 정보학에 관심이 있지만 도움을 줄 수 있는 훈련 기회를 식별할 수 없었다고 지적했다. 그러나 이러한 지식 격차는 의료 교육 환경으로의 새로운 교수진 개발을 위한 중요한 기회를 제공하기 때문에 부정적인 방식으로만 특징지어져서는 안 된다. 새로운 영역에서 기술을 가진 새로운 교육자 집단을 고용하는 것은 필요하지만 또한 혁신적이다. AMA 의료 교육 위원회는 기관 지도자와 학장이 데이터 과학자 및 엔지니어와 같은 비임상인을 교수 명단에 포함시키는 것을 적극적으로 가속화해야 한다고 썼다.
The exposure of gaps in medical faculty knowledge of AI is in the process of research but is likely to conclude that there is a need to restructure faculty positions to bridge these gaps; these gaps may be inhibiting learners who have an active interest in AI applications but lack exposure to knowledgeable faculty to help them understand, access, and apply them.42,56 A 2015 publication noted that 30% of US medical students surveyed had interest in clinical informatics, but were not able to identify training opportunities to help.57 These knowledge gaps, however, should not be solely characterized in a negative fashion, as they also present important opportunities for new faculty development into the medical education environment. Employing a new cohort of educators with skills in new areas is necessary but also innovative.58 The AMA Council on Medical Education wrote that institutional leaders and academic deans must proactively accelerate their inclusion of nonclinicians, such as data scientists and engineers, onto their faculty rosters.52
적절한 계획은 의료 교육 연속체에 걸쳐 AI를 연구하기 위한 종단 모델을 통합해야 한다. 이 연구는 의과대학에서 AI를 학습하는 태도와 기대치에 대한 의대생과 교육자를 대상으로 한 [전국 설문조사]에서 시작해야 한다. 이러한 설문조사는 미래의 의사가 무엇을 기대해야 하는지, 그리고 교수진이 이러한 기대를 충족시키기 위해 무엇을 필요로 하는지에 대한 현실적인 목표와 기대를 식별해야 한다. 이러한 조사는 측정 가능하고 해석 가능한 데이터가 있어야 하며, UME에서 AI의 구현을 진전시킬 수 있다. 이러한 목표를 달성하기 위한 논리적인 다중 기관 연구가 필요하다. 그 후, 의학에서 AI의 역할에 대한 다학제적 연구, 협업, 훈련 및 학습은 유사한 급격한 커리큘럼 변화에서 이전에 입증된 방법을 통해 통합되어야 한다.
The proper plan should incorporate a longitudinal model to study AI across the medical education continuum. This study should begin with national surveys of medical students and educators on the attitudes and expectations of learning AI in medical school. These surveys should identify the realistic goals and expectations of what future physicians should expect and what faculty members need to meet these expectations. These surveys should be measurable and have interpretable data to advance the implementation of AI in UME. A concerted multiinstitutional study is a logical approach to achieve these goals. Thereafter, multidisciplinary research, collaboration, training, and learning about the role of AI in medicine should be incorporated through previously proven methods in similar drastic curricular changes.
변화에 대한 장벽
Barriers to Change
의료 교육 분야 AI 추진이 계속 발전하면서 도전과 장벽이 없는 것은 아니다. 변화는 그러한 임상 실습의 진화에 적합하지 않은 교수진과 의사들의 저항에 직면한다. 기계에 의한 최종 의사 교체에 대한 두려움은 이러한 기회를 막을 수 있다. 또한, 인공지능에 대한 오해와 배경 부족은 기계 학습 예측의 신뢰성에 회의감을 불러일으킬 수 있다. 이는 대규모 교육과정 변경을 조직하는 데 있어 정기적인 어려움으로 인해 더욱 복잡해진다. 이러한 변경사항을 가장 잘 통합하는 방법에 대한 추가 연구가 보증됩니다.
As the push for AI in medical education continues to make advancements, it is not without challenges and barriers. Change is met with resistance from faculty and physicians ill equipped for such an evolution of clinical practice.49 Fear of eventual physician replacement by machine may prevent these opportunities. Furthermore, misunderstanding and lack of background in AI can create skepticism in the trustworthiness of a machine learning prediction. This is compounded by the regular difficulties in organizing large curricular change. Further research on how best to incorporate these changes is warranted.
앞으로 이동
Moving Forward
의료 기술과 발전을 따라잡고자 하는 학교는 [의학에서 AI의 역할에 대한 수많은 의사들의 경고]에 주의를 기울여야 한다. 그렇게 하지 않는 것은 미래 세대의 의사들에게 불이익을 줄 것이고, 허겁지겁 기술을 따라잡는 입장에 처하게 만들 것이다.
A school wishing to keep up with the technologies and advances in healthcare should heed the warnings of numerous physicians about the role of AI as a partner in medicine. Not doing so would disadvantage the future generation of physicians and place them at the mercy of catching up to technology.
의사는 AI의 기술적 능력과 한계를 이해하고 이를 실제로 안전한 도구로 적절히 활용할 수 있도록 훈련해야 한다. 의사는 결과를 해석하고 환자에게 권장 사항을 전달할 수 있는 능력이 필요합니다. 게다가, 의사들은 AI가 직장에 가져올 새로운 윤리적 문제에 노출되어야 한다. 인공지능 분야에서 훈련을 받지 않은 의사가 어떻게 컴퓨터 알고리즘이 환자의 높은 사망 확률을 예측하는 것과 같은 윤리적 시나리오를 탐색할 수 있다고 기대할 수 있습니까?
Physicians should be trained to understand the technological capabilities and limitations of AI so that they can properly employ it as a safe tool in practice. Physicians need the ability to interpret the results and communicate a recommendation to the patients. Furthermore, physicians should be exposed to the emerging ethical challenges that AI will bring to the workplace. How can a physician untrained in the field of AI expect to navigate ethical scenarios such as if a computer algorithm predicts a high chance of death for a patient.
의료 분야에서의 AI의 구현은 앞으로 의료 전달 방식을 바꿀 것이다. 의학은 대부분의 다른 분야와 함께 정보기술의 시대로 향하고 있다. [Normalization Process Theory]을 사용하여, 우리는 AI가 개별적이고 집단적으로 사회에 내재될 것이며 궁극적으로 의사의 일상 생활에서 필수가 될 것이라는 것을 인식한다. 그러나 이 과정은 인공지능의 필요성을 요구하는 극단적인 상황에서 가속화될 수 있다.
The implementation of AI in medicine will change the way care is delivered going forward. Medicine is headed along with most other fields, into the age of information technology. Using the Normalization Process Theory, we perceive that AI will become embedded in society both individually and collectively and ultimately will be a necessity in the daily routine of a physician.53 However, this process can be accelerated in extreme circumstances that call upon the need for AI.
코로나 19 기간 동안 의학이 바뀌었고 AI를 비롯한 첨단 기술에 의해 가속도가 붙었다. 우리는 전체 진료와 병원이 원격 의료로 전환하는 것을 보았고, 이것은 고급 의료 기술에서 의사를 훈련시켜야 할 필요성을 예시한다. 전염병이 해결된 후 의학 교육과 의학의 미래는 불확실하지만 AI 중심의 미래는 학습과 의학의 미래를 안내할 파괴적인 변화일 가능성이 높다.
During COVID-19, medicine changed and it was accelerated by AI and other advanced technologies. We saw entire practices and hospitals switch over to telehealth and this exemplifies the need to train doctors in advanced healthcare technology. The future of medical education and medicine is uncertain after the pandemic resolves but an AI-centric future is likely to be the disruptive change that will guide the future of learning and medicine.
환자 대기 시간, 의료 비용 및 불충분한 진료와 같은 의료의 큰 문제 중 일부는 AI가 매우 도움이 될 수 있다. 그러나 인공지능을 잘못 사용하는 비용도 마찬가지로 문제가 될 수 있다. 이러한 [장점과 부채]는 미래의 의사들이 AI의 사용과 함정에 대해 적절하게 훈련되기 시작할 것을 요구한다.
Some of the large problems in healthcare, such as patient wait times, cost of healthcare, and insufficient care may find AI to be extremely helpful.59 But the costs of misusing AI may be equally problematic.60 These advantages and liabilities call for future physicians to begin to be properly trained in AI's uses and pitfalls.
우리의 연구는 제한된 양의 데이터베이스 검색과 제한된 검색 쿼리를 포함하여 한계가 있다. 우리는 누락된 가능성이 있는 연구에 대한 결과를 포함한 연구의 참조를 살펴봄으로써 이를 설명했습니다. 또한, 우리는 제3의 저자의 의견을 사용하고 모든 불일치를 논의함으로써 관련성과 채점 과정의 편향을 제한하려고 했다.
Our study has its limitations including a limited amount of databases searched and limited search queries. We accounted for this by looking through the resulting included studies’ references for possible missed studies. Additionally, we tried to limit bias in the relevance and scoring process by using a third author opinion and discussing all discrepancies.
우리가 검토한 문헌들 중에서 AI가 의학의 미래가 될 것이라는 것에는 의심의 여지가 거의 없다. 오늘날과 내일을 처리할 수 있도록 의사들을 효율적으로 계속 훈련시키기 위해, 국가 및 여러 기관의 계획이 보장된다.
There is little doubt among the literature we reviewed that AI will be the future of medicine. In order to continue to efficiently train physicians to be able to handle today and tomorrow, a national and multiinstitutional initiative is warranted.
J Med Educ Curric Dev. 2021 Sep 6;8:23821205211036836. doi: 10.1177/23821205211036836. eCollection 2021 Jan-Dec.
Educating Future Physicians in Artificial Intelligence (AI): An Integrative Review and Proposed Changes
PMID: 34778562
PMCID: PMC8580487
DOI: 10.1177/23821205211036836
Free PMC article
Abstract
Background: As medicine and the delivery of healthcare enters the age of Artificial Intelligence (AI), the need for competent human-machine interaction to aid clinical decisions will rise. Medical students need to be sufficiently proficient in AI, its advantages to improve healthcare's expenses, quality, and access. Similarly, students must be educated about the shortfalls of AI such as bias, transparency, and liability. Overlooking a technology that will be transformative for the foreseeable future would place medical students at a disadvantage. However, there has been little interest in researching a proper method to implement AI in the medical education curriculum. This study aims to review the current literature that covers the attitudes of medical students towards AI, implementation of AI in the medical curriculum, and describe the need for more research in this area.
Methods: An integrative review was performed to combine data from various research designs and literature. Pubmed, Medline (Ovid), GoogleScholar, and Web of Science articles between 2010 and 2020 were all searched with particular inclusion and exclusion criteria. Full text of the selected articles was analyzed using the Extension of Technology Acceptance Model and the Diffusions of Innovations theory. Data were successively pooled together, recorded, and analyzed quantitatively using a modified Hawkings evaluation form. The Preferred Reporting Items for Systematic Reviews and Meta-Analyses was utilized to help improve reporting.
Results: A total of 39 articles meeting inclusion criteria were identified. Primary assessments of medical students attitudes were identified (n = 5). Plans to implement AI in the curriculum for the purpose of teaching students about AI (n = 6) and articles reporting actual implemented changes (n = 2) were assessed. Finally, 26 articles described the need for more research on this topic or calling for the need of change in medical curriculum to anticipate AI in healthcare.
Conclusions: There are few plans or implementations reported on how to incorporate AI in the medical curriculum. Medical schools must work together to create a longitudinal study and initiative on how to successfully equip medical students with knowledge in AI.
Keywords: artificial intelligence; education; empathy; humans; medical/methods; undergraduate medical.
© The Author(s) 2021.
'Articles (Medical Education) > 교육과정 개발&평가' 카테고리의 다른 글
교육적 퀄리티의 지속적 개선 - 퀄리티 문화 촉진: AMEE Guide No. 147 (Med Teach, 2022) (0) | 2022.09.14 |
---|---|
보건전문직교육을 위한 인공지능(NAM Perspect. 2021) (0) | 2022.09.05 |
머신러닝: 의학교육의 다음 패러다임 시프트(Acad Med, 2021) (0) | 2022.09.05 |
의과대학생이 AI에 대해서 알아야 하는 것은 무엇인가? (J Educ Eval Health Prof. 2019) (0) | 2022.09.01 |
보건의료전문직 교육에서 인공지능: 스코핑 리뷰(JMIR Med Educ. 2021) (0) | 2022.09.01 |