질적연구에서 포화를 위한 표본 수: 실증 시험의 체계적 문헌고찰(Soc Sci Med. 2022)
Sample sizes for saturation in qualitative research: A systematic review of empirical tests
Monique Hennink a,*, Bonnie N. Kaiser b
1. 서론
1. Introduction
[포화]는 질적 연구에서 목적적 표본의 적절성을 평가하기 위한 가장 일반적인 지침 원칙입니다(Morse, 1995, 2015; Sandelowski, 1995). 그러나 포화 평가에 대한 지침과 포화에 도달하는 데 필요한 표본 크기는 모호했습니다. 최근까지 포화는 다양한 유형의 정성적 데이터로 경험적으로 평가되지 않았습니다. 포화에 대한 경험적 평가에 대한 관심이 높아지면서 이 주제에 대한 연구가 많이 이루어졌고, 이를 종합하고 이를 통해 무엇을 배울 수 있는지 파악할 수 있는 적절한 시기가 되었습니다. 이 체계적인 검토에서는 정성적 연구에서 포화를 경험적으로 평가하는 연구를 식별하고, 포화에 필요한 표본 크기, 포화를 평가하는 데 사용되는 전략 및 이러한 연구에서 도출할 수 있는 지침을 확인하고자 했습니다.
Saturation is the most common guiding principle for assessing the adequacy of purposive samples in qualitative research (Morse, 1995, 2015; Sandelowski, 1995). However, guidance on assessing saturation and the sample sizes needed to reach saturation have been vague. Until recently, saturation had not been empirically assessed with different types of qualitative data. A growing interest in empirical assessment of saturation has now generated a body of research on the topic, making it an opportune time to synthesize it and identify what we can learn from it. This systematic review sought to identify studies that empirically assess saturation in qualitative research, to identify sample sizes needed for saturation, strategies used to assess saturation, and guidance we can draw from these studies.
포화의 개념은 Glaser와 Strauss(1967)에 의해 ['이론적 포화']로 개발되었으며, 질적 연구에 대한 영향력 있는 근거 이론 접근법의 일부였습니다. 근거 이론은 사회 현상을 설명하기 위해 텍스트 데이터로부터 사회학 이론을 개발하는 데 중점을 둡니다. 이 접근법에서 [이론적 포화]란 "이론적 구성에 대한 더 많은 데이터를 수집해도 새로운 속성이 드러나지 않고, 새로운 근거 이론에 대한 더 이상의 이론적 통찰을 얻지 못하는 지점"을 의미합니다(Bryant와 Charmaz, 2007, 611쪽). 따라서 데이터 수집에서 [중요한 이슈나 통찰력이 데이터에서 모두 소진되는 시점]은 이론을 구성하는 개념적 범주가 '포화 상태'에 이르렀음을 의미하며, 이는 새로운 이론이 포괄적이고 데이터에 근거한 이론이 될 수 있음을 의미합니다. 이론적 포화 상태는 샘플링, 데이터 수집, 데이터 분석이 동시에 이루어지는 반복적인 과정에도 내재되어 있으며(Sandelowski, 1995), 데이터는 포화 상태가 될 때까지 샘플링에 지속적으로 정보를 제공합니다.
The concept of saturation was developed by Glaser and Strauss (1967) as ‘theoretical saturation’ and was part of their influential grounded theory approach to qualitative research. Grounded theory focuses on developing sociological theory from textual data to explain social phenomena. Within this approach, theoretical saturation refers to “the point at which gathering more data about a theoretical construct reveals no new properties, nor yields any further theoretical insights about the emerging grounded theory” (Bryant and Charmaz, 2007 p.611). Thus, it is the point in data collection when all important issues or insights are exhausted from data, which signifies that the conceptual categories that comprise the theory are ‘saturated’, so that the emerging theory is comprehensive and well-grounded in data. Theoretical saturation is also embedded in an iterative process of concurrently sampling, collecting data, and analyzing data (Sandelowski, 1995), whereby data continuously inform sampling until saturation.
대부분의 질적 연구는 근거 이론 접근법을 따르지 않지만, 포화 개념은 다른 질적 연구 접근법에서 널리 사용되며, 일반적으로 '데이터 포화' 또는 '주제 포화'라고 불립니다(Hennink et al., 2017). 이러한 포화의 광범위한 적용은 이론적 포화에서와 같이 이론을 개발하기 위한 데이터의 적절성보다는 표본 크기를 평가하는 데 더 중점을 둡니다. 더 넓은 맥락에서 사용되는 포화는 데이터 수집에서 [추가적인 문제나 인사이트]가 발견되지 않고 [데이터가 반복]되기 시작하여 더 이상의 데이터 수집이 [중복되는 시점]을 의미하며, 이는 적절한 표본 크기에 도달했음을 의미합니다. 포화는 표본이 연구 대상 현상에 적합한지, 즉 수집된 데이터가 연구 대상 이슈의 다양성, 깊이, 미묘한 차이를 포착하고 있는지, 따라서 [내용 타당성]을 입증할 수 있는지를 나타내는 중요한 지표입니다(Francis et al., 2010). 포화에 도달하는 것은 데이터 수집을 강력하고 타당하게 만드는 데 도움이 되는 질적 연구의 중요한 구성 요소가 되었습니다(O'Reilly and Parker, 2013). 또한 포화는 "저자가 리뷰어와 독자에게 제공하는 질적 엄격성에 대한 가장 빈번한 보증"입니다(Morse, 2015, 587쪽). 이 리뷰에서는 [근거 이론]의 매개변수 외부에서 사용될 때 포화를 위한 적절한 표본 크기에 대해 알려진 바가 적기 때문에 더 넓은 맥락에서 포화에 초점을 맞춥니다.
Although most qualitative research does not follow a grounded theory approach, the concept of saturation is widely used in other approaches to qualitative research, where it is typically called ‘data saturation’ or ‘thematic saturation’ (Hennink et al., 2017). This broader application of saturation focuses more on assessing sample size rather than the adequacy of data to develop theory (as in theoretical saturation). When used in the broader context, saturation refers to the point in data collection when no additional issues or insights are identified and data begin to repeat so that further data collection is redundant, signifying that an adequate sample size is reached. Saturation is an important indicator that a sample is adequate for the phenomenon studied – that data collected have captured the diversity, depth, and nuances of the issues studied – and thereby demonstrates content validity (Francis et al., 2010). Reaching saturation has become a critical component of qualitative research that helps make data collection robust and valid (O’Reilly and Parker, 2013). Moreover, saturation is “the most frequently touted guarantee of qualitative rigor offered by authors to reviewers and readers" (Morse, 2015, p. 587). In this review, we focus on saturation in the broader context, since less is known about adequate sample sizes for saturation when used outside of the parameters of grounded theory.
질적 표본의 엄밀성을 뒷받침하는 포화의 중요성에도 불구하고, 발표된 질적 연구에서 표본 크기가 정당화되는 방식에 대한 투명성이 일관되게 부족합니다(Morse, 1995; Guest 외., 2006; Kerr 외., 2010; Carlsen and Glenton, 2011; Hennink 외., 2017). [포화는 적절한 표본 크기에 대한 가장 일반적인 근거로 인용]되지만(Morse, 1995, 2015), 포화를 평가한 방법과 그 근거에 대한 자세한 내용은 질적 연구에서 거의 찾아볼 수 없습니다. Vasileiou 등(2018)은 15년 동안 건강 관련 저널에서 [심층 인터뷰를 사용한 질적 연구]를 체계적으로 검토한 결과, 대다수의 논문에서 표본 크기에 대한 정당성을 제공하지 않는다는 사실을 발견했습니다. 정당성이 제시된 경우 55%의 논문에서 포화가 인용되었지만, 포화에 대한 주장은 "연구 자체에서 수행된 절차와 관련하여 입증되지 않았으며"(12쪽), 당면한 연구와 거리가 먼 다른 문헌의 추가 인용만 제시되었습니다.
Despite the importance of saturation to support the rigor of qualitative samples, there is a consistent lack of transparency in how sample sizes are justified in published qualitative research (Morse, 1995; Guest et al., 2006; Kerr et al., 2010; Carlsen and Glenton, 2011; Hennink et al., 2017). Although saturation is the most commonly cited justification for an adequate sample size (Morse, 1995, 2015), details of how saturation was assessed and the grounds on which it was determined are largely absent in qualitative studies. Vasileiou et al. (2018) conducted a systematic review of qualitative studies using in-depth interviews in health-related journals over a 15-year period and found the vast majority of articles provided no justification for their sample size. Where justifications were given, saturation was cited in 55% of articles; however, claims of saturation were “never substantiated in relation to procedures conducted in the study itself” (p. 12); only further citations of other literature were given that moved away from the study at hand.
마찬가지로 [포커스 그룹 토론]을 사용한 220개의 연구를 체계적으로 검토한 결과(Carlsen and Glenton, 2011), 83%가 표본 크기를 정당화하기 위해 포화를 사용했지만, 포화에 대한 근거 없는 주장이나 미리 정해진 표본 크기를 사용하면서 포화를 달성했다는 언급 등 포화를 달성한 방법에 대한 피상적인 보고만 제공한 것으로 밝혀졌습니다. 또 다른 연구(Francis 외, 2010)에서는 16개월에 걸쳐 사회과학 및 의학 저널의 논문을 검토한 결과 대부분의 논문이 포화 상태에 도달했다고 주장하지만 포화 상태를 정의, 달성 또는 정당화하는 방법에 대해 명확하게 설명하지 않는 것으로 나타났습니다. Marshall 등(2013)도 83개의 [질적 연구를 검토]한 결과 포화가 어떤 연구에서도 설명되지 않았다는 사실을 발견했습니다. 포화가 어떻게 평가되거나 결정되었는지에 대한 연구 기반의 설명 없이 포화를 주장하는 것에 대한 우려가 커지고 있습니다. 포화 상태에 도달했다는 근거 없는 주장은 포화 상태라는 개념의 가치를 훼손합니다. 부분적으로 이러한 투명성 부족은 포화를 평가하는 방법에 대한 공개된 지침이 없기 때문일 수 있습니다(Morse, 1995; Guest et al., 2006). 이 검토에서는 경험적 연구에서 포화를 평가하는 데 사용되는 전략을 파악하여 질적 연구에서 포화를 보고할 때 투명성을 높일 수 있는 방법을 모색하고자 합니다.
Similarly, a systematic review of 220 studies using focus group discussions (Carlsen and Glenton, 2011) found that 83% used saturation to justify their sample size; however, they provided only superficial reporting of how it was achieved, including unsubstantiated claims of saturation and references to achieving saturation while still using a predetermined sample size. Another study (Francis et al., 2010) reviewed articles in the journal Social Science and Medicine over 16 months and found most articles claimed they had reached saturation but provided no clarity on how saturation was defined, achieved, or justified. Marshall et al. (2013) also reviewed 83 qualitative studies and found saturation was not explained in any study. There are increasing concerns over claims of saturation without study-based explanations of how it was assessed or determined. Unsubstantiated claims of reaching saturation undermine the value of the concept. In part, this lack of transparency may reflect the absence of published guidance on how to assess saturation (Morse, 1995; Guest et al., 2006). In this review, we seek to identify the strategies used to assess saturation in empirical research, which may encourage greater transparency in reporting saturation in qualitative studies.
또한, 다양한 질적 방법에서 포화에 도달하는 데 필요한 특정 표본 크기에 대한 지침은 방법론 문헌에 없거나 모호하며, 근거에 기반하지 않은 일반적인 '경험 법칙'만 제공합니다(Morse, 1995; Guest 외, 2006; Kerr 외, 2010; Bryman, 2012; Hennink 외, 2019). 포화를 경험적으로 평가하는 연구가 이러한 격차를 메우기 시작하면서, 질적 연구에서 포화에 대한 표본 크기에 대해 절실히 필요한 경험적 기반 지침을 제공할 수 있게 되었습니다.
In addition, guidance on specific sample sizes needed to reach saturation in different qualitative methods has been absent or vague in the methodological literature, providing only general “rules of thumb” that are rarely evidence-based (Morse, 1995; Guest et al., 2006; Kerr et al., 2010; Bryman, 2012; Hennink et al., 2019). As research empirically assessing saturation begins to fill this gap, it allows us to provide much-needed empirically based guidance on sample sizes for saturation in qualitative research.
이 체계적 검토에서는 [정성적 데이터의 포화를 평가하는 실증적 연구를 종합]하고자 합니다. 특히, 포화를 평가하는 데 사용되는 전략을 문서화하고, 다양한 질적 방법을 사용하여 포화에 도달하는 데 필요한 표본 크기를 식별하며, 질적 연구를 위한 표본 크기에 대한 지침을 제시하고자 합니다. 저희가 알기로는 포화에 대한 경험적 연구에 대한 체계적인 검토는 이번이 처음이며, 따라서 질적 연구를 검토하는 연구자, 학술지, 학술지 심사자, 윤리 검토 위원회 및 자금 지원 기관에 유용한 리소스를 제공합니다. 연구자는 연구 제안서 및 프로토콜에서 적절한 표본 크기를 추정할 때 이 결과를 참조할 수 있으며, 이를 통해 연구 자원을 보다 효율적으로 사용하고 제안된 표본 크기에 대한 명확한 근거를 마련할 수 있습니다. 마찬가지로, 연구 결과는 질적 연구에 적합한 표본 크기에 대한 증거 기반의 기대치를 제공하여 연구를 검토하고 자금을 지원하는 사람들에게 지침을 제공할 수 있습니다.
In this systematic review, we aim to synthesize empirical studies that assess saturation in qualitative data. In particular, we aim to document strategies used to assess saturation, identify sample sizes needed to reach saturation using different qualitative methods, and suggest guidance on sample sizes for qualitative research. To our knowledge, this is the first systematic review on empirical studies of saturation and therefore provides a valuable resource for researchers, academic journals, journal reviewers, ethical review boards, and funding agencies that review qualitative research. Researchers can refer to our results when estimating an appropriate sample size in research proposals and protocols, which may lead to more efficient use of research resources and clearer justifications for proposed sample sizes. Similarly, our results may provide evidence-based expectations regarding adequate sample sizes for qualitative research to guide those who review and fund research.
2. 조사 방법
2. Methods
저희는 체계적 문헌고찰을 수행하고 보고할 때 체계적 문헌고찰 및 메타분석에 대한 선호 보고 항목(PRISMA) 가이드라인을 따랐습니다(Moher et al., 2009). 그림 1은 식별, 선별 및 포함된 논문 수를 보여줍니다. 데이터베이스 검색과 인용 검색을 포함한 2단계 검색 프로세스를 사용했습니다.
We followed the Preferred Reporting Items for Systematic Reviews and Meta-Analyses (PRISMA) guidelines in conducting and reporting our systematic review (Moher et al., 2009). Fig. 1 shows the number of articles identified, screened, and included. We used a two-stage search process, including database searches and citation searches.
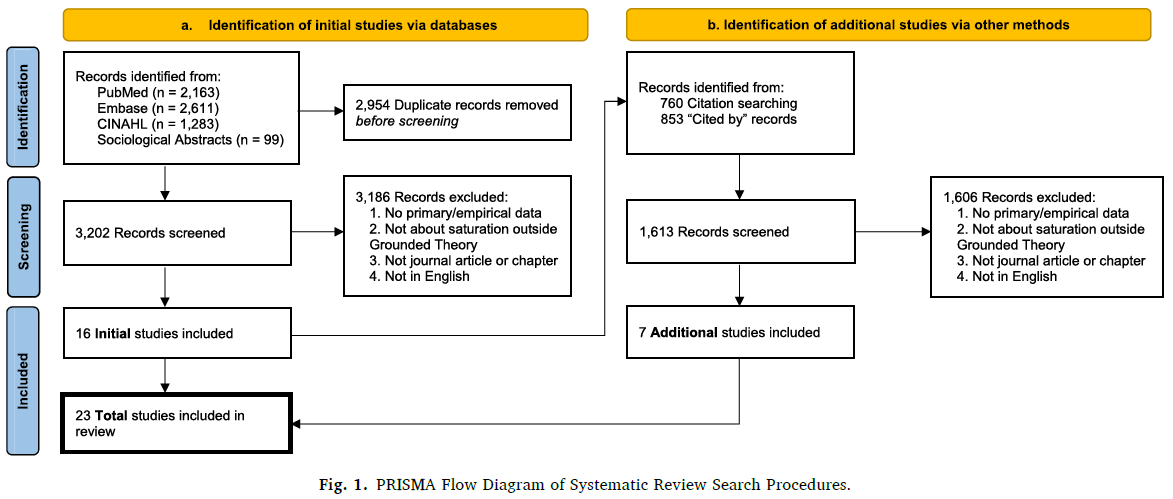
먼저 PubMed, Embase, Sociological Abstracts, CINAHL 등 4개의 데이터베이스를 사용하여 제목, 초록, 키워드/색인에 '포화'와 다음 용어 중 하나가 포함된 논문 또는 서적 챕터를 검색했습니다: "인터뷰", "포커스 그룹", "질적" 또는 "주제"(전체 검색어는 부록 표 참조). 검색 결과는 영어 및 인간 연구로 제한되었습니다. 데이터베이스 검색은 2019년 1월 31일부터 2월 1일까지 수행되었으며 2020년 7월 10일에 업데이트되었습니다. 두 저자는 모든 논문 제목, 초록, 필요한 경우 전문을 독립적으로 선별하여 적격성을 결정했습니다. 불일치하는 부분은 논의하고 합의를 통해 해결했습니다. 연구에 포함되려면,
- a) 질적 연구에서 포화를 평가하기 위해 경험적 데이터를 사용하거나 가상의 데이터를 사용하여 포화를 결정하는 통계 모델을 사용해야 하고,
- b) 근거 이론을 벗어난 포화에 초점을 맞춰야 하며,
- c) 저널 논문 또는 책 챕터로 출판되어야 하고,
- d) 영어로 이용 가능해야 합니다.
데이터베이스 검색을 통해 16개의 논문이 포함되었습니다.
First, we used four databases -- PubMed, Embase, Sociological Abstracts, and CINAHL -- to search for articles or book chapters that included “saturation” and one of the following terms in the title, abstract, or key words/index: “interview,” “focus group,” “qualitative,” or “thematic” (see Supplemental Table for full search terms). Search results were limited to English-language and human studies. Database searches were conducted on January 31 – February 1, 2019 and updated July 10, 2020. Both authors independently screened all article titles, abstracts, and, where needed, full texts to determine eligibility. Discrepancies were discussed and resolved by consensus. To be eligible for inclusion, studies needed to:
- a) use empirical data to assess saturation in qualitative research or use a statistical model to determine saturation using hypothetical data,
- b) focus on saturation outside of grounded theory,
- c) be published in journal articles or book chapters, and
- d) be available in English.
Sixteen articles were included from database searches.
둘째, 포함된 논문의 참고문헌 목록을 검토하고 Google Scholar의 '인용 출처' 검색 옵션을 사용하여 인용 검색을 수행하여 포함 기준을 충족하는 추가 기록을 식별했습니다. Google Scholar에서 250개 이상의 인용 논문이 있는 연구의 경우, 인용 논문 내에서 "포화"를 검색하여 처음 250개의 결과(관련성 순으로 정렬됨)를 검토했습니다. 이 단계에서 추가로 7개의 논문이 포함되었습니다.
Second, we conducted citation searches by reviewing the reference lists of included articles and using the “cited by” search option in Google Scholar to identify further records meeting the inclusion criteria. For studies with more than 250 citing articles on Google Scholar, we searched within citing articles for “saturation” and reviewed the first 250 results (which are ordered by relevance). An additional seven articles were included during this step.
23개의 적격 논문에서 다음과 같은 정보를 추출했습니다:
- a) 논문에 대한 메타 데이터(저자, 저널, 연도),
- b) 사용된 데이터에 대한 정보(가설적 대 경험적, 인터뷰, 포커스 그룹 토론 등), 연구 목적, 표본 크기, 연구 집단(동종, 이종), 데이터 수집이 반복적이었는지 여부,
- c) 정의, 목표, 데이터 무작위 배정, 포화 평가 전략, 포화를 위한 표본 크기 및 달성한 포화 수준(예, 코드의 90%) 및
- d) 추가 정보(제한 사항, 제안된 포화 매개변수).
두 저자는 6개의 논문에서 독립적으로 데이터를 추출하고 결과를 논의했습니다. 이는 명확성 부족이나 중복성 등 데이터 추출 범주에 문제가 있는지 확인하고 두 저자 간의 신뢰성을 확립하기 위해 수행되었습니다. 그런 다음 나머지 각 논문은 두 저자 중 한 명이 데이터 추출을 수행했습니다.
We extracted the following information from the 23 eligible articles:
- a) meta-data about the article (author, journal, year),
- b) information about data used (hypothetical vs. empirical; interviews, focus group discussions, etc.), research objective, sample size, study population (homogenous, heterogenous), and whether data collection was iterative,
- c) information about saturation, including: definition, goal, data randomization, strategy to assess saturation, sample size for saturation, and level of saturation achieved (e.g., 90% of codes), and
- d) additional information (limitations, any parameters of saturation suggested).
Both authors independently extracted data from 6 articles and discussed results. This was done to identify any issues with the data extraction categories, such as lack of clarity or redundancy, as well as to establish reliability between the two authors. Each remaining article then underwent data extraction by one of the two authors.
경험적 데이터를 사용하여 포화를 평가한 연구와 통계적 모델을 사용한 연구의 결과를 별도로 분석했습니다. 인터뷰 또는 포커스 그룹 토론과 같은 정성적 방법을 통해 포화에 대한 샘플 크기를 분석했습니다. 연구 모집단의 동질성 및 데이터 무작위 추출을 통해 포화를 비교하여 패턴을 파악했습니다.
We analyzed results separately for studies using empirical data to assess saturation versus those using statistical models. We analyzed sample sizes for saturation by qualitative method: interviews or focus group discussions. We conducted comparisons of saturation by homogeneity of the study population and randomization of data to identify any patterns.
3. 결과
3. Results
체계적인 검토를 통해 질적 연구의 포화를 평가한 23편의 논문을 확인했습니다. 모든 논문은 2006년부터 2020년 사이에 출판되었으며, 대다수(87%, 20/23)가 2014년 이후에 출판되었습니다. 연구 방법론 저널(43%, 10/23)과 사회과학(6/23) 또는 주제별 저널(7/23)(예: 공학, 컴퓨팅, 자연자원)에 게재된 논문이 많았습니다. 경험적 데이터를 사용하여 포화를 평가하는 논문(표 1, 17개 논문)과 통계적 모델링을 사용하여 포화를 예측하는 논문(표 2, 6개 논문)으로 분류했습니다. 이러한 접근 방식과 결과는 비교할 수 없으므로 아래에서 각각을 개별적으로 보고합니다.
Our systematic review identified 23 articles assessing saturation for qualitative research. All articles were published between 2006 and 2020, with the majority (87%, 20/23) published since 2014. Many articles were published in research methodology journals (43%, 10/23) and others in social science (6/23) or topical journals (7/23) (e.g., engineering, computing, natural resources). We categorized the articles into those assessing saturation using empirical data (Table 1, 17 articles) and those using statistical modeling to predict saturation (Table 2, 6 articles). Since these approaches and results are not comparable, we report each separately below.
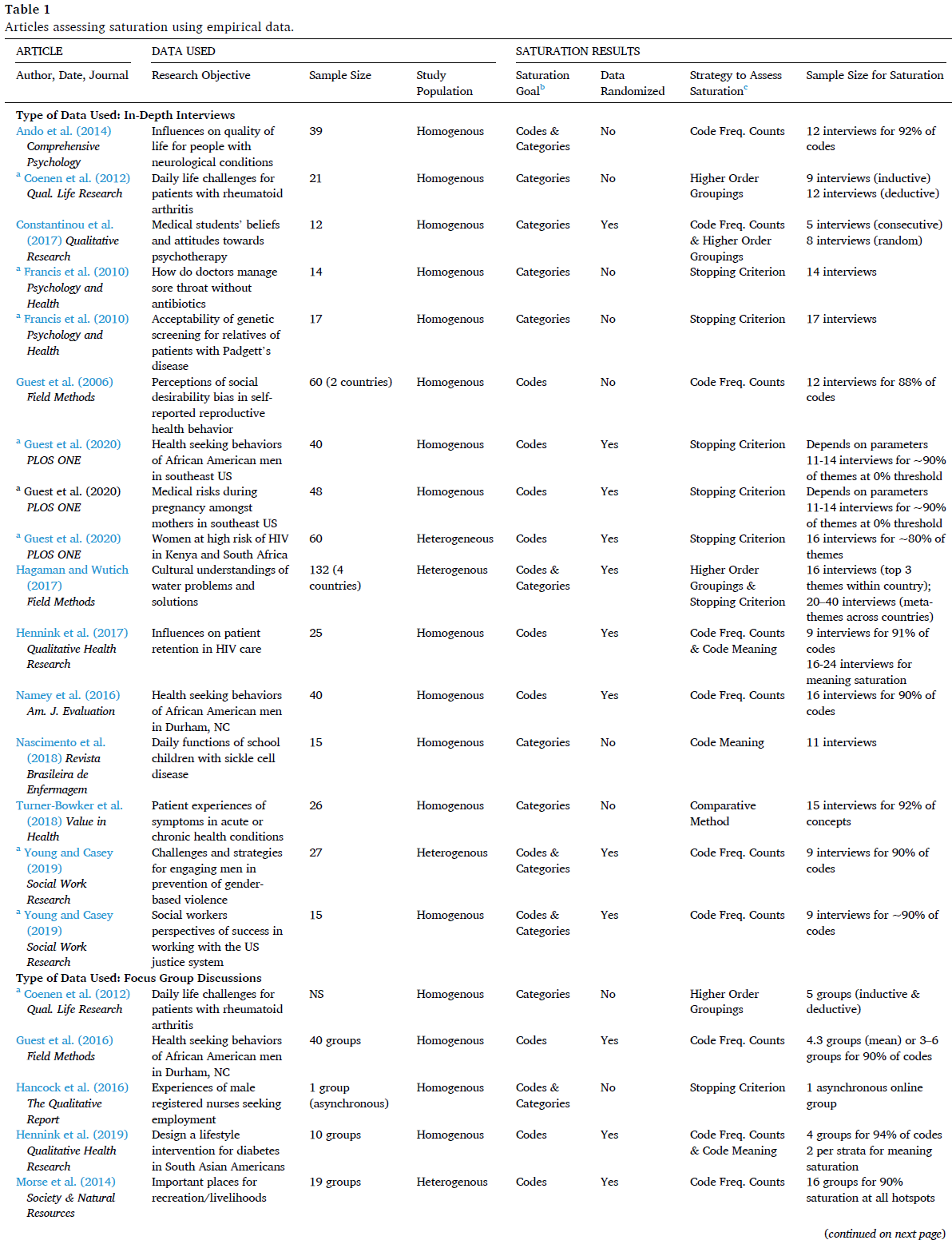
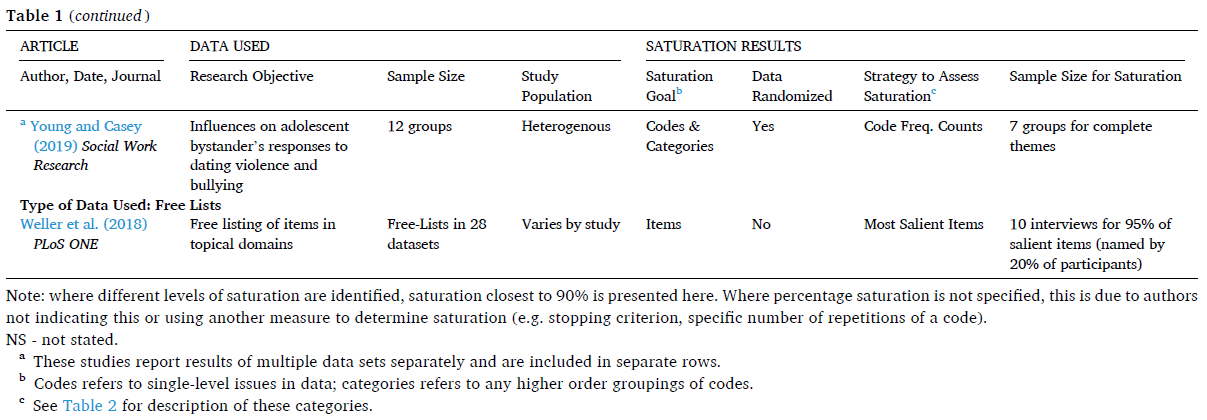
3.1. 포화
평가에 대한 접근 방식
3.1. Approaches to assessing saturation
3.1.1. 경험적 기반 테스트
3.1.1. Empirically based tests
표 1에는 경험적 데이터를 사용하여 포화를 평가한 17개의 논문이 요약되어 있습니다. 일부 논문에서는 여러 데이터 세트를 사용하여 포화를 평가하고 각각의 결과를 개별적으로 보고했기 때문에 표 1에는 17개 논문에서 23개의 테스트가 나와 있습니다(참고: 이러한 연구는 실험적 테스트를 수행하지 않았지만, 간결성을 위해 '테스트'라는 용어를 사용하여 통계적 모델링이 아닌 경험적 데이터를 사용하여 포화를 평가하는 개별 연구를 지칭합니다). 대부분의 논문은 심층 인터뷰(10/17) 또는 포커스 그룹 토론(4/17)에서 얻은 데이터를 사용했으며, 두 가지 유형의 데이터를 모두 사용한 논문은 두 편, 자유 목록 데이터를 사용한 논문은 한 편(Weller et al., 2018)이었습니다. 자유 목록 데이터는 인터뷰 및 포커스 그룹 토론에서 나온 자유로운 서술형 데이터와 비교할 수 없기 때문에 분석에서 Weller 등의 논문은 제외했습니다. 따라서 모든 논문을 설명할 때는 분모 16을 사용하고, 경험적 데이터로 모든 테스트의 데이터 세트와 결과를 설명할 때는 분모 22를 사용합니다.
Table 1 summarizes 17 articles that assess saturation using empirical data. Some articles used multiple datasets to assess saturation and report the results of each separately; therefore, Table 1 shows 23 tests from 17 articles (NB: while these studies were not conducting experimental tests, we use the term ‘test’ for brevity to refer to individual studies using empirical data, as opposed to statistical modeling, to assess saturation). Most articles used data from in-depth interviews (10/17) or focus group discussions (4/17); two articles used both types of data, and one article (Weller et al., 2018) used free list data. We excluded the article by Weller et al. in our analysis because free list data are not comparable to free-flowing narrative data from interviews and focus group discussions. We therefore use the denominator of 16 when describing all articles and 22 when describing the datasets and results of all tests with empirical data.
테스트에 사용된 각 데이터 세트의 원래 연구 목적은 다양했지만, 대부분의 연구(14/16)는 특정 건강 상태(예: 겸상 적혈구 질환, 다발성 경화증, 파제트병), 의료 서비스 또는 개입(예: 유전자 검사, 폭력 예방, 라이프스타일 개입, 환자 유지)의 [경험]과 같은 [건강 문제]에 초점을 맞췄습니다. 이러한 연구 목표는 많은 질적 건강 연구의 전형적인 목표입니다. 사용된 데이터 세트의 표본 크기는 14~132건의 인터뷰와 1~40개의 포커스 그룹으로 다양했습니다. 한 데이터셋(Francis et al., 2010)을 제외한 모든 데이터셋은 포화를 평가하는 데 필요한 표본보다 훨씬 더 큰 표본을 가지고 있어 포화를 평가하는 데 효과적이었습니다. Francis 등(2010)은 사용된 두 데이터 세트 모두에서 연구의 표본 크기와 정확히 일치하는 포화에 도달했다고 보고했습니다. 대부분의 데이터세트(18/22)는 특정 질병(예: HIV, 류마티스 관절염, 겸상 적혈구)을 앓고 있거나 특정 인구 집단(예: 남성 간호사, 의대생, 남아시아 성인, 아프리카계 미국인 남성)의 환자 등 [동질적인 연구 모집단]을 가지고 있었습니다. 나머지 데이터 세트에는 미국 전역의 20~72세 남성 또는 14~18세 청소년과 같이 이질적인 샘플이 더 많았습니다.
The original research objective for each dataset used in the tests varied, but most studies (14/16) focused on health issues, such as experiences of a specific health condition (e.g., sickle cell disease, multiple sclerosis, Paget's disease), health service, or intervention (e.g., genetic screening, violence prevention, lifestyle interventions, patient retention). These research objectives are typical of much qualitative heath research. The sample size of the datasets used varied from 14 to 132 interviews and 1 to 40 focus groups. All datasets except one (Francis et al., 2010) had a sample that was much larger than the sample ultimately needed for saturation, making them effective for assessing saturation. Francis et al. (2010) report saturation was reached at exactly the sample size of the study for both datasets used. Most datasets (18/22) had a homogenous study population, such as patients with a specific disease (e.g., HIV, rheumatoid arthritis, sickle cell) or from a specific demographic group (e.g., male nurses, medical students, South Asian adults, African American men). The remaining datasets had more heterogeneous samples, such as men aged 20–72 across the US or youths aged 14–18.
저자들은 포화의 목표를 [개별 코드 또는 카테고리의 포화]라는 두 가지 방식으로 설명했습니다. 용어는 기사마다 다르지만,
- [코드]는 일반적으로 데이터의 개별 이슈, 주제 또는 항목으로 설명되었고
- [카테고리]는 이슈의 상위 그룹(예: 더 넓은 주제, 메타 주제, 개념)을 나타냅니다.
44%(7/16)의 논문이 코드의 포화를, 31%(5/16)의 논문이 카테고리의 포화를, 25%의 논문이 두 가지 모두를 명시했습니다.
Authors described their goal of saturation in two ways, either as saturation of individual codes or categories. Although terminology varied across articles,
- codes were typically described as individual issues, topics, or items in data, while
- categories represented higher-order groupings of issues (e.g., broader themes, meta-themes, concepts).
Forty-four percent (7/16) of articles sought saturation of codes, 31% (5/16) saturation of categories, and 25% stated both.
포화가 정의된 경우, 저자들은 비슷한 정의를 사용했습니다. 전반적으로 포화 상태는 [데이터에서 관련성이 있는 새로운 코드 및/또는 범주가 거의 또는 전혀 발견되지 않는 시점]으로, [연구 현상, 차원, 뉘앙스 또는 가변성에 대한 더 이상의 이해나 기여 없이] [문제가 반복되기 시작하는 시점]으로 설명되었습니다.
- 일부 논문에서는 두세 번의 연속 인터뷰 또는 포커스 그룹에서 새로운 이슈가 발견되지 않은 후에야 포화 상태를 확인해야 한다고 명시하거나(Coenen 등, 2012; Francis 등, 2010; Morse 등, 2014),
- 두 명의 연구자에 의해 포화 상태를 결정해야 한다고 명시하기도 했습니다(Morse 등, 2014).
- 절반 이상의 논문(56%, 9/16)은 포화에 영향을 줄 수 있는 인터뷰 순서를 고려하여 분석 데이터의 순서를 무작위로 지정했습니다.
- 일부는 무작위 인터뷰 순서와 실제 인터뷰가 진행된 순서 사이의 포화를 비교했고,
- 다른 일부는 여러 무작위 데이터 순서에 걸쳐 포화를 계산하여 평균을 확인했습니다.
Where saturation was defined, authors used similar definitions. Overall, saturation was described as the point at which little or no relevant new codes and/or categories were found in data, when issues begin to be repeated with no further understanding or contribution to the study phenomenon, its dimensions, nuances, or variability.
- Some articles further specified that saturation should be confirmed only after no new issues were found in two or three consecutive interviews or focus groups (Coenen et al., 2012; Francis et al., 2010; Morse et al., 2014) or
- that it was determined by two researchers (Morse et al., 2014).
- Over half of articles (56%, 9/16) randomized the order of data for analysis to account for interview order, which might influence saturation.
- Some compared saturation between the randomized order of interviews and the actual order in which interviews were conducted,
- while others calculated saturation across multiple randomized orderings of data to identify an average.
[포화를 평가하기 위해 다양한 전략]이 사용되었습니다. 이러한 전략은 표 1에 분류되어 있으며 표 3에 설명된 범주에 따라 분류되어 있습니다.
- 대부분의 기사(75%, 12/16)는 포화를 평가하기 위해 [단일 전략을 사용]했습니다.
- 모든 논문은 코드 빈도수, 비교 방법, 중지 기준, 고차 그룹화 등 어떤 형태로든 [코드 빈도수를 사용]하여 포화를 평가했으며,
- 4편의 논문은 코드 빈도수 외에 다른 접근법을 사용하여 각각의 포화를 비교했습니다(Hennink 외, 2017, 2019; Constantinou 외, 2017; Hagaman과 Wutich, 2017).
- 많은 논문(37%, 6/16)은 포화를 평가하기 위해 코드 빈도 수만을 사용했는데, 이는 새로운 코드의 빈도가 감소하여 포화에 도달할 때까지 연속된 녹취록 또는 녹취록 세트의 코드를 계산하는 방식이었습니다.
- 3건(18%, 3/16)의 논문에서는 배치 비교, 중단 기준, 고차 코드 그룹을 계산(개별 코드가 아닌 메타 주제 또는 코드 범주 등)하는 등 코드 빈도 수에 특정 추가 요소를 추가했습니다.
- 세 논문(Hennink 외., 2017, 2019; Nascimento 외., 2018)에서는 코드 빈도 수와는 완전히 다른 접근 방식인 '코드 의미'를 사용하여 포화를 평가했습니다. 이 접근 방식은 문제, 차원 및 뉘앙스가 완전히 식별되고 이해되었는지를 평가하여 데이터의 문제를 완전히 이해하는 데 중점을 두어 포화에 도달했음을 나타내는 지표로 삼았습니다.
- 그 후 두 논문(Hennink 외., 2017, Hennink 외., 2019)에서 이 접근 방식을 사용한 포화를 코드 빈도 접근 방식과 비교했습니다.
Various strategies were used to assess saturation. These are categorized in Table 1 and the categories described in Table 3.
- Most articles (75%, 12/16) used a single strategy to assess saturation.
- All articles used some form of code frequency counts to assess saturation (including code frequency counts, comparative method, stopping criterion, higher-order groupings), and four articles used another approach in addition to code frequency counts and compared saturation for each (Hennink et al., 2017, 2019; Constantinou et al., 2017; Hagaman and Wutich, 2017).
- Many articles (37% 6/16) used only code frequency counts to assess saturation, which involved counting codes in successive transcripts or sets of transcripts until the frequency of new codes diminishes, signaling saturation is reached.
- Three articles (18%, 3/16) added specific additional elements to code frequency counts, such as batch comparisons, a stopping criterion, or counting higher-order groupings of codes, such as meta-themes or categories of codes rather than individual codes.
- Three articles (Hennink et al., 2017, 2019; Nascimento et al., 2018) used ‘code meaning’ to assess saturation, an entirely different approach from code frequency counts. This approach focused on reaching a full understanding of issues in data as the indicator that saturation is reached, by assessing whether the issue, its dimensions, and nuances are fully identified and understood.
- Two articles (Hennink et al., 2017, Hennink et al., 2019) then compared saturation using this approach with the code frequency approach.
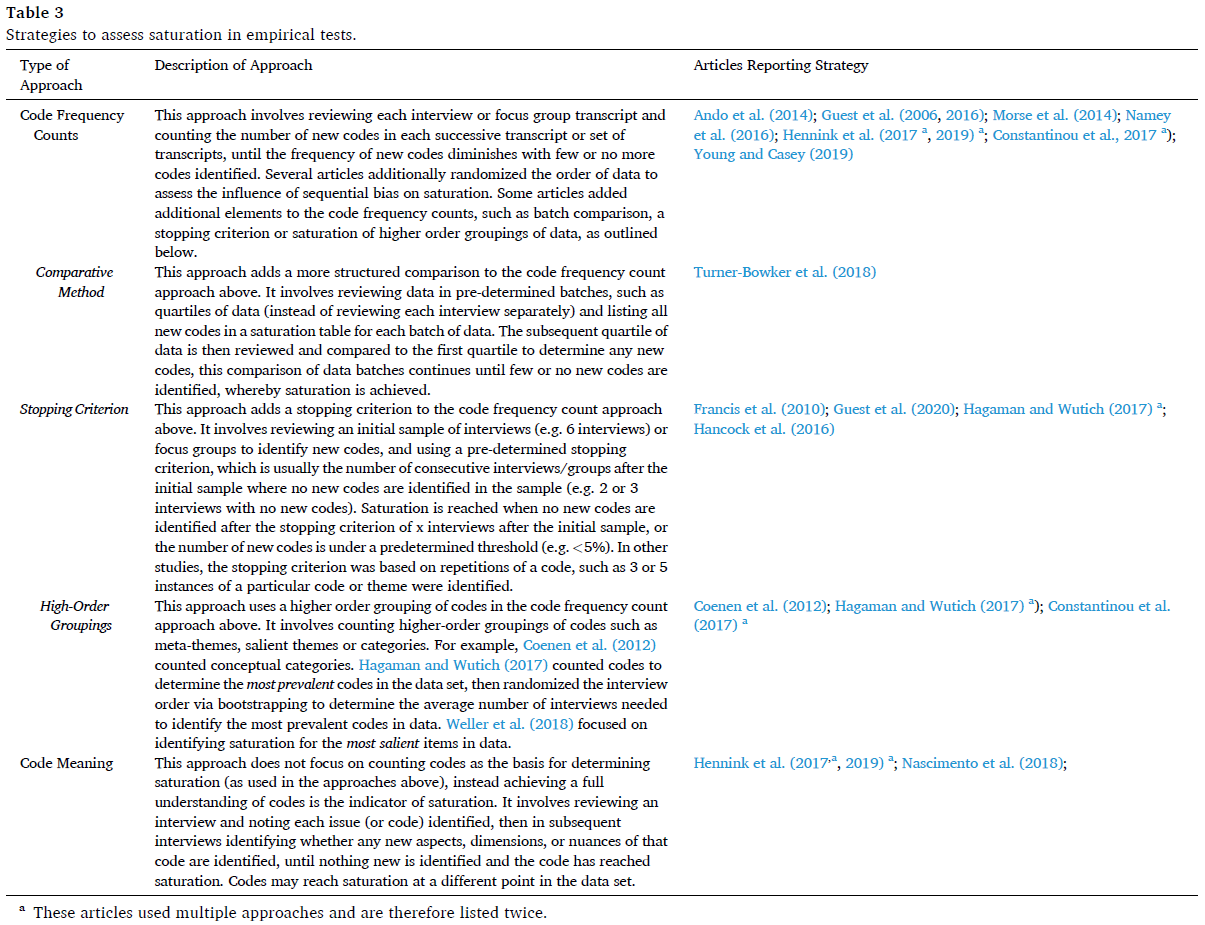
3.1.2. 통계적 모델
3.1.2. Statistical models
표 2는 포화를 추정하기 위해 통계적 모델링을 사용한 6개의 논문을 요약한 것입니다. 이 논문들은 위에 요약된 것과는 다른 접근법을 사용했는데, 포화에 필요한 표본 크기를 추정하는 [공식을 개발]하여 데이터 수집 전에 연구 설계에 정보를 제공하기 위해 사용할 수 있습니다.
- 몇몇 공식은 모집단 내 테마의 유병률 또는 데이터에서 원하는 테마의 인스턴스 수와 같은 유사한 매개변수를 기반으로 한 반면(Fugard and Potts, 2015; Galvin, 2015; Lowe 외, 2018),
- 로그 정규 분포(Rowlands 외, 2016; Van Rijnsoever, 2017) 또는 집합 이론(Fofana 외, 2020)을 사용한 공식도 있었습니다.
- 이러한 연구 중 다수는 무작위 표본을 가정한 반면, 무작위 표본과 의도적 표본을 모두 고려한 연구도 있었습니다(Van Rijnsoever, 2017).
- 대부분의 공식은 인터뷰 데이터를 위해 개발되었으며, 두 개의 논문은 인터뷰, 포커스 그룹, 문서, 문헌 조사 등 다양한 형태의 데이터에 대한 포화 추정에 대해 논의했습니다. 그런 다음 공식의 절반을 경험적 데이터 세트에 적용했습니다.
Table 2 summarizes six articles that used statistical modeling to estimate saturation. These articles used a different approach than those summarized above: they developed a formula to estimate the sample size needed for saturation, which may be used prior to data collection to inform study design.
- Several formulas were based on similar parameters, such as prevalence of a theme in a population or the desired instances of a theme in data (Fugard and Potts, 2015; Galvin, 2015; Lowe et al., 2018),
- while others used a lognormal distribution (Rowlands et al., 2016; Van Rijnsoever, 2017) or set theory (Fofana et al., 2020).
- Many of these studies assumed a random sample, while one accounts for both random and purposive samples (Van Rijnsoever, 2017).
- Most formulas were developed for interview data, while two articles discussed estimating saturation for various forms of data, including interviews, focus groups, documents, and literature surveys. Half of the formulas were then applied to empirical datasets.
3.2. 포화를 위한 샘플 크기
3.2. Sample size for saturation
그림 2는 [심층 인터뷰 데이터]를 사용한 경험적 기반 테스트의 포화에 대한 샘플 크기를 보여줍니다. 테스트에 사용된 각 데이터 세트(n = 16)에 대한 결과는 별도의 데이터 포인트로 표시됩니다. 서로 다른 표본 크기로 결과가 보고된 경우, 보고된 가장 낮은 표본 크기부터 가장 높은 표본 크기까지 선으로 표시되며, 이 범위에 영향을 미치는 매개변수가 표시됩니다. 저자가 서로 다른 포화 수준을 보고한 경우, 연구 간의 비교 가능성을 위해 90%에 가장 가까운 포화가 표시됩니다. 결과에 따르면 포화에 대한 다양한 접근 방식을 사용한 16개의 테스트에서 포화의 표본 크기는 5개에서 24개의 인터뷰 사이였습니다.
- 가장 작은 포화 표본 크기는 5개의 인터뷰로, 설문조사 결과를 뒷받침하기 위한 동질적인 연구 모집단을 대상으로 광범위한 범주에서 포화를 구한 연구(Constantinou et al., 2017)였습니다. 이러한 연구 특성을 종합하면 5번의 인터뷰로 포화 상태에 도달한 것을 설명할 수 있습니다.
- 포화에 대한 가장 큰 표본 크기는 4개국의 메타주제에 대한 포화를 추구한 20~40개(Hagaman and Wutich, 2017)와 연구 질문의 중심이 아닌 코드를 포함한 코드의 의미에 대한 포화를 추구한 24개(Hennink et al., 2017)였습니다.이러한 포화 목표를 달성하려면 더 많은 데이터가 필요하며, 이는 포화에 대한 더 높은 표본 크기를 뒷받침할 수 있습니다.
Fig. 2 shows sample sizes for saturation from empirically based tests using in-depth interview data. The results for each dataset used in the tests (n = 16) are shown as separate data points. Where results are reported at different sample sizes, this is depicted with a line from the lowest to highest sample size reported, and the parameters influencing this range are noted. Where authors report different levels of saturation, saturation closest to 90% is shown for comparability across studies. Results show that across 16 tests using various approaches to saturation, the sample size for saturation ranges between 5 and 24 interviews.
- The lowest sample size for saturation was 5 interviews (Constantinou et al., 2017), in a study with a homogenous study population that was intended to support survey findings and where saturation was sought in broad categories. Together, these study characteristics may explain reaching saturation at 5 interviews.
- The highest sample sizes for saturation were 20–40 (Hagaman and Wutich, 2017), where saturation of meta-themes across four countries was sought, and 24 (Hennink et al., 2017), where saturation was sought in the meaning of codes, including codes less central to the research question. These saturation goals require more data, which may support the higher sample sizes found for saturation.
이러한 이상값을 제외하면, 대부분의 데이터 세트는 포화를 평가하기 위해 다양한 접근 방식을 사용했음에도 불구하고 9~17개의 인터뷰, 평균 12~13개의 인터뷰 사이에 포화에 도달했습니다. 대부분의 연구는 비교적 [동질적인 연구 모집단]을 사용했으며 코드, 카테고리 또는 그 조합의 포화 목표가 다양했습니다. 이질적인 샘플을 사용한 연구는 단 3건에 불과했습니다. 이 중 두 연구는 평균보다 큰 표본 크기(16개 인터뷰)에서 포화에 도달했고, 한 연구는 작은 표본 크기(9개 인터뷰)에서 포화에 도달했습니다. 따라서 이 특성에 따른 포화 패턴은 발견되지 않았습니다. 마찬가지로, 대부분의 테스트에서 실제 인터뷰 순서대로 데이터를 분석할 때 무작위 순서와 포화를 비교하지 않았기 때문에 데이터 순서에 따른 포화의 패턴을 식별하기 어려웠습니다. 비교를 수행한 연구에서는 무작위 인터뷰 순서와 실제 인터뷰 순서에서 포화에 차이가 없거나 샘플 크기가 약간 더 큰 것으로 나타났습니다. 무작위 추출을 사용한 연구와 표본 크기의 전체 스펙트럼을 다루지 않은 연구 모두 검토 대상에 포함되지 않았습니다.
Excluding these outliers, most datasets reached saturation between 9 and 17 interviews, with a mean of 12–13 interviews, despite using different approaches to assess saturation. Most of these studies had a relatively homogenous study population and varied in their saturation goal of codes, categories, or a combination. Only three studies used a heterogeneous sample. Two of these studies reached saturation at a larger sample size than the mean (at 16 interviews), and one reached saturation at a smaller sample size (at 9 interviews). Therefore, we found no pattern in saturation by this characteristic. Similarly, it was difficult to identify any pattern of saturation by the order of data, since most tests did not compare saturation when analyzing data in the actual interview order with the randomized order. Those that did make a comparison found no difference or a slightly higher sample size for saturation in the random versus actual order of interviews. Both studies that used randomization and those that did not cover the full spectrum of sample sizes seen in our review.
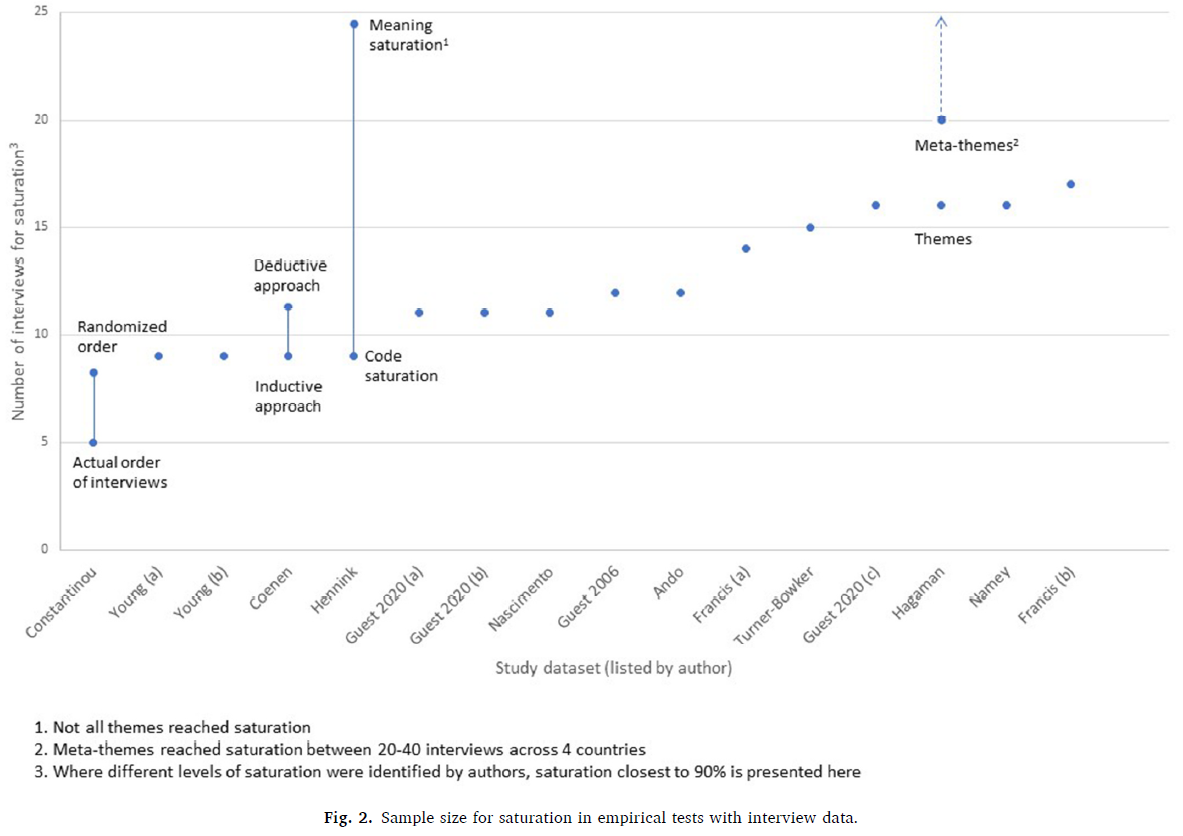
그림 3은 [포커스 그룹 토론 데이터]를 사용한 6개의 경험적 테스트에서 포화에 대한 샘플 크기를 보여줍니다. 비교 가능성을 위해 다양한 수준의 포화가 보고된 경우 90%에 가장 가까운 포화가 그림에 표시되어 있습니다. 6개의 테스트 모두에서 1~16개의 포커스 그룹 사이에서 포화에 도달했습니다. 두 테스트는 이상치이므로 다른 테스트와 비교할 수 없습니다.
- 가장 낮은 수치인 Hancock 등(2016)은 단일 비동기 온라인 포커스 그룹의 포화에 대해 보고하며, 포화는 요일 및 참여자별로 보고됩니다.
- 상위 연구에서는 Morse 등(2014)이 16개 그룹에서 포화에 도달했다고 보고했지만, 코드나 테마가 아닌 공간적 위치에 초점을 맞추었기 때문에 포화에 대한 표본 크기가 더 클 수 있습니다.
- 나머지 4개의 테스트는 유사한 포화 정의를 사용했으며, 평균 5~6개의 포커스 그룹으로 4~8개의 포커스 그룹 사이에서 포화에 도달했습니다.
대부분의 테스트(4/6)는 연구 모집단이 균질했지만 포화를 평가하는 접근 방식과 코드 또는 카테고리의 포화 목표가 다양했습니다. 이질적인 샘플을 사용한 두 테스트에서는 모두 평균 그룹 수보다 많은 샘플 크기(7개 및 17개 그룹)에서 포화에 도달했습니다.
Fig. 3 shows the sample size for saturation from six empirical tests using data from focus group discussions. For comparability, where various levels of saturation are reported, those closest to 90% are shown in the figure. Across all six tests, saturation was reached between 1 and 16 focus groups. Two tests are outliers and thus not comparable to others.
- At the lower end, Hancock et al. (2016) report on saturation in a single asynchronous, online focus group, and saturation is reported by day and participant.
- At the higher end, Morse et al. (2014) report reaching saturation at 16 groups; however, they focus on spatial locations rather than codes or themes, which may account for the higher sample size for saturation.
- The remaining four tests used similar definitions of saturation and reached saturation between 4 and 8 focus groups, with a mean of 5–6 groups.
Most tests (4/6) had a homogenous study population but varied in their approach to assessing saturation and the saturation goal of codes or categories. In the two tests using heterogeneous samples, both reached saturation at sample sizes above the mean number of groups (at 7 and 17 groups).
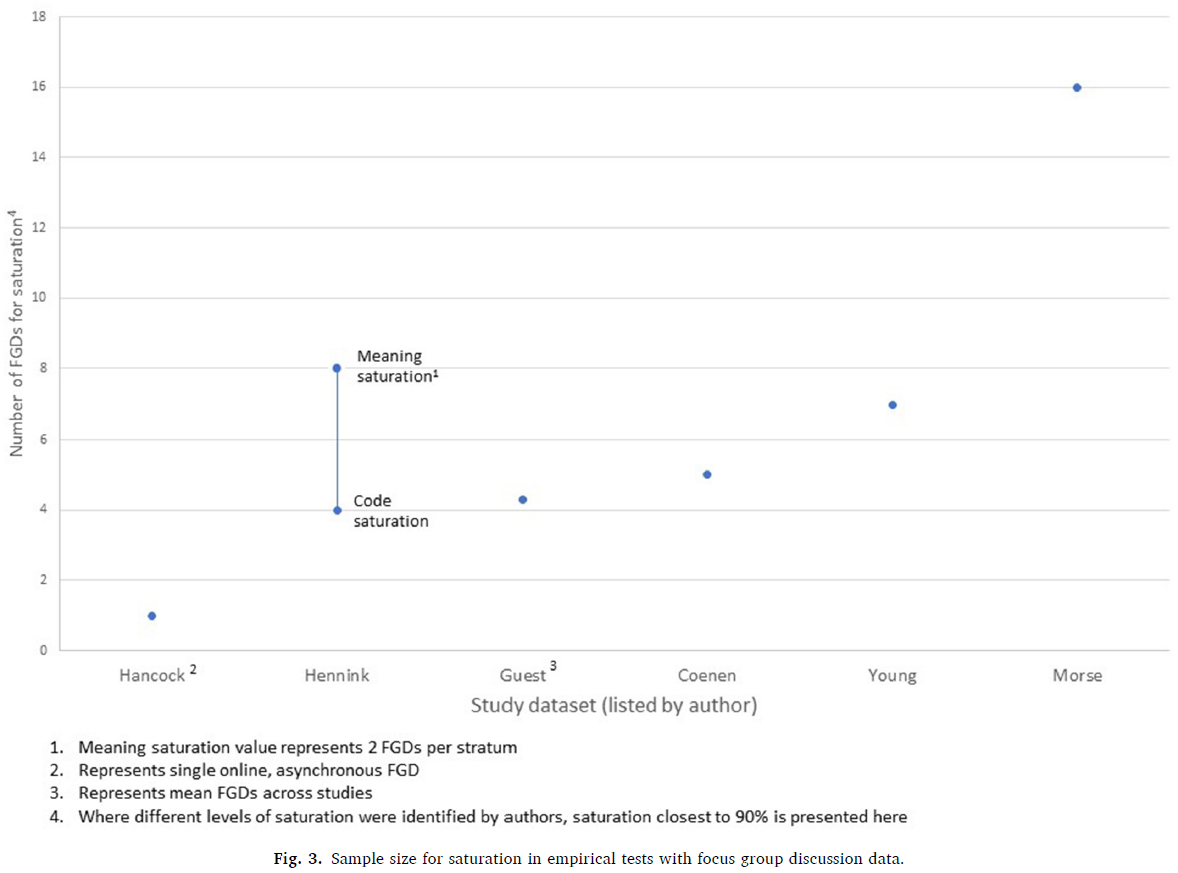
경험적 데이터에 적용된 포화에 대한 통계 모델을 개발한 연구에서도 포화에 대한 표본 크기는 위와 유사했습니다(표 2). 예를 들어, Rowlands 등(2016)은 로그 정규 분포를 사용하여 [세 가지 인터뷰 데이터 세트]에서 포화를 추정했으며, 그 결과 95% 신뢰 수준에서 포화에 대한 표본 크기는 10개, 10개, 13개로 나타났습니다. (2020)은 [집합 이론과 부분 최소 제곱 회귀]를 사용하여 12개의 인터뷰 데이터 세트에 적용했을 때 포화를 추정했습니다.
In studies that developed statistical models for saturation that were applied to empirical data, the sample sizes for saturation were similar to those above (Table 2). For example, Rowlands et al. (2016) used the lognormal distribution to estimate saturation in three datasets of interviews, and results found the sample sizes for saturation at 95% confidence to be 10, 10, and 13 interviews. Fofana et al. (2020) used set theory and partial least squares regression to estimate saturation at 12 interviews when applied to an empirical dataset.
4. 토론
4. Discussion
이 체계적 문헌고찰은 포화를 평가하는 실증 연구를 식별하고, 포화에 필요한 표본 크기, 포화를 평가하는 데 사용되는 전략, 그리고 이러한 연구에서 도출할 수 있는 지침을 파악하고자 했습니다. 포화를 실증적으로 평가한 23개의 연구를 확인했으며, 이 중 80%가 2014년 이후에 발표된 연구였습니다. 포화를 평가하는 데는 경험적 데이터를 사용한 연구와 통계적 모델을 사용한 연구라는 두 가지 접근 방식이 있었습니다.
This systematic review sought to identify empirical studies that assess saturation, to identify sample sizes needed for saturation, strategies used to assess saturation, and guidelines we can draw from these studies. We identified 23 studies that empirically assessed saturation, with 80% published since 2014. We identified two different approaches to assess saturation: studies that used empirical data and those that used statistical models.
포화를 평가하는 한 가지 접근 방식은 데이터를 수집하기 전에 포화에 대한 표본 크기를 추정하기 위한 통계 모델을 개발하는 데 중점을 두었습니다. 포화를 선험적으로 추정하려는 노력에는 박수를 보내지만, 개발된 많은 공식은 [정성적 연구 수행에 부합하지 않는 암묵적 가정을 기반]으로 하기 때문에 그 유용성이 크게 제한됩니다. 이러한 연구 중 상당수는 [무작위 표본]을 사용하여 전체 인구에서 특정 주제의 유병률 또는 데이터에서 원하는 주제의 인스턴스를 파악하는 등 [확률 기반 가정]을 사용합니다. 게다가 연구자들은 연구를 수행하기 전에 이러한 매개변수를 알지 못하는 경우가 많으며, 항목의 유병률은 질적 연구의 중요한 초점이 아닙니다. [통계 공식]은 정량적 연구자에게 익숙한 검정력 계산과 유사하게 보일 수 있기 때문에, 정성적 연구와 가정이 일치하지 않을 경우 [궁극적으로 달성할 수 없는 과학적 신뢰성]을 오해의 소지가 있는 베일로 만들 수 있다고 생각합니다. 이러한 접근 방식에 대한 우려를 감안하여 더 이상 고려하지 않습니다.
One approach to assessing saturation focused on developing statistical models to estimate sample sizes for saturation prior to data collection. While we applaud efforts to estimate saturation a priori, many of the formulas developed are based on implicit assumptions that do not align with the conduct of qualitative research, thereby significantly limiting their utility. Many of these studies use probability-based assumptions, such as having a random sample and knowing the prevalence of a theme in the broader population or the desired instances of a theme in data. Moreover, researchers often do not know these parameters prior to conducting a study, nor is prevalence of items an important focus of qualitative research. Since a statistical formula may be seen as akin to a power calculation familiar to quantitative researchers, we feel that this may provide a misleading veil of scientific authenticity that ultimately cannot be achieved given the misalignment of assumptions with qualitative research. Given our concerns about these approaches, we do not consider them further.
포화를 평가하는 두 번째 접근 방식은 [경험적 데이터를 사용]했습니다. 심층 인터뷰 데이터를 사용한 16개의 포화 테스트에서 모두 25개 미만의 인터뷰, 더 구체적으로는 [이상값을 제외한 9~17개의 인터뷰]에서 포화에 도달했습니다. 포화를 평가하기 위해 [다양한 접근 방식, 다양한 데이터 세트, 다양한 포화 목표(코드 대 카테고리), 동질적 및 이질적인 연구 모집단을 사용]했음에도 불구하고 연구는 여전히 좁은 범위의 인터뷰 내에서 포화에 도달했습니다. 이는 다양한 접근 방식에 걸쳐 강력한 외부 신뢰도를 보여줍니다. 모든 테스트에서 평균 12~13개의 인터뷰가 포화 상태에 도달했는데, 이는 포화 상태를 경험적으로 평가한 최초의 연구 중 하나인 Guest 외(2006)의 연구 결과(12개의 인터뷰에서 포화 상태에 도달했다고 보고)와 매우 유사합니다. [연구 모집단의 동질성, 무작위 배정 사용, 포화 목표 등] [연구 특성]에 따른 포화에 대한 명확한 패턴을 발견하지 못했는데, 이는 이러한 매개변수를 실제로 평가한 연구가 거의 없었기 때문입니다.
A second approach to assess saturation used empirical data. In all 16 tests of saturation with data from in-depth interviews, saturation was reached in under 25 interviews, more specifically between 9 and 17 interviews excluding outliers. Despite using different approaches to assess saturation, different datasets, varying saturation goals (codes vs categories), and homogenous and heterogeneous study populations, studies still reached saturation within a narrow range of interviews. This demonstrates strong external reliability across the different approaches. Across all tests, an average of 12–13 interviews reached saturation, which is remarkably similar to findings from Guest et al. (2006), one of the first studies to empirically assess saturation, which reported saturation at 12 interviews. We found no clear pattern in saturation by study characteristics, such as homogeneity of the study population, use of randomization, or saturation goal, largely because few studies actually assessed these parameters in their approach.
포커스 그룹 토론 데이터를 사용한 6개의 테스트에서 포화에 도달한 그룹은 4~8개 그룹으로, 비슷한 좁은 범위였습니다. 인구통계학적 계층화, 이질적인 샘플, 더 넓은 포화 목표(예: 코드 의미, 모든 주제 대 주요 주제)를 사용한 연구에서는 포화에 도달하기 위해 더 많은 그룹이 필요했습니다. 그러나 포커스 그룹 데이터를 비교한 추가 연구 없이 이러한 특성의 영향에 대한 결론을 내리는 것은 신중해야 합니다. 전반적으로 이러한 결과는 다양한 정성적 방법의 포화에 대한 표본 크기에 대한 절실히 필요한 경험적 증거를 제공합니다. 특정 범위의 인터뷰 또는 포커스 그룹 내에서 포화가 수렴하더라도, 아래에서 설명하는 바와 같이 이러한 방법을 사용하는 모든 질적 연구의 일반적인 표본 크기로 사용하거나 잘못 설계되거나 실행된 질적 연구를 정당화하기 위해 이러한 결과를 사용하지 않도록 주의해야 합니다. 대신, 이러한 결과를 질적 연구의 표본 크기를 추정할 때 특정 연구 특성과 함께 고려할 수 있는 지침으로 사용하는 것이 좋습니다.
In six tests using data from focus group discussions, saturation was reached by 4–8 groups, a similarly narrow range. Studies using demographic stratification, heterogeneous samples, and broader saturation goals (e.g., code meaning, all themes vs main themes) needed more groups to reach saturation. However, we are cautious about drawing conclusions regarding the influences of these characteristics without more studies with focus group data to compare. Overall, these findings provide much-needed empirical evidence of sample sizes for saturation for different qualitative methods. Despite convergence of saturation within a specific range of interviews or focus groups, we caution not to use these findings as generic sample sizes for any qualitative study using these methods, or to justify poorly designed or executed qualitative studies, as we discuss below. Instead, we recommend using these results as guidance to consider alongside the specific study characteristics when estimating the sample size for a qualitative study.
4.1. 연구에 대한 시사점
4.1. Implications for research
체계적 문헌고찰의 결과는 몇 가지 중요한 시사점을 제공합니다. 여기서는 경험적 기반 연구의 시사점에만 초점을 맞춥니다. 이러한 결과는 인터뷰와 포커스 그룹 토론을 사용할 때 포화를 위한 적절한 표본 크기에 관한 경험적 지침을 제공하며, 이는 질적 연구 제안서를 개발할 때 유용할 수 있습니다. 검토 대상에 포함된 대부분의 경험적 기반 연구는 연구 모집단이 균질하고 연구 목표가 집중되어 있었기 때문에 이러한 결과를 다른 유형의 표본이나 더 광범위한 목표를 사용하는 연구에 자신 있게 추정할 수는 없습니다. 따라서 [이러한 결과를 출발점]으로 삼아 인터뷰 또는 포커스 그룹의 잠재적 범위를 파악한 다음 연구 특성(예: 연구 목표, 연구 대상 현상의 성격 및 복잡성, 도구 구조, 샘플링 전략, 표본의 계층화, 연구자의 질적 연구 경험, 포화 목표 및 추구하는 포화 정도)을 고려하여 표본 크기를 구체화하는 것이 좋습니다(Baker and Edwards, 2012; Galvin, 2015; Morse, 1995; 연구 변수를 사용하여 포화를 추정하는 데 대한 자세한 논의는 Hennink et al., 2017 참조). 이러한 고려사항은 각 특정 연구에 보다 맞춤화된 표본 크기로 이어질 뿐만 아니라 제안된 표본 크기에 대한 보다 명확한 정당성을 제공하여 엄밀성을 더합니다.
The results of our systematic review have several important implications. We focus here only on implications of empirically based studies. These results provide empirical guidance regarding adequate samples sizes for saturation when using interviews and focus group discussions, which can be useful when developing qualitative research proposals. The majority of empirically based studies in our review had a homogenous study population and focused research objectives, so these results cannot be confidently extrapolated to studies using different types of samples or broader goals. Therefore, we recommend using these results as a starting point to identify a potential range of interviews or focus groups then refining the sample size by considering the study characteristics (e.g., study goals, nature and complexity of phenomenon studied, instrument structure, sampling strategy, stratification of sample, researcher's experience in qualitative research, saturation goal, and degree of saturation sought) (Baker and Edwards, 2012; Galvin, 2015; Morse, 1995; see Hennink et al., 2017 for fuller discussion on using study parameters to estimate saturation). These considerations will not only lead to a more tailored sample size for each particular study but also provide clearer justification for the proposed sample size, thereby adding rigor.
또한, 본 연구 결과는 연구자들에게 질적 표본 크기가 '너무 작다'는 일반적인 비판을 반박할 수 있는 강력한 경험적 증거를 제공하며, 이는 일반적으로 이러한 주장에 대한 근거가 제시되지 않음에도 불구하고 비효율적이라는 것을 암시합니다. 우리의 연구 결과는 '작은' 표본 크기가 질적 연구에 효과적이라는 것을 입증하고, 질적 연구에서 적절한 표본 크기의 기준이 되는 포화 상태에 도달할 수 있기 때문에 왜 효과적인지 그 이유를 보여줄 수 있습니다. 또한, 다양한 질적 방법에서 포화 상태에 도달할 수 있는 다양한 표본 크기를 제공함(예: 9~17명의 인터뷰 또는 4~8명의 포커스 그룹)으로써 '작은' 표본이 실제로 어떤 것인지 보여줍니다. [질적 연구를 위한 표본 크기에 대한 일반적인 조언]은 일반적으로 이보다 더 큰 표본 크기를 제안하기 때문에 이 점이 중요합니다.
Our results also provide researchers with strong empirical evidence to refute the common critique that qualitative sample sizes are ‘too small’, implying that they are ineffective, although no evidence is usually given for these claims. Our results can be used to demonstrate that ‘small’ sample sizes are effective for qualitative research and to show why they are effective – because they are able to reach saturation, the long-held benchmark for an adequate sample size in qualitative research. Furthermore, our results show what a ‘small’ sample actually is, by providing a range of sample sizes for saturation in different qualitative methods (e.g., 9–17 interviews or 4–8 focus groups). This is important because general advice on sample sizes for qualitative research usually suggest higher sample sizes than this.
질적 연구 방법론에 관한 [교과서를 검토]한 결과, 5~60개의 인터뷰(Guest 외, 2006; Constantinou 외, 2017; Hagaman and Wutich, 2017), 2~40개의 포커스 그룹(Guest 외, 2016) 등 표본 크기 권장 사항이 매우 다양하다는 것을 알 수 있었습니다. 더 중요한 것은 이러한 권장 사항 중 어느 것도 경험적 근거가 없다는 것입니다. 적절한 주의 사항과 함께 근거에 기반한 표본 크기 권장 사항을 제공하는 것이 중요합니다.
- 필요 이상으로 큰 질적 표본은 연구비 낭비, 연구 참여자의 과도한 부담, 데이터 낭비 등 윤리적 문제를 야기하며(Carlsen and Glenton, 2011; Francis 등, 2010),
- 표본이 너무 작아 포화 상태에 도달하면 연구 결과의 타당성이 떨어집니다(Hennink 등, 2017).
따라서 본 연구 결과는 질적 연구 지침서의 가이드라인의 일부로 포함될 수 있는 포화에 대한 경험적 기반 표본 크기를 제공합니다.
Reviews of textbooks on qualitative research methodology found that sample size recommendations vary widely, for example 5–60 interviews (Guest et al., 2006; Constantinou et al., 2017; Hagaman and Wutich, 2017) and 2 to 40 focus groups (Guest et al., 2016). More importantly, none of these recommendations is empirically based. Providing evidence-based sample size recommendations, with appropriate caveats, is important.
- Qualitative samples that are larger than needed raise ethical issues, such as wasting research funds, overburdening study participants, and leading to wasted data (Carlsen and Glenton, 2011; Francis et al., 2010),
- while samples that are too small to reach saturation reduce the validity of study findings (Hennink et al., 2017).
Our results thus provide empirically based samples sizes for saturation that could be included as part of the guidelines in instructional textbooks on qualitative research.
또한 바실레이우 외(2018)는 일부 질적 연구자조차도 자신의 표본 크기가 '작다'고 표현했지만, 이는 "후회나 사과 담론에 따른 한계로 해석된다"(12쪽)는 점을 발견했습니다. 이러한 저자들은 [실증주의를 지향하는 독자들의 우려]에 부응하기 위해 글을 썼을지 모르지만, 질적 근거를 들어 '작은' 표본을 옹호하는 사람은 거의 없었습니다. 우리는 연구자들이 [실증주의 패러다임의 (대부분 부적절한) 우려와 수치적 기대에 대응하기]보다는 [질적 연구의 원칙을 사용하여 표본 크기를 보다 자신 있게 정당화할 수 있도록 우리의 결과를 반영할 것을 권장]합니다. 질적 연구의 표본 크기는 데이터의 적절성에 따라 결정되므로, 효과적인 표본 크기는 숫자(n)보다는 연구 대상 현상에 대한 풍부하고 미묘한 설명을 제공할 수 있는 데이터의 능력에 더 중점을 둡니다. 궁극적으로, 질적 연구를 위한 표본 크기를 결정하고 정당화하는 것은 포화에 영향을 미치는 연구 특성과 분리할 수 없습니다. 우리의 결과는 "소규모 표본에서 엄격하게 수집된 질적 데이터는 사람들의 경험의 전체 차원을 실질적으로 나타낼 수 있다"(Young and Casey, 2019, 12쪽)는 다른 연구 결과를 반영하므로 질적 연구의 엄격성을 평가할 때 이를 한계로 보거나 제시해서는 안 됩니다.
Furthermore, Vasileiou et al. (2018) found that even some qualitative researchers characterized their own sample size as ‘small’, but this was “construed as a limitation couched in a discourse of regret or apology” (p. 12). Although these authors may be writing to the concerns of more positivist-oriented readers, few defended their ‘small’ sample on qualitative grounds. We encourage researchers to reflect on our results to more confidently justify their sample sizes using the principles of qualitative research rather than responding to the (mostly inappropriate) concerns of a more dominant positivist paradigm and their numerical expectations. Sample sizes in qualitative research are guided by data adequacy, so an effective sample size is less about numbers (n's) and more about the ability of data to provide a rich and nuanced account of the phenomenon studied. Ultimately, determining and justifying sample sizes for qualitative research cannot be detached from the study characteristics that influence saturation. Our results echo others, that “rigorously collected qualitative data from small samples can substantially represent the full dimensionality of people's experiences” (Young and Casey, 2019, p.12) and therefore should not be viewed or presented as a limitation when evaluating the rigor of qualitative research.
또한, 이번 연구 결과는 [질적 연구 검토자]에게 [포화에 맞는 효과적인 표본 크기에 대한 경험적 지침]을 제공합니다. 이는 '작은' 표본 크기에 대해 질적 연구를 비판하는 일상적인 관행에 다시 초점을 맞추는 데 도움이 될 수 있으며, 검토자는 대신 연구자에게 다음과 같은 질문을 통해 표본 크기에 대한 보다 명확한 근거를 제시하도록 요청할 수 있습니다:
- "귀하와 같은 동질적인 연구 모집단에서는 일반적으로 25명 미만으로 포화 상태에 도달할 수 있는데, 왜 40명의 인터뷰 표본을 사용했습니까?"라고 질문할 수 있습니다.
우리는 일반적으로 질적 연구에 효과적인 표본 크기를 결정할 때 수치적 지침만을 사용하는 것을 지지하지는 않지만, 이러한 유형의 질문은 포화에 대한 이용 가능한 경험적 증거를 사용하여 연구자가 표본 크기를 정당화하고 각 개별 연구의 특성을 보다 투명하게 사용하도록 도전하는 보다 정보에 입각한 비판을 반영합니다. 따라서 우리는 질적 연구자들이 표본 크기에 대해 보다 충분한 근거를 제시하고, 질적 연구 검토자들이 이러한 결과를 적용하여 질적 연구에 대한 표본 크기에 대해 보다 효과적인 비평을 제공할 것을 촉구합니다. 이를 통해 질적 연구에 대한 보고와 비평의 질을 개선하고 '작은' 표본 크기에 대한 근거 없는 비판에서 벗어날 수 있습니다.
Our results also provide empirical guidance on effective sample sizes for saturation for reviewers of qualitative research. This may help to refocus the routine practice of criticizing qualitative research for ‘small’ sample sizes so that reviewers may instead ask researchers to provide more explicit justifications for their sample size by asking, for example:
- “why do you have a sample of 40 interviews, when saturation can typically be reached in less than 25 with a homogenous study population such as yours?”
Although, we generally do not support using only numerical guidance in determining an effective sample size for qualitative research, these types of questions reflect a more informed critique that uses available empirical evidence on saturation to challenge researchers to be more transparent in justifying their sample sizes and using the characteristics of each individual study to do so. We therefore encourage qualitative researchers to provide fuller justifications of their sample sizes and urge reviewers of qualitative studies to apply these findings to provide more effective critiques of sample sizes for qualitative research. This may improve the quality of reporting and critiquing qualitative research and move away from often unsubstantiated critiques of ‘small’ sample sizes.
또한 이번 연구 결과는 [코드 빈도 수]와 [코드 의미 평가의 여러 변형]을 포함하여 [포화를 평가하는 5가지 접근 방식]을 종합적으로 보여줍니다. 이제 질적 연구자들은 데이터 수집 중에 포화를 평가할 수 있는 다양한 전략을 사용할 수 있습니다. 정성적 연구에 대한 수많은 검토에 따르면 포화는 종종 표본 크기를 정당화하기 위해 사용되지만, 포화를 평가하거나 결정하는 방법에 대한 투명성이 압도적으로 부족하다는 사실이 밝혀졌습니다(Carlsen and Glenton, 2011; Francis 외., 2010; Marshall 외., 2013; Vasileiou 외., 2018). 특히 포화가 질적 연구에서 품질의 지표로 환영받는다는 점을 고려할 때 이러한 투명성 부족은 우려스러운 부분입니다. 이는 포화가 연구자들에 의해 거의 근거가 없고 질적 연구 검토자들에 의해 도전받지 않는 엄격함의 외관을 제공하기 위해 "엄격함의 덮개"(Constantinou 외., 2017, 2페이지)로 사용되고 있음을 시사합니다.
Our results also synthesize five distinct approaches to assess saturation, including several variations of code frequency counts and assessing code meaning. Qualitative researchers now have an array of strategies to assess saturation during data collection. Numerous reviews of qualitative studies have found that saturation is often used to justify a sample size, but there was an overwhelming lack of transparency in how it was assessed or determined (Carlsen and Glenton, 2011; Francis et al., 2010; Marshall et al., 2013; Vasileiou et al., 2018). This lack of transparency is concerning, particularly given that saturation is hailed as an indicator of quality in qualitative research. It suggests that saturation is being used as a “mantle of rigor” (Constantinou et al., 2017, p. 2) to provide the appearance of rigor that is largely unsubstantiated by researchers and left unchallenged by reviewers of qualitative studies.
이러한 투명성 부족은 어느 정도 [포화 평가에 대한 지침이 없기 때문]일 수 있습니다. 이번 검토에서는 질적 연구자가 포화 평가 방법, 포화에 도달했는지 여부 또는 연구에서 포화가 어느 정도 달성되었는지를 보다 투명하게 보고하기 위해 사용할 수 있는 다양한 전략을 종합했습니다. 이제 연구자는 [포화를 평가하는 전략]과 [포화를 결정한 기준(예: 중지 기준, 누적 빈도 그래프, 코드 비율, 코드 의미)]을 지정할 수 있습니다. 이러한 투명성 강화는 개별 연구의 엄격성뿐만 아니라 질적 연구 전반의 품질에도 분명한 이점이 있습니다. 포화에 대한 투명성이 높아지면 연구의 재현성이 향상되고 포화를 보고하는 방법에 대한 기대치가 높아지며, '포화까지 데이터를 수집했다'와 같이 [일반적이고 근거가 없는 문구]를 사용하는 것에서 벗어날 수 있습니다.
To some extent, this lack of transparency may reflect the absence of guidance on assessing saturation. Our review has synthesized a range of strategies that can be used by qualitative researchers to become more transparent in reporting how saturation was assessed, whether it was reached, or the extent to which it was achieved in a study. Researchers can now specify a strategy for assessing saturation and the criteria on which it was determined (e.g., a stopping criterion, cumulative frequency graphs, percentage of codes, code meaning). Such greater transparency has clear benefits for the rigor of individual studies but also for the quality of qualitative research as a whole. Greater transparency regarding saturation improves reproducibility of the research and raises expectations on how to report saturation, all of which move away from using generic and unsupported statements such as ‘data were collected until saturation’.
또한 [질적 연구를 발표하는 저널]은 포화에 대한 투명한 보고를 장려하는 데 중요한 역할을 합니다. Vasileiou 등(2018)은 [표본 크기의 정당성 보고]가 [특정 학술지와 일치]한다는 사실을 발견했으며, 이는 학술지 요건이 포화 보고의 투명성 강화 규범을 장려할 수 있음을 시사합니다. 학술지 심사자는 연구자에게 다음 등의 질문을 할 수 있습니다.
- '포화를 어떻게 평가했습니까?',
- '포화에 도달했다는 것을 어떻게 알 수 있습니까? ' 또는
- '핵심 코드, 범주, 의미 등에서 어느 정도 포화 상태에 도달했습니까?'
이러한 요청은 포화에 대한 보다 투명하고 미묘하며 엄격한 보고가 필요하다는 신호입니다. 이는 단순한 체크리스트 요건을 넘어서는 것으로, 포화가 어떻게 결정되었는지에 대한 연구별 세부 정보 없이 포화에 도달했다는 모호한 보고를 지속시킬 수 있습니다.
In addition, journals publishing qualitative research play a critical role in encouraging transparent reporting of saturation. Vasileiou et al. (2018) found that reporting of sample size justifications aligned with particular academic journals, suggesting that journal requirements may encourage norms of greater transparency in reporting saturation. Journal reviewers may also encourage transparency by asking researchers, for example:
- ‘how did you assess saturation ?’,
- ‘how do you know you reached saturation ? ’ , or
- ‘to what extent was saturation reached – in core codes, categories, meaning etc.?'.
Such requests signal that more transparent, nuanced, and rigorous reporting of saturation is expected. This should go beyond simple check-list requirements, which may simply perpetuate vague reporting that saturation was reached without study-specific details on how it was determined.
본 연구에는 몇 가지 잠재적인 한계가 있습니다. 영어로 출판되고 근거 이론의 인식론적 접근 방식을 벗어난 연구만 포함했으며, 특정 질적 방법에 대한 검색어를 제한적으로 사용했지만 일반적인 방법도 포함했습니다. 이러한 기준 때문에 이미 발표된 다른 포화 테스트는 제외되었을 수 있지만, 검색 기준은 해당 주제에 대한 중요한 연구를 포괄할 수 있을 만큼 충분히 광범위하다고 생각합니다. 검토에서 확인된 논문은 압도적으로 건강 연구에 초점을 맞추고 있으며 포화에 대한 유사한 개념화를 가지고 있습니다. 따라서 연구 결과를 비교하기는 쉽지만, 포화를 다르게 개념화하는 다른 분야에는 이러한 결과를 적용하지 못할 수도 있습니다.
Our study has some potential limitations. We included only studies that were published in English and were outside the epistemological approach of grounded theory, and we used limited search terms for specific qualitative methods but included common methods. While these criteria may have excluded other published tests of saturation, we believe our search criteria were broad enough to capture a significant body of research on the topic. Articles identified in our review focus overwhelmingly on health research and have similar conceptualizations of saturation. While this makes the studies more comparable, these results may not be applicable to other disciplines that may conceptualize saturation differently.
5. 결론
5. Conclusion
포화는 질적 연구에서 표본 크기를 결정할 때 엄격함의 초석으로 간주되지만, 근거 이론을 제외하고는 포화 운영에 대한 지침이 거의 없습니다. 이 체계적 고찰에서는 질적 연구에서 포화를 경험적으로 평가한 연구, 포화를 평가하는 문서화된 접근법, 포화를 위한 표본 크기를 확인한 연구를 확인했습니다. 특히 연구 모집단이 비교적 균질하고 목표가 좁게 정의된 연구에서 좁은 범위의 인터뷰(9~17개) 또는 포커스 그룹 토론(4~8개)으로도 포화를 달성할 수 있음을 보여주는 다양한 포화 평가 접근법을 설명합니다. 체계적인 검토를 통해 포화에 대한 표본 크기를 확인했지만, 특정 매개변수가 포화에 어떤 영향을 미치는지 결정하기 위한 경험적 기반 연구는 거의 발견되지 않았습니다. 연구 목표, 연구 모집단의 특성, 사용된 샘플링 전략(예: 귀납적 샘플링과 고정 샘플링), 데이터 유형, 포화 목표 및 기타 영향과 같은 특정 매개변수가 포화에 미치는 영향에 대한 추가 연구가 필요합니다.
Saturation is considered the cornerstone of rigor in determining sample sizes in qualitative research, yet there is little guidance on its operationalization outside of grounded theory. In this systematic review, we identified studies that empirically assessed saturation in qualitative research, documented approaches to assess saturation, and identified sample sizes for saturation. We describe an array of approaches to assess saturation that demonstrate saturation can be achieved in a narrow range of interviews (9–17) or focus group discussions (4–8), particularly in studies with relatively homogenous study populations and narrowly defined objectives. Although our systematic review identified sample sizes for saturation, we found little empirically based research to determine how specific parameters influence saturation. Further research is needed on how specific parameters influence saturation, such as the study goal, nature of the study population, sampling strategy used (i.e. inductive vs fixed sampling), type of data, saturation goal, and other influences.
Sample sizes for saturation in qualitative research: A systematic review of empirical tests
PMID: 34785096
DOI: 10.1016/j.socscimed.2021.114523
Abstract
Objective: To review empirical studies that assess saturation in qualitative research in order to identify sample sizes for saturation, strategies used to assess saturation, and guidance we can draw from these studies.
Methods: We conducted a systematic review of four databases to identify studies empirically assessing sample sizes for saturation in qualitative research, supplemented by searching citing articles and reference lists.
Results: We identified 23 articles that used empirical data (n = 17) or statistical modeling (n = 6) to assess saturation. Studies using empirical data reached saturation within a narrow range of interviews (9-17) or focus group discussions (4-8), particularly those with relatively homogenous study populations and narrowly defined objectives. Most studies had a relatively homogenous study population and assessed code saturation; the few outliers (e.g., multi-country research, meta-themes, "code meaning" saturation) needed larger samples for saturation.
Conclusions: Despite varied research topics and approaches to assessing saturation, studies converged on a relatively consistent sample size for saturation for commonly used qualitative research methods. However, these findings apply to certain types of studies (e.g., those with homogenous study populations). These results provide strong empirical guidance on effective sample sizes for qualitative research, which can be used in conjunction with the characteristics of individual studies to estimate an appropriate sample size prior to data collection. This synthesis also provides an important resource for researchers, academic journals, journal reviewers, ethical review boards, and funding agencies to facilitate greater transparency in justifying and reporting sample sizes in qualitative research. Future empirical research is needed to explore how various parameters affect sample sizes for saturation.
Keywords: Focus group discussions; Interviews; Qualitative research; Sample size; Saturation.
Copyright © 2021. Published by Elsevier Ltd.
'Articles (Medical Education) > 의학교육연구(Research)' 카테고리의 다른 글
인터뷰 기반 연구에서 표본 크기 충분성의 특성화 및 정당화: 15년간 질적 건강연구의 체계적 문헌고찰(BMC Med Res Methodol. 2018) (0) | 2023.03.17 |
---|---|
코드 포화 대 의미 포화: 얼마나 많은 인터뷰가 필요한가? (Qual Health Res. 2017) (0) | 2023.03.17 |
양적연구 질문과 질적연구 질문 및 가설 작성의 실용 가이드 (J Korean Med Sci. 2022) (0) | 2023.03.04 |
주관적 평가를 측정할 때 동의-비동의 문항 사용의 재고(Res Social Adm Pharm. 2022) (0) | 2023.01.17 |
크리스마스 2022: 과학자: 크리스마스 12일째날, 통계학자가 보내주었죠(BMJ, 2022) (0) | 2023.01.15 |