의학교육에서 인공지능 훈련 도입하기 (JMIR Med Educ. 2019)
Introducing Artificial Intelligence Training in Medical Education
Ketan Paranjape1*, MS, MBA; Michiel Schinkel2*, MD; Rishi Nannan Panday3, MD; Josip Car4, MD, PhD, FRCP;
Prabath Nanayakkara2, MD, PhD, FRCP
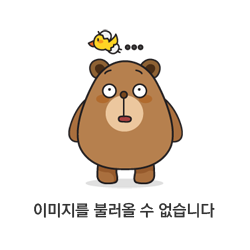
의료 서비스 트렌드
Trends in Health Care
전 세계 의료 서비스 지출은 2017년 7조 7,000억 달러에서 2022년 10조 달러로 5.4%의 비율로 증가할 것으로 예상됩니다[1]. 이는 선진국의 경우 국내총생산에서 의료비가 차지하는 비중이 평균 9%에 달한다는 것을 의미합니다[2,3]. 의료 서비스 접근성 및 경제성 확대에 영향을 미칠 수 있는 미국의 세제 개혁 및 정책 변화(건강보험개혁법)[4], 유럽연합 탈퇴 결정에 따른 영국의 의료 서비스 지출에 대한 영향[5] 등이 주요 글로벌 동향으로 꼽힙니다, 중국과 인도의 인구 증가와 부의 증가[6-8], 러시아의 의료 서비스를 위한 사회경제 정책 개혁 시행[9], 아르헨티나의 보편적 의료 서비스를 효과적으로 만들기 위한 시도[10], 아프리카의 전자 의료 및 원격 의료에 대한 대대적인 추진[11], 전 세계적으로 전례 없는 인구 고령화 속도의 영향[12] 등이 있습니다.
Global health care expenditure has been projected to grow from US $7.7 trillion in 2017 to US $10 trillion in 2022 at a rate of 5.4% [1]. This translates into health care being an average of 9% of gross domestic product among developed countries [2,3]. Some key global trends that have led to this include tax reform and policy changes in the United States that could impact the expansion of health care access and affordability (Affordable Care Act) [4], implications on the United Kingdom’s health care spend based on the decision to leave the European Union [5], population growth and rise in wealth in both China and India [6-8], implementation of socioeconomic policy reform for health care in Russia [9], attempts to make universal health care effective in Argentina [10], massive push for electronic health and telemedicine in Africa [11], and the impact of an unprecedented pace of population aging around the world [12].
임상의의 관점에서 볼 때 의료 정보의 증가는 놀라울 정도로 의료 서비스 제공 방식에 영향을 미치는 중요한 트렌드가 많이 있습니다. 1950년에 의료 정보가 두 배로 늘어나는 데 50년이 걸렸습니다. 1980년에는 7년이 걸렸습니다. 2010년에는 3.5년이 걸렸고 2020년에는 73일 만에 두 배가 될 것으로 예상됩니다[13]. 이러한 성장은 의료 전문가들이 의료 정보를 보유하고 이를 효과적으로 진료에 활용해야 하는 과제를 안겨주고 있습니다.
From clinicians’ perspective there are many important trends that are affecting the way they deliver care of which the growth in medical information is alarming. It took 50 years for medical information to double in 1950. In 1980, it took 7 years. In 2010, it was 3.5 years and is now projected to double in 73 days by 2020 [13]. This growth is posing a challenge to health care professionals to both retain and use it effectively to practice medicine.
의료 분야에서 인공지능의 부상
Rise of Artificial Intelligence in Health Care
의료 분야의 인공 지능
Artificial Intelligence in Health Care
인공 지능(AI)은 일반적으로 인간의 특성인 작업을 수행할 수 있는 컴퓨터 알고리즘을 이해하고 만드는 데 중점을 둔 과학 분야입니다[14]. AI는 이제 의료 분야에서 탄력을 받고 있습니다. "기계가 생각할 수 있는가?"라는 질문을 제기한 튜링의 중요한 논문인 컴퓨팅 기계와 지능[15]에 뿌리를 둔 AI는 먼 길을 걸어왔습니다. 자연어 처리(NLP)[16], 음성 인식[17], 가상 에이전트[18], 의사 결정 관리[19], 머신 러닝[20], 딥 러닝[21], 로봇 프로세스 자동화[22] 등이 AI의 발전 사례로 꼽힙니다.
Artificial intelligence (AI) is a scientific discipline that focuses on understanding and creating computer algorithms that can perform tasks that are usually characteristics of humans [14]. AI is now gaining momentum in health care. From its early roots in Turing’s seminal paper, Computing Machinery and Intelligence [15], where he proposed the question “Can machines think?”, AI has come a long way. Examples of advances in AI include natural language processing (NLP) [16], speech recognition [17], virtual agents [18], decision management [19], machine learning [20], deep learning [21], and robotic process automation [22].
오늘날 AI는 의료 분야에서 더 빠르고 정확한 진단을 위해[23], 영상의학을 보강하고[24], 사람의 피로로 인한 오류를 줄이고, 의료 비용을 절감하고[25], 지루하고 반복적이며 노동 집약적인 작업을 보조 및 대체하고[26], 최소 침습 수술[27], 사망률을 줄이기 위해[28] 시범적으로 도입되고 있습니다.
Today, AI is being piloted in health care [23] for faster and accurate diagnosis, to augment radiology [24], reduce errors due to human fatigue, decrease medical costs [25], assist and replace dull, repetitive, and labor-intensive tasks [26], minimally invasive surgery [27], and reduce mortality rates [28].
인공 지능의 도전 과제
Challenges With Artificial Intelligence
의료 분야에서 인공지능이 부상하고 일상적인 임상 진료에 통합되는 것은 어려운 과제가 될 것입니다. 의사의 기존 업무 방식 변화와 함께 블랙박스 문제[29]와 책임 문제[30]가 가장 예상되는 과제 중 하나입니다.
The rise of AI in health care and its integration into routine clinical practice is going to be a challenge. Along with changing the conventional ways physician work, the black box problem [29] and liability issues [30] are some of the most anticipated challenges.
블랙박스
Black Box
마운트 사이나이 병원의 연구원들은 70만 명의 환자 데이터로 학습된 딥러닝 알고리즘을 개발했습니다. 이 알고리즘은 정신분열증과 같은 질병의 발병을 높은 정확도로 예측할 수 있었습니다[31]. 이 질환은 전문가도 진단하기 어렵다는 사실을 고려하면 더욱 인상적입니다. 이 알고리즘의 가장 큰 문제점은 시스템이 어떻게 이러한 예측을 생성했는지, 어떤 요소를 고려했는지 알 수 없다는 것입니다. 이러한 현상을 블랙박스 현상이라고 합니다. 의학에서는 전례가 없는 일이지만, 시스템이 어떻게 작동하는지에 대한 이해가 없는 경우 시스템을 신뢰하기는 어렵습니다. 의사는 입력과 알고리즘을 이해하고 AI가 제안한 진단을 해석하여 오류가 없는지 확인해야 합니다. 또한 표준 치료법에 비해 좋은 결과를 입증할 수 있는 경우에도 블랙박스 의학의 결과 또는 의도하지 않은 부작용이 무엇인지 이해해야 합니다.
Researchers at Mount Sinai Hospital have created a deep learning algorithm that was trained on the data of 700,000 patients. This algorithm was able to predict onset of a disease such as schizophrenia with high accuracy [31]. This is even more impressive considering the fact that this condition is difficult to diagnose even for experts. The main problem with this algorithm is that there is no way to know how the system created this prediction and what factors were taken into consideration. This phenomenon is called the black box phenomenon. It would not be a precedent in medicine, nevertheless it is difficult to trust a system when there is no understanding on how it works. The physician needs to understand the inputs and the algorithm and interpret the AI-proposed diagnosis to ensure no errors are made. We also need to understand what the consequences or unintended side effects are of black box medicine, even when good outcomes can be demonstrated against a standard of care.
마지막으로, 많은 AI 시스템이 인간과 동물의 중추 신경계의 측면을 모방하려고 시도하지만, 이는 여전히 블랙박스에 가깝습니다. 최근 논문에서 자도르[32]는 동물의 뇌에서 이 현상을 이해하기 위해 더 많은 것을 배울 수 있다고 주장했습니다.
Finally, many of the AI systems attempt to mimic aspects of human and animal central nervous systems that are, at large, still a black box. In a recent paper, Zador [32] argued that we have much more to learn from animal brains to unravel this phenomenon.
개인정보 보호 및 데이터 제어
Privacy and Control Over Data
AI 알고리즘을 개발하려면 거의 대부분 많은 환자의 데이터가 필요합니다. 예를 들어, Google은 2개 병원에서 11년 동안 총 216,221명의 성인 비식별 데이터에서 수집한 460억 개의 데이터 포인트를 사용하여 입원 환자의 결과를 예측하고 있습니다[33,34]. 이는 환자 개인정보 보호 및 통제와 관련하여 많은 우려를 불러일으킵니다. 환자가 자신의 정보가 알고리즘 개발에 사용되는 연구에 참여하기를 원하지 않는다면 어떻게 될까요? 유럽연합에서는 잊혀질 권리에 따라 환자가 동의를 철회한 경우 개인 데이터를 삭제할 수 있습니다[35]. 환자 데이터가 제한적인 상황에서 알고리즘 개발자는 합성 데이터 또는 가상의 데이터로 모델을 학습시키므로 안전하지 않고 잘못된 치료 권장 사항을 생성할 위험이 있습니다[36]. 마지막으로, AI 시스템은 알고리즘이 의료 정보를 잘못 분류할 수 있는 사이버 보안 공격에도 취약합니다[37].
The development of AI algorithms almost as a rule requires data from a large number of patients. Google, for example, is using 46 billion data points collected from 216,221 adults’ deidentified data over 11 combined years from 2 hospitals to predict the outcomes of hospitalized patients [33,34]. This raises many concerns including relating to patient privacy and control. What happens if a patient does not want to participate in a study where their information is used in algorithm development? In the European Union, the Right to be Forgotten would allow personal data to be erased when the patient has withdrawn their consent [35]. In situations where patient data are limited, algorithm developers train the models on synthetic or hypothetical data, with the risk of generating unsafe and incorrect treatment recommendations [36]. Finally, AI systems are also vulnerable to cybersecurity attacks that could cause the algorithm to misclassify medical information [37].
환자 치료 및 책임에 대한 인공지능 사용 기준의 부재
Lack of Standards for Use of Artificial Intelligence in Patient Care and Liability
의료 분야에서 인공지능의 사용과 관련하여 해결되지 않은 또 다른 문제는 알고리즘의 예측에 대한 책임입니다. 부정확한 예측으로 인해 환자가 심각한 피해를 입었을 때 누가 책임을 져야 하는지 불분명합니다. 의사, 병원, 소프트웨어를 개발한 회사, 소프트웨어를 개발한 사람, 심지어 데이터를 제공한 사람 등 모든 관련 당사자가 책임을 져야 한다고 주장할 수 있습니다. 의료 분야에서 AI를 사용하기 위한 표준은 여전히 개발 중입니다[38,39]. 임상 치료, 품질, 안전, 의료 과실, 커뮤니케이션 가이드라인에 대한 새로운 표준을 개발하여 AI를 더 많이 사용할 수 있도록 해야 합니다. 최근 출시된 당뇨망막병증의 자율 감지를 위한 AI 시스템은 의료 과실 및 책임 보험에 가입되어 있습니다[40,41].
Another unresolved question related to the use of AI in health care is liability for the predictions of an algorithm. It is unclear who is liable when a patient experiences serious harm because of an inaccurate prediction. One could argue for any of the involved parties: the physician, the hospital, the company that developed the software, the person who developed the software, or even the person who delivered the data. Standards for use of AI in health care are still being developed [38,39]. New standards for clinical care, quality, safety, malpractice, and communication guidelines have to be developed to allow for greater use of AI. A recently launched AI system for autonomous detection of diabetic retinopathy carries medical malpractice and liability insurance [40,41].
AI 사용과 챗봇[42]과 같은 도구의 적극적인 사용이 증가함에 따라 의사와 환자는 이러한 기술의 강점과 한계를 인식하고 효과적이고 안전하게 사용하는 방법에 대한 교육을 받아야 합니다[43,44].
As use of AI and proactive use of tools such as chatbots [42] increases, physicians and patients will need to be aware of strengths and limitations of such technologies and be trained in how to effectively and safely use them [43,44].
인공지능은 오늘날 의사의 과제를 어떻게 해결할 수 있을까요?
How Can Artificial Intelligence Address Today’s Physician Challenges?
의료 정보가 빠른 속도로 증가함에 따라 의사들은 이를 따라잡는 데 어려움을 겪고 있습니다. 이로 인해 정보 과부하가 발생하고 레지던트 자격을 얻기 위해 미국 의사 면허 시험(USMLE)을 통과하기 위해 이 모든 내용을 암기해야 한다는 압박감이 커지고 있습니다. 오늘날 의사들은 의료 비용이 증가하고 규제로 인해 행정 절차에 추가적인 부담이 가중되는 복잡한 질환과 동반 질환이 있는 고령화 사회에서 더 긴 시간 동안 일하고 있으며[45,46] 조정된 치료를 제공해야 할 것으로 예상됩니다.
With medical information growing at a breakneck speed, physicians are having trouble keeping up. This is leading to information overload and creates pressure to memorize all this content to pass the United States Medical Licensing Examinations (USMLE) to qualify for residency positions. Physicians today are working longer hours and are also expected to deliver coordinated care [45,46] in an aging society with complex conditions and comorbidities where health care costs are increasing and regulations are putting an additional burden on administrative processes.
AI는 대량의 데이터를 통합하고 의사 결정 과정을 보완하여 진단을 식별하고 치료법을 추천함으로써 의사를 도울 수 있습니다. 의사는 결과를 해석하고 환자에게 권장 사항을 전달할 수 있는 능력이 필요합니다.
- 또한 AI는 일상적인 업무 수행에 대한 의사의 부담을 덜어줌으로써 영향을 미칠 수 있습니다[47].
- 음성 인식은 키보드 사용을 대체하여 정보를 입력하고 검색하는 데 도움이 될 수 있습니다[48].
- 의사 결정 관리는 방대한 양의 데이터를 선별하고 의사가 정보에 입각하여 의미 있는 결정을 내리는 데 도움이 될 수 있습니다[49,50].
- 자동화 도구는 의료 접근성 보호법과 같은 규제 요건을 관리하는 데 도움이 될 수 있으며 의사가 비용 결정을 내리기 전에 적절한 기준을 검토할 수 있도록 지원합니다[51].
- 마지막으로, 의료 전문가의 심각한 부족 문제를 해결하기 위해 가상 에이전트는 향후 환자 치료의 일부 측면을 지원하고 환자를 위한 신뢰할 수 있는 정보원이 될 수 있습니다[52].
AI could help physicians by amalgamating large amounts of data and complementing their decision-making process to identify diagnosis and recommend treatments. Physicians in turn need the ability to interpret the results and communicate a recommendation to the patient.
- In addition, AI could have an impact by alleviating the burden from physicians for performing day-to-day tasks [47].
- Speech recognition could help with replacing the use of keyboards to enter and retrieve information [48].
- Decision management can help with sifting enormous amounts of data and enable the physician to make an informed and meaningful decision [49,50].
- Automation tools can help with managing regulatory requirements such as Protecting Access to Medicare Act and enable physicians to review the appropriate criteria before making a cost decision [51].
- Finally, to help with the acute shortage of health care professionals, virtual agents could, in the future, help with some aspects of patient care and become a trusted source of information for patients [52].
의학교육에서의 인공 지능 교육
Artificial Intelligence Training in Medical Education
오늘날 의학교육의 현황
State of Medical Education Today
의사는 전문의가 되기까지 오랜 기간의 수련 과정을 거칩니다. 의학은 지난 수십 년 동안 큰 변화를 겪었지만, 의학교육은 여전히 전통적인 커리큘럼을 기반으로 하고 있습니다[53]. 구체적인 교육 기간은 국가마다 다르지만 이러한 커리큘럼의 핵심 역량은 전 세계적으로 유사합니다[54]. 전임상 교육의 핵심 단계 이후 교육은 대부분 실습 기반 학습을 중심으로 이루어집니다[53]. 의학교육은 환자 치료, 의학 지식, 대인관계 및 의사소통 기술, 실습 기반 학습 및 개선, 전문성, 시스템 기반 실습 등 6가지 영역을 기반으로 하는 경우가 많습니다[55]. 이러한 영역은 의학 교육 졸업 인증 위원회(ACGME)에 의해 도입되었습니다. 의료 교육의 대부분은 가능한 한 많은 정보를 소비하고 이 지식을 환자 치료에 적용하는 방법을 배우는 데 중점을 둡니다. 이 과정은 여전히 대부분 암기 위주입니다[56]. 의대생이나 레지던트들이 AI, 모바일 의료 애플리케이션, 원격 의료와 같은 새로운 기술에 익숙해지는 데 소요되는 시간은 적습니다[53,55,56]. 미국에서는 USMLE에서 이러한 주제에 대한 시험을 치르지 않습니다[57]. 그러나 2018년 미국 의학 협회(AMA) 연례 회의에서 증강 지능에 대한 AMA의 첫 번째 정책이 채택되어 의학교육에서 AI를 어떻게 다뤄야 하는지에 대한 연구가 장려된 이후 변화는 불가피한 것으로 보입니다[58]. 표 1에는 AMA에서 제시한 의학교육에 AI를 통합하기 위한 몇 가지 이니셔티브가 나와 있습니다[58].
Physicians go through extensive periods of training before they can eventually register as specialists. Although medicine has seen major changes over the last decades, medical education is still largely based on traditional curricula [53]. The specific length of training differs between countries, but the core competencies of these curricula are globally similar [54]. After a core phase of preclinical didactics, training is mostly centered around practice-based learning [53]. Medical education is often based on 6 domains: patient care, medical knowledge, interpersonal and communication skills, practice-based learning and improvement, professionalism, and systems-based practice [55]. These fields were introduced by the Accreditation Council for Graduating Medical Education (ACGME). A large part of medical training focuses on consuming as much information as possible and learning how to apply this knowledge to patient care. This process is still largely memorization based [56]. Less time is spent on familiarizing medical students or residents with new technologies such as AI, mobile health care applications, and telemedicine [53,55,56]. In the United States, USMLE does not test on these subjects [57]. However, change seems inevitable since the 2018 annual meeting of the American Medical Association (AMA) saw the adoption of AMA’s first policy on augmented intelligence, encouraging research into how AI should be addressed in medical education [58]. In Table 1, several initiatives for incorporating AI in medical education are shown, as presented by the AMA [58].
Institution | Project |
Duke Institute for Health Innovation | Medical students work together with data experts to develop care-enhanced technologies made for physicians |
University of Florida | Radiology residents work with a technology-based company to develop computer-aided detection for mammography |
Carle Illinois College of Medicine | Offers a course by a scientist, clinical scientist, and engineer to learn about new technologies |
Sharon Lund Medical Intelligence and Innovation Institute | Organizes a summer course on all new technologies in health care, open to medical students |
Stanford University Center for Artificial Intelligence in Medicine and Imaging | Involves graduate and postgraduate students in solving heath care problems with the use of machine learning |
University of Virginia Center for Engineering in Medicine | Involves medical students in the engineering labs to create innovative ideas in health care |
AMA는 의사와 수련 중인 의사를 위한 AI 교육 모듈 개발 장려, 환자 치료에 영향을 미칠 수 있는 AI 교육 격차 해소, 의사가 AI 교육 자료 개발 및 실행에 참여하도록 보장하는 등 의학교육에 AI를 통합하기 위한 정책을 채택했습니다. 이러한 새로운 정책의 결과로 AMA는 다음과 같이 장려할 것입니다:
The AMA adopted policies on integrating AI into medical education, which include encouraging the development of AI education modules for physicians and physicians-in-training, addressing disparities in AI education that could impact patient care, and ensuring that physicians are involved in the development and implementation of educational materials on AI. As a result of these new policies, the AMA will encourage:
- 인증 및 면허 기관은 인증 및 면허 기준에서 AI를 가장 적절하게 다루는 방법을 연구해야 합니다.
- 의료 전문 학회 및 이사회는 AI와 관련된 전문 분야별 교육 모듈 제작을 고려합니다.
- 의학교육에서 AI 교육이 학습 및 임상 결과에 미치는 효과에 관한 연구.
- 교육기관과 프로그램은 AI 보조 기술을 언제 가르쳐야 하는지 결정할 때 신중해야 하며, 여기에는 확립된 근거 기반 치료법을 고려하고 새로운 교육 모듈을 수용하기 위해 제거해야 할 다른 커리큘럼에 대한 고려가 포함됩니다.
- 이해관계자는 학습자가 AI 시스템에 내재되어 있을 수 있는 편견이 의도치 않게 전파되는 것을 방지하는 데 도움이 되는 교육 자료를 제공해야 합니다.
- AI에 대한 기관별 접근성의 차이가 자원이 적고 AI 기술에 대한 접근성이 낮은 학교의 학생 교육 격차에 어떤 영향을 미칠 수 있는지에 대한 연구.
- 환자 치료에서 데이터의 평가, 이해 및 적용에 관한 의학교육 전반에 걸친 교육 강화.
- AI 교육 자원의 격차가 자원이 부족하고 AI 기술에 대한 접근성이 낮은 지역사회의 환자들의 의료 서비스 격차에 어떤 영향을 미칠 수 있는지에 대한 연구.
- 교육 기관의 리더와 학장은 학습자의 AI 이해와 사용을 돕기 위해 데이터 과학자 및 엔지니어와 같은 비임상의를 교수진 명단에 포함시키는 것을 적극적으로 추진해야 합니다.
- AI 애플리케이션 개발 시 임상의사와 긴밀히 협력하고 감독합니다.
- Accrediting and licensing bodies to study how AI should be most appropriately addressed in accrediting and licensing standards.
- Medical specialty societies and boards to consider production of specialty-specific educational modules related to AI.
- Research regarding the effectiveness of AI instruction in medical education on learning and clinical outcomes.
- Institutions and programs to be deliberative in the determination of when AI-assisted technologies should be taught, including consideration of established evidence-based treatments, and including consideration regarding what other curricula may need to be eliminated in order to accommodate new training modules.
- Stakeholders to provide educational materials to help learners guard against inadvertent dissemination of bias that may be inherent in AI systems.
- The study of how differences in institutional access to AI may impact disparities in education for students at schools with fewer resources and less access to AI technologies.
- Enhanced training across the continuum of medical education regarding assessment, understanding, and application of data in the care of patients.
- The study of how disparities in AI educational resources may impact health care disparities for patients in communities with fewer resources and less access to AI technologies.
- Institutional leaders and academic deans to proactively accelerate the inclusion of non-clinicians, such as data scientists and engineers, onto their faculty rosters in order to assist learners in their understanding and use of AI.
- Close collaboration with and oversight by practicing physicians in the development of AI applications.
의료 교육에서 종종 간과되는 또 다른 중요한 기술 관련 측면은 전자 건강 기록(EHR)을 사용하는 것입니다. EHR은 환자 안전 개선과 같은 많은 이점을 제공할 뿐만 아니라 의료 서비스에서 AI를 구현하는 데도 도움이 됩니다. AI 알고리즘은 EHR의 정보를 사용하므로 편향되지 않은 데이터를 EHR에 입력하는 방법에 대한 지식이 필수적입니다. 그렇지 않으면 AI 알고리즘도 편향될 가능성이 높습니다[59]. 현재 의대생과 의사를 대상으로 한 EHR 사용 교육은 의료 커리큘럼에 일반적으로 포함되지 않아[60], 의료 전문가가 이 기술의 진정한 잠재력을 이해하지 못한 채 종이로 정보를 캡처하는 대체 수단으로 EHR을 사용하고 있습니다[61]. EHR 사용에 대한 교육은 일반적으로 병원 시스템을 실제로 사용하기 위한 기본 기술만 가르치는 임시 임시 입문 과정으로 구성됩니다. 데이터의 품질과 컴퓨터가 환자-의사 관계에 미치는 영향에 대한 우려는 거의 다루어지지 않으며[60], USMLE에서도 이러한 주제에 대해 테스트하지 않습니다[57].
Another important technology-related aspect that is often overlooked in medical training is working with electronic health records (EHRs). EHRs have many benefits, such as improved patient safety, but also assist the implementation of AI in health care. AI algorithms use information from the EHR, and therefore, the knowledge on how to input unbiased data into the EHR is essential. Otherwise, the AI algorithm will likely be biased as well [59]. At present, training on use of EHRs for medical students and physicians is not commonly incorporated in the medical curriculum [60], resulting in the medical professional using the EHR as a replacement to capture information on paper without understanding the true potential of this technology [61]. Training on the use of EHRs usually consists of ad hoc brief introductory courses that just teach the basic skills to use the hospital’s system in practice. Quality of data and concerns on the impact of the computer on the patient-physician relationship are rarely addressed [60] and the USMLE does not test on these subjects either [57].
임상의료행위의 변화
How Clinical Practice Is Changing
의료 서비스의 급속한 디지털화로 인해 EHR은 정보에 입각한 결정을 내리는 데 사용할 수 있는 귀중한 정보를 획득하고 처리하는 새로운 방법을 촉진합니다[62]. 이러한 발전과 정보화 시대에서 AI 시대로의 전환[56]은 임상 진료와 환자 치료 결과를 더 나은 방향으로 변화시킵니다. 미래의 의사들은 데이터 관리, AI 도구 감독, 정보에 입각한 의사 결정을 내리기 위한 AI 애플리케이션 사용 등 다양한 기술과 역량을 갖춰야 할 것입니다.
With the rapid digitization of health care, EHRs facilitate new ways to acquire and process valuable information that can be used to make an informed decision [62]. These advances and transitioning from an information age to the age of AI [56] change clinical practice and patient outcomes for the better. Physicians of the future will have to add to the armory of their skills and competencies, the ability to manage data, supervise AI tools, and use AI applications to make informed decisions.
의사는 이러한 도구 중 어떤 것이 환자에게 가장 적합한지 결정하는 데 중요한 역할을 하게 될 것입니다. 이는 결국 의사와 환자의 관계를 변화시킬 가능성이 높습니다[63]. 정보 처리가 주로 컴퓨터에 의해 이루어지면 의사가 환자를 돌보고 소통하는 데 더 집중할 수 있다는 점에서 의료 분야에서 AI의 주요 이점 중 하나가 부각됩니다[64]. 마지막으로, AI 시대에는 "의사는 내러티브, 기계론적, 수학적 사고를 훈련에 결합하고, 환자를 중심에 두고 질병의 생체-사회적 모델을 고려해야 한다"고 말합니다. "컴퓨터는 인간의 강점과 한계, 그리고 정보 과부하가 특징인 환경을 인식하는 자기 성찰적인 의료 전문가를 결코 대체할 수 없습니다."[65,66].
Physicians will have a crucial role in deciding which of these tools is best for their patients. In turn, this will likely change the physician-patient relationship [63]. When information processing is done mainly by computers, this highlights one of the major benefits of AI in medicine: it allows the physician to focus more on caring for and communicating with patients [64]. Finally, in the age of AI, “the physician should combine narrative, mechanistic and mathematical thinking in their training and consider the biopsycho-social model of the disease with the patient at its center.” “Computers will never substitute for self-reflective medical expert who is aware of the strengths and limitations of human beings and of an environment characterized by information overload” [65,66].
미래에는 의사에게 무엇을 요구할까요?
What Will Be Asked From Physicians in the Future?
미래의 의사들은 임상에서 AI를 적절히 활용하기 위해 광범위한 기술을 필요로 할 것입니다. 의사는 의학의 원리를 이해하는 것 외에도 수학적 개념, AI 기초, 데이터 과학 및 관련 윤리적, 법적 문제에 대한 충분한 지식을 습득해야 할 것입니다. 이러한 기술은 다양한 출처의 데이터를 사용하고, AI 도구를 감독하며, 알고리즘이 예상보다 정확하지 않을 수 있는 경우를 인식하는 데 도움이 됩니다[67]. 또한, AI 기반 시스템이 환자의 신체적, 정서적 상태를 모두 고려할 수 없기 때문에 커뮤니케이션 및 리더십 기술과 감성 지능이 그 어느 때보다 중요해질 것입니다[56]. 이러한 특성은 컴퓨터가 습득하기 어렵기 때문에 AI 시대에 훌륭한 의사의 특징이 될 것입니다.
Future physicians will need a broad range of skills to adequately use AI in clinical practice. Besides understanding the principles of medicine, physicians will also need to acquire satisfactory knowledge of mathematical concepts, AI fundamentals, data science, and corresponding ethical and legal issues. These skills will help them to use data from a broad array of sources, supervise AI tools, and recognize cases where algorithms might not be as accurate as expected [67]. Furthermore, communication and leadership skills as well as emotional intelligence will be more important than ever as AI-based systems will not be able to consider all the physical and emotional states of the patient [56]. These traits are hard to master for computers and will characterize a great physician in the age of AI.
실용적인 고려 사항
Practical Considerations
의료 정보를 암기하는 데 소요되던 시간 중 일부는 이제 다른 기술에 투자해야 할 것입니다. 이는 학생과 레지던트가 수련을 경험하는 방식에 큰 영향을 미칠 것입니다. 더 이상 사실적 지식이 아닌 의사소통 능력, 감성 지능, 컴퓨터 사용법에 대한 지식으로 역량을 판단하는 방식으로 시스템이 바뀌어야 합니다.
Some of the time that was originally spent on memorizing medical information will now have to be devoted to other skills. This will have a major impact on the way students and residents will experience their training. The system has to change in such a way that competence will no longer be judged based on factual knowledge but rather on communication skills, emotional intelligence, and knowledge on how to use computers.
2016년 AMA의 설문조사에 따르면 의사의 85%가 새로운 디지털 도구의 이점을 인식하고 있지만[58], 커리큘럼이 지나치게 많기 때문에 새로운 주제를 채택하는 데 관심이 제한되어 있습니다[68]. AI 중심 교육을 의료 커리큘럼에 통합하는 것은 기술이 발전함에 따라 시간이 걸릴 것입니다. 학습을 위한 새로운 인프라를 도입해야 하며 컴퓨터 과학, 수학, 민족지학, 경제학 등의 분야에서 새로운 교육자를 채용해야 합니다. 현재 이러한 과목은 ACGME의 핵심 역량에 포함되지도 않지만 이러한 역량은 "변화하는 지식에 적응할 수 있을 만큼 충분히 견고합니다"[69].
With an overfull curriculum, there is limited interest in adopting new topics [68], although a 2016 survey by AMA shows that 85% of physicians perceive benefits from new digital tools [58]. The integration of AI-oriented education into the medical curriculum will take time as the technology evolves. A new infrastructure for learning has to be introduced, and new educators from disciplines such as computer sciences, mathematics, ethnography, and economics will need to be hired. At the moment, these subjects are not even covered by the core competencies of ACGME, but these competencies “are robust enough to adapt to changing knowledge” [69].
커리큘럼의 변화를 이루기 위해서는 많은 정치적, 관료적 장애물을 극복해야 합니다. 새로운 학습 성과를 창출하기 위해서는 교육 시스템, 프로그램 구조, 목표가 바뀌어야 합니다[70]. 변화는 많은 양의 증거가 생성될 때만 실행될 수 있습니다. 우리는 아직 인공지능을 위한 변화를 구현할 단계에 이르지 못했습니다. 또한 의학 내 다른 많은 분야에서도 마땅히 받아야 할 관심을 받지 못했다고 주장합니다[71,72]. AI는 그 이점을 증명해야 하며, 현재 적절한 의학 교육이 부족한 다른 중요한 과목보다 의학 커리큘럼의 중요한 주제라는 점을 정당화해야 합니다.
To achieve a change in curriculum, many political and bureaucratic hurdles have to be overcome. Educational systems, program structures, and objectives have to change to create new learning outcomes [70]. A change can only be implemented when large amount of evidence is generated. We have not reached that stage of implementing changes for AI. Furthermore, many other fields within medicine argue that they have not received the attention they deserve [71,72]. AI needs to prove its benefits and also justify that it is an important topic for medical curriculum over other important subjects that lack adequate medical training at present.
그러나 의학교육에서 AI 교육을 실시해야 한다는 가장 설득력 있는 주장 중 하나는 이 교육이 기존 교과 과정을 대체하기보다는 기존 교과 과정을 보강할 수 있다는 것입니다. 학생들이 AI 도구 사용법을 교육받을 때는 도구 사용법에 대한 기본 지식을 습득하는 것에서 기본 원리에 대한 기본적인 이해로 초점을 옮겨야 합니다. 이렇게 하면 학생들은 현재 도구가 구식이 되고 새로운 도구가 도입되었을 때 이러한 기본 지식을 활용할 수 있습니다.
However, one of the most compelling arguments for the implementation of AI training in medical education is that this training will augment existing curriculum rather than replace existing coursework. When students are trained to use AI tools, focus should shift from acquiring basic knowledge on how to use the tool to a basic understanding of the underlying principles. This will enable the students to use this fundamental knowledge when current tools get outdated and new tools are introduced.
또 다른 현실적인 문제는 기존의 의료 교육이 주로 주치의와 레지던트 또는 의대생 간의 상호작용을 중심으로 이루어졌다는 점입니다. AI가 임상 진료에 점점 더 많이 도입되면 문제가 될 수 있습니다. 많은 시니어 의사들은 AI에 대한 경험이 거의 없거나 전혀 없습니다. AI 교육은 평생 의학 교육(CME) 프로그램을 통해 제공될 수 있으며, 의료계 외부의 교육자에게도 교육을 받아야 할 수 있습니다. 예를 들어, AI와 임상 진료의 미래에 관한 2학점짜리 CME 과정은 전산 생물학자와 비즈니스 경제학자가 제공합니다[73].
Another practical problem is that traditional medical training revolves mainly around the interactions between an attending physician and the residents or medical students. When AI is increasingly introduced into clinical practice, this could be problematic. Many senior physicians have little to no experience with AI. AI training could be delivered via Continuing Medical Education (CME) programs and might need to be also taught by educators from outside the medical community. For example, a 2-credit CME course on AI and the Future of Clinical Practice is delivered by a computational biologist and business economists [73].
권장 사항
Recommendations
프레임워크
Framework
암기 위주의 전통적인 의학 커리큘럼은 정보화 시대에서 AI 시대로의 전환을 따라야 합니다. 미래의 의사들은 점점 더 다양한 출처의 정보를 효과적으로 통합하고 활용할 수 있는 역량을 배워야 합니다[56]. 이러한 지식을 의학에 포함시키기 위해서는 교육 초기부터 이러한 개념을 도입하는 것이 필수적입니다. 많은 국가에서 의과대학에 입학하기 위해서는 의과대학 입학시험(MCAT)을 치러야 합니다. 예를 들어, 현재 미국 MCAT 시험은 생물학, 화학, 물리학, 심리학, 사회학 및 추론에 중점을 둡니다 [78]. 이러한 시험은 선형 대수 및 미적분의 기초와 같은 수학적 개념에 대한 테스트를 시작할 수 있습니다. 이러한 개념은 AI에 대한 기초적인 이해에 필수적이며 나머지 커리큘럼의 분위기를 조성합니다.
The traditional medical curriculum, which is mostly memorization based, must follow the transition from the information age to the age of AI. Future physicians have to be taught competence in the effective integration and utilization of information from a growing array of sources [56]. To embed this knowledge into medicine, it is of the essence to start introducing these concepts from the beginning of training. In many countries, a Medical College Admission Test (MCAT) has to be taken to be admitted into medical school. The current US MCAT exam, for example, focuses on biology, chemistry, physics, psychology, sociology, and reasoning [78]. These exams could start testing on mathematical concepts such as basis of linear algebra and calculus. These concepts are vital to the elementary understanding of AI and will set the tone for the rest of the curriculum.
전임상 교육 과정의 핵심 단계에서는 의료 데이터 큐레이션 및 품질[79], 출처[80], 통합[81], 거버넌스, EHR[60], AI 기초, AI의 윤리 및 법적 문제[82,83]를 다루는 데 시간을 할애해야 합니다. AI 및 로봇 기술에 대한 비판적 평가와 통계적 해석에 대한 교과목도 중요합니다[84].
- 첫째, 이러한 과목은 현재 응용 프로그램이 구식이 된 후에도 사용할 수있는 이러한 과목의 기초에 대해 가르치기 위해 독립된 과정으로 가르 칠 수 있습니다 [68]. 이러한 독립적인 과목은 현재 커리큘럼에서 의료 정보학 및 통계학 과목을 대체하고 보완할 수 있습니다.
- 둘째, 임상 과정에서도 반복하여 학생들이 AI의 임상 적용에 익숙해지고 다양한 환경에서 EHR과 함께 작업할 수 있도록 해야 합니다[68].
In the core phase of preclinical didactics, time should be devoted to working with health data curation and quality [79], provenance [80], integration [81], and governance, working with EHRs [60], AI fundamentals, and ethics and legal issues with AI [82,83]. Course work in critical appraisal and statistical interpretation of AI and robotic technologies is also important [84].
- First, these subjects could be taught in self-contained courses to teach about the fundamentals of these subjects that can be used even after current applications become outdated [68]. These self-contained courses could potentially replace and augment courses on medical informatics and statistics in the current curriculum.
- Second, they should also recur in clinical courses to familiarize students with the clinical applications of AI and work with EHRs in diverse settings [68].
AI를 도입하는 한 가지 접근 방식은 근거 기반 의학[85]과 같은 과목에서 이 기술을 통합하는 것입니다. 학생은 PubMed와 같은 데이터베이스나 진단 테스트 또는 체계적 문헌고찰을 통해 증거를 평가하는 방법을 배우게 되는데, 이 과정은 데이터 과학의 개념을 적용하고 NLP와 같은 AI 기술을 적용하고 시나리오를 분석하여 윤리 및 책임 문제에 대해 테스트함으로써 강화될 수 있습니다[86]. 또한, 학생들은 컴퓨터 및 소프트웨어 공학의 기초에 대한 교육을 받아 실제 AI 애플리케이션의 의미를 이해해야 합니다. 예를 들어, 하드웨어 및 소프트웨어 개발과 사용자 경험 설계의 기초도 유용할 수 있습니다.
An approach to introducing AI could be to incorporate this technology during courses such as Evidence Based Medicine [85]. As the student is taught to appraise evidence through databases such as PubMed or diagnostic tests or systematic reviews, this process could be augmented by applying concepts from data sciences, applying AI technologies such as NLP and analyzing scenarios to test them on questions of ethics and liability [86]. In addition, the students should also be trained in the fundamentals of computer and software engineering to understand the semantics behind real-world AI applications. For example, basics of hardware and software development and user experience design may also be valuable.
임상 로테이션과 레지던트 기간 동안에는 AI를 실제로 적용하는 데 초점을 맞춰야 합니다. 디지털 바이오마커[87]와 디지털 치료법[88]이 발전함에 따라 학생들도 AI에 의존하는 이러한 기술에 대한 교육을 받아야 합니다. 이러한 기술은 가까운 미래에 가정 내 환경에서 대규모 진단과 치료를 가능하게 할 잠재력을 가지고 있습니다[89]. 교육이 끝나면 USMLE는 최종 시험에 데이터 과학 및 AI 기초에 관한 상당수의 문제를 포함시켜야 합니다. 의료 전문가들이 최신 개발 동향을 파악할 수 있도록 의료 AI 관련 컨퍼런스에 참석하도록 인센티브를 제공할 수 있습니다. 참석하는 의사를 위해 AI 및 데이터 과학에 대한 광범위한 과정이 CME의 일부가 되어야 합니다. 자세한 내용은 표 2를 참조하십시오.
During clinical rotations and residency, focus should shift toward relevant applications of AI in practice. With advancements in digital biomarkers [87] and digital therapeutics [88], students should also be trained in these technologies as they rely on AI. They have the potential to enable large-scale diagnostics and treatments in in-home environments in the near future [89]. At the end of training, the USMLE should include a substantial number of questions on data science and AI fundamentals in their final exams. Attendance of conferences on health care AI could be incentivized, so that health care professionals stay up-to-date with the latest developments. For attending physicians, extensive courses on AI and data science should be part of CME. See Table 2 for more details.
Program | Faculty; organization | Number of Continuing Medical Education credits |
Artificial Intelligence and the Future of Clinical Practice [73] | Computational biologist, Business economist; Massachusetts Medical Society | 2.0 |
Intro to AI and Machine Learning: Why All the Buzz [74] | Medical Informatics, Radiology; The Radiological Society of North America | 1.0 |
Current Applications and Future of Cardiology [75] | Healthcare Technologists, Bioinformatics, Cardiology; Mayo Clinic | 10.0 |
Artificial Intelligence and Machine Learning: Application in the Care of Children [76] | Pediatric Medicine; University of Pittsburgh School of Medicine | 1.0 |
Artificial Intelligence in Healthcare: The Hope, The Hype, The Promise, The Peril [77] | Medical Informatics, Business Administration; Stanford University School of Medicine | 6.0 |
보다 균형 잡힌 미래의 의사를 양성하기 위해 AI 기술은 비분석 및 사람 중심의 의료 측면과도 균형을 이루어야 합니다. 의사 소통, 공감, 공동 의사 결정, 리더십, 팀 구축, 창의성과 같은 다른 기술도 의사에게 계속 중요해질 기술입니다. 텍사스 대학교 오스틴 캠퍼스의 Dell 의과 대학에서는 리더십, 창의성, 커뮤니케이션과 같은 소프트 스킬 교육에 집중하기 위해 기초 과학 커리큘럼의 기간을 단축했습니다[63].
AI skills must also be balanced with nonanalytics and person-centered aspects of medicine to develop a more rounded doctor of the future. Other skills such as communications, empathy, shared decision making, leadership, team building, and creativity are all skills that will continue to gain importance for physicians. At the Dell Medical School at the University of Texas, Austin, the curriculum in basic sciences has been reduced in duration to accommodate training in soft skills such as leadership, creativity, and communication [63].
임상의가 혁신적으로 사고하고 기술 기반 치료 모델을 만들 수 있도록 하려면 구현 과학, 운영 및 임상 정보학에 대한 다학제적 교육이 필요합니다. 스탠포드 의과대학은 대학원 의학교육에 인간 중심의 설계 접근 방식을 도입하여 디지털 미래를 위한 임상의사 혁신가를 양성하는 프로그램을 만들었습니다[90]. 보스턴 매사추세츠 종합병원의 헬스케어 혁신 연구소에서는 레지던트 수련생에게 데이터 과학, 머신러닝, 의료 운영, 서비스, 디자인 사고, 지적 재산권, 기업가 정신 등의 주제를 다루는 의료 혁신 분야의 1년 펠로우십을 제공합니다[91]. 이러한 프로젝트는 새로운 개발이며 의학교육에 AI를 도입하기 위해 취한 첫 번째 단계입니다.
To enable clinicians to think innovatively and create technology-enabled care models, multidisciplinary training is needed in implementation science, operations, and clinical informatics. The Stanford medical school has created such a program to train clinician-innovators for the digital future by introducing a human-centered design approach to graduate medical education [90]. At the Healthcare Transformation Laboratory at Massachusetts General Hospital in Boston, a 1-year fellowship is offered in health care innovation exposing resident trainees to topics in data sciences, machine learning, health care operations, services, design thinking, intellectual property, and entrepreneurship [91]. These projects are new developments and are the first steps taken to introduce AI in medical education.
첫 단계
First Steps
이러한 모든 개입을 동시에 도입할 수는 없으므로 향후 몇 년 동안의 토대를 마련할 몇 가지 첫 단계를 제안합니다. 먼저 의학전문대학원입학자격시험의 수학 영역과 유사한 수학적 개념에 관한 문제를 MCAT에 도입하는 것부터 시작할 것을 제안합니다. 의학교육의 핵심 단계에서 데이터 과학 및 AI 기초에 대한 고품질 웹 기반 강좌를 자유롭게 제공해야 합니다. 이를 통해 학생들은 다음 수련 과정에서 자연스럽게 이러한 과목을 응용하는 데 집중할 수 있습니다.
As not all of these interventions can be introduced simultaneously, we suggest a few first steps that will lay the foundation for the upcoming years. We suggest to start off by introducing questions on mathematical concepts into the MCAT similar to the mathematics section in the Graduate Record Examination. High quality Web-based courses on data sciences and AI fundamentals should be freely offered in the core phase of medical education. This might lead to students focusing on applications of these subjects more naturally in following years of training.
이미 이 교육 단계를 마친 레지던트와 의대생에게는 남은 의학교육 기간 동안 기초 과목에 대한 강좌가 제공되고 필수로 이수해야 합니다. 새로운 기술을 활용한 진료 모델을 만드는 데 관심이 있는 학생의 경우, 임상 기간 중 또는 레지던트 졸업 후 갭이어 기간 동안 의료 혁신에 대한 전문 교육을 받을 수 있도록 장려해야 합니다. 현직 의사를 위한 입문 과정과 재교육 과정도 제공되어야 합니다. 특히 이 그룹은 향후 의대생과 레지던트에게 이러한 주제에 대해 교육하는 임무를 부분적으로 다시 맡을 수 있도록 광범위한 교육이 필요합니다. 표 3에는 의학교육의 다양한 단계에 추가할 수 있는 권장 콘텐츠가 나열되어 있습니다. 표 4에는 의사와 수련의가 이 기술과 의료 분야에서의 적용에 대해 자세히 알아보기 위해 참석할 수 있는 의료 컨퍼런스에서 빠르게 진화하는 AI의 일부가 나열되어 있습니다.
For residents and medical students who have already finished this phase of training, courses on the fundamental subjects should be available and mandatory throughout the remaining part of their medical education. For students interested in creating new technology-enabled care models, dedicated training in health care innovation during a gap year during the clinical years or after residency should be encouraged. For attending physicians, introductory courses and refresher courses should also be made available. Extensive training is especially necessary for this group so that they can partly take back the task of educating medical students and residents on these subjects in the future. Table 3 lists suggested content that can be added to the various phases of medical education. Table 4 lists a small subset of rapidly evolving AI in health care conferences that physicians and trainees can attend to learn more about this technology and its applications in health care.
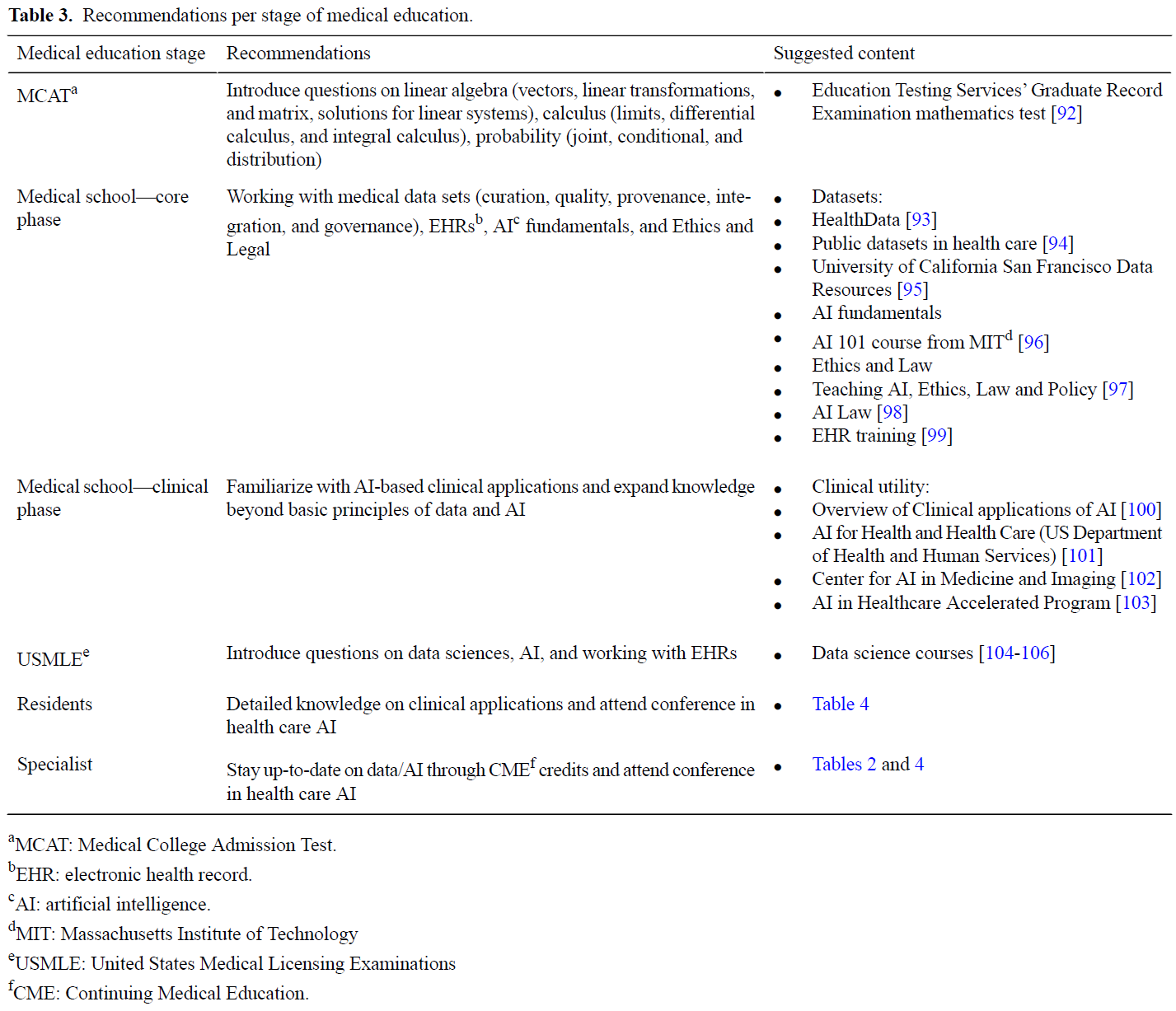
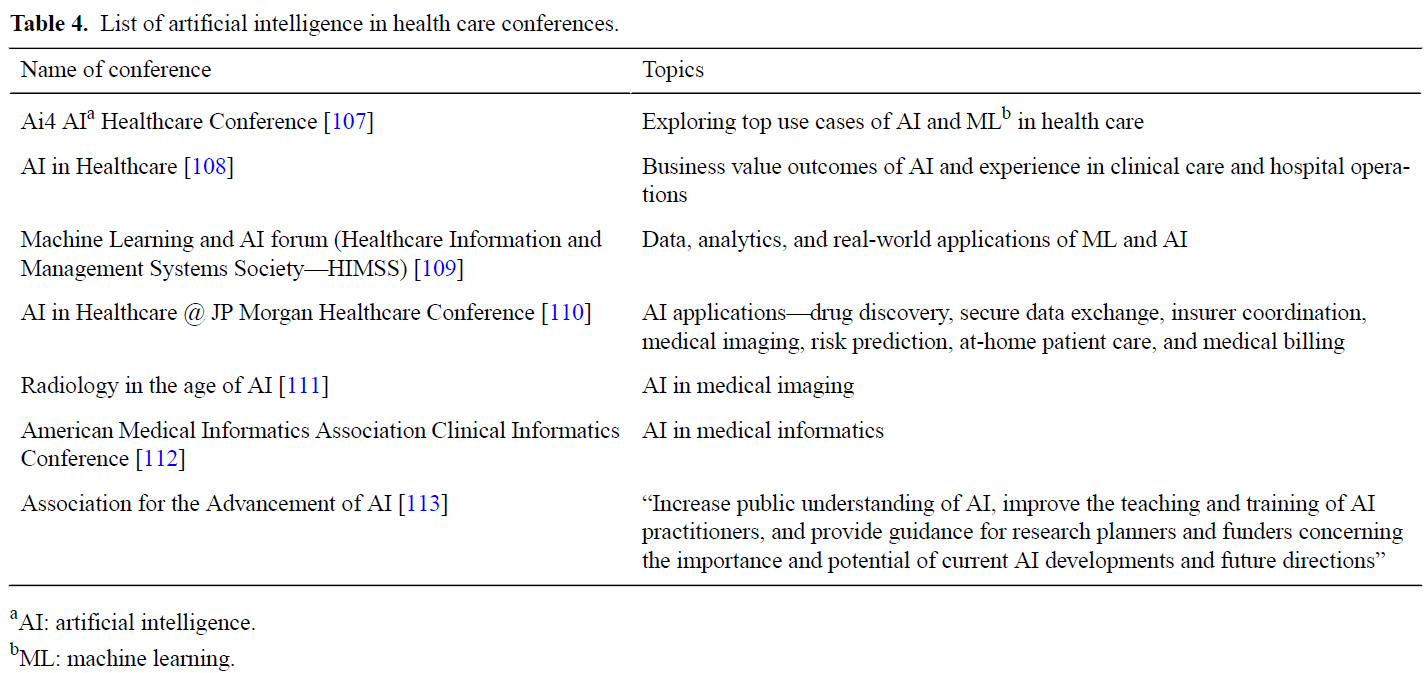
결론
Conclusions
의사와 기계의 협업은 임상 의사 결정과 환자 건강 결과를 개선할 수 있는 가장 큰 잠재력을 가지고 있습니다[114]. AI는 의료 기록, 유전자 보고서, 약국 기록, 환경 데이터 등 더 많은 데이터를 큐레이션하고 처리하여 더 많은 의료 정보를 보유, 액세스, 분석할 수 있습니다. 그러나 돌봄의 기술을 대체할 수는 없습니다. AI와 그 응용이 의료 분야에서 주류가 되면서 의대생, 레지던트, 펠로우, 개업의는 AI, 데이터 과학, EHR 기본 사항, AI와 관련된 윤리 및 법적 문제에 대한 지식을 갖춰야 합니다. 의과대학은 이러한 내용을 커리큘럼의 일부로 포함시켜야 합니다. 의대생이 의사가 되기까지 단계적으로 교육하는 것이 좋습니다.
Physicians and machines working in combination have the greatest potential to improve clinical decision making and patient health outcomes [114]. AI can curate and process more data such as medical records, genetic reports, pharmacy notes, and environment data and in turn retain, access, and analyze more medical information. However, it cannot replace the art of caring. As AI and its application become mainstream in health care, medical students, residents, fellows, and practicing physicians need to have knowledge of AI, data sciences, EHR fundamentals, and ethics and legal issues concerning AI. Medical schools will need to include them as part of the curriculum. A staged approach to educating the medical student through their journey is recommended.
AI는 더 빠르고 정확한 진단을 가능하게 하고, 영상의학을 보강하며, 인간의 피로로 인한 오류를 줄이고, 의료 비용을 절감하며, 지루하고 반복적이며 노동 집약적인 작업을 보조 및 대체하고, 최소 침습 수술을 가능하게 하며, 사망률을 감소시킬 것입니다.
AI will enable faster and accurate diagnosis, augment radiology, reduce errors due to human fatigue, decrease medical costs, assist and replace dull, repetitive, and labor-intensive tasks, minimally invasive surgery, and reduce mortality rates.
2022년까지 전 세계 의료비 지출이 미화 10조 달러에 달할 것으로 예상되는 가운데, AI는 환자 경험 향상, 인구 건강 개선, 비용 절감, 의료진 경험 개선이라는 의료 분야의 네 가지 목표를 달성할 수 있는 귀중한 잠재력을 가지고 있습니다[115,116].
With the global health care expenditure projected to reach US $10 trillion by 2022, AI has the invaluable potential to advance the quadruple aim in health care—enhance the patient experience, improve population health, reduce costs, and improve the provider experience [115,116].
JMIR Med Educ. 2019 Dec 3;5(2):e16048. doi: 10.2196/16048.
Introducing Artificial Intelligence Training in Medical Education
PMID: 31793895
PMCID: PMC6918207
DOI: 10.2196/16048
Free PMC article
Abstract
Health care is evolving and with it the need to reform medical education. As the practice of medicine enters the age of artificial intelligence (AI), the use of data to improve clinical decision making will grow, pushing the need for skillful medicine-machine interaction. As the rate of medical knowledge grows, technologies such as AI are needed to enable health care professionals to effectively use this knowledge to practice medicine. Medical professionals need to be adequately trained in this new technology, its advantages to improve cost, quality, and access to health care, and its shortfalls such as transparency and liability. AI needs to be seamlessly integrated across different aspects of the curriculum. In this paper, we have addressed the state of medical education at present and have recommended a framework on how to evolve the medical education curriculum to include AI.
Keywords: algorithm; artificial intelligence; black box; continuing education; curriculum; data sciences; deep learning; machine learning; medical education.
©Ketan Paranjape, Michiel Schinkel, Rishi Nannan Panday, Josip Car, Prabath Nanayakkara. Originally published in JMIR Medical Education (http://mededu.jmir.org), 03.12.2019.
'Articles (Medical Education) > 교육과정 개발&평가' 카테고리의 다른 글
연구-바탕 학습이 효과적인가? 사회과학에서 사전-사후 분석(STUDIES IN HIGHER EDUCATION, 2021) (0) | 2023.08.03 |
---|---|
의학교육커리큘럼에서 인공지능의 필요성, 도전, 적용(JMIR Med Educ. 2022) (0) | 2023.07.26 |
인공지능이 의학교육에 갖는 함의 (Lancet Digit Health. 2020) (0) | 2023.07.07 |
의학교육은 정보시대에서 인공지능시대로 옮겨가야 한다(Acad Med, 2018) (0) | 2023.07.07 |
사회적 책무성 프레임워크와 그것이 의학교육과 프로그램 평가에 갖는 함의: 내러티브 리뷰(Acad Med, 2020) (0) | 2023.07.07 |