일반화가능도 이론 간단히: G-studies를 위한 프라이머(J Grad Med Educ, 2019)
Generalizability Theory Made Simple(r): An Introductory Primer to G-Studies
Sandra Monteiro, PhD (@monteiro_meded) Gail M. Sullivan, MD, MPH (@DrMedEd_itor) Teresa M. Chan, MD, MHPE (@TChanMD)
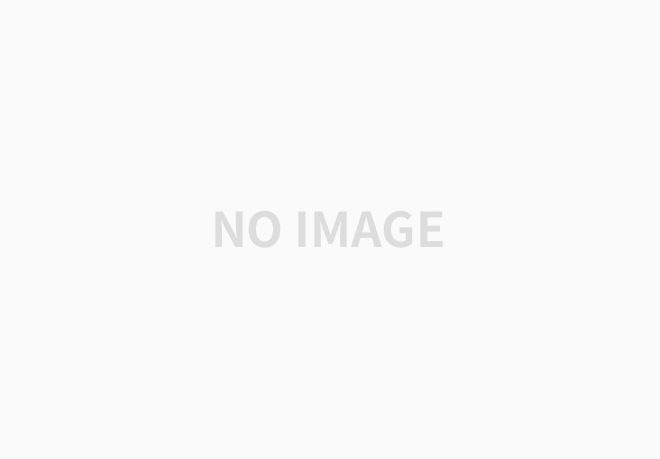
다음과 같이 진행된 사건을 상상해 보십시오.
- 교육생의 소생술을 평가하기 위해 6개 스테이션 시뮬레이션 목표 구조 임상 검사(OSCE)를 설계했습니다.
- 각 스테이션에서 연습생은 시나리오와 관련된 전문성, 커뮤니케이션, 리더십 및 기술력에 대해 평가받는다.
- 이러한 개별 점수는 평균화되어 각 스테이션에 대해 단일 점수를 만듭니다.
- 평가자가 교육생을 평가하는 방법에 있어 교육생의 성별이 역할을 할 수 있다는 점을 인지하고, OSCE의 신뢰성과 차이의 원인을 검토하여 성별 효과가 역량 결정을 유도하지 않음을 확인하고자 합니다.
Imagine the sequence of events:
- You have designed a 6-station simulation objective structured clinical examination (OSCE) to assess the resuscitation skills of your trainees.
- At each station trainees are assessed on professionalism, communication, leadership, and technical skills relevant to the scenario.
- These individual scores are averaged to create a singular score for each station.
- Cognizant that gender of the trainee may play a role in how raters assess trainees, you wish to examine your OSCE for reliability and sources of variance, to ensure that gender effects are not driving competency decisions.
결정을 뒷받침할 수 있는 타당한 증거를 어떻게 수집할 수 있습니까?
How can you gather validity evidence to support your decisions?
G-이론은 정확히 무엇이며 이것은 믿을 만한 접근법인가?
What exactly is G-theory and is this a credible approach?
임상 역량 측정
Measuring Clinical Competence
역량 기반 의료 교육(CBME) 시대에 우리는 점점 더 우리의 평가 프로세스를 기반으로 의사 결정을 내리게 되고, 이것은 우리의 평가의 신뢰성과 타당성을 보장할 필요가 있다.1-3 우리는 점수에 의존하여 실력에 따라 연습생들을 구별할 수 있는가? 그 과정을 믿을 수 있을까? 관련 구성(즉, 임상 역량의 특정 측면)을 측정하기 위해 새로운 평가를 작성하거나 오래된 평가를 수정할 때, 우리는 우리의 평가 데이터가 의사결정에 대한 타당성을 유지하는지 결정해야 한다.
- 모든 평가는 측정 도구로 간주될 수 있으므로, 우리는 타당성을 검사하기 위해 측정 원칙을 적용할 수 있다.3
- 타당성은 측정 도구의 안정적인 특성이 아니기 때문에 측정 오류를 잠재적으로 야기하는 다수의 구성 관련 요인에 의해 위협받을 수 있다.3
- 마찬가지로 신뢰성은 측정 도구의 안정적인 특성이 아니며 역량 기반 평가의 맥락 변화에 민감하다.
In the age of competency-based medical education (CBME), we increasingly make decisions based on our assessment processes, which necessitate ensuring the reliability and validity of our assessments.1–3 Can we rely on the score to discriminate between trainees based on competence? Can we trust the process? When we create new or modify older assessments to measure relevant constructs (ie, specific aspects of clinical competence), we must determine whether our assessment data maintain validity for decision-making.
- Any assessment can be considered a measurement tool; thus, we can apply measurement principles to examine validity.3
- As validity is not a stable characteristic of any measurement tool, it can be threatened by a multitude of construct irrelevant factors that potentially introduce measurement error.3
- Reliability, similarly, is not a stable characteristic of any measurement tool, and is sensitive to changes in context within competency-based assessments.
측정 오차를 줄이는 방법에 대한 문헌 검토를 수행하면 어지럽게 많은 연구 설계 및 분석 접근법이 나타납니다. 이러한 접근법 중 독자는 측정 신뢰성을 검토하기 위한 크론바흐의 알파에 익숙할 가능성이 높다. 크론바흐의 알파를 계산하면 시험 점수의 신뢰도를 알 수 있지만, [체계적인 평가자 편향이 점수에 영향을 미쳤는지를 보여주지는 않는다]. 즉, trainee의 성별이 평가자가 내린 수행능력 평가에 영향을 주었더라도, 우리는 크론바흐의 알파만으로는 이것을 발견할 수 없었다.3
If you conduct a literature review regarding methods to reduce measurement error, you will find a dizzying multitude of study designs and analysis approaches. Of these approaches, readers are likely familiar with Cronbach's alpha to examine measurement reliability. A calculation of Cronbach's alpha can inform test score reliability, but not whether systematic rater bias has an influence on scores. That is, if trainee gender influenced a rater's assessment of performance, we could not discover this from Cronbach's alpha alone.3
신뢰성, 타당성, 연구 설계 요소, 데이터 분석을 알려주는 매우 유용한 이론이 일반화 가능성 이론(G-이론)이다.3-6 G이론은 다양한 관찰이나 등급의 신뢰성을 검토, 결정 및 설계하기 위한 통계적 프레임워크이다.3–6
A highly useful theory that informs reliability, validity, elements of study design, and data analysis is Generalizability theory (G-theory).3–6 G-theory is a statistical framework for examining, determining, and designing the reliability of various observations or ratings.3–6
G 이론을 사용하여 우리는 평가 점수의 구성을 더 잘 이해하기 위해 일반화 가능성 연구(G-studies)를 설계할 수 있다(즉, OSCE 종료 시 얻는 실제 점수에 기여하는 것). 그런 다음 서로 다른 조건에서 수집된 동일한 데이터의 신뢰성을 예측하는 데 도움이 되는 의사 결정 연구(D-Studies)를 설계할 수 있다.
Using G-theory we can design Generalizability studies (G-studies) to better understand the composition of assessment scores (ie, what contributes to the actual score that you get at the end of an OSCE). We can then design Decision studies (D-studies) to help predict the reliability of the same data collected under different conditions.
수행능력 기반 평가에서 우리는 평가 점수(예: 비율 편향, 항목 또는 스테이션의 상대적 난이도, 평가자 또는 검사자의 주의력 또는 기분, 표준화된 환자의 능력 및 전체 환경)에 대한 잠재적 영향을 고려해야 한다.3.7
- G-이론은 이러한 요소들에 의해 야기되는 분산을 정량화하는 방법을 제공한다. 3,5,6
- 주어진 면facet의 각 형태form를 조건condition이라고 한다.3
- 우리의 예인 vignette에서, 연습생은 면facet이고 그들의 성별은 조건condition이다. (그리고 이 예에서는, 우리는 단지 2개의 성별만을 가정한다.) 위의 예제를 사용하여 표 1의 정의로 보완된 중요한 용어와 개념을 검토해보자.
In performance-based assessments we need to consider potential influences on assessment scores, such as rater bias, relative difficulty of items or stations, the rater's or examinee's attention or mood, the abilities of standardized patients, and the overall environment.3,7
- G-theory offers a way to quantify the variance contributed by these factors, which G-theory refers to as facets.3,5,6
- Each form of a given facet is called a condition.3
- In our example vignette, the trainees are a facet and their gender is a condition (and for this example, we assume only 2 genders). Let's use the above example to review important terminology and concepts, which are supplemented by definitions in table 1.
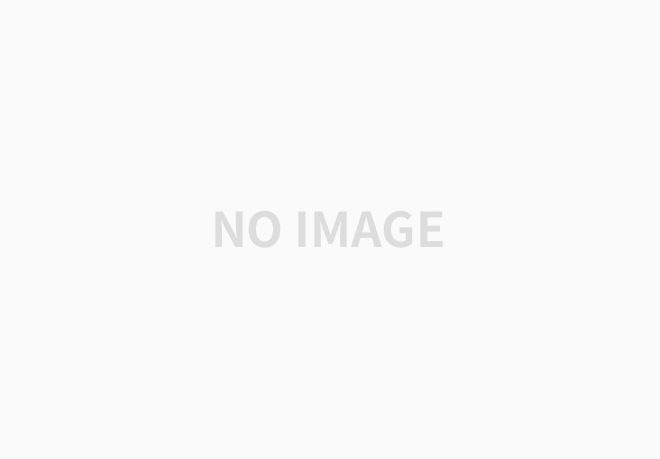
예: G이론의 평가 적용
Example: Application of G-Theory to Assessment
표준 OSCE 설계는 동일한 구조(즉, 임상 기술)의 반복 측정이 있기 때문에 G-연구에 이상적이다. 이는 절단을 하기 전에 측정 테이프를 사용하여 일부 목재(또는 다른 측정 테이프를 사용하는 5명의 평균 측정)를 여러 번 측정하는 것과 유사하다. 동일한 구조의 반복 측정값을 수집하면 신뢰성이 향상되는 것으로 나타났다. 이는 [랜덤 분산]이 동일한 구조의 여러 측정에서 cancel out될 수 있기 때문입니다. 그러나 측정 오류의 [체계적인 원인]이 남아 있을 수 있습니다. 예를 들어, 평가자 인식 문헌은 다른 종류의 평가자 편향과 평가 점수와 결정에 미치는 영향의 예들로 가득 차 있다.8
A standard OSCE design is ideal for a G-study because there are repeated measurements of the same construct (ie, clinical skills), much like we might use a measuring tape to measure some lumber several times before making a cut (or better yet, taking the average measurement from 5 people using different measuring tapes). Collecting repeated measures of the same construct has been shown to improve reliability. This is because random variance can cancel out in multiple measurements of the same construct. But there may remain systematic sources of measurement error. For example, the rater cognition literature is filled with examples of different kinds of rater bias and its impact on assessment scores and decisions.8
실험 설계에서 초점은 [그룹 간의 실제 차이를 찾기 위해 모든 유형의 오류를 최소화]하는 데 있다.3,9 G-연구에서 목표는 중요한 측정을 신뢰할 수 있는지 여부를 결정하기 위해 오류 소스(분산이라고 함)를 강조하는 것이다.3 우리의 vignet에서, 6개의 모든 스테이션의 점수는 전체 OSCE의 총 점수를 결정하기 위해 결합되거나 평균적이다.10 복수의 스테이션과 평균 OSCE 점수를 사용하는 것은 평가자에 의해 발생한 측정 오류를 처리하는 방법이다.8 그러나 스테이션 점수는 총점에 대한 차이를 유발합니다. 각 스테이션은 더 또는 덜 lenient하고, 점수에 고유한unique 영향을 미치며, G 이론을 사용하여 이러한 기여도를 정량화할 수 있다.
In experimental designs the focus is on minimizing error of all types to find true differences between groups.3,9 In G-studies the goal is to highlight sources of error (called variance) in order to determine if we can trust the critical measurement.3 In our vignette, all 6 stations' scores are combined or averaged to determine the total score for the entire OSCE.10 The use of multiple stations and an average OSCE score is a way to deal with measurement error introduced by raters.8 However, each station score contributes variance toward the total score. Each station is more or less difficult or each rater is more or less lenient and has unique influences on the trainee's score; rather than ignore them, using G-theory we can quantify these contributions.
그림은 이 개념을 보여준다. G이론은 우리에게 레시피를 개발할 수 있게 해준다. 분산은 파이의 한 조각에서 성분의 분포와 같습니다.
- 때로는 분산이 examinee에게 유리하게 작용할 수도 있고, 때로는 분산이 연습생에게 불리하게 작용할 수 있습니다.
- 때로는 분산이 예측 가능한 방법(체계적인 오류)으로 작용하고 다른 때에는 예측할 수 없는 방법(랜덤 오차)으로 작용합니다.3
신뢰도를 완전히 평가하기 위해서는 이러한 분산 측면을 고려하는 것이 필수적이며, 이는 궁극적으로 평가의 유효성(정확도)에 대한 상한을 설정한다.3
The figure demonstrates this concept. G-theory allows us to develop a recipe. Variance is like the distribution of ingredients in a single slice of pie;
- sometimes the variance works in an examinee's favor, and sometimes the variance may work against the trainee.
- Sometimes the variance acts in predictable ways (systematic error) and other times in unpredictable ways (random error).3
It is essential to consider these aspects of variance in order to fully evaluate reliability, which ultimately sets an upper limit on the validity (accuracy) of the assessment.3
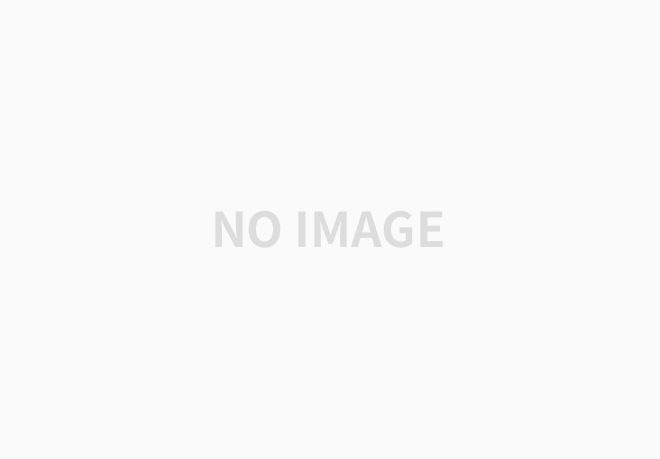
우리의 vignette에서, 우리는 G-이론을 사용하여 전문성, 의사소통, 리더십, 그리고 기술 능력의 차이를 고려할 수 있다. 그러나 G 이론의 진짜 힘은 연습생의 성별과 같은 추가적인 분산 원인도 고려할 수 있다는 것이다. 관심이 있다면 하루 중 시간, 다른 표준화된 환자 또는 OSCE 관측소의 수와 같은 분산 원인을 추가할 수 있습니다. 표 2는 vignette의 다양한 면facets을 추적하여, 다양한 용도로 평가할 수 있는 방법을 보여줍니다.
In our vignette, we could use G-theory to consider the variance among scores of professionalism, communication, leadership, and technical skills. However, the real power of G-theory is that we can also consider additional sources of variance, such as the gender of the trainee. We could, if we were interested, add in sources of variance like the time of day, different standardized patients, or number of OSCE stations. Table 2 shows how we can keep track of various facets in our vignette to evaluate them for different purposes.
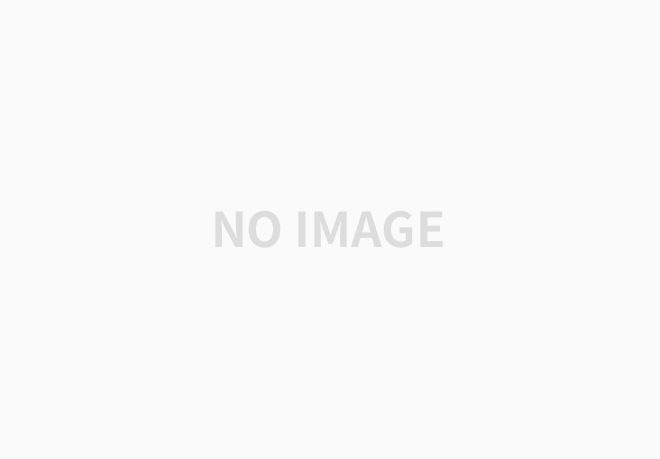
G- 연구를 진행하기 위해 [먼저 OSCE에서 소생 기술에 대해 가능한 모든 분산원sources of variance 또는 측면facets을 식별하고 그것들이 고정인지 무작위인지 여부를 결정]한다. G-Study를 수행할 모든 pieces를 확보했는지 판단하려면, 표 2와 같이 데이터가 가장 잘 정리되어 있습니다. 다시 이야기하자면, 우리는 (1) 소생술 OSCE에서 신뢰할 수 있는 평가 데이터를 수집했는지 여부 및 (2) 성별(임상 기술 평가와 무관해야 하는 요소)이 전체 평가에 영향을 미치는지 여부를 결정하는 데 관심이 있다.
To proceed with our G-study, we first identify all likely sources of variance or facets in the OSCE for resuscitation skills and determine if they are fixed or random. In order to ensure we have all the pieces to conduct a G-study, data are best organized as in table 2. As a reminder, we are interested in (1) Determining if we collected reliable assessment data in the resuscitation OSCE, and (2) Whether there is any indication that gender—a factor that should be irrelevant to the evaluation of a clinical skill—contributes any variance to the overall assessment.
일반화 가능성 이론 Generalizability Theory
"개인으로부터 측정되는 모든 측정은 가능한 측정의 우주에서 추출한 샘플로 간주됩니다."11
“Any one measurement from an individual is viewed as a sample from a universe of possible measurements.”11
G-이론에서 우리는 먼저 우리가 [일반화하고자 하는 점수와 면scores and facets의 우주]를 정의한다.
- G-연구에서 고려 중인 면이 고정인지 랜덤인지는 미리 결정되어 있다. 그런 다음 우리는 G-계수를 계산하기 위해 몇 가지 G- 연구를 수행한다. 계산된 각 G 계수는 측정 도구의 [특정 측면(예: 평가자간 신뢰성)의 신뢰성]을 평가한다.
- D-studies에서 우리는 facet의 레이블 변경의 영향을 평가할 수 있다(예컨대, fixed에서 random으로). 우리는 이러한 계산을 사용하여 유사한 평가 상황에서 퍼포먼스에 대한 예측을 할 수 있다. 예를 들어, 우리는 OSCE station 수를 줄임으로써 G-계수가 어떻게 영향을 받는지 물어볼 수 있다. 또는 station에 늘어난 평가자 수가 G-계수를 증가시킬 수 있는지 물어볼 수 있습니다.
In G-theory we first define the universe of scores and facets we wish to generalize from and to.
- In a G-study, the facets being considered are predetermined to be fixed or random. We then conduct several G-studies to calculate G-coefficients. Each calculated G-coefficient evaluates the reliability of a given aspect of the measurement tool, for example, interrater reliability.
- In D-studies we can evaluate the impact of changing a facet's label, such as from fixed to random. We can use these calculations to make predictions about performance in a similar assessment situation. For example, we can ask how the G-coefficient would be affected by reducing the number of OSCE stations. Or we can ask if multiple raters per station would increase the G-coefficient.
일반적으로 표 3에 표시된 것처럼 여러 스테이션이 신뢰성을 향상시킬 수 있지만 스테이션당 여러 레이터는 큰 영향을 미치지 않습니다.
Typically, as shown in table 3, multiple stations can improve reliability, but multiple raters per station do not have a big impact.
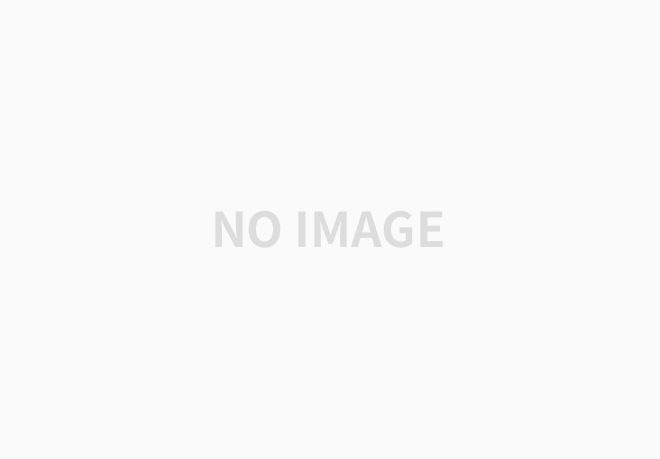
측정 도구의 신뢰도를 고려할 때, 우리는 다른 분산원이나 오차의 상관 관계를 설명하는 기본 공식으로 시작할 수 있다.3,6
When considering the reliability of a measurement tool, we can start with a basic formula to describe how different sources of variance or error relate.3,6
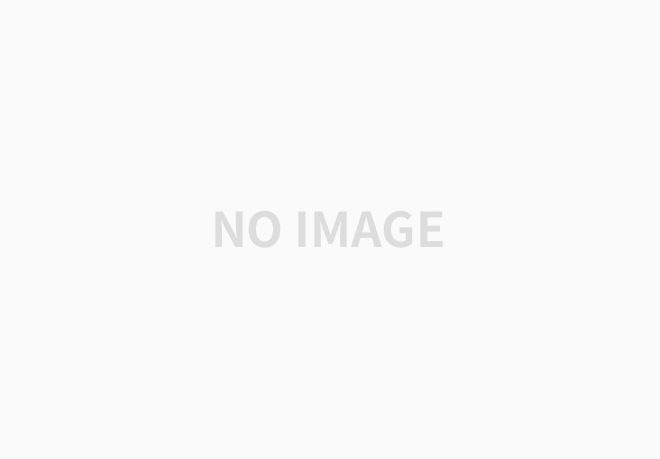
파이 비유를 사용하자면, 오류 분산(레시피)이 여러 성분(개별 성분)으로 구성되어 있다고 가정합니다. 다양한 오류 유발 면(예: 측정 컵 정밀도, 물 순도, 베이커리의 고도 등)에 의해 분산이 약간 변경될 수 있습니다. 이러한 면은 각각 결과 파이의 최종 구성 과정에서 오류의 몇 가지 요소를 도입introduce할 것이다.
Using the pie analogy, consider that Error variance (the recipe) is composed of multiple components (individual ingredients). The variance may be slightly altered by various error-inducing facets (eg, the measuring cup precision, water purity, altitude of the bakery, etc). These facets would each introduce some element of error in the final composition of the resulting pie.
우리의 vignette에서, 소생술 OSCE가 한 번에 수행된다고 가정할 때, facet은 연습생, 훈련생의 성별, station(레이터 포함)이 될 것이다. Trainee의 facet은 성별의 facet에 nested되어 있다. (station에 nested된) 평가자는 일반화generalization의 한 facet으로, 한 관측치 또는 한 관측치 또는 점수에서 다른 등급의 점수로 일반화할 수 있기를 희망하고 있다.
In our vignette, assuming that the resuscitation OSCE is conducted on one occasion, the facets would be the trainees, gender of the trainees, and stations (which include raters). The facet of trainee is nested in the facet of gender. Raters (nested in stations) are facets of generalization, as we hope to generalize from one observation or score at one station, or recorded by one rater, to another score from a different rater.
이 예에서 trainee는 facet of differentiation이다. 왜냐하면 OSCE가 측정한 기술 수준에 따라 개별 연습생 간의 differentiate를 하고 싶기 때문이다다. 이 면facets들은 이 연구에서 알려진 점수의 우주를 묘사한다.
- 향후 행정에서 OSCE station이나 시나리오가 결코 바뀌지 않을 경우, 우리는 스테이션의 facet이 고정된 것으로 간주할 수 있다.
- 마찬가지로, 매번 동일한 임상 교수진이 이 OSCE의 평가자로 작용할 것으로 예상한다면, 비율의 측면도 고정된 것으로 간주될 수 있다.
In this example, trainees are the facet of differentiation as we wish to differentiate between individual trainees based on their skill level as measured by the OSCE. These facets describe the known universe of scores in this study.
- If the OSCE stations or scenarios are never going to change in future administrations, we can consider the facet of station to be fixed.
- Similarly, if you foresee the same clinical faculty acting as raters for this OSCE every time, the facet of rater may also be considered fixed.
고부담 OSCE에서는 시험 보안상의 이유로 스테이션이 변경됨에 따라 [이러한 두 가지 측면이 모두 무작위적]이라는 점에 유의한다. 프로그램 평가에서, [교수진은 전담 평가 직원이 아니라 일반적으로 자원 봉사자이기 때문에 변경될 수도] 있습니다. Facet이 fixed인지 random인지 여부가 G 계수 계산에 포함되는 분산 성분을 변경합니다(표 4 참조).3–5
Note that in high-stakes OSCEs both of these facets are random as stations change for test security reasons. In program assessments, faculty also may change as they are typically volunteers and not dedicated assessment staff. Whether a facet is fixed or random changes what variance components are included in the calculation of the G-coefficient (see table 4).3–5
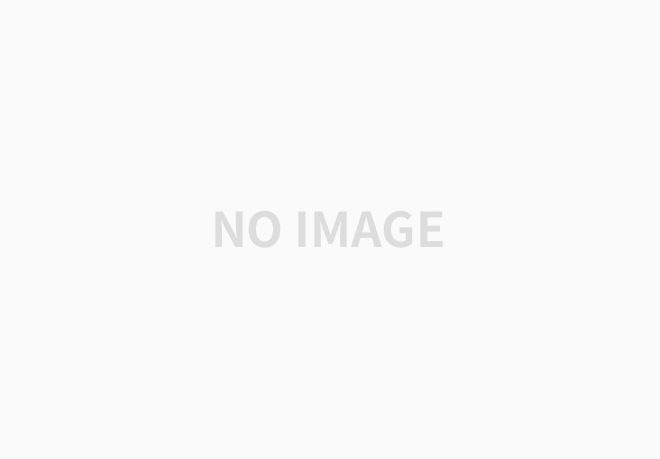
모든 소생술 OSCE 관리에서, 평균 OSCE 점수는 모든 개별 점수를 평가하는 우주 점수 역할을 합니다. G-연구는 표준 편차, 평균 제곱 오차 및 분산을 분산 분석(ANOVA)으로 결정하는 시작점이 같습니다.3-5 표준 분산 분석의 요인부터 시작하여 분산 성분 또는 G-연구의 면으로 계속합니다. 분산 분석ANOVA과의 차이점은 G-이론에서 우리는 그룹 간의 유의한 차이를 확립하는 데 관심이 있는 것이 아니라 오차 분산이 다양한 측면에 어떻게 분포되는지를 결정하는 데 관심이 있다는 것입니다. 목표는 고전적인 신뢰도 계수를 확장하여 측정 대상, 이 경우 연습생으로 인한 분산의 양을 설명하는 것이다. 이상적으로, 가장 큰 분산의 원천은 연습생 자신이며, 이는 능력의 개별적인 차이를 나타낼 것이다. 이러한 요인들이 임상적 역량에 대한 결정에 영향을 미치지 않아야 하기 때문에, 비율자나 성별과 같은 다른 측면에 기인하는 많은 양의 차이는 바람직하지 않다.
For any administration of the resuscitation OSCE, the average OSCE score acts as the universe score against which all individual scores are evaluated. G-studies have the same starting point for determining standard deviation, mean square error, and variance as an analysis of variance (ANOVA).3–5 We start with factors in a standard ANOVA, but then continue with the variance components, or facets in a G-study. The difference from an ANOVA analysis is that in G-theory we are not as concerned about establishing a significant difference between groups, but rather to determine how error variance is distributed among the various facets. The goal is to extend classical reliability coefficients to describe how much variance is due to the object of measurement, in this case the trainees. Ideally, the greatest source of variance is the trainees themselves, which would indicate individual differences in ability. Large amounts of variance attributed to raters or other facets such as gender are undesirable, as these factors should not influence decisions about clinical competence.
이상적으로, G-연구는 모든 변동 원인 또는 오차를 평가할 수 있습니다. 다만 assessment를 evaluation할 때의 한계는 [알 수 없는 분산 출처]에 대해서는 기여도를 추정할 수 없다는 것이다. 그러나 알려진 많은 중요 변수에 조명을 집중함으로써 무엇이 누락되었는지 이해하기 시작할 수 있습니다.
Ideally, G-studies would assess all sources of variance, or error. The limitation for any evaluation of an assessment is the inability to estimate contributions from unknown sources of variance. However, by focusing a light on as many known important variables, it is possible to begin to understand what may be missing.
다음 번에 G- 연구를 포함하는 기사를 읽을 때, 이 전략은 가장 큰 분산 원인이 (1)테스트 대상(평가를 통해 다양한 연습생 역량을 판단할 것이라는 기대)인지, 또는 (2) 평가자, 하루 중 시간, 테스트 상황 수 또는 기타 요인 등의 다른 요인 때문인지 여부를 결정하는 데 도움이 될 것이라는 점을 기억하십시오.
Next time you read an article that includes a G-study, remember that this strategy will help determine whether the largest source of variance was the subjects being tested—which we would expect in an assessment to determine different trainee competence—or due to other factors, such as the person rating the trainee, time of day, number of test situations, or other factors.
참고로 G 이론을 사용하여 측정 오류(박스)를 조사하는 3개의 문서를 나열합니다. 여러분만의 평가 프로그램을 만들 때, 여러분 자신의 측정의 신뢰성을 향상시킬 뿐만 아니라 여러분의 작업을 다른 사람들에게 전파할 때 이익을 더하기 위해 G-이론을 사용하는 것을 고려해보세요. G-이론과 신뢰성 연구에 대한 당신의 질문과 의견을 기대합니다.
For reference, we list 3 articles that use G-theory to examine measurement error (box). When creating your own assessment programs, consider using G-theory to understand the role of sources of variance, not only to enhance the reliability of your own measurements, but also to add benefit when disseminating your work to others. We look forward to your questions and comments about G-theory and reliability studies.
boxArticles Using Generalizability Study Design to Examine Test Properties
- Lang VJ, Berman NB, Bronander K, Harrell H, Hingle S, Holthouser A, et al. Validity evidence for a brief online key features examination in the internal medicine clerkship. Acad Med. 2019;94(2):259–266. doi:10.1097/ACM.0000000000002506.
- Monteiro S, Sibbald D, Coetzee K. i-Assess: evaluating the impact of electronic data capture for OSCE. Perspect Med Educ. 20181;7(2):110–119. doi:10.1007/s40037-018-0410-4.
- Lord JA, Zuege DJ, Mackay P, Roze des Ordons A, Jocelyn L. Picking the right tool for the job: a reliability study of 4 assessment tools for central venous catheter insertion. J Grad Med Educ. 2019;11(4):422–429.
J Grad Med Educ. 2019 Aug;11(4):365-370. doi: 10.4300/JGME-D-19-00464.1.
Generalizability Theory Made Simple(r): An Introductory Primer to G-Studies
Sandra Monteiro, Gail M Sullivan, Teresa M Chan
- PMID: 31440326
- PMCID: PMC6699529
'Articles (Medical Education) > 의학교육연구(Research)' 카테고리의 다른 글
문헌고찰: 양질의 의학교육연구를 위한 초석(J Grad Med Educ. 2016) (0) | 2021.08.25 |
---|---|
The Green Lumber Fallacy, Jingle-Jangle Fallacies (0) | 2021.05.13 |
설문 설계의 단계 따라가기: GME 연구 사례(J Grad Med Educ, 2013) (0) | 2021.05.06 |
N을 어떻게 할까? 포커스그룹 연구에서 샘플 사이즈 보고에 관한 방법론적 연구(BMC Med Res Methodol, 2011) (0) | 2021.05.06 |
질적 면담 연구에서 샘플 사이즈: 정보력에 의하여 (Qual Health Res, 2016) (0) | 2021.05.06 |