질적연구에서 포화: 개념과 조작화 탐색(Qual Quant, 2018)
Saturation in qualitative research: exploring its conceptualization and operationalization
Benjamin Saunders1 • Julius Sim1 • Tom Kingstone1 • Shula Baker1 • Jackie Waterfield2 • Bernadette Bartlam1 • Heather Burroughs1 • Clare Jinks
도입 Introduction
넓은 의미에서, 포화는 질적 연구에서 데이터 수집 및/또는 분석을 중단하는 기준으로 사용된다. 그것의 기원은 근거이론(글레이저와 스트라우스 1967)에 있지만, 현재 질적 연구에 대한 다양한 접근법에 걸쳐 어떤 형태로든 받아들여지고 있다. 실제로, 포화는 종종 그러한 작업 내에서 필수적인 방법론적 요소로 제안된다. Fusch와 Ness (2015: 페이지 1408)는 '포화에 도달하는 데 실패하는 것은 수행된 연구의 질에 영향을 미친다'고 단정적으로 주장한다. Morse (2015: 페이지 587)는 포화도가 '저자들이 제공하는 질적 엄격성의 가장 빈번하게 주장하는 보증'이라고 언급한다. Guest 등은 포화를 '건강 과학 연구에서 목적적 표본 크기가 결정되는 황금률'가 되었다고 언급한다. 많은 저자들이 질적 연구의 포화를 '규칙'(Denny 2009; Sparkes et al. 2011) 또는 '칙령'(Morse 1995)으로 언급하고 있으며, 질적 방법에 대한 여러 가지 일반적인 퀄리티 기준에 등장한다.
In broad terms, saturation is used in qualitative research as a criterion for discontinuing data collection and/or analysis.Footnote1 Its origins lie in grounded theory (Glaser and Strauss 1967), but in one form or another it now commands acceptance across a range of approaches to qualitative research. Indeed, saturation is often proposed as an essential methodological element within such work. Fusch and Ness (2015: p. 1408) claim categorically that ‘failure to reach saturation has an impact on the quality of the research conducted’;Footnote2 Morse (2015: p. 587) notes that saturation is ‘the most frequently touted guarantee of qualitative rigor offered by authors’; and Guest et al. (2006: p. 60) refer to it as having become ‘the gold standard by which purposive sample sizes are determined in health science research.’ A number of authors refer to saturation as a ‘rule’ (Denny 2009; Sparkes et al. 2011), or an ‘edict’ (Morse 1995), of qualitative research, and it features in a number of generic quality criteria for qualitative methods (Leininger 1994; Morse et al. 2002).
- 1. 일차 연구에 주로 사용되었지만, 포화 원칙은 정성적 합성에도 적용되었다. 그러나 여기서 우리의 초점은 1차 연구에서 사용하는 것에 있다.
1. Although primarily employed in primary research, principles of saturation have also been applied to qualitative synthesis (Garrett et al. 2012; Lipworth et al. 2013). However, our focus here is on its use in primary studies.
- 2. 이 저자들은 포화가 '양적, 질적 또는 혼합된 방법에 관계없이 모든 연구에서 중요하다'는 보다 극단적인 주장을 펼친다(Fusch and Ness 2015: 페이지 1411).
2. These authors proceed to make the more extreme claim that saturation ‘is important in any study, whether quantitative, qualitative, or mixed methods’ (Fusch and Ness 2015: p. 1411).
그러나, 명백하게 정통성의 지위에 도달했음에도 불구하고, 포화상태는 문헌 내에서 다양한 방식으로 정의되거나 때로는 정의되지 않으며, 여러 가지 개념적, 방법론적 문제를 제기한다(Dey 1999; Bowen 2008; O'Reilly and Parker 2013) 본 논문은 문헌의 여러 예를 바탕으로 세 가지 핵심 질문과 관련하여 이러한 문제 중 일부를 탐구하고자 한다.
However, despite having apparently attained something of the status of orthodoxy, saturation is defined within the literature in varying ways—or is sometimes undefined—and raises a number of problematic conceptual and methodological issues (Dey 1999; Bowen 2008; O’Reilly and Parker 2013). Drawing on a number of examples in the literature, this paper seeks to explore some of these issues in relation to three core questions:
- "포화는 무엇인가?"—포화는 어떤 방식으로 정의되는 거죠?
- '어디서 왜?'—어떤 유형의 질적 연구, 그리고 어떤 목적을 위해 포화를 추구해야 하는가?
- '언제 어떻게?—연구의 어떤 단계에서 포화를 추구하며, 그것이 달성되었는지 어떻게 평가할 수 있는가?
- ‘What?’—in what way(s) is saturation defined?
- ‘Where and why?’—in what types of qualitative research, and for what purpose, should saturation be sought?
- ‘When and how?’—at what stage in the research is saturation sought, and how can we assess if it has been achieved?
"포화란 무엇인가?"—포화는 어떤 방식으로 정의되는 거죠?
‘What?’—in what way(s) is saturation defined?
글레이저와 슈트라우스는 그들의 근거이론에 대한 최초의 논문에서 포화를 다음과 같이 정의했다.
In their original treatise on grounded theory, Glaser and Strauss (1967: p. 61) defined saturation in these terms:
[특정 범주와 관련하여 또 다른 그룹의 표본수집을 중단해야 하는 시기를 판단하는 기준]은 범주의 이론적 포화도이다. 포화상태는 사회학자가 범주의 속성을 개발할 수 있는 추가 데이터가 발견되지 않음을 의미한다. 유사한 사례를 반복해서 보면서, 연구자들은 한 범주가 포화 상태라는 것을 경험적으로 확신하게 된다. 연구자는 가능한 한 다양한 데이터를 확장시켜줄 수 있는 그룹을 찾기 위해 노력합니다. 이러한 노력을 통해 '포화'는 해당 범주에서 가능한 가장 광범위한 데이터를 기반으로 한다는 것을 확실하게 해준다.
The criterion for judging when to stop sampling the different groups pertinent to a category is the category’s theoretical saturation. Saturation means that no additional data are being found whereby the sociologist can develop properties of the category. As he sees similar instances over and over again, the researcher becomes empirically confident that a category is saturated. He goes out of his way to look for groups that stretch diversity of data as far as possible, just to make certain that saturation is based on the widest possible range of data on the category.
여기서, [의사결정은 '추가 표본 추출을 할 것인지']와 관련이 있으며, 적절한 표본 추출의 결정 요인은 분석 과정에서 이론적 범주theoretical category의 개발 정도와 관련이 있다. 따라서 포화는 [이론적 표본 추출]의 개념과 밀접한 관련이 있다. 이는 표본 추출이 '새롭게 등장하는emerging 이론에 필요한 유사점 및 대비점'에 의해 유도된다는 생각이다. 포화는 연구자가 '샘플링, 데이터 수집, 데이터 분석을 (선형적으로 이뤄지는 별도의 단계로 취급하기보다는) 결합하게끔' 한다.
Here, the decision to be made relates to further sampling, and the determinant of adequate sampling has to do with the degree of development of a theoretical category in the process of analysis. Saturation is therefore closely related to the notion of theoretical sampling—the idea that sampling is guided by ‘the necessary similarities and contrasts required by the emerging theory’ (Dey 1999: p. 30)—and causes the researcher to ‘combine sampling, data collection and data analysis, rather than treating them as separate stages in a linear process’ (Bryman 2012: p. 18).
Urquhart(2013: 페이지 194)는 근거이론의 관점에서 포화를 다음과 같이 정의한다. '데이터에서 새로운 코드가 발생하지 않는 것을 발견하는 지점을 말한다. 같은 코드를 가진 인스턴스가 있지만 새로운 코드는 없는 것이다' Given은 은 포화 상태를 '추가 데이터가 새로운 Emergent themes를 초래하지 않는' 지점이라고 이야기했다. 다른 사람들도 새로운 코드나 테마의 출현에 대해 비슷한 입장을 취했다. 이러한 정의는 강조의 변화를 보여주며, [두 번째 포화 모델]을 제안합니다. 초점은 [분석 수준]에 머물러 있지만, 결정은 (이미 확인된 코드의 개발 정도보다는) [새로운 코드나 테마의 출현]과 관련이 있는 것으로 보인다. 또한 Urqhart(2013)와 Birks and Mills(2015)는 포화 상태를 [새로운 데이터 수집보다는 분석의 종료]와 주로 연관시킨다.
Also writing from a grounded theory standpoint, Urquhart (2013: p. 194) defines saturation as: ‘the point in coding when you find that no new codes occur in the data. There are mounting instances of the same codes, but no new ones’, whilst Given (2016: p. 135) considers saturation as the point at which ‘additional data do not lead to any new emergent themes’. A similar position regarding the (non)emergence of new codes or themes has been taken by others (e.g. Birks and Mills 2015; Olshansky 2015).Footnote3 These definitions show a change of emphasis, and suggest a second model of saturation. Whilst the focus remains at the level of analysis, the decision to be made appears to relate to the emergence of new codes or themes, rather than the degree of development of those already identified. Moreover, Urqhart (2013) and Birks and Mills (2015) relate saturation primarily to the termination of analysis, rather than to the collection of new data.
- 3. Birks and Mills (2015)는 또한 이론적 포화의 일부로서 '범주는 명확하게 정의되고 차원화된 특성으로 명확하게 표현된다'고 언급하고 있으며, 이는 새로운 테마의 (비) 출현의 사실보다는 포화에 대한 다소 광범위한 관점을 시사한다는 점에 주목해야 한다.
3. It should be noted that Birks and Mills (2015) also state that, as part of theoretical saturation, ‘Categories are clearly articulated with sharply defined and dimensionalized properties’, suggesting a somewhat broader view of saturation, in which the nature of emerging themes is important, rather than just the fact of their (non)emergence.
그러나 Starks and Trinidad (2007: p. 1375)에 따르면, 이론적인 포화는 '이론을 구성하는 전체 범위의 구조가 데이터로 완전히 표현될 때' 발생한다. 완전히 명시적이지는 않지만, 이 정의는 [다른 논리의 방향성을 가진 세 번째 포화 모델]을 제안한다: '데이터를 고려할 때, 우리는 분석적 또는 이론적 적절성을 가지고 있는가?'가 아니라, '이론을 고려할 때, 우리는 그것을 설명하기에 충분한 데이터를 가지고 있는가?'인 것이다.
According to Starks and Trinidad (2007: p. 1375), however, theoretical saturation occurs ‘when the complete range of constructs that make up the theory is fully represented by the data’. Whilst not wholly explicit, this definition suggests a third model of saturation with a different directional logic: not ‘given the data, do we have analytical or theoretical adequacy?’, but ‘given the theory, do we have sufficient data to illustrate it?’Footnote4
- 4. 이것은 슈트라우스의 표본 추출에 대한 접근에 대한 글레이저의 비판을 불러일으킨다. 그는 그것을 이론적인 표본 추출이라기 보다는 관습적인 표본 추출로 간주한다. 슈트라우스에게 발견은 귀납induction와 출현emergence을 의미하는 것이 아니라, 그의 이론을 시험할 수 있도록 데이터에서 찾는 것finding을 의미한다.' (Glaser 1992: 페이지 103).
4. This evokes Glaser’s criticism of Strauss’s approach to sampling, which he regards as conventional, rather than theoretical, sampling: ‘In conventional sampling the analyst questions, guesses and uses experience to go where he thinks he will have the data to test his hypotheses and find the theory that he has preconceived. Discovery to Strauss does not mean induction and emergence, it means finding his theory in data so that it can be tested’ (Glaser 1992: p. 103).
근거이론 문헌 밖으로 이동하면, [네 번째 관점은 이론적 포화theoretical saturation보다는 데이터 포화data saturation를 참조하는 것]이 명백해진다(예: Fusch 및 Ness 2015). 이러한 포화 관점은 새로운 것이 드러나지 않을 때까지 [얼마나 많은 데이터(보통 인터뷰 횟수)가 필요]한가, 또는 Sandelowski(2008: 페이지 875)가 '정보 중복성'(예: 프랜시스 외 2010; Guest et al. 2006)이라고 부르는 것에 초점을 맞춘 것으로 보인다. Grady(1998: 페이지 26)는 [데이터 포화]에 대한 유사한 설명을 제공한다:
If we move outside the grounded theory literature,Footnote5 a fourth perspective becomes apparent in which there are references to data saturation, rather than theoretical saturation (e.g. Fusch and Ness 2015).Footnote6 This view of saturation seems to centre on the question of how much data (usually number of interviews) is needed until nothing new is apparent, or what Sandelowski (2008: p. 875) calls ‘informational redundancy’ (e.g. Francis et al. 2010; Guest et al. 2006). Grady (1998: p. 26) provides a similar description of data saturation as the point at which:
새로운 데이터는 이미 수집된 데이터를 중복하는 경향이 있습니다. 인터뷰에서 연구원이 동일한 의견을 계속해서 듣기 시작하면 데이터 포화 상태에 도달하게 됩니다. 이제 정보 수집을 중단하고 수집된 정보를 분석하기 시작해야 할 때입니다.
New data tend to be redundant of data already collected. In interviews, when the researcher begins to hear the same comments again and again, data saturation is being reached… It is then time to stop collecting information and to start analysing what has been collected.
- 5. Charmaz(2008, 2014)는 근거이론의 맥락을 넘어선 포화 개념의 확장에 비판적이다. 특히 데이터 포화라고 부르는 것으로의 확장에 대해 그렇다.
5. Charmaz (2008, 2014) is critical of the extension of the notion of saturation beyond the context of grounded theory, and in particular of its extension into what we here refer to as data saturation. - 6. 데이터 포화와 이론적 포화를 명시적으로 구분하는 저자는 거의 없다.
6. Few authors draw an explicit distinction between data and theoretical saturation—among the exceptions are Bowen (2008), Sandelowski (2008), O’Reilly and Parker (2013), and Hennink et al. (2017).
다른 몇몇 연구진도 유사한 방식으로 데이터 포화도를 정의한 반면, 레가드 등은 [데이터 포화에 관하여 조금 더 협소하고 개인 지향적인 관점]을 채택하는 것으로 보인다. 즉, 포화는 데이터 세트 전체 수준이 아니라 [개별 참가자가 제공한 데이터]와 관련하여 작동한다. 즉, 포화는 [특정 인터뷰 내의 특정 지점]에서 달성된다.
Whilst several others have defined data saturation in a similar way (e.g. Hill et al. 2014: p. 2; Middlemiss et al. 2015; Jackson et al. 2015), Legard et al. (2003) seem to adopt a narrower, more individual-oriented perspective on data saturation, whereby saturation operates not at the level of the dataset as a whole, but in relation to the data provided by an individual participant; i.e. it is achieved at a particular point within a specific interview:
연구자가 참가자의 관점을 완전히 이해한 포화 상태에 도달했다고 느낄 때까지 조사를 계속할 필요가 있다(Legard 등 2003: 페이지 152).
Probing needs to continue until the researcher feels they have reached saturation, a full understanding of the participant’s perspective (Legard et al. 2003: p. 152).
이러한 관점에서, 데이터에 대한 연구자의 대응(새로운 '정보'의 생성 여부에 대한 의사결정)이 반드시 분석 자체의 일부로 인식되지는 않는다. 따라서 이 모델에서 포화 과정은 [주로 데이터 수집 수준에 위치]하며, 데이터 분석의 전체 프로세스, 즉 이론과 분리된다.
From this perspective, the researcher’s response to the data—through which decisions are made about whether or not any new ‘information’ is being generated—is not necessarily perceived as forming part of the analysis itself. Thus, in this model, the process of saturation is located principally at the level of data collection and is thereby separated from a fuller process of data analysis, and hence from theory.
따라서 네 가지 다른 포화 모델이 존재하는 것으로 보인다(표 1).
1. 첫 번째는 전통적인 근거이론에 뿌리를 두고 있으며, [이론적 표본 추출]의 개념에 따라서, [분석 과정에서의 범주 개발과 새롭게 만들어지는 이론Emerging theory]을 [추가 데이터 수집의 기준]으로 사용한다. 일반적으로 사용되는 용어를 사용하지만, 더 구체적인 정의에 초점을 둔다면, 이 모델은 [이론적인 포화theoretical saturation]로 분류될 수 있다.
2. 두 번째 모델은 유사한 접근방식을 취하지만, 포화도는 [새로운 코드나 테마의 식별]에 초점을 맞추고 있으며, 기존의 [이론적 범주의 완전성]보다는, 그러한 [코드나 테마의 수]를 기반으로 한다. 이것은 [귀납적 주제 포화inductive thematic saturation]이라고 할 수 있다. 이 모델에서 포화도는 [분석 수준에 국한된 것]으로 보이며, 데이터 수집에 대한 의미는 기껏해야 함축적이다.
3. 세 번째 모델에서는 앞선 논리가 반전되는데, 여기서 데이터는 (이론을 발전시키거나 정제refine하기보다는), [낮은 단계의 코드나 테마 수준에서 이론을 예시exemplify]하기 위해 수집된다. 이 모델은 사전 결정된 이론적 범주의 개념을 가리키고 근거 이론의 '귀납적 논리'라는 특성으로부터 우리를 멀어지게 하기 때문에, [선험적 주제 포화a priori thematic saturation]라고 불릴 수 있다.
4. 네 번째 모델은 포화도를 [데이터에서 중복성을 식별하는 문제]로 간주하며, 이러한 데이터에 연결된 이론을 참조할 필요가 없다. 이러한 포화를 일반적으로 사용되고 있는 용어에 맞추어 [데이터 포화data saturation]라고 합니다. 네 번째 모델에서 포화는 공식적인 [데이터 분석과는 구별되는 것]으로 보입니다.
Four different models of saturation seem therefore to exist (Table 1).
The first of these, rooted in traditional grounded theory, uses the development of categories and the emerging theory in the analysis process as the criterion for additional data collection, driven by the notion of theoretical sampling; using a term in common use, but with a more specific definitional focus, this model could thus be labelled as theoretical saturation.
The second model takes a similar approach, but saturation focuses on the identification of new codes or themes, and is based on the number of such codes or themes rather than the completeness of existing theoretical categories. This can be termed inductive thematic saturation. In this model, saturation appears confined to the level of analysis; its implication for data collection is at best implicit.
In the third model, a reversal of the preceding logic is suggested, whereby data is collected so as to exemplify theory, at the level of lower-order codes or themes, rather than to develop or refine theory. This model can be termed a priori thematic saturation, as it points to the idea of pre-determined theoretical categories and leads us away from the inductive logic characteristic of grounded theory.
Finally, the fourth model—which, again aligning with the term already in common use, we will refer to as data saturation—sees saturation as a matter of identifying redundancy in the data, with no necessary reference to the theory linked to these data; saturation appears to be distinct from formal data analysis.
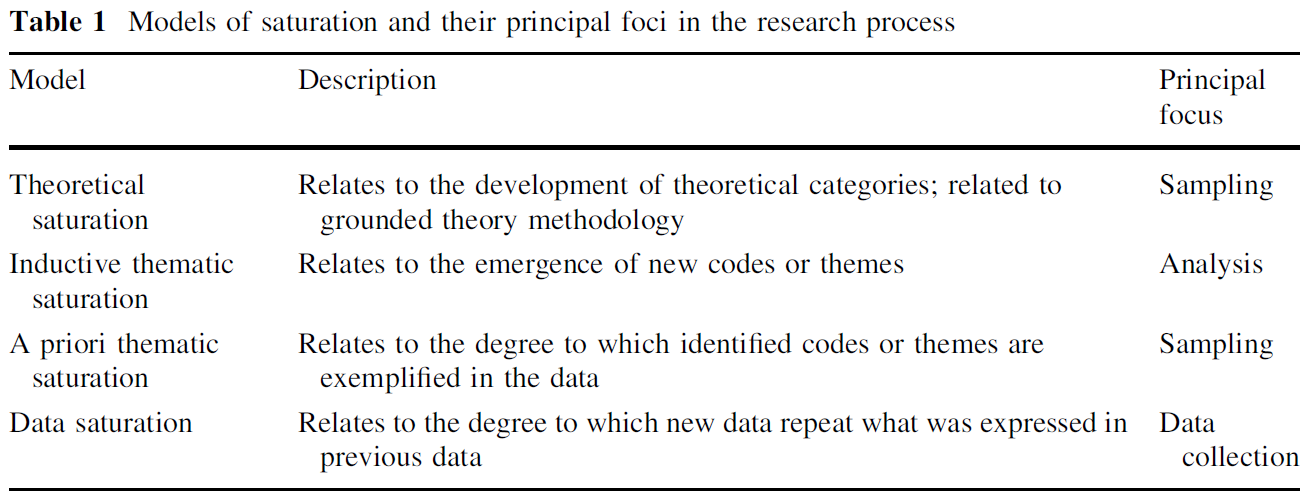
'하이브리드' 형태의 포화
‘Hybrid’ forms of saturation
일부 저자들은 위에서 정의한 [두 개 이상의 모델을 결합]하여 개념화를 덜 구별되게 하는 포화 해석을 지지하는 것으로 보인다. 예를 들어, Goulding(2005)은 데이터와 이론 모두 근거이론 내에서 포화되어야 한다고 제안했고, Drisko(1997: 페이지 192)는 포화를를 '데이터 수집과 분석의 포괄성' 측면에서 정의했다. 비슷하게, 모스의 포화에 대한 관점은 [이론적인 요소와 데이터 포화 요소 모두를 구체화한 것]으로 보인다. 그녀는 데이터 포화 과정을 제안하는 방식으로 포화 상태를 복제 개념과 연결한다.
Some authors appear to espouse interpretations of saturation that combine two or more of the models defined above, making its conceptualization less distinct. For example, Goulding (2005) suggests that both data and theory should be saturated within grounded theory, and Drisko (1997: p. 192) defines saturation in terms of ‘the comprehensiveness of both the data collection and analysis’. Similarly, Morse’s view of saturation seems to embody elements of both theoretical and data saturation. She links saturation with the idea of replication, in a way that suggests a process of data saturation:
그러나 도메인이 완전히 샘플링되면(모든 데이터가 수집되면) 데이터의 복제가 발생하고 이 복제를 통해 포화 신호가 발생합니다(Morse 1995: 페이지 148).
However, when the domain has been fully sampled – when all data have been collected – then replication of data occurs and, with this replication… the signal of saturation (Morse 1995: p. 148).
모스는 자신의 학생들이 '언제 포화 상태에 이르렀는지를 알 수 있다. 바로 더 일반적인 용어로 데이터에 대해 이야기하기 시작하는 때, 그리고 질문을 받았을 때 예시를 쉽게 제공할 수 있는 때이다. 학생들이 자신의 데이터를 '알고' 있는 것이다.' (모스 2015: 페이지 588) 이 역시 [데이터 포화 형태]를 시사한다. 그러나 모스는 또한 '개념의 특성을 파악하고 이론을 발전시키기에는 각 범주에 예시가 너무 적을 때'는 포화가 결여되었다고 제안한다. 이러한 관점은 [데이터 수준]과 [데이터가 이론을 예시하는 방식]에 큰 중점을 두고 있으므로 데이터와 이론적 포화 상태를 모두 환기시키는 것처럼 보이지만, 이론 개발의 개념에 확고히 자리 잡고 있는 것으로 보인다.
Morse notes elsewhere that she is able to tell when her students have achieved saturation, as they begin to talk about the data in more generalized terms and ‘can readily supply examples when asked. These students know their data’ (Morse 2015: p. 588). This too suggests a form of data saturation. However, Morse also proposes that saturation is lacking when ‘there are too few examples in each category to identify the characteristics of concepts, and to develop theory’ (Morse 2015: p. 588). This perspective seems to be located firmly in the idea of theory development (as other parts of the quoted papers by Morse make clear), though a heavy emphasis is placed at the level of the data and the way in which the data exemplify theory, thereby seeming to evoke both data and theoretical saturation.
Hennink 등(2017)은 더 나아가 네 가지 포화 모델의 요소를 모두 결합한 것으로 보인다. 그들은 먼저 '코드 포화'를 식별한다. 이는 [귀납적 주제 포화 요소]와 [데이터 포화] 요소를 결합한 것으로 보인다. 즉, '추가 문제가 식별되지 않고 코드북이 안정화되기 시작하는 지점'이다. 그러나, 이 접근법 내에서 포화는 귀납적으로 개발된 코드뿐만 아니라 세 번째 모델인 [선험적 주제적 포화]를 반영하는 [선험적 코드]와도 관련이 있는 것으로 논의된다. 그들은 또한 '코드 포화'와 '의미 포화'를 구별한다. '의미 포화'에서 분석가는 '[구체적 코드]로부터 [개념적 코드나 개념적 차원]을 완전히 이해하려고 한다'. 코드의 차원을 포화시키는 것에 대한 이러한 초점은 이론적 포화와 더 유사한 것처럼 보인다. 그러나 그들의 분석은 이러한 [코드에서 개발된 이론적 범주]보다는 [코드 수준]에 머무르며, Hennink 등은 그들의 접근방식을 명시적으로 근거이론 방법의 외부에 배치한다.
Hennink et al. (2017) go further, appearing to combine elements of all four models of saturation. They firstly identify ‘code saturation’, the point at which ‘no additional issues are identified and the codebook begins to stabilize’ (2017: p. 4), which seems to combine elements of both inductive thematic saturation and data saturation. However, within this approach saturation is discussed as relating not only to codes developed inductively, but also to a priori codes, which echoes the third model: a priori thematic saturation. They go on to distinguish ‘code saturation’ from ‘meaning saturation’; in the latter, the analyst attempts to ‘fully understand conceptual codes or the conceptual dimensions of… concrete codes’ (2017: p. 14). This focus on saturating the dimensions of codes seems more akin to theoretical saturation; however, their analysis remains at the level of codes, rather than theoretical categories developed from these codes, and Hennink et al. explicitly position their approach outside grounded theory methods.
'어디서 왜?'—어떤 유형의 질적 연구, 그리고 어떤 목적을 위해 포화를 추구해야 하는가?
‘Where and why?’—in what types of qualitative research, and for what purpose, should saturation be sought?
모스(2015: 페이지 587)는 포화가 모든 질적 연구에서 '존재'하고 있으며, 질적 연구에서 표본 크기를 결정하기 위한 '황금률'로 간주되며, 질적 연구의 다른 유형 간 차이는 거의 없다. 우리는 이 관점에 의문을 제기한다. 대신 이전 절에서 고려된 다른 포화 모델에서 제안된 바와 같이, [포화는 이론의 역할에 따라 다른 관련성과 다른 의미]를 가지며, 이러한 관점은 질적 연구방법의 여러 스펙트럼에 걸쳐 그것의 적용에 의문을 제기했던 다른 연구자들도 어느 정도 지지하고 있다. (Walker 2012; O'Reilly and Parker 2013; van Manen et al. 2016)
Morse (2015: p. 587) takes the view that saturation is ‘present in all qualitative research’ and as previously noted, it is commonly considered as the ‘gold standard’ for determining sample size in qualitative research, with little distinction between different types of qualitative research. We question this perspective, and would instead argue—as is suggested by the different models of saturation considered in the previous section—that saturation has differing relevance, and a different meaning, depending on the role of theory, a viewpoint somewhat supported by other commentators who have questioned its application across the spectrum of qualitative methods (Walker 2012; O’Reilly and Parker 2013; van Manen et al. 2016).
연역적 접근법(즉, 데이터에 사전 식별된 코드, 테마 또는 기타 분석 범주를 귀납적으로 나타내기 보다는 전체적으로 또는 주로 적용하는 접근법)에서 포화는 [사전 결정된 코드 또는 테마가 데이터에 적절하게 표현되는 정도]를 나타낼 수 있다. 위에 요약된 [선행 주제 포화 모델]에서 제안된 바와 같이, 범주가 데이터의 인스턴스(instance)로 충분히 채워지는 것을 의미한다. 따라서 포화도에 대한 적절한 표본 크기를 설정하려는 시도에서, 프랜시스 등은 [포화]를 [내용 타당도 개념]과 연결하였다. 대조적으로, 귀납적 접근법(예: 위에서 설명한 귀납적 주제 포화 모델 및 이론적 포화 모델)에서, 포화도는 데이터 내에서 '새로운' 코드나 테마가 식별되는 정도 및 이 과정을 통해 데이터에서 새로운 이론적 통찰력을 얻는 정도를 제시한다.
In a largely deductive approach (i.e. one that relies wholly or predominantly on applying pre-identified codes, themes or other analytical categories to the data, rather than allowing these to emerge inductively) saturation may refer to the extent to which pre-determined codes or themes are adequately represented in the data—rather like the idea of the categories being sufficiently replete with instances, or ‘examples’, of data, as suggested in the a priori thematic saturation model outlined above. Thus, in their attempt to establish an adequate sample size for saturation, Francis et al. (2010) refer explicitly to research in which conceptual categories have been pre-established through existing theory, and it is significant in this respect that they link saturation with the notion of content validity. In contrast, within a more inductive approach (e.g. the inductive thematic saturation and theoretical saturation models outlined above), saturation suggests the extent to which ‘new’ codes or themes are identified within the data, and/or the extent to which new theoretical insights are gained from the data via this process.
연역적 접근법과 귀납적 접근법 모두에서, 분석에 대한 근본적인 접근법은 본질적으로 주제적이며, 대개 다수의 정보 제공자를 포함하는 인터뷰 또는 포커스 그룹 연구의 맥락에서 발생하기 때문에, 각각의 경우에 따라 포화의 역할을 이해할 수 있다. 분석에 대한 전기적 또는 서술적 접근법에 기초하거나 보다 일반적으로 개별 정보 제공자의 설명에 대한 특정 초점을 포함하는 질적 접근법에서 포화 역할을 식별하는 것은 덜 간단하다(예: 해석적 현상학적 분석. 이러한 연구에서 분석은 분석적 주제themes보다는 개별 accounts의 가닥strand에 더 집중하는 경향이 있다. 가닥은 본질적으로 연속적인 반면, 테마는 본질적으로 반복적이다.
In both the deductive and the inductive approach, we can make sense of the role of saturation, however much it differs in each case, because the underlying approach to analysis is essentially thematic, and usually occurs in the context of interview or focus group studies involving a number of informants. It is less straightforward to identify a role for saturation in qualitative approaches that are based on a biographical or narrative approach to analysis, or that, more generally, include a specific focus on accounts of individual informants (e.g. interpretative phenomenological analysis). In such studies, analysis tends to focus more on strands within individual accounts rather than on analytical themes; these strands are essentially continuous, whereas themes are essentially recurrent.
포화가 목적을 명확히 하는 데 도움이 될 수 있는 다양한 유형의 연구를 고려한다.
- 연역적 접근법에서 사용될 때, 포화는 데이터가 이전에 결정된 개념 범주를 instantiate하는 정도를 입증하는 역할을 한다.
- 반면, 귀납적 접근법, 특히 근거이론에서 포화는 이론 개발과 관련하여 표본 추출의 적절성에 대해 무언가를 알려준다.(비록 이것이 얼마나 구체적으로 달성되어야 하는지에 대한 서로 다른 설명들이 있다는 것을 알긴 하지만.)
Considering the various types of research in which saturation might feature helps to clarify the purposes it is intended to fulfil.
- When used in a deductive approach to analysis, saturation serves to demonstrate the extent to which the data instantiate previously determined conceptual categories,
- whereas in more inductive approaches, and grounded theory in particular, it says something about the adequacy of sampling in relation to theory development (although we have seen that there are differing accounts of how specifically this should be achieved).
내러티브 연구에서, 포화를 위한 역할은 구별하기가 더 어렵다. 이론의 충분한 발전이라기보다는 전기적인 서술의 '완전성'을 나타내는 것으로 보일 수 있다. 그러나 참가자의 이야기가 '완전'으로 해석되는 지점(연구의 초점과 관련이 있어 보이는 모든 것을 전달한 때)이 사실 포화 개념에 의해 유용하게 묘사되는 것인지 의문을 가질 수 있다. 이것은 더 나아가 잠재적인 개념화와 용도가 너무 광범위하게 확장되면, [포화의 일관성과 효용성을 잃을 위험이 있는지]에 대한 의문을 갖게 만들 수도 있다.
In narrative research, a role for saturation is harder to discern. Rather than the sufficient development of theory, it might be seen to indicate the ‘completeness’ of a biographical account. However, one could question whether the point at which a participant’s story is interpreted as being ‘complete’—having presumably conveyed everything seen to be relevant to the focus of the study—is, in fact, usefully described by the concept of saturation, given the distance that this moves us away from the operationalization of saturation in broadly thematic approaches. This might, furthermore, lead us to ask whether there is the risk of saturation losing its coherence and utility if its potential conceptualization and uses are stretched too widely.
동일한 이슈가 [포화를 위해 제안된 여러 가지 목적]과도 관련이 있다. 예를 들어 코딩의 신뢰성을 입증한다고 되었지만, 포화는 [코딩 결정의 직접적이고 자동적인 결과]이기 때문에, 어떻게 그것이 품질의 독립적인 척도가 될 수 있는지는 명확하지 않다. Dubé 등은 포화 상태가 (결정적이지는 않더라도) 발견을 추론하는 능력에 대해 무언가를 말해준다고 제안한다. Boddy는 '포화에 도달하면, 그 결과는 어느 정도 일반화될 수 있어야 한다'고 주장한다. Boddy의 주장은 우리를 [분석의 이론적 적절성의 개념과 이론의 설명적 범위]에서 훨씬 더 [경험적인 일반화 가능성]의 감각으로 이동시키는 것처럼 보인다. 이러한 두 가지 경우에 포화도를 사용하면 앞에서 설명한 다른 포화 모델을 고려하더라도 포화의 의미와 목적에 대한 일부 연구에서 혼란의 정도를 나타낼 수 있다. 따라서, 우리는 포화가 개념적으로 의미 있고 실질적으로 유용하기 위해서는 포화가 적용될 수 있는 목적에 어느 정도 제한이 있어야 한다고 제안한다.
The same issue is relevant with regard to a number of other, less obvious, purposes that have been proposed for saturation. For example, it has been claimed to demonstrate the trustworthiness of coding (Damschroder et al. 2007)—but as saturation will be a direct and automatic consequence of one’s coding decisions, it is not clear how it can be an independent measure of their quality. Dubé et al. (2016) suggest that saturation says something about (though not conclusively) the ability to extrapolate findings, and Boddy (2016: p. 428) claims that ‘once saturation is reached, the results must be capable of some degree of generalisation’; this seems to move us away from the notion of the theoretical adequacy of an analysis, and the explanatory scope of a theory, toward a much more empirical sense of generalizability. The use of saturation in these two cases could perhaps indicate a degree of confusion in some studies about the meaning of saturation and its purpose, even when taking into account the differing models of saturation outlined earlier. Therefore, we would suggest that for saturation to be conceptually meaningful and practically useful there should be some limit to the purposes to which it can be applied.
'언제 어떻게?—연구의 어떤 단계에서 포화를 추구하며, 그것이 달성되었는지 어떻게 평가할 수 있는가?
‘When and how?’—at what stage in the research is saturation sought, and how can we assess if it has been achieved?
포화에 대한 관점
Perspectives taken on saturation
주어진 연구 내에서 포화가 의미하는 바를 어떻게 바라보느냐에 따라, 언제 포화를 추구할지가 달라질 것이다. 앞에서 식별된 [네 번째 포화 모델](정보 중복성의 개념에 기초한 데이터 포화 접근방식)을 취하면, 이러한 관점에서 포화는 종종 [공식적인 분석으로부터 분리]되고, [공식적인 분석 이전 단계의 무엇]으로 간주되기 때문에, 프로세스의 초기 단계에서 식별될 수 있는 것이 분명하다. [추가 데이터 수집이 불필요한 시기에 대한 결정]은 일반적으로 인터뷰 내에서 듣고 있는 것에 대한 연구자의 감각에 기초하며, 따라서 이 결정은 코딩과 범주 개발 전에 내려질 수 있다.
The perspective taken on what is meant by saturation within a given study will have implications for when it will be sought. Taking the fourth model of saturation identified earlier—the data saturation approach, as based on the notion of informational redundancy—it is clear that saturation can be identified at an early stage in the process, as from this perspective saturation is often seen as separate from, and preceding, formal analysis. Decisions about when further data collection is unnecessary are commonly based on the researcher’s sense of what they are hearing within interviews, and this decision can therefore be made prior to coding and category development.
그러나 이 단계에서 [무엇이 주제theme를 구성할 수 있는지에 대한 해석]은, 심지어 식별된 주제가 포화 상태인지에 대해 고려하기도 전에 이뤄지는 것으로, 기껏해야 피상적일 것이다. 게다가, 이 단계에서 도달한 결론은 후속 이론 개발과 관련하여 특별히 유익하지 않을 수 있다. 처음 고려되었을 때 매우 유사한 것으로 보이는 데이터 조각이 상세한 분석후에 서로 다른 이론적 구성의 예시로 발견될 수 있고, 반대로, 경험적으로 서로 다른 데이터가 이론적으로 많이 일치하는 것으로 판명될 수 있다. 이 단계의 판단은 [이론적으로 미숙하며, 상당한 수정이 필요할 수 있는] 주제와 범주의 프레임과 관련되어있을 것이다. 예를 들어, 근거이론에서 개방코딩, 선택코딩, 이론코딩의 연속적인 단계 동안 변화들이 발생할 수 있다.
However, interpretations at this stage regarding what might constitute a theme, before even beginning to consider whether identified themes are saturated, will be superficial at best. Moreover, conclusions reached at this stage may not be particularly informative as regards subsequent theory development—pieces of data that appear to be very similar when first considered may be found to exemplify different theoretical constructs on detailed analysis, and correspondingly, data that are empirically dissimilar may turn out to have much in common theoretically. Judgments at this stage will also relate to a framework of themes and categories that is theoretically immature, and that may be subject to considerable modification; for example, the changes that may occur during the successive stages of open, selective and theoretical coding in grounded theory (Glaser 1978).
[두 번째 모델]인 [귀납적 주제 포화]에 관하여, 포화를 판단할 때 분석 수준(즉, 코드 또는 테마가 출현하거나 출현하지 않는 것과 관련)에 더 명시적으로 초점을 맞춘다는 사실은, 포화가 (데이터 포화 접근방식보다) 더 후기 단계에서 달성될 것임을 암시할 수 있다(많은 정성적 접근방식에서 데이터 수집과 분석의 동시적에 진행되긴 하지만). 그렇긴 하지만, [이론적 개발]보다 [코드의 출현]에 초점을 맞추게 되면, 여전히 비교적 이른 단계에서 포화에 달성되게 한다.
With regard to the second model identified, inductive thematic saturation, the fact that the focus is more explicitly on reaching saturation at the level of analysis—i.e. in relation to the (non-)emergence of new codes or themes—might suggest it will be achieved at a later stage than in data saturation approaches (notwithstanding the concurrent nature of data-collection and analysis in many qualitative approaches). However, focusing on the emergence or otherwise of codes rather than on their theoretical development still points us towards saturation being achieved at a relatively early stage.
Hennink 등은 [코드의 포화]가 [코드와 관련된 '차원, 뉘앙스 또는 통찰력'의 포화]보다 더 이른 시점에 달성되었음을 발견했다. 헤닝크 등은 코드의 수에만 의존하는 포화 접근방식은 이러한 코드의 '의미'에 대한 이해 부족으로 인해 '포화 지점을 놓친다'고 주장한다.
Hennink et al. (2017) highlight this in a study on patient retention in HIV care, in which they found that saturation of codes was achieved at an earlier point than saturation of the ‘dimensions, nuances, or insights’ related to codes. Hennink et al. argue that an approach to saturation relying only on the number of codes ‘misses the point of saturation’ (2017: p. 15) owing to a lack of understanding of the ‘meaning’ of these codes.
[데이터 포화(네 번째) 및 [귀납적 주제 포화(두 번째)와는 달리, [첫 번째 포화 모델]인 [이론적 포화]는 분석 과정이 [더 발전된 단계와 더 높은 수준의 이론적 일반성]에 있음을 나타낸다. 첫 번째 모델은 [이론적 범주의 특성이 적절하게 개발된 시점이 언제인가]를 결정하는 근거이론에 기반을 두고 있다. 따라서, 자오와 데이비(2015: 페이지 1178)는 '이론적 완전성'에 의해 결정되는 포화의 형태를 가리키며 '근거 이론의 각 범주의 차원과 간극이 설명되었을 때' 샘플링을 중단했다.
In contrast to data saturation and inductive thematic saturation, the first model of saturation considered, theoretical saturation—as based on the grounded theory notion of determining when the properties of theoretical categories are adequately developed—indicates that the process of analysis is at a more advanced stage and at a higher level of theoretical generality. Accordingly, Zhao and Davey (2015: p. 1178) refer to a form of saturation determined by ‘theoretical completeness’ and ceased sampling ‘when dimensions and gaps of each category of the grounded theory had been explicated,’ .
사건 또는 프로세스로서의 포화
Saturation as event or process
포화를 식별하는 데 기초가 되는 핵심 이슈는 [사건이나 과정으로 보는 범위가 어디까지인가]이다. 일반적으로 포화는 '점point'이라고 부르며, 이는 분석가가 인지할 수 있는 이산적 사건으로 간주해야 함을 시사한다. 그러나
- Strauss와 Corbin은 [포화상태에 대해 '정도의 문제']로서 언급하며, '새로운 것'이 항상 나타날 가능성이 있다고 주장한다. 그들은 포화 상태가 추가적인 데이터 수집이 '반생산적'이 되는지 여부를 고려해야 하며, '반생산적'이란 '새로운 것'이 전체 이야기나 이론에 어떤 것도 추가하지 않는 지점에 도달하는 것이다.
- Mason(2010)은 [추가 데이터 수집으로 인한 '수익성의 저하diminishing returns'가 발생하는 지점]에 대해 유사한 주장을 하며, 많은 연구자들은 포화에 대해 이러한 점진적 접근법을 취하는 것으로 보인다.
- 넬슨(2016)은 Dey (1999)의 초기 관점을 반영하며, '포화'라는 용어는 문제가 있는데, 왜냐하면 직관적으로 고정된 지점과 '완전성completeness'의 관점에서 사고하게끔 만들기 때문이다. 따라서 그는 적어도 근거이론의 관점에서는, '개념의 깊이conceptual depth'가 더 적절한 용어일 수 있다고 주장한다. 이러한 관점에서 포화를 판단할 때 연구자는 [새로운 이론적 범주와 관련하여 충분한 이해 깊이가 달성되었는지 여부]를 고려한다.
A key issue underlying the identification of saturation is the extent to which it is viewed as an event or a process. Commonly, saturation is referred to as a ‘point’ (e.g. Otmar et al. 2011; Jassim and Whitford 2014; Kazley et al. 2015), suggesting that it should be thought of as a discrete event that can be recognized as such by the analyst.
- Strauss and Corbin (1998: p. 136), however, talk about saturation as a ‘matter of degree’, arguing that there will always be the potential for ‘the “new” to emerge’. They suggest that saturation should be more concerned with reaching the point where further data collection becomes ‘counter-productive’, and where the ‘new’ does not necessarily add anything to the overall story or theory.
- Mason (2010) makes a similar argument, talking of the point at which there are ‘diminishing returns’ from further data-collection, and a number of researchers seem to take this more incremental approach to saturation.
- Nelson (2016), echoing Dey’s (1999) earlier view, argues that the term ‘saturation’ is itself problematic, as it intuitively lends itself to thinking in terms of a fixed point and a sense of ‘completeness’. He thus argues that ‘conceptual depth’ may be a more appropriate term—at least from a grounded theory perspective—whereby the researcher considers whether sufficient depth of understanding has been achieved in relation to emergent theoretical categories.
포화에 대한 이러한 점증적 읽기에서, 분석결과는 한 번의 추가 인터뷰 후에 [어느 순간 갑자기 '풍부rich'해지거나 '통찰적insightful'이 되는 것]이 아니라, 아마도 [점진적으로 더 풍부해지거나richer 더 통찰력more insightful이 있게] 된다. 그렇다면 질문은 '포화가 얼마나 일어났는가'가 아니라 ['지금의 포화가 얼마나 충분한가']가 될 것이다. 이것은 덜 간단한 질문이지만, 이것이 분석가의 결정의 문제일 수 있다는 사실을 더 잘 강조하는 질문이다. 즉, 포화상태는 특정 시점에서 정확히 지적할 수 있는 것이 아니라, [진행 중인 누적적 판단]이며 아마도 결코 완료되지 않을 것이다.
On this incremental reading of saturation, the analysis does not suddenly become ‘rich’ or ‘insightful’ after that one additional interview, but presumably becomes richer or more insightful. The question will then be ‘how much saturation is enough?’, rather than ‘has saturation occurred?’Footnote7 This is a less straightforward question, but one that much better highlights the fact that this can only be a matter of the analyst’s decision—saturation is an ongoing, cumulative judgment that one makes, and perhaps never completes,Footnote8 rather than something that can be pinpointed at a specific juncture.
- 7. 따라서 Dey(1999: 페이지 117)는 '포화' 대신에 '충족'이라는 용어를 제안한다.
7. Hence, Dey (1999: p. 117) suggests the term ‘sufficiency’ in preference to ‘saturation’. - 8. 이것은 이론 생성에 대한 글레이저와 스트라우스의 관점을 반영한다: '누군가는 그의 이론을 변화시키고 발전시키는 데 도움이 될 새로운 관점에 지속적으로 주의를 기울인다. 이러한 관점은 연구 마지막 날이나 심지어 원고를 페이지 교정본으로 검토할 때에도 쉽게 나타날 수 있기 때문에 출판된 단어는 최종적인 것이 아니라 이론 생성 과정의 끝없는 중단일 뿐이다.'
8. This reflects Glaser and Strauss’s (1967: p. 40) view of theory generation: ‘one is constantly alert to emergent perspectives that will change and help develop his theory. These perspectives can easily occur even on the final day of study or even when the manuscript is reviewed in page proof; so the published word is not the final one, but only a pause in the never-ending process of generating theory’.
불확실성과 애매모호
Uncertainty and equivocation
포화가 달성되는 특정 시점을 식별하려는 욕구는 종종 불확실성이나 애매모호함을 야기하는 것으로 보인다. 많은 연구에서 포화에 달성했다고 언급하지만, 추가적인 데이터 수집은 이러한 주장을 'confirm' 또는 '유효성 확인validate'하기 위한 명백한 시도로 이루어진다.
A desire to identify a specific point in time at which saturation is achieved seems often to give rise to a degree of uncertainty or equivocation. In a number of studies, saturation is claimed, but further data collection takes place in an apparent attempt to ‘confirm’ (Jassim and Whitford 2014: p. 191; Forsberg et al. 2000: p. 328) or ‘validate’ (Vandecasteele et al. 2015: p. 2789) this claim; for example:
10차 면접이 끝난 후, 면접을 통해 창출된 새로운 테마는 없었다. 따라서, 데이터 수집이 포화 상태에 도달했다고 간주되었다. 새로운 테마가 등장하지 않음을 확인하고 확인하기 위해 두 번의 인터뷰를 위해 데이터 수집을 계속했습니다.
After the 10th interview, there were no new themes generated from the interviews. Therefore, it was deemed that the data collection had reached a saturation point. We continued data collection for two more interviews to ensure and confirm that there are no new themes emerging (Jassim and Whitford (2014: pp. 190–191).
또한, 포화 증거에 대한 의존을 꺼리는 것은, 포화가 기껏해야 불분명하거나, 최악의 경우 일관성이 없는 방식으로 사용되고 있음을 나타낸다. 예를 들어, Hill 등(2014: 페이지 2)은 포화 원리를 지지하면서도 이를 완전히 신뢰하지는 않는 것으로 보인다.
Furthermore, a reluctance to rely on evidence of saturation sometimes indicates that saturation is being used in at best an unclear, or at worst an inconsistent or incoherent, fashion. For example, Hill et al. (2014: p. 2), whilst espousing the principle of saturation, seem not fully to trust it:
포화는 채용 기간 내내 지속적으로 모니터링되었습니다. 완성도를 위해 테마를 놓칠 가능성을 줄이기 위해 모든 참가자 그룹 전체 모집을 선택했습니다.
Saturation was monitored continuously throughout recruitment. For completeness we chose to fully recruit to all participant groups to reduce the chance of missed themes.
잭슨 외 연구진도 비슷합니다. (2000: 페이지 1406)는 포화가 확립되었다고 주장하지만, 그리고나서는 다소 후퇴하는 것으로 보인다.
Similarly, Jackson et al. (2000: p. 1406) claim that saturation had been established, but then appear to retreat somewhat from this conclusion:
8개 데이터 세트를 분석한 후 데이터 포화도를 설정했지만 데이터 포화도를 확보하기 위해 2명의 추가 참가자를 모집했다.
Following analysis of eight sets of data, data saturation was established… however, two additional participants were recruited to ensure data saturation was achieved.
콘스탄티누 외 연구진(2017)은 포화 지점에 대한 불확실성의 가능성을 고려할 때, 이것이 발생한 지점에 대한 우려보다는, [포화 지점에 도달했다는 증거를 제공]하는 데 더 집중해야 한다고 제안한다. 따라서 그들은 '최초 표본 추출의 모든 인터뷰를 포함해도 나쁠 것이 없다'고 제안한다. 그러나 이러한 관점은 본질적으로 문제가 있다. 왜냐하면 포화도가 [지속적인 샘플링 결정을 안내하는 것]이 아니라 [데이터 수집의 완료 후의 후향적 결정]이라는 것을 의미하기 때문이다. 하지만, 모든 데이터가 추가 통찰력에 기여하는지 또는 개념 이해에 추가하는지 여부에 관계없이, [(무조건) 모든 데이터가 포함]된다면, 포화가 관련성relevance을 잃게 된다고 주장할 수도 있다. 이 접근방식은 [표본의 적절성을 판단하는 데 포화가 도움이 되는지]보다는, 충분한 데이터를 가지고 포화의 증거를 보여주는 것(즉, 너무 적은 인터뷰는 안 됨)에 대한 선입견을 나타내는 듯 하다.
Constantinou et al. (2017) propose that, given the potential for uncertainty about the point at which saturation is reached, attention should focus more on providing evidence that saturation has been reached, than on concerns about the point at which this occurred. Thus, rather curiously, they propose that it ‘does not hurt to include all interviews from the initial sampling’ (2017: p. 13). This view is inherently problematic, however, as not only does it imply that saturation is a retrospective consideration following the completion of data collection, rather than as guiding ongoing sampling decisions, but one could also argue that saturation loses its relevance if all data are included regardless of whether or not they contribute further insights or add to conceptual understanding. This approach appears to indicate a preoccupation with having enough data to show evidence of saturation, i.e. not too few interviews, rather than saturation aiding decisions about the adequacy of the sample.
위의 내용은 [포화가 달성된 지점]의 평가에 양면성이 있음을 시사하는 반면, 다른 보고서에서는 의도적으로 포화 상태를 넘어 샘플링을 계속하기로 결정을 내렸다. 이는 표본 추출 결정을 강화하기 위한 추가적인 객관적 증거를 찾는 것으로 보인다. 그러한 시도를 한 연구자들은, 정확히 무엇인지에 대해 명확히 설명하지는 않지만, [포화에 의해 포착되지 않는 이론적으로 중요한 어떤 것이 있다]는 견해를 시사한다.
Whilst the above suggests ambivalence towards assessing the point at which saturation is achieved, others report having made the conscious decision to continue sampling beyond saturation, appearing to seek additional objective evidence to bolster their sampling decisions. These examples suggest a view that there is something of theoretical importance that is not captured by saturation, though it is unclear from the explanations given as to exactly what this is.Footnote9
- 9. 때때로, 포화를 넘어서는 이유는 방법론적인 것이라기보다는 윤리적인 것으로 보인다. 포화상태에 이르렀음에도 불구하고, 프랑스 등은. (2008: 페이지 22)에 따르면 '연구에 참여하고자 하는 모든 여성에 대한 헌신과 존중'으로 인해, 데이터 수집은 모든 사람이 인터뷰를 마칠 때까지 끝나지 않았다. 비슷하게, 케네디 외 연구진(2012: 페이지 858)은 이것이 '재면접을 위해 의도적으로 개인을 선택하는 것보다, 또는 포화될 때까지 인터뷰만 하는 것보다 더 윤리적인' 것처럼 보였기 때문에 포화도를 초과했다고 보고했다.
9. On occasions, a reason for going beyond saturation appears to be ethical rather than methodological. Despite reaching saturation, France et al. (2008: p. 22) note that owing to their ‘commitment to and respect for all the women who wanted to participate in the study, data collection did not end until all had been interviewed.’ Similarly, Kennedy et al. (2012: p. 858) report that they exceeded saturation as this appeared to be ‘more ethical than purposefully choosing individuals to re-interview, or only interviewing until saturation’.
포화에 대한 양면적인 관점의 또 다른 징후는 인터뷰에 기초한 연구에서 표본 크기가 일반적으로 10의 배수라는 메이슨의 관찰(2010)에 의해 제안된다. 이는 실제로 포화도와 같은 적응적 접근법에 우선하여 경험칙이나 다른 선험적 지침이 일반적으로 사용된다는 것을 시사한다. 꽤 자주, 포화라는 기준을 채택하는 연구는, 선행 연구에서 제안된 표본 크기를 동시에 언급한다. 니콜라이 등은 분석 중 포화를 추구하면서, 또한 다음과 같이 진술했다.
Another indication of an ambivalent view taken on saturation is suggested by Mason’s (2010) observation that sample sizes in studies based on interviews are commonly multiples of ten. This suggests that, in practice, rules of thumb or other a priori guidelines are commonly used in preference to an adaptive approach such as saturation. Quite frequently, studies that adopt the criterion of saturation propose at the same time a prior sample size (e.g. McNulty et al. 2015; Long-Sutehall et al. 2011). In a similar way, Niccolai et al. (2016) sought saturation during their analysis, but also state (p. 843) that:
이러한 성격의 정성적 연구에 대한 권장 사항과 연구 질문에 대한 예상 복잡성 및 원하는 깊이 수준을 바탕으로
30 - 40의 선험 표본 크기를 선택했다.
An a priori sample size of 30 to 40 was selected based on recommendations for qualitative studies of this nature… and the anticipated complexity and desired level of depth for our research questions.
Fush와 Ness는 (연구자가 '데이터 포화도에 도달할 수 있는 최고의 기회'를 가진 표본 크기를 선택해야 한다고 주장할 때) 다소 일관되지 않은 이 접근법을 지지하는 것으로 보인다.
Fusch and Ness (2015: p. 1409) appear to endorse this somewhat inconsistent approach when advocating that the researcher should choose a sample size that has ‘the best opportunity for the researcher to reach data saturation’.Footnote10
- 10. Bloor and Wood (2006: 페이지 165)는 이러한 경향은 연구자들이 방법론적 신뢰성을 유지하기 위해 포화 상태에 대한 주장을 하면서 이전에 자금 지원 기관이나 윤리 위원회에 공표된 표본 크기를 준수해야 한다고 느끼는 것에서 기인할 수 있다고 시사한다. 일부 작성자—예: 게스트 등. (2006년), 프랜시스 외(2010년), 헤닝크 외(2017년)—포화 달성에 필요한 특정 참여자 수가 사전에 계산되는 절차를 공식화하려고 시도했다.
10. Bloor and Wood (2006: p. 165) suggest that this tendency may stem from researchers feeling obliged to abide by sample sizes previously declared to funding bodies or ethics committees, whilst making claims to saturation in order to retain a sense of methodological credibility. Some authors—e.g. Guest et al. (2006), Francis et al. (2010), Hennink et al. (2017)—have attempted for formulate procedures whereby the specific number of participants required to achieve saturation is calculated in advance.
이러한 포화 상태에 대한 잠정적이고 모호한 약속을 하는 모습은, 현실적으로 연구를 시작하기 전에 [표본 크기에 대한 명확한 진술을 요구하는 연구비 지원 기관과 윤리 위원회의 요구] 때문일 수 있다. 즉, 현실적으로 방법론적 고려사항보다 따라야 하는 의무로 부과되는 것이다. 그러나 (포화에 대한 모호한 모습은) 포화의 기반이 되는 [구체적이지만 다소 불확실한 논리] 때문에 발생할 수도 있다. [지금까지 수집된 데이터로부터 내린 결론에 기초하여 추가적인 데이터 수집이나 분석이 불필요하다고 판단하는 것]은 본질적으로 [관측한 것(지금까지 수행된 데이터 수집 및/또는 분석)]에 기반해서 [관측되지 않은 것(데이터 수집 및/또는 분석 프로세스가 진행되었더라면 발생했을 것)]에 대한 판단이다. 게다가, 만약 포화가 근거이론에서와 같이 [부정적 사례 분석(즉, 새로운 이론의 측면에 의문을 제기하거나 disconfirm하는 자료)]과 관련하여 사용된다면, (포화의) 논리는 더 빈약해진다.
This tentative and equivocal commitment to saturation may reflect a practical response to the demands of funding bodies and ethics committees for a clear statement of sample size prior to starting a study (O’Reilly and Parker 2013)—perceived obligations that, in practice, may be given priority over methodological considerations. However, it may also arise from the specific but somewhat uncertain logic that underlies saturation. Determining that further data collection or analysis is unnecessary on the basis of what has been concluded from data gathered hitherto is essentially a statement about the unobserved (what would have happened if the process of data collection and/or analysis had proceeded) based on the observed (the data collection and/or analysis that has taken place hitherto). Furthermore, if saturation is used in relation to negative case analysis in grounded theory (i.e. sources of data that may question or disconfirm aspects of the emergent theory) the logic becomes more tenuous—a statement about the unobserved based on the unobserved.Footnote11
- 11. 첫 번째 논리는 반직관적이다. 데이터, 코드 또는 이론적 통찰력의 미래의 미발생이 이전의 발생에 기초하여 가정된다. 그러나 부정적인 사례 분석과 관련하여, 논리는 귀납적이 되며, 미래의 비발생은 이전의 비발생에 기초하여 가정된다.
11. The first logic is counter-inductive—future non-occurrences of data, codes or theoretical insights are posited on the basis of prior occurrences. In relation to negative case analysis, however, the logic becomes inductive—future non-occurrences are posited on the basis of prior non-occurrences.
어떤 경우이든, [아직 수집되지 않은 데이터의 성격]에 대한 [불확실한 예측적 주장]이며, 더 나아가 데이터 수집 중단 결정이 뒤집힐 경우(즉, 데이터를 수집하기로 할 경우)에만 검증될 수 있는 주장이다. 또한, 기본적인 추론은 분석이 이론을 생성하는 방법에 대한 구체적인 가정을 하며, 이론 개발 과정에서 이러한 가정이 일찍 발생할수록 그러한 가정은 덜 정당화될 수 있다. 따라서 연구를 시작할 때 포화를 샘플링 기준으로 자신 있게 제안하는 연구자들은, 연구가 진행되면 어떻게 운영되어야 하는지에 대해 덜 확신하게 되어, 결과적으로 최초의 샘플링 기준을 준수하기를 꺼릴 수 있다.
In either case, an uncertain predictive claim is made about the nature of data yet to be collected, and furthermore a claim that could only be tested if the decision to halt data collection were to be overturned. Additionally, the underlying reasoning makes specific assumptions about the way in which the analysis will generate theory, and the earlier in the process of theory development that this occurs the less warranted such assumptions may be. Accordingly, researchers who confidently propose saturation as a criterion for sampling at the outset of a study may become less certain as to how it should be operationalized once the study is in progress, and may therefore be reluctant to abide by it.
결론
Conclusion
질적 연구의 퀄리티를 평가할 때 '황금률'로서의 사용되어온 포화의 명백한 위치와 거의 보편적인 적용에 대한 의문은 이전에도 제기되었다. 마찬가지로, 데이터 수집 및 분석이나 또는 이론 개발의 적절성을 판단하는 유일한 기준으로 흔하게 채택되는 것에 대해서도 의구심이 제기되어왔다. '우아함, 정확성, 일관성 및 명확성은 이론을 평가하는 전통적인 기준이나, 포화를 은유적으로 강조하면서 다소 침수되어 왔다.' (Dey) 2007: 페이지 186).
Saturation’s apparent position as a ‘gold standard’ in assessing quality and its near universal application in qualitative research have been previously questioned (Guest et al. 2006; O’Reilly and Parker 2013; Malterud et al. 2016). Similarly, doubts have been raised regarding its common adoption as a sole criterion of the adequacy of data collection and analysis (Charmaz 2005), or of the adequacy of theory development: ‘Elegance, precision, coherence, and clarity are traditional criteria for evaluating theory, somewhat swamped by the metaphorical emphasis on saturation’ (Dey 2007: p. 186).
포화 상태가 의심할 여지 없이 제공되어서는 안 된다는 주장에 동의하지만, 적용 가능 vs 적용 불가능의 양극화로 보는 것은 너무 단순한 것이다. 대신, 우리는 포화가 이론의 역할, 채택된 분석적 접근법 등에 따라 다른 관련성과 다른 의미를 가진다고 주장한다. 따라서 연구자에 의해 명확하게 표현될 필요가 있는 각 유형의 연구에 맞춰서, 각각의 목적에 따라 유용하게 사용될 수 있다고 제안한다.
Whilst we concur with the argument that saturation should not be afforded unquestioned status, polarization of saturation as either applicable or non-applicable to different approaches, as has been suggested (Walker 2012), may be too simplistic. Instead we propose that saturation has differing relevance, and a different meaning, depending on the role of theory, the analytic approach adopted, and so forth, and thus may usefully serve different purposes for different types of research—purposes that need to be clearly articulated by the researcher.
포화의 목적과 사용 측면에서 유연성이 있어야 한다고 주장하면서, 동시에 우리는 이러한 [(포화를 사용하는) 목적의 범위]에는 어느 정도 [제한이 있어야 한다]고 제안한다. 포화 상태가 운영화된 방법 중 일부는 너무 광범위하게 포괄될 정도로 의미를 확장하거나 희석시킬 위험이 있어 일관성과 효용성을 훼손할 수 있다고 제안할 수 있다.
Whilst arguing for flexibility in terms of the purpose and use of saturation, we also suggest that there must be some limit to this range of purposes. Some of the ways in which saturation has been operationalized, we would suggest, risk stretching or diluting its meaning to the point where it becomes too widely encompassing, thereby undermining its coherence and utility.
언제 그리고 어떻게 포화 상태에 도달했다고 판단될 수 있는지는, 연구 유형뿐만 아니라 그것이 [뚜렷한distinct 사건인지] 또는 [진행 중인 과정을 나타내는지]에 대한 가정에 따라서도 달라진다. 포화를 사건으로 보는 관점은 다른 사람들에 의해 문제화되었다. 우리가 보기에 포화는 부분적으로 [개념 자체의 불확실한 논리]에 의해 추진되는 것으로 보인다고 주장했다. 즉, 포화는 [관찰된 것을 바탕으로 관찰되지 않은 것에 대한 설명]을 하는 것이다. 이러한 불확실성은 그것의 사용에 있어서 비일관성과 모순을 야기하는 것으로 보이며, 우리는 포화를 단순히 달성되거나 달성되지 않은 것으로 간주하기 보다는, 최소한 부분적으로라도 정도의 문제로 간주한다면 해결될 수 있다고 주장한다. 그러나 '점진적'이라는 측면에서 포화를 고려하는 것은, 포화를 주장하는 연구자들의 자신감을 높일 수 있지만, 우리는 연구자가 포화를 사용하는 구체적인 목적이 무엇인지, 포화시키기를 바라는 것이 무엇인지를 적절히 고려해야만 개념을 둘러싼 불확실성을 해결할 수 있다고 제안한다.
When and how saturation may be judged to have been reached will differ depending on the type of study, as well as assumptions about whether it represents a distinct event or an ongoing process. The view of saturation as an event has been problematized by others (Strauss and Corbin 1998; Dey 1999; Nelson 2016), and we have explored the implications of conceptualizing saturation in this way, arguing that it appears to give rise to a degree of uncertainty and equivocation, in part driven by the uncertain logic of the concept itself—as a statement about the unobserved based on the observed. This uncertainty appears to give rise to inconsistencies and contradictions in its use, which we would argue could be resolved, at least in part, if saturation were to be considered as a matter of degree, rather than simply as something either attained or unattained. However, whilst considering saturation in incremental terms may increase researchers’ confidence in making claims to it, we suggest it is only through due consideration of the specific purpose for which saturation is being used, and what one is hoping to saturate, that the uncertainty around the concept can be resolved
doi: 10.1007/s11135-017-0574-8. Epub 2017 Sep 14.
Saturation in qualitative research: exploring its conceptualization and operationalization
PMID: 29937585
PMCID: PMC5993836
DOI: 10.1007/s11135-017-0574-8
Free PMC article
Abstract
Saturation has attained widespread acceptance as a methodological principle in qualitative research. It is commonly taken to indicate that, on the basis of the data that have been collected or analysed hitherto, further data collection and/or analysis are unnecessary. However, there appears to be uncertainty as to how saturation should be conceptualized, and inconsistencies in its use. In this paper, we look to clarify the nature, purposes and uses of saturation, and in doing so add to theoretical debate on the role of saturation across different methodologies. We identify four distinct approaches to saturation, which differ in terms of the extent to which an inductive or a deductive logic is adopted, and the relative emphasis on data collection, data analysis, and theorizing. We explore the purposes saturation might serve in relation to these different approaches, and the implications for how and when saturation will be sought. In examining these issues, we highlight the uncertain logic underlying saturation-as essentially a predictive statement about the unobserved based on the observed, a judgement that, we argue, results in equivocation, and may in part explain the confusion surrounding its use. We conclude that saturation should be operationalized in a way that is consistent with the research question(s), and the theoretical position and analytic framework adopted, but also that there should be some limit to its scope, so as not to risk saturation losing its coherence and potency if its conceptualization and uses are stretched too widely.
Keywords: Data analysis; Data collection; Grounded theory; Qualitative research; Saturation.
'Articles (Medical Education) > 의학교육연구(Research)' 카테고리의 다른 글
효과적인 문헌고찰 쓰기 파트1: 매핑 더 갭 (Perspect Med Educ, 2018) (0) | 2021.12.13 |
---|---|
질적연구의 일반화가능성: 오해, 기회, 권고(Qualitative Research in Sport, Exercise and Health, 2018) (0) | 2021.11.23 |
의료전문직 학술활동: 그것의 등장, 현재상태, 미래(FASEB Bioadv. 2021) (0) | 2021.11.19 |
When I say … 리커트 문항(Med Educ, 2021) (0) | 2021.11.02 |
정답은 하나? (성찰적) 주제분석의 옳바른 실천은 무엇인가? (Qualitative Research in Psychology, 2021) (0) | 2021.10.22 |